Abstract
Purpose
Materials and Methods
Results
Figures and Tables
Fig. 1
Specific genetic alteration counts of each patient. A minimum of 1 to a maximum of 13 mutations per patient were found by multi-cancer panel analysis. Blue bars show SNP and Indel mutations and orange bars show number of structural variations.
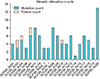
Fig. 2
Integrative analysis of cancer panel analysis of 20 patients. Each grey column represents specific data for 1 of the 20 patients in order. Genomic polymorphism of SNP/Indel mutation by truncating, in the frame, missense is noted by a color dot in a grey column with black, brown, and green. Structural variation was found in 5 patients annotated by purple color; the most common finding was ERG: TMPRSS2 fusion. No CNV amplification was found by our targeted next-generation sequencing (NGS) panel. This oncoprint was obtained by use of The cBioPortal for Cancer Genomics (http://cbioportal.org) graphic visualization Genetic alteration tool.
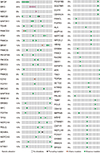
Fig. 3
Kaplan–Meier curve of BRAF mutation on biochemical recurrence with the log-rank test. Positive BRAF mutation worsened biochemical-recurrence-free survival with statistically significant difference (p=0.03).
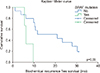
Table 2
Comparison of the incidence of top-ranked genetic alteration of this study with the TCGA database. Muse, mutect, somaticsniper, and varscan were used to determine true mutations
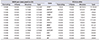
ACKNOWLEDGMENTS
Notes
AUTHORS' CONTRIBUTIONS
Research conception and design: Chang Wook Jeong and Jungyo Suh.
Data acquisition: Jungyo Suh and Seongmin Choi.
Statistical analysis: Chang Wook Jeong and Jungyo Suh.
Data analysis and interpretation: Jungyo Suh and Seongmin Choi.
Drafting of the manuscript: Jungyo Suh.
Critical revision of the manuscript: Chang Wook Jeong, Ja Hyeon Ku, and Hyeon Hoe Kim.
Obtaining funding: Chang Wook Jeong.
Administrative, technical, or material support: Seongmin Choi and Kwang Soo Kim.
Supervision: Chang Wook Jeong, Ja Hyeon Ku, Hyeon Hoe Kim, and Cheol Kwak.
Approval of the final manuscript: Cheol Kwak.
References
















































