This article has been
cited by other articles in ScienceCentral.
Abstract
Background and Objectives
The objective was to compare the rate of onset of diabetes mellitus (DM) in patients with atrial fibrillation (AF) as compared with atrial flutter (AFL).
Methods
The incidence of DM through the national cohort registry in 14,014 newly diagnosed AF and 14,014 newly diagnosed AFL was investigated. Propensity score matching was used to optimize comparability between these 2 groups. Further analysis with Cox model, Kaplan-Meier methods and competing risk analysis were used to compare the incidence of DM in patients with AF and AFL.
Results
The overall incidence of diabetes was higher among cases in AF cohort (1,653 diabetes cases, 11.7%) than in AFL cohort (1,448 diabetes cases, 10.3%). The risk of diabetes was 1.17-fold greater in the AF cohort compared with that in the AFL cohort (95% confidence interval [CI], 1.09–1.25; p<0.001). After adjustment for all relevant confounding factors in the competing risk regression model, the risk of diabetes remained significantly increased 1.16-fold in the AF cohort (95% CI, 1.09–1.25; p<0.001).
Conclusions
The message of higher incidence rate of DM in patients with AF compared to those with AFL was delivered in this study.
Keywords: Atrial fibrillation, Atrial flutter, Diabetes mellitus
INTRODUCTION
In the aging society, the incidence of diabetes mellitus (DM) and atrial arrhythmia is increasing.
1)2)3)4) DM, although once was thought to be an endocrine disorder, has been well established as some kind of vascular disorder.
5)6)7) Impact of atrial arrhythmia on cardiovascular diseases was clearly documented; and the underlying mechanisms were complex, among them, atrial arrhythmia triggering vascular inflammation was one of the proposed theories.
8)9)10) Based upon current knowledge, atrial arrhythmia might be only one of several markers for an increased risk of ischemic events.
Since DM and atrial arrhythmia are likely all part of the same pattern of vascular biology and given the growing number of patients with atrial arrhythmia, it would be of importance if the data were robust enough to make a distinction between atrial fibrillation (AF) and atrial flutter (AFL), the 2 common atrial arrhythmias, for the subsequent DM risk per preventive medical point of view.
11)12)13)14)15) As such the distinction between AF and AFL is affected by the fact that management of the two conditions remains similar and many patients with AFL tend to convert to AF at some point.
15)16)
From Taiwanese large national database, data from patients with AF/AFL hospitalization between 2000 and 2013 were collected, with a population of 28,028 study participants. This is an impressive data set with long-term outcomes that are not available in randomized controlled trials. Moreover, propensity score matching was used to eliminate differences in age, sex, comorbidities in this register-based, observational study on the comparative association of AF/AFL and DM.
METHODS
Data source
This study was a secondary data analysis that adopted a sample of all beneficiaries who were retrieved from Taiwan's National Health Insurance Research Database (NHIRD).
17) NHIRD was built by the National Health Research Institute which contains 23 million National Health Insurance (NHI) enrollees. More than 20,000 medical care facilities, including hospitals, clinics, pharmacies, which represent over 93% of all healthcare facilities in Taiwan, were contracted by the NHI project. Under the universal health coverage project, virtually all healthcare services, including medical facility registries, medication prescriptions, and outpatient, inpatient, and emergency visiting data for Taiwan's general public were collected. This study investigated the risk of new onset of DM among patients with AF or AFL, using the inpatients file from NHIRD.
18)19) This study was approved by the Ethics Review Board of China Medical University (CMUH-104-REC2-115).
Sample participant
The diagnostic code of NHIRD in Taiwan is based upon the International Classification of Diseases, Ninth Clinical Modification (ICD-9-CM). We selected a cohort of AF patients with ICD-9-CM code 427.31 who had an inpatient visit and a comparable cohort of patients with AFL (ICD-9-CM code 427.32). The index date was defined as the earliest time of a diagnose of either AF or AFL. Both cohorts were followed until the diagnosis of diabetes, withdrawal from this program, death or at the end of the study period (December 31, 2013), whichever came first. We also excluded those with a diagnosis of diabetes before the entry time of the study. Finally, we used propensity score matching to select 14,014 patients with AF and 1-fold corresponding amount of AFL cohort by age of index date, sex, comorbidities and index year.
Outcome and comorbidity
The end point in this study was newly diagnosed diabetes (ICD-9-CM code 250.x0 and 250.x2). We also analyzed diabetes-associated comorbidities, including abnormal liver function, hypertension, hyperlipidemia, coronary artery disease (CAD), congestive heart failure (CHF), chronic obstructive pulmonary disease (COPD), autoimmune disease, gout, peripheral arterial occlusion disease (PAOD), chronic kidney disease (CKD), and stroke. Comorbidities were identified before the index date.
Statistical analysis
Propensity score matching was used to optimize the comparability between 2 cohorts. The propensity score was estimated for each patient by a non-parsimonious multivariable logistic regression, with receipt of disease status as the dependent variable. Age, sex, comorbidities and index year were independent variables for matching between these 2 cohorts. Demographic characteristics and the prevalence of comorbidities were compared by χ2 test and t-test between the two cohorts. Incidence density of diabetes according to person-years in each cohort were calculated. Multivariate models were simultaneously adjusted for covariables found significantly associated with diabetes in the univariable models. The Cox model was used to calculate the adjusted hazard ratios (HRs) and 95% confidence intervals (CIs) to compare the risk of diabetes in the AF cohort relative to the AFL cohort. The Fine and Gray model, which extends the univariate and multivariate Cox proportional-hazard regression model, were used to estimate the subhazard ratios (SHRs) of diabetes by considering death as a competing risk. Cumulative incidence curves of diabetes for AF cohort and AFL cohort were assessed using Kaplan-Meier analysis, and the differences between the cohorts were evaluated by the log-rank test. All analyses were performed using SAS software (version 9.4 for windows; SAS Institute, Cary, NC, USA). A 2-tailed p value < 0.05 was considered to indicate the statistical significance.
RESULTS
After propensity score matching, we identified 14,014 patients with AF and an identical number with AFL, with arrhythmias diagnosed between January 1, 2000 and December 31, 2012. The matched pairs were similar with regard to all covariates which including age, sex, abnormal liver function, hypertension, hyperlipidemia, CAD, CHF, COPD, autoimmune disease, gout, PAOD, CKD, and stroke. (
Table 1)
Table 1
Baseline characteristics of hospitalized patients, by the presence of with AF and with AFL, and after matching for propensity score
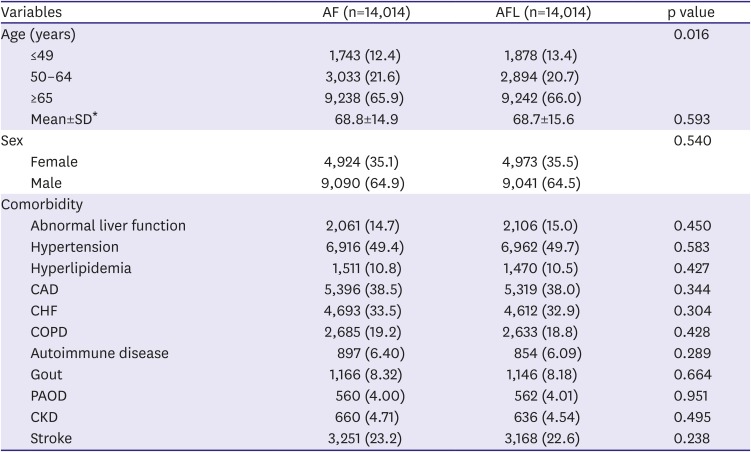
Variables |
AF (n=14,014) |
AFL (n=14,014) |
p value |
Age (years) |
|
|
0.016 |
|
≤49 |
1,743 (12.4) |
1,878 (13.4) |
|
50–64 |
3,033 (21.6) |
2,894 (20.7) |
|
≥65 |
9,238 (65.9) |
9,242 (66.0) |
|
Mean±SD*
|
68.8±14.9 |
68.7±15.6 |
0.593 |
Sex |
|
|
0.540 |
|
Female |
4,924 (35.1) |
4,973 (35.5) |
|
Male |
9,090 (64.9) |
9,041 (64.5) |
Comorbidity |
|
|
|
|
Abnormal liver function |
2,061 (14.7) |
2,106 (15.0) |
0.450 |
|
Hypertension |
6,916 (49.4) |
6,962 (49.7) |
0.583 |
|
Hyperlipidemia |
1,511 (10.8) |
1,470 (10.5) |
0.427 |
|
CAD |
5,396 (38.5) |
5,319 (38.0) |
0.344 |
|
CHF |
4,693 (33.5) |
4,612 (32.9) |
0.304 |
|
COPD |
2,685 (19.2) |
2,633 (18.8) |
0.428 |
|
Autoimmune disease |
897 (6.40) |
854 (6.09) |
0.289 |
|
Gout |
1,166 (8.32) |
1,146 (8.18) |
0.664 |
|
PAOD |
560 (4.00) |
562 (4.01) |
0.951 |
|
CKD |
660 (4.71) |
636 (4.54) |
0.495 |
|
Stroke |
3,251 (23.2) |
3,168 (22.6) |
0.238 |
Table 2 shows the incidence and hazard ratio of diabetes in patients with AF compared to those with AFL. The follow-up time was 4.20±3.69 years and 4.33±3.68 years in AF cohort and in AFL cohort, respectively. The overall incidence of diabetes was higher among cases in AF cohort (1,653 diabetes cases, 11.7%) than in AFL cohort (1,448 diabetes cases, 10.3%). The incidence density rate of diabetes was also higher in AF cohort than in AFL cohort (28.1 vs. 23.9 per 1,000 person-years). The risk of diabetes was 1.17-fold greater in the AF cohort compared to that in the AFL cohort (95% CI, 1.09–1.25; p<0.001). The competing risk regression model took into consideration of the risk factor of death. After adjustment for all relevant confounding factors in the competing risk regression model, the risk of diabetes remained 1.16-fold higher in the AF cohort (95% CI, 1.09–1.25; p<0.001) than in the AFL cohort.
Figure 1 shows that the cumulative incidence of diabetes was significant higher in the AF cohort than in the AFL cohort (p<0.001).
Table 2
Incidence and HR or SHR of diabetes in patients with AF compared to those with AFL

Outcome |
AF (n=14,014) |
AFL (n=14,014) |
Compared to AFL |
Event |
PY |
Rate |
Event |
PY |
Rate |
Crude HR (95% CI) |
Adjusted HR† (95% CI) |
Crude SHR (95% CI) |
Adjusted SHR§ (95% CI) |
Overall |
1,653 |
58,885 |
28.1 |
1,448 |
60,642 |
23.9 |
1.17 (1.09–1.26)*
|
1.17 (1.09–1.25)*
|
1.16 (1.08–1.25)*
|
1.16 (1.09–1.25)*
|
Figure 1
Cumulative incidence curves of diabetes mellitus for study patients with AFL (dashed line) or with AF (solid line).
AF = atrial fibrillation; AFL = atrial flutter.
DISCUSSION
This study investigated the association between new onset DM with 2 common atrial arrhythmias, AF and AFL, using the database of a population-based large-scale cohort study of the inpatients enrolled in the Taiwan NHI program. The major advantage of this study is clearly that using a nation-wide database there is little bias in terms of prevalence which is usually present in studies performed in tertiary centers. Moreover, sample size was large with 14,014 AF patients and 14,014 AFL patients, and AF has a stronger association than the AFL with regard to new onset DM was concluded.
Atrial arrhythmia is a heterogeneous disorder, and there are certain differences between patient populations with AF and AFL involvement of the pathological process.
15)16) Given the increasing burden of DM for major morbidity and mortality, it is of clinical relevance to establish the relationship between DM and 2 common atrial arrhythmias. While baseline characteristic might modify the association between AF/AFL and incident DM, both cohorts were propensity scoring matching with the covariates considering risk component of DM to alleviate the concern about the strength of each covariate to predict the incident DM and the trend of DM risk increase in these two atrial arrhythmias is evident from this analysis. Although the analysis with large-scale national data enables to detect the risk differences among each stratum with enough power, and the difference is statistically significant, the actual difference is considered as modest since the adjusted HR and adjusted SHR were 1.17 and 1,16, respectively.
DM is a common comorbidity in atrial arrhythmia.
11)12)13)14) Association of atrial arrhythmias and DM is given as an established fact.
11)12)13)14) DM and chronic arrhythmia management has improved such that patients are living longer before dying from the underlying heart diseases. As a result, DM appears to be one of the fastest growing causes of morbidity and mortality in clinical practice. While the difference between the 2 study cohorts might be explained by a higher degree of ventricular disease with cardio-endocrinology interactions in the AF group is a plausible theory. Further large scales mechanism-exploring studies are expected to explore this research question.
First, this research applied the method of cohort study, but the time of hospitalization in enrolled patients was widely ranged. During the study period, standard treatment or trend of intervention for AF/AFL might be possibly changed. Second, diagnoses were retrieved from only inpatient files, and many patients with asymptomatic AF/AFL and acceptable heart rate were managed as outpatients. In addition, in this study, data regarding the use of Holter electrocardiogram was also unavailable, and it should also be listed as the limitation of research since transient AF/AFL cannot be registered. Moreover, although the long follow-up period allowed accumulation of outcome events, the chance of switching exposure status (AF to AFL, or AFL to AF) and unbalanced censoring also increases with longer follow-up period, thus it may introduce bias which are not controllable by the current analysis. Finally, some of the concerns which remain with the observational study design should also be mentioned. Furthermore, the inherent limitations of national administrative database use with no information on biological data and the need to rely on ICD-9-CM codes that may be very partial might also be acknowledged.
The message of higher incidence rate of DM in patients with AF compared to those with AFL was delivered in this study.