Abstract
Background
We sought to compare the performance of three commercially available automated urine sediment analyzers that represent the current urine sediment analysis technology.
Methods
A total of 232 patient samples were analyzed using manual microscopy and three automated analyzers: IRIS Iq200 (Beckman Coulter, USA), UF-1000i (Sysmex, Japan), and Cobas u701 (Roche, Switzerland). We analyzed precision, linearity, carry-over, concordance rate, and agreement between the three analyzers and manual microscopy.
Results
The repeatability and within-laboratory precision showed results similar to those of previous studies. All analyzers showed excellent linearity. The carry-over rates were within 1%. The correlation coefficient (r) between the three analyzers and manual microscopy was good. Regarding red blood cell (RBC), the UF-1000i showed a better concordance rate (90.52%) with manual microscopy than the other two analyzers and the agreement was substantial for UF-1000i (κ=0.63) and IRIS Iq200 (κ=0.61). Regarding white blood cell (WBC), Cobas u701 showed the best concordance rate (96.55%) and the agreement was moderate for IRIS Iq200 (κ=0.57) and Cobas u701 (κ=0.56), and fair for UF-1000i (κ=0.47). Regarding epithelial cell (EPI), IRIS Iq200 showed the highest concordance rate (99.2%) and the agreement was moderate for IRIS Iq200 (κ=0.59) and Cobas u701 (κ=0.54), and fair for UF-1000i (κ=0.40).
초록
방법
총 232명의 환자 검체를 사용하여 IRIS Iq200 (Beckman Coulter, USA), UF-1000i (Sysmex Diagnostic, Japan), Cobas u701 (Roche Diagnostics International, Switzerland)의 세 종류의 자동화 요침사 장비와 전통적인 현미경 검사법으로 정밀도, 직선성, 상호오염도, 상관관계, 일치율(concordance rate, %) 및 일치도(kappa value)를 분석하였다.
결과
반복정밀도와 검사실내 정밀도의 변이계수는 기존 연구 결과와 유사하였다. 직선성은 우수하였으며 상호오염도는 1% 미만이었다. 세 장비와 현미경 검사법 사이의 상관관계는 좋았다. 적혈구의 경우, UF-1000i의 현미경 검사법과의 일치율은 다른 두 장비보다 더 우수하였고(90.52%), 일치도(κ)는 UF-1000i에서 0.63, IRIS Iq200에서 0.61이었다. 백혈구의 경우, Cobas u701이 현미경법과 가장 우수한 일치율(96.55%)을 보였으며, IRIS Iq200와 Cobas u701의 일치도는(κ) 각각 0.57과 0.56이었고 UF-1000i에서는 0.47이었다. 상피세포에서는 IRIS Iq200과 현미경법 사이의 일치율이 가장 높았고(99.2%), IRIS Iq200과 Cobas u701의 일치도(κ)는 각각 0.59와 0.54였으며 UF-1000i에서는 0.40이었다.
Urine analysis is one of the most commonly performed tests in clinical laboratories. Urine sediment analysis determines the presence and quantity of various urine particles, such as red blood cells (RBC), white blood cells (WBC), epithelial cells (EPI), bacteria, and fungus, among others [1]. This is helpful for the diagnosis and treatment of kidney and urinary system diseases [2] and provides clinicians with essential information on renal diseases [3, 4]. Although the manual standardized microscopic sediment test has been acknowledged as a reference method [5, 6], it is time consuming, labor intensive, and shows poor reproducibility due to the interpretation proficiency of technicians [7-9]. For this reason, automated urine sediment analyzers have begun to emerge to overcome longstanding issues [4, 10-12], and they have now become a necessity in tertiary hospitals, in Korea.
This automated testing system has reduced the labor and time required, enabling laboratories to handle many more tests and to reduce the turnaround time, while also improving precision [13, 14]. Despite the advantages offered by automated urine sediment analyzers, it seems practically impossible to completely abandon manual microscopy in the laboratory due to automated analyzer imperfections. To improve the performance of automated urine sediment analyzers, various technologies have been applied. Automated urine sediment analyzers are based on two main technologies, one of which is flow cytometry and the other is image-based analysis [14, 15]. One widely used urine sediment analyzer adopting flow cytometry technology is the UF-1000i (Sysmex Diagnostic, Kobe, Japan) and one widely used image-based urine sediment analyzer is the Cobas u701 (Roche Diagnostics International, Rotkreuz, Switzerland). Additionally, the IRIS Iq200 (Beckman Coulter, Brea, USA) has a unique system adopting image-based analysis plus staining and laminar flow technologies, used similarly as in flow cytometry [16].
In the current work, we compared the performance of these automated urine sediment analyzers representing current technologies for urine sediment analysis. Several articles evaluating these analyzers have been published; however, none of them simultaneously compared the performance of three automated urine sediment analyzers. Therefore, in this study, we compared the three automated urine sediment analyzers side-by-side.
We collected leftover patient urine samples from outpatient clinics in our hospital. A total of 232 patient samples were used. After completing the requested tests from the outpatient clinics, each sample leftover was given a new code and was divided into four tubes for the analyses. The volume of each individual urine sample required for this study was 16 mL, 10 mL of which were used for manual microscopy, and the remaining 6 mL were divided into 2 mL samples for each analyzer. All specimens were processed within 2 hours from receipt in our laboratory. This study was approved by the institutional review board of Kosin University Gospel Hospital and informed consent was exempted (KUGH 2015-06-107-001). If the volume of sample was too low to perform all experiments, we set a priority for WBC and RBC testing in this study.
A senior technician manually counted the number of RBCs, WBCs, and EPIs. A total volume of 10 mL of urine sample was centrifuged at 400×g for 5 minutes and 9 mL of supernatant were removed. The remaining sample was resuspended, and a drop of sample was placed on a slide. An 18×18 mm cover slip was applied. RBCs, WBCs, and EPIs were counted 10 to 20 times using a 400×magnification in a microscope and the mean value of each sediment was recorded. Eight concentrations of RBCs, WBCs, and EPIs were recorded: 0-1/high-power field (HPF), 2-4/HPF, 5-10/HPF, 11-15/HPF, 16-20/HPF, 21-30/HPF, 31-40/HPF, and higher than 40/HPF. The technician was blinded to the test results of any automated analyzers. The results of the manual microscopic examination were considered correct and were compared with those of the automated urine sediment analyzers.
UF-1000i is based on an automated flow cytometry system [17]. When a urine sample is introduced into the analyzer, it automatically adds stain to visualize the cellular structures of the particles. A laser beam is used for identifying each particle using forward scattered light, side scattered light, and fluorescence signals. UF-1000i converts electrical signals to scatter grams and histograms, with particles being classified to parameters such as counts of RBC, WBC, bacteria, EPI, and casts [15, 18]. The results are reported in μL and transformed into counts per HPF by multiplying a converting factor (5.5) [19]. We used three parameters: RBC count, WBC count, and EPI count.
Cobas u701 is an image-based analyzer. Digital images are obtained and evaluated using Auto Evaluation Module (AIEM) processing software after samples are transferred to a cuvette and centrifuged [20, 21]. Particles are classified as RBC, WBC, EPI, bacteria, hyaline casts, crystals, yeasts, sperm, and mucus. The results are reported in quantitative and semi-quantitative forms. RBC and WBC counts from Cobas u701 were set to recalculate particles in the images to achieve particle number per HPF [22]. The instrument was operated, and reagents were used according to the manufacturer’s instructions.
Inside the IRIS Iq200, laminar flow is created toward the lens of a charged coupled device (CCD) camera. It uses captured images from the CCD camera and an algorithm of automated particle recognition (APR) which distinguishes particles by size, shape, texture, and contrast. Particles were automatically sorted into 12 categories including RBC, WBC, white cell clumps, EPI (squamous and non-squamous), hyaline casts, bacteria, crystals, yeast, unclassified casts, sperm, and mucus [23]. Cell counts per HPF were automatically calculated in the instrument and reported [15, 24]. The manufacturer’s instructions of the analyzer and reagent were followed. All results from the automated analyzers were provided both in counts/HPF and counts/μL.
Analyses of precision of the three automated sediment analyzers were performed only for RBC and WBC. To calculate the precision, we used the protocols from Clinical and Laboratory Standards Institute (CLSI) EP05-2A [25]. For the consistency of the test results, we used urine particle quality control (QC) materials. QC materials containing WBCs and RBCs at two concentrations (low and high) were used for two analyzers: UF II control (Sysmex Diagnostic) for UF 1000i and Bio-Rad qUAntify (Bio-Rad, Hercules, CA, USA) for Cobas u701. In IRIS Iq200, iQ Body Fluids Controls (Iris Diagnostics, Chatsworth, USA) were used as QC materials. These controls consisted of two concentrations (low and high), and the results were presented as particles/μL. The tests were performed twice per run, two runs per day, for 20 days to calculate repeatability and within-laboratory precision.
To analyze linearity, we used the protocol from CLSI EP06-A [26]. We prepared two sets of five samples with equally spaced concentrations for the WBC and RBC experiments, respectively. The high concentration samples (RBC, 1,306/μL, WBC 110/μL for Iris; RBC, 325/μL, WBC, 195/μL for Cobas; RBC, 5,174/μL, WBC 5,313/μL for UF) and low concentration samples (the supernatant of centrifuged normal urine) were used. The high and low concentrations of urines were mixed proportionally at 0:4, 1:3, 2:2, 3:1, and 4:0. We randomly measured the concentration of samples twice. The results were interpreted using both the flowchart of EP06-A, which is based on polynomial evaluation, and using a linear regression graph with the correlation coefficient (R2).
Carry-over (%) was analyzed using RBCs and WBCs for each instrument. Four tests were performed for high concentration specimens (H1, H2, H3, and H4), and then, the same tests were carried out for low concentration specimens (L1, L2, L3, and L4). The formula used for calculating the carry-over (%) is as follows:
Carry-over (%)=[L1−(L3+L4)/2]/[(H2+H3)/2−(L3+L4)/2]×100 [27]
As the results from the three automated analyzers and manual microscopy were reported as quantitative values (counts/HPF) and semi-quantitative values (grades), respectively, we needed to transform the semi-quantitative values of manual microscopy into quantitative values, for comparison. Hence, we converted the grades from manual microscopy to those of mean concentration as 0-1/HPF to 0.5/HPF, 2-4/HPF to 3/HPF, 5-10/HPF to 7.5/HPF, 11-15/HPF to 13/HPF, 16-20/HPF to 18/HPF, 21-30/HPF to 25.5/HPF, 31-40/HPF to 35.5, and higher than 40/HPF to 40/HPF. We compared the results from three main sediment counts (RBC, WBC, and EPI) among the three analyzers and manual microscopy. To analyze the correlation between them, we used correlation coefficients, Passing-Bablok regression, and Bland-Altman analysis for slope, intercept, bias, and 95% limits of agreement. We calculated the concordance rate and kappa values for the agreements between the methods.
For the agreement between semi-quantitative results of the three analyzers and manual microscopy, analyzer quantitative results were assigned to the categories from manual microscopic methods. The results of RBC, WBC, and EPI between methods were considered concordant if they were within one grade of difference.
For precision, the linearity test using flow chart of CLSI EP06-A [26] was analyzed using Analyze-it version 5.11 (Analyze-it Software Ltd., Leeds, UK). This software can evaluate polynomial methods for linearity by automatically analyzing polynomial regression for different order polynomials, finding the best fit regression model, and showing allowable nonlinearity. Passing-Bablok regression and Bland-Altman analysis were also analyzed using Analyze-it. The remaining statistical analyses were performed using SPSS v.25 (IBM Corp., Armonk, NY, USA). Kolmogorov-Smirnov test was performed to determine the normality of the results, and all results were confirmed to have normal distributions. Spearmen’s correlation was used for the correlation between methods and weighted kappa was used for the agreements. Kappa values were interpreted as follows: 0.00-0.20 for slight agreement; 0.21-0.40 for fair agreement; 0.41-0.60 for moderate agreement; 0.61-0.80 for substantial agreement; and 0.81-1.00 for almost perfect agreement [28]. The differences were considered statistically significant at a P<0.05. Microsoft Excel (Microsoft Corporation, Redmond, WA, USA) was also used to draw the carry-over and linear regression graphs.
The repeatability and within-laboratory precision of UF-1000i and Cobas u701 for RBC and WBC are shown in Table 1. The repeatability of the low concentration material of RBC and WBC counts in Cobas u701 are not presented because the mean values were calculated using negative control material, which is near zero. The CVs in samples of low and high concentration materials ranged from 5.1% to 6.6% and from 1.9% to 11.9%, respectively.
The results of IRIS Iq200, which used particle QC material, are also presented in Table 1. The CVs ranged from 40.7% to 50.7% in samples of low concentration and from 4.5% to 5.4% in samples of high concentration, respectively.
All automated analyzers showed excellent linearity within each analytical measurement range in the linear regression plots shown in Fig. 1. RBC counts ranged up to 1,315.5/μL, 324/μL, and 5,174.3/μL for IRIS Iq200, Cobas u701, and UF-1000i, respectively. WBC counts ranged up to 110/μL, 195.0/μL, and 5,313.0/μL, respectively. As analyzed using the flowchart of CLSI EP06-A, no 2nd or 3rd order polynomial fit was statistically better than a linear fit at the 5% significance concentration, for all automated analyzers.
The carry-over rates (%) of all results were within 1%, which is acceptable. Regarding IRIS Iq200, there was 0% carry-over for RBC and WBC. Carry-over rates were 0.02% for RBC and 0.65% for WBC in Cobas 650. UF-1000i presented 0.08% for RBC and 0% for WBC.
A total of 232 samples were tested for analyses of WBC and RBC, and 125 samples were used for EPI. The results of Passing-Bablok regression and Bland-Altman analysis of the RBC, WBC, and EPI values compared between the automated analyzers and manual microscopy are shown in Table 2. In Passing-Bablok regression, the slopes between the IRIS Iq200, Cobas u701, and UF-1000i and manual microscopy for WBC were 1.000, 1.050, and 1.229, respectively. For RBC, the IRIS Iq200, Cobas u701, and UF-1000i had slopes of 1.000, 1.024, and 1.007, respectively. The slopes for EPI were 1.000, 1.000, and 1.510 using the IRIS Iq200, Cobas u701, and UF-1000i, respectively. The correlation coefficient values for RBC, WBC, and EPI between IRIS Iq200 and manual microscopy were 0.881, 0.793, and 0.879, respectively (Table 2). Analogous correlation coefficient values in Cobas u701 were 0.857, 0.820, and 0.815, respectively. In UF-1000i, the values were 0.868, 0.782, and 0.797, respectively. IRIS Iq200 and Cobas u701 showed a lower cell counts tendency than manual microscopy, while UF-1000i cell counts were higher than when using the manual method.
In pairwise concordance for RBC, WBC, and EPI, the concordance rate within one grade of difference was analyzed. For RBC (Table 3, 4), UF-1000i showed a better concordance rate with the manual method than the other two analyzers (90.52%) and the agreement was substantial in UF-1000i (κ=0.63, P<0.001) and IRIS Iq200 (κ=0.61, P<0.001). For WBC (Table 3, 5), Cobas u701 showed the best concordance rate (96.55%) with manual microscopy and the agreement in IRIS Iq200 and Cobas u701 was moderate (κ=0.57 and 0.56, P<0.001, respectively) while UF-1000i showed a fair agreement (κ=0.47, P<0.001). For EPI (Table 3, 6), IRIS Iq200 showed the highest concordance rate (99.2%) and IRIS Iq200 and Cobas u701 showed a moderate agreement (κ=0.59 and 0.54, P<0.001, respectively), while UF-1000i showed a fair agreement (κ=0.40, P<0.001).
The IRIS Iq200 CV value of low concentration was 50.7% in the within-laboratory precision of our study (Table 1). In previous IRIS Iq200 studies, the within-run precision of low concentration was between 23.9% and 33.0% [23, 29, 30]. Although the absolute comparison of CV values between studies may be impossible due to the various mean values in each study, the CVs of low concentration in IRIS Iq200 were higher than those of other previous studies. On the other hand, the low concentration CVs in UF1000i were relatively low (5.3%). We couldn’t draw a conclusion on the superiority because the mean value of low concentration was higher than that of IRIS Iq200 (41.46 vs. 3.30).
The repeatability and within-laboratory precision CVs of high concentrations in UF1000i, Cobas u701, and IRIS Iq200 in our study showed similar results to those of previous studies [14, 15, 22, 29-31]. The CVs of repeatability and within-laboratory precision using high concentration RBC and WBC in UF-1000i showed lower values compared to IRIS IQ200 and Cobas u701. Considering the concentration of WBC in Cobas u701 (mean=109.47/μL), the repeatability and within-laboratory precision CVs (11.4% and 11.9%, respectively) were much higher than UF1000i (mean, 818.92/μL; repeatability CV, 1.9%; within-laboratory precision CV, 2.0%) and IRIS IQ200 (mean, 1,083.80/μL; repeatability CV, 4.5%; within-laboratory precision CV, 5.4%). The RBC concentration measured using UF1000i (mean=202.91/μL) was lower than that measured using Cobas u701 (mean=807.30/μL), however, the CVs of repeatability and within-laboratory precision of Cobas u701 were higher (5.8% and 9.0% for Cobas u701; 2.5% and 2.5% for UF1000i, respectively). Regarding precision, UF-1000i showed a better performance than the other two analyzers.
Linearity was estimated in RBC, WBC, and EPI. All three analyzers showed satisfactory linearity in each sediment (Fig. 1, all R2 >0.99). Carry-over was negligible in RBC and WBC for all three analyzers (less than 1%). Linearity and carry-over were also considered acceptable in previous studies [15, 23].
The correlation coefficients (r) between the three analyzers and manual microscopy in WBC, RBC, and EPI were good. Regarding WBC, IRIS Iq200 was the one that better correlated with manual microscopy (r=0.881). The difference was the greatest between UF-1000i and Cobas u701 (r=0.789, Bias=-1.62). Regarding RBC, Cobas u701 was best correlated with manual microscopy (r=0.820). The difference between Cobas u701 and UF-1000i was the largest (r=0.64, Bias=-1.56). Regarding EPI, IRIS Iq200 was best correlated with manual microscopy (r=0.879). And the difference was the greatest between UF-1000i and Cobas u701 (r=0.692, Bias=-1.64). Overall, IRIS Iq200 and Cobas u701 showed a relatively good correlation with manual microscopy regarding WBC, EPI, and RBC, respectively.
In Bland-Altman analysis, IRIS Iq200 and Cobas u701 showed a lower cell count tendency than manual microscopy and the difference was lower than 1 cell/HPF. This was described in other studies as well [15, 22, 24, 32]. On the other hand, more cells were counted using UF-1000i than using manual microscopy in our study. This was also described in previous studies [13, 33]. For the UF series, a tendency toward false positives regarding RBC and WBC because of misclassification has been reported [14, 33, 34]. This false-positive tendency has also been reported for EPI [14, 35].
Several factors have been reported as error-causing components of the counting process. Incomplete centrifugation and counting variation among technicians can occur, even if processes are followed to standardized methods [1]. Sometimes, some cells can be missed in manual microscopy because of focus adjustment out of the field of view [15]. In our studies, there were occasions where ghost cells were not identified, fungus and yeast were misclassified as RBCs, and some RBC casts were misclassified as crystals or WBCs. In such cases, the discrepancies between automated analyzers and manual microscopy should be examined by technicians using manual microscopy, including pre-analytical and analytical error sources [36].
When we calculated the concordance rate between the analyzers and manual microscopy, all results showed good concordance regarding WBC, RBC, and EPI (90.52-96.55%, 87.93-90.52%, and 89.60-99.20%, respectively), similarly to previous studies [14, 15, 19]. The best concordance rates for WBC, RBC, and EPI were shown by Cobas u701 (96.55%), UF-1000i (90.52%), and IRIS Iq200 (99.20%), respectively (Table 3). However, the agreement regarding WBC in IRIS Iq200 (κ=0.57, P<0.001) was slightly better than that of Cobas u701 (κ=0.56, P<0.001) and the other agreements for RBC and EPI were good (UF-1000i: κ=0.63, P<0.001; IRIS Iq200: κ=0.59, P<0.001). The agreements regarding RBC for IRIS Iq200 and UF-1000i were substantial (κ=0.61 and 0.63, respectively), and fair and moderate agreements were shown for the other parameters (κ=0.40-0.59, P<0.001). Excellent agreement was not observed between any of the compared automated urine sediment analyzers and manual microscopy in this study. In previous studies, Enko et al. reported that Cobas u701 showed substantial agreement for WBC and a fair agreement for RBC and EPI [37], and Bartosova et al. found that IRIS Iq200 was nearly perfect regarding RBC evaluation [38]. This is not in full accordance with the results of our study, but the agreement for WBC in Cobas u701 (κ=0.56) and the agreement for RBC in IRIS Iq200 (κ=0.61) were proximately relevant to the previous studies.
The agreement between the IRIS Iq200 and Cobas u701 analyzers for WBC and EPI was good (κ=0.69 and 0.72, respectively). On the other hand, the agreement for RBC was worse than for WBC and EPI. The best RBC agreement obtained was between IRIS Iq200 and UF-1000i (κ=0.50, P<0.001). This was observed in some previous studies. The concordance regarding RBC was worse than for the other two items (78.6% vs. 90.7%-92.2%) between Cobas u701 and UF-1000i in a previous study [15], and the correlation for RBC between Cobas u701 and IRIS Iq200 was worse than for WBC (r=0.61 vs. r=0.81) [20]. However, Budak et al. reported that the concordance between UF-1000i and IRIS Iq200 was good for RBC, WBC, and EPI (96.3%, 95.3%, and 97.5%, respectively) [29]. As a whole, the concordance for WBC and EPI was the best between IRIS Iq200 and Cobas u701; however, considering the concordance for RBC, the overall performance was better correlated between IRIS Iq200 and UF-1000i, which showed a lower difference in agreement between the compared items with a relatively good concordance.
The strength of agreement analyzed using kappa statistics was relatively lower than that of the concordance rate between the methods. This situation was observed in previous studies as well [15, 16]. We agree that it was caused by the way concordance was assigned. When we calculated the concordance rate between the methods, concordance within one grade of difference was considered to be concordant. On the other hand, one grade difference counts were not considered as concordant in the agreement analysis using kappa statistics and were significantly abundant to contribute to the relatively lower agreement in our study.
Although the Cobas u701 was considered to be the closest to manual microscopy due to its slide making process, the agreement for RBC, WBC, and EPI was not significantly superior to that of other analyzers. Each analyzer has its own merits in the clinical laboratory. The Cobas u701 and UF-1000i store all images so that revision can be easily accomplished by rechecking the particle images without subsequent manual microscopy [13, 15]. Technicians need to be well trained to identify the images presented in IRIS Iq200 [23]. UF-1000i offers a scatter gram which is especially useful for RBC morphology [13], which might explain its relative superiority to other analyzers in RBC identification and, by means of machine learning technology, manual microscopic review rate has been reduced by more than before [39].
In this study, several limitations were noted. Cell counting in manual microscopy usually has been performed by two technicians to ensure proficiency; however, only one technician was involved in our study, limiting our interpretation accuracy. Since manual microscopy presented results as semi-quantitative values, we needed to reassign each value to the actual quantitative values for analysis, which may have caused some bias. Although other sediments such as bacteria, yeast, cast, crystal, sperm, and mucus were present, our study focused on RBC, WBC, and EPI. Therefore, our study is of limited value in comparing the full performance of each analyzer.
In conclusion, the overall performance of the three analyzers was in good concordance with manual microscopy. The IRIS Iq200 offered the best agreement with manual microscopy for WBC and EPI, while UF-1000i had the best agreement for RBC. The agreement ranged between fair and substantial, which is not enough for fully replacing the manual microscopy process. There is still the need to confirm the uncertain cases analyzed using automated analyzers using manual microscopy.
REFERENCES
1. Clinical and Laboratory Standards Institute. 2009. Urinalysis; Approved Guideline-Third Edition. CLSI Document GP16-A3. Clinical and Laboratory Standards Institute;Wayne, PA:
2. McPherson RA, Ben-Ezra J, et al. McPherson RA, Pincus MR, editors. 2017. Basic examination of urine. Henry's clinical diagnosis and management by laboratory methods. 23rd ed. Elsevier-Saunders;Philadelphia: p. 442.


3. Perazella MA. 2015; The urine sediment as a biomarker of kidney disease. Am J Kidney Dis. 66:748–55. DOI: 10.1053/j.ajkd.2015.02.342. PMID: 25943719. PMCID: PMC7446794.


4. Becker GJ, Garigali G, Fogazzi GB. 2016; Advances in urine microscopy. Am J Kidney Dis. 67:954–64. DOI: 10.1053/j.ajkd.2015.11.011. PMID: 26806004. PMCID: PMC7466446.


5. Manoni F, Gessoni G, Caleffi A, Alessio MG, Rosso R, Menozzi P, et al. 2013; Pediatric reference values for urine particle quantification by using automated flow cytometer: results of a multicenter study of Italian urinalysis group. Clin Biochem. 46:1820–4. DOI: 10.1016/j.clinbiochem.2013.09.005. PMID: 24051212.


6. Kouri TT, Gant VA, Fogazzi GB, Hofmann W, Hallander HO, Guder WG. 2000; Towards European urinalysis guidelines. Introduction of a project under European Confederation of Laboratory Medicine. Clin Chim Acta. 297:305–11. DOI: 10.1016/S0009-8981(00)00256-4. PMID: 10841931.
7. Du J, Xu J, Wang F, Guo Y, Zhang F, Wu W, et al. 2015; Establishment and development of the personalized criteria for microscopic review following multiple automated routine urinalysis systems. Clin Chim Acta. 444:221–8. DOI: 10.1016/j.cca.2015.02.022. PMID: 25701652.


8. Winkel P, Statland BE, Jørgensen K. 1974; Urine microscopy, an Ⅲ-defined method, examined by a multifactorial technique. Clin Chem. 20:436–9. DOI: 10.1093/clinchem/20.4.436. PMID: 4818195.
9. Chase J, Hammond J, Bilbrough G, DeNicola DB. 2018; Urine sediment examination: Potential impact of red and white blood cell counts using different sediment methods. Vet Clin Pathol. 47:608–16. DOI: 10.1111/vcp.12674. PMID: 30537173.


10. Wargotz ES, Hyde JE, Karcher DS, Hitlan JP, Wilkinson DS. 1987; Urine sediment analysis by the Yellow IRIS automated urinalysis workstation. Am J Clin Pathol. 88:746–8. PMID: 3687846.
11. Deindoerfer FH, Gangwer JR, Laird CW, Ringold RR. 1985; "The Yellow IRIS" urinalysis workstation--the first commercial application of "automated intelligent microscopy". Clin Chem. 31:1491–9. DOI: 10.1093/clinchem/31.9.1491. PMID: 4028398.


12. Roe CE, Carlson DA, Daigneault RW, Statland BE. 1986; Evaluation of the Yellow IRIS®: an automated method for urinalysis. Am J Clin Pathol. 86:661–5. DOI: 10.1093/ajcp/86.5.661. PMID: 3776920.
13. Mayo S, Acevedo D, Quiñones-Torrelo C, Canós I, Sancho M. 2008; Clinical laboratory automated urinalysis: comparison among automated microscopy, flow cytometry, two test strips analyzers, and manual microscopic examination of the urine sediments. J Clin Lab Anal. 22:262–70. DOI: 10.1002/jcla.20257. PMID: 18623125. PMCID: PMC6649239.


14. Chien TI, Kao JT, Liu HL, Lin PC, Hong JS, Hsieh HP, et al. 2007; Urine sediment examination: a comparison of automated urinalysis systems and manual microscopy. Clin Chim Acta. 384:28–34. DOI: 10.1016/j.cca.2007.05.012. PMID: 17604012. PMCID: PMC6039169.


15. Lee W, Ha JS, Ryoo NH. 2016; Comparison of the automated cobas u701 urine microscopy and UF-1000i flow cytometry systems and manual microscopy in the examination of urine sediments. J Clin Lab Anal. 30:663–71. DOI: 10.1002/jcla.21919. PMID: 26842372. PMCID: PMC6807231.
16. Cho J, Oh KJ, Jeon BC, Lee SG, Kim JH. 2019; Comparison of five automated urine sediment analyzers with manual microscopy for accurate identification of urine sediment. Clin Chem Lab Med. 57:1744–53. DOI: 10.1515/cclm-2019-0211. PMID: 31280239.


17. Wang J, Zhang Y, Xu D, Shao W, Lu Y. 2010; Evaluation of the Sysmex UF-1000i for the diagnosis of urinary tract infection. Am J Clin Pathol. 133:577–82. DOI: 10.1309/AJCP1GT2JXOCQBCZ. PMID: 20231611.


18. Manoni F, Tinello A, Fornasiero L, Hoffer P, Temporin V, Valverde S, et al. 2010; Urine particle evaluation: a comparison between the UF-1000i and quantitative microscopy. Clin Chem Lab Med. 48:1107–11. DOI: 10.1515/CCLM.2010.233. PMID: 20482296.


19. Manoni F, Fornasiero L, Ercolin M, Tinello A, Ferrian M, Hoffer P, et al. 2009; Cutoff values for bacteria and leukocytes for urine flow cytometer Sysmex UF-1000i in urinary tract infections. Diagn Microbiol Infect Dis. 65:103–7. DOI: 10.1016/j.diagmicrobio.2009.06.003. PMID: 19748419.


20. Bakan E, Ozturk N, Baygutalp NK, Polat E, Akpinar K, Dorman E, et al. 2016; Comparison of Cobas 6500 and Iris IQ200 fully-automated urine analyzers to manual urine microscopy. Biochem Med (Zagreb). 26:365–75. DOI: 10.11613/BM.2016.040. PMID: 27812305. PMCID: PMC5082210.


21. Bakan E, Bayraktutan Z, Baygutalp NK, Gul MA, Umudum FZ, Bakan N. 2018; Evaluation of the analytical performances of Cobas 6500 and Sysmex UN series automated urinalysis systems with manual microscopic particle counting. Biochem Med (Zagreb). 28:020712. DOI: 10.11613/BM.2018.020712. PMID: 30022887. PMCID: PMC6039169.


22. Wesarachkitti B, Khejonnit V, Pratumvinit B, Reesukumal K, Meepanya S, Pattanavin C, et al. 2016; Performance evaluation and comparison of the fully automated urinalysis analyzers UX-2000 and Cobas 6500. Lab Med. 47:124–33. DOI: 10.1093/labmed/lmw002. PMID: 27069030.


23. Linko S, Kouri TT, Toivonen E, Ranta PH, Chapoulaud E, Lalla M. 2006; Analytical performance of the Iris iQ200 automated urine microscopy analyzer. Clin Chim Acta. 372:54–64. DOI: 10.1016/j.cca.2006.03.015. PMID: 16696963.


24. Wah DT, Wises PK, Butch AW. 2005; Analytic performance of the iQ200 automated urine microscopy analyzer and comparison with manual counts using Fuchs-Rosenthal cell chambers. Am J Clin Pathol. 123:290–6. DOI: 10.1309/VNGU9Q5V932D74NU. PMID: 15842056.


25. Clinical and Laboratory Standards Institute. 2004. Evaluation of precision performance of quantitative measurement methods; Approved guideline. CLSI document EP05-A2. 2nd ed. Clinical and Laboratory Standards Institute;Wayne, PA:
26. Clinical and Laboratory Standards Institute. 2003. Evaluation of the linearity of quantitative measurement procedures: A statistical approach; Approved guideline. CLSI document EP06-A. Clinical and Laboratory Standards Institute;Wayne, PA:
27. 2003. Laboratory automation. Clinical chemistry: theory, analysis, correlation. 4th ed. Mosby;Baltimore: p. 294.
28. Landis JR, Koch GG. 1977; The measurement of observer agreement for categorical data. Biometrics. 33:159–74. DOI: 10.2307/2529310. PMID: 843571.


29. Budak YU, Huysal K. 2011; Comparison of three automated systems for urine chemistry and sediment analysis in routine laboratory practice. Clin Lab. 57:47–52. PMID: 21391464.
30. Cui M, Ju S, Shi Y, Jing R. 2017; Performance verification of the Iris iQ200 Sprint automated urine microscopy analyzer in a hospital routine laboratory. Clin Lab. 63:1607–12. DOI: 10.7754/Clin.Lab.2017.170318. PMID: 29035449.


31. Park J, Kim J. 2008; Evaluation of iQ200 automated urine microscopy analyzer. Korean J Lab Med. 28:267–73. DOI: 10.3343/kjlm.2008.28.4.267. PMID: 18728375. PMCID: PMC6804654.


32. Altekin E, Kadiçesme O, Akan P, Kume T, Vupa O, Ergor G, et al. 2010; New generation IQ-200 automated urine microscopy analyzer compared with KOVA cell chamber. J Clin Lab Anal. 24:67–71. DOI: 10.1002/jcla.20319. PMID: 20333768. PMCID: PMC6647716.


33. Ottiger C, Huber AR. 2003; Quantitative urine particle analysis: integrative approach for the optimal combination of automation with UF-100 and microscopic review with KOVA cell chamber. Clinl Chem. 49:617–23. DOI: 10.1373/49.4.617. PMID: 12651815.


34. Langlois MR, Delanghe JR, Steyaert SR, Everaert KC, De Buyzere ML. 1999; Automated flow cytometry compared with an automated dipstick reader for urinalysis. Clin Chem. 45:118–22. DOI: 10.1093/clinchem/45.1.118. PMID: 9895347.


35. Ben-Ezra J, Bork L, McPherson RA. 1998; Evaluation of the Sysmex UF-100 automated urinalysis analyzer. Clin Chem. 44:92–5. DOI: 10.1093/clinchem/44.1.92. PMID: 9550564.


36. Zaman Z, Fogazzi GB, Garigali G, Croci MD, Bayer G, Kránicz T. 2010; Urine sediment analysis: Analytical and diagnostic performance of sediMAX® - A new automated microscopy image-based urine sediment analyser. Clin Chima Acta. 411:147–54. DOI: 10.1016/j.cca.2009.10.018. PMID: 19861122.


37. Enko D, Stelzer I, Böckl M, Derler B, Schnedl WJ, Anderssohn P, et al. 2020; Comparison of the diagnostic performance of two automated urine sediment analyzers with manual phase-contrast microscopy. Clin Chem Lab Med. 58:268–73. DOI: 10.1515/cclm-2019-0919. PMID: 31605578.


38. Bartosova K, Kubicek Z, Franekova J, Louzensky G, Lavrikova P, Jabor A. 2016; Analysis of four automated urinalysis systems compared to reference methods. Clin Lab. 62:2115–23. DOI: 10.7754/Clin.Lab.2016.160316. PMID: 28164659.


39. Cao Y, Cheng M, Hu C. 2012; UrineCART, a machine learning method for establishment of review rules based on UF-1000i flow cytometry and dipstick or reflectance photometer. Clin Chem Lab Med. 50:2155–61. DOI: 10.1515/cclm-2012-0272. PMID: 23093270.


FIGURES AND TABLES
Fig. 1
Linearity of RBC and WBC counts tested using IRIS Iq200 (A, RBC; B, WBC), Cobas u701 (C, RBC; D, WBC), and UF-1000i (E, RBC; F, WBC) automated analyzers.
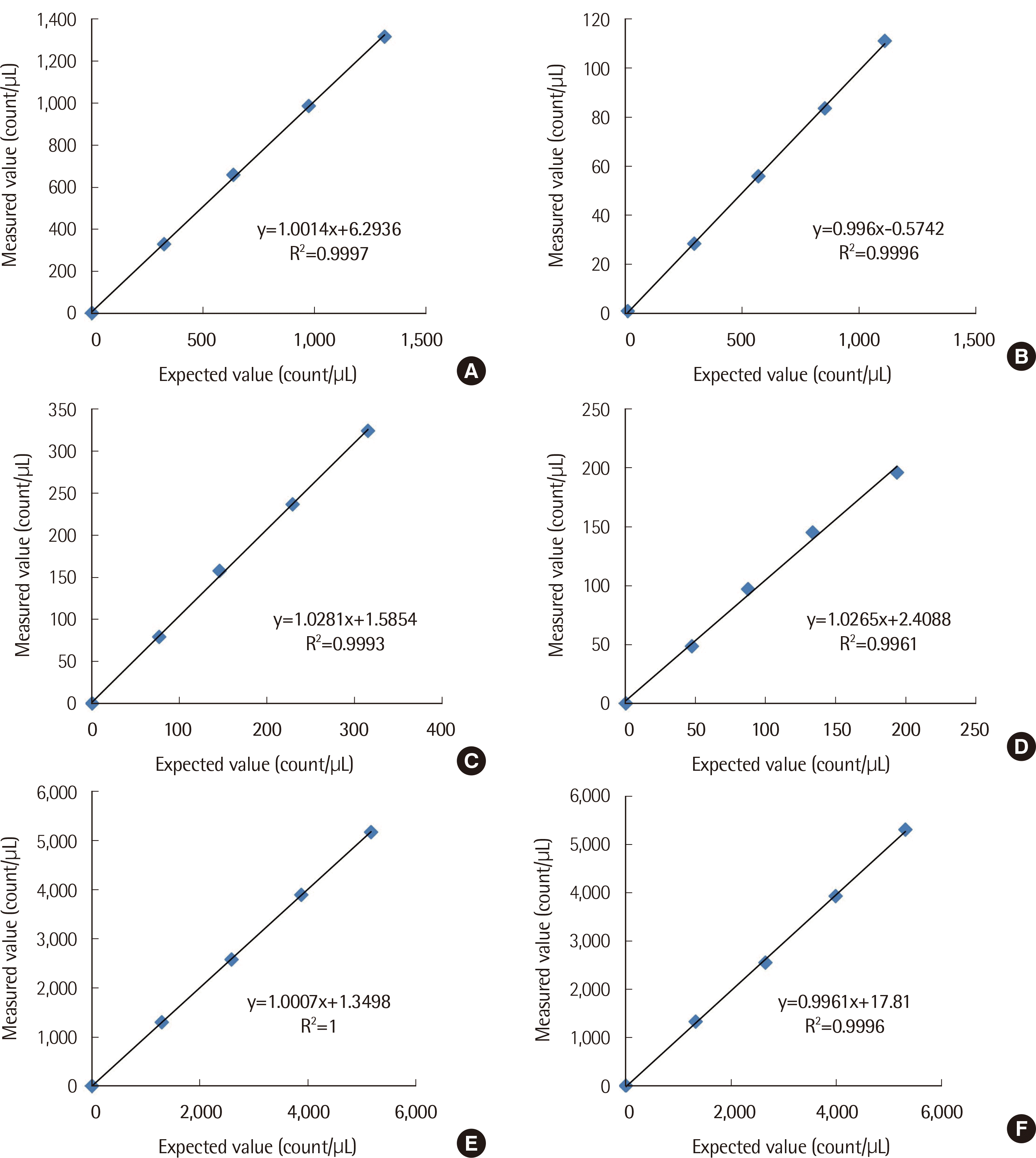
Table 1
Repeatability and within-laboratory precision of Cobas u701, IRIS Iq200, and UF-1000i analyzers in counting various cell types
Instruments | Sample | Repeatability | Within-laboratory precision | ||||||
---|---|---|---|---|---|---|---|---|---|
|
|
||||||||
Low | High | Low | High | ||||||
|
|
|
|
||||||
Mean±SD (counts/μL) | CV (%) | Mean±SD (counts/μL) | CV (%) | Mean±SD (counts/μL) | CV (%) | Mean±SD (counts/μL) | CV (%) | ||
IRIS Iq200 | Particle | 3.30±1.30 | 40.7 | 1,083.80±48.4 | 4.5 | 3.30±1.70 | 50.7 | 1,083.80±58.2 | 5.4 |
UF-1000i | RBC | 41.46±2.10 | 5.1 | 202.91±5.13 | 2.5 | 41.46±2.18 | 5.3 | 202.91±5.17 | 2.5 |
WBC | 41.87±2.69 | 5.3 | 818.92±15.19 | 1.9 | 41.87±2.75 | 6.6 | 818.92±16.72 | 2.0 | |
Cobas u701 | RBC | 0.00±* | * | 807.30±46.72 | 5.8 | 0.00±* | * | 807.30±72.55 | 9.0 |
WBC | 0.00±* | * | 109.47±12.49 | 11.4 | 0.00±* | * | 109.47±2.97 | 11.9 |
Table 2
Passing-Bablok, Bland-Altman, and Spearman’s correlation coefficient for WBC, RBC, and EPI of the three automated analyzers and manual microscopy
Method x | Method y | Slope | Intercept (count/HPF) | r* | Bias† (count/HPF) | Limits of agreement‡ | |
---|---|---|---|---|---|---|---|
|
|||||||
Low (count/HPF) | High (count/HPF) | ||||||
WBC | |||||||
Manual | IRIS Iq200 | 1.000 | -0.500 | 0.881 | -0.53 | -7.72 | 6.66 |
Manual | Cobas u701 | 1.050 | -0.525 | 0.857 | -0.54 | -7.74 | 6.67 |
Manual | UF-1000i | 1.229 | -0.288 | 0.868 | 1.08 | -7.96 | 10.11 |
Cobas u701 | IRIS Iq200 | 1.000 | 0.000 | 0.876 | 0.01 | -10.12 | 10.14 |
UF-1000i | IRIS Iq200 | 0.787 | -0.110 | 0.863 | -1.61 | -11.82 | 8.61 |
UF-1000i | Cobas u701 | 0.882 | -0.168 | 0.789 | -1.62 | -13.2 | 9.95 |
RBC | |||||||
MANUAL | IRIS Iq200 | 1.000 | -0.500 | 0.793 | -0.27 | -13.44 | 12.90 |
MANUAL | Cobas u701 | 1.024 | -0.512 | 0.820 | -0.82 | -12.02 | 10.39 |
MANUAL | UF-1000i | 1.007 | 0.019 | 0.782 | 0.75 | -11.37 | 12.87 |
Cobas u701 | IRIS Iq200 | 1.000 | 0.000 | 0.690 | 0.55 | -16.95 | 18.05 |
UF-1000i | IRIS Iq200 | 0.988 | -0.388 | 0.782 | -1.02 | -15.54 | 13.51 |
UF-1000i | Cobas u701 | 1.012 | -0.749 | 0.640 | -1.56 | -17.43 | 14.30 |
EPI | |||||||
MANUAL | IRIS Iq200 | 1.000 | -0.500 | 0.879 | -0.73 | -5.36 | 3.90 |
MANUAL | Cobas u701 | 1.000 | -0.500 | 0.815 | -0.94 | -6.66 | 4.78 |
MANUAL | UF-1000i | 1.510 | -0.615 | 0.797 | 0.70 | -7.33 | 8.72 |
Cobas u701 | IRIS Iq200 | 1.000 | 0.000 | 0.818 | 0.20 | -5.09 | 5.49 |
UF-1000i | IRIS Iq200 | 0.654 | -0.105 | 0.797 | -1.42 | -9.74 | 6.90 |
UF-1000i | Cobas u701 | 0.603 | -0.966 | 0.692 | -1.64 | -11.46 | 8.19 |
Table 3
Concordance rate (%) and agreement within one grade difference between three automated analyzers and manual microscopy for RBC, WBC, and EPI
Table 4
Comparison of pairwise results for RBC among three automated analyzers and manual microscopy
Table 5
Comparison of pairwise results for WBC among three automated analyzers and manual microscopy
Table 6
Comparison of pairwise results for EPI among three automated analyzers and manual microscopy