Abstract
Purpose
Materials and Methods
Results
ACKNOWLEDGMENTS
Notes
AUTHORS' CONTRIBUTIONS:
Research conception and design: Hongzoo Park and Jeong Hyun Kim.
Data acquisition: Hongzoo Park, Sang Wook Lee, Geehyun Song, Tae Wook Kang, Jae Hung Jung, Hyun Chul Chung, Sung Jin Kim, Jong Yeon Park, Tae Young Shin, and Jeong Hyun Kim.
Data analysis and interpretation: Hongzoo Park and Jeong Hyun Kim.
Statistical analysis: Hongzoo Park.
Drafting of the manuscript: Hongzoo Park and Jeong Hyun Kim.
Receiving grant: Hongzoo Park.
Approval of final manuscript: all authors.
References

























Fig. 1
Percentage of p2PSA to free PSA (%p2PSA) and prostate health index (PHI) relative to tumor stage, Gleason sum (GS), and GS upgrading. PSA, prostate specific antigen; p2PSA, [−2]proPSA; %p2PSA, percentage of p2PSA to total PSA.
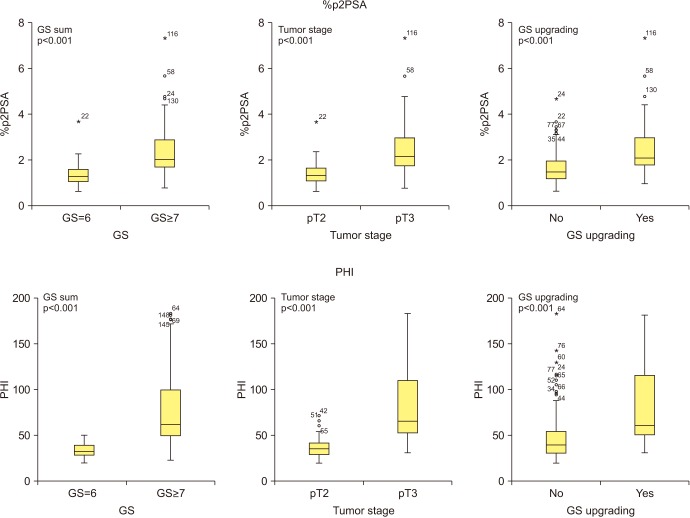
Fig. 2
Receiver operating characteristic curve predicting the probability of (A) T3 at pathologic staging, (B) Gleason sum (GS) ≥7 at pathologic grading, and (C) GS upgrading. PSA, prostate specific antigen; fPSA, free PSA; p2PSA, [−2]proPSA; %p2PSA, percentage of p2PSA to tPSA; PHI, prostate health index.

Fig. 3
Decision curve analysis of the effect of the prediction models. The net benefit is plotted against various threshold probabilities. (A) Detection of pT3 at radical prostatectomy. T-stage model 1 is a basic model that includes clinical stage, percentage of positive biopsy core, total prostate-specific antigen (tPSA), free PSA (fPSA), percent fPSA (%fPSA), biopsy Gleason sum (GS), and PSA density. T-stage model 2 is a basic model that includes all factors of model 1 plus a percentage of p2PSA to fPSA (%p2PSA). T stage model 3 is a basic model, which includes all factors of model 1 plus the Prostate Health Index (PHI). (B) Detection of pathologic GS ≥7 at radical prostatectomy. GS model 1 is a basic model that includes clinical stage, percentage of positive biopsy core, tPSA, and %fPSA. GS Model 2 is a basic model that includes all of the factors of model 1 plus %p2PSA. GS model 3 is a basic model that includes all of the factors of model 1 plus the PHI. (C) Detection of the presence of GS upgrading at radical prostatectomy. Upgrading model 1 is a basic model that includes clinical stage, percentage of positive biopsy core, tPSA, and %fPSA. Upgrading model 2 is a basic model that includes all of the factors of model 1 plus %p2PSA. Upgrading model 3 is a basic model that includes all of the factors of model 1 plus the PHI.
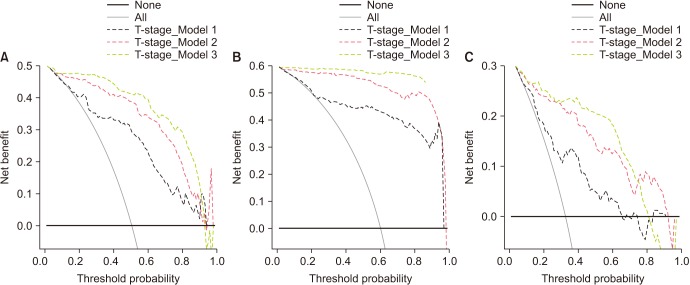
Table 1
Demographic and clinical characteristics of all study subjects
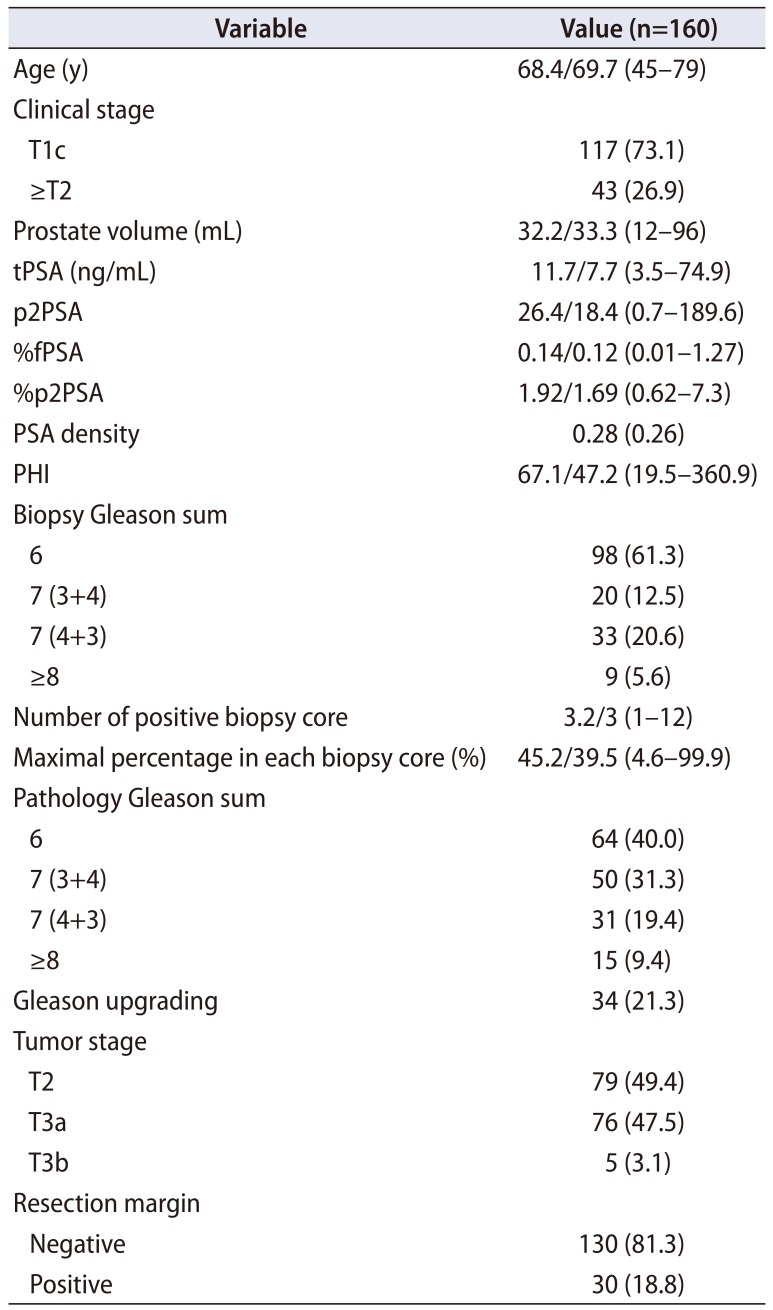
Table 2
Univariate and multivariate analysis predicting the probability of pT3 by pathologic stage

AUC, area under the curve; OR, odds ratio; PHI, prostate health index; PSA, prostate-specific antigen; tPSA, total PSA; fPSA, free PSA; %fPSA, the percentage of free PSA to total PSA; %p2PSA, the percentage of p2PSA to free PSA.
The AUC reflects the predictive values of individual variables (columns) and of the multivariate models in predicting the probability of pT3.
Table 3
Univariate and multivariate analysis predicting the probability of Gleason sum ≥7 at pathologic staging
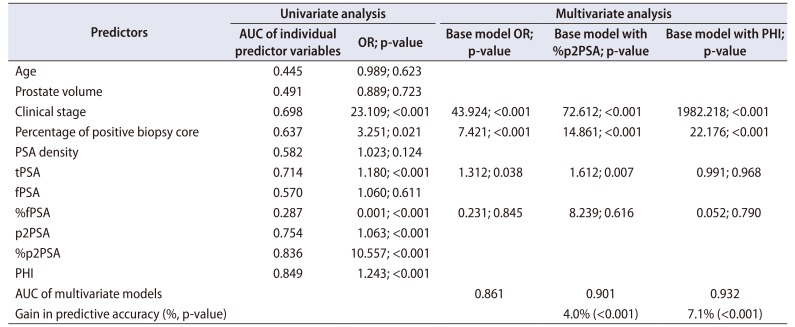
AUC, area under the curve; OR, odds ratio; PHI, prostate health index; PSA, prostate-specific antigen; tPSA, total PSA; fPSA, free PSA; %fPSA, the percentage of free PSA to total PSA; %p2PSA, the percentage of p2PSA to free PSA.
The AUC reflects the predictive values of individual variables (columns) and of the multivariate models for predicting the probability of Gleason sum ≥7.
Table 4
Univariate and multivariate analyses predicting the probability of Gleason sum upgrading
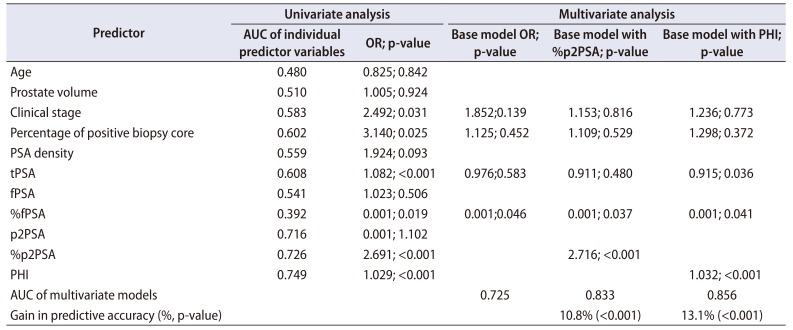
AUC, area under the curve; OR, odds ratio; PHI, Prostate Health Index; PSA, prostate-specific antigen; tPSA, total PSA; fPSA, free PSA; %fPSA, the percentage of free PSA to total PSA; %p2PSA, the percentage of p2PSA to free PSA.
The AUC reflects the predictive values of individual variables (columns) and of the multivariate models for predicting the probability of Gleason sum upgrading.
Table 5
Sensitivity and specificity at three levels of prostate health index (high sensitivity, best combination, high specificity) for prediction of pT3 disease at pathology, Gleason sum ≥7, and Gleason sum upgrading
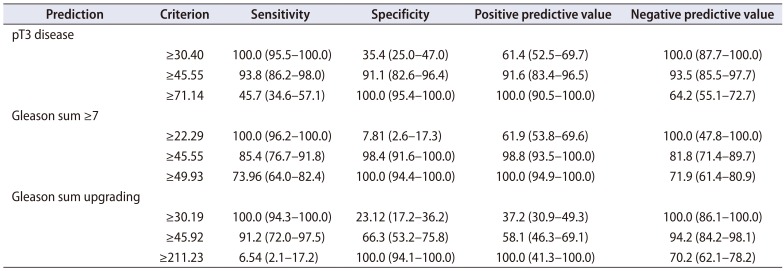