Abstract
Lung cancer is the most common cause of cancer deaths worldwide and several molecular signatures have been developed to predict survival in lung cancer. Increasing evidence suggests that proliferation and migration to promote tumor growth are associated with dysregulated ion channel expression. In this study, by analyzing high-throughput gene expression data, we identify the differentially expressed K+ channel genes in lung cancer. In total, we prioritize ten dysregulated K+ channel genes (5 up-regulated and 5 down-regulated genes, which were designated as K-10) in lung tumor tissue compared with normal tissue. A risk scoring system combined with the K-10 signature accurately predicts clinical outcome in lung cancer, which is independent of standard clinical and pathological prognostic factors including patient age, lymph node involvement, tumor size, and tumor grade. We further indicate that the K-10 potentially predicts clinical outcome in breast and colon cancers. Molecular signature discovered through K+ gene expression profiling may serve as a novel biomarker to assess the risk in lung cancer.
Ion channels are ion-permeable protein complexes in the lipid membranes. They establish and control the voltage gradient across biological membranes by gating the flow of specific small ions down their electrochemical gradient, which play important roles in diverse cellular processes including cardiac, skeletal, and smooth muscle contraction, epithelial transport of nutrients and ions, T-cell activation and pancreatic beta-cell insulin release, hormonal secretion, osmotic regulation of blood pressure and so on [12345]. Previous studies have shown that ion channels are involved in the development of various human diseases including cancers [67891011]. The roles of ion channels, especially potassium channels, in the growth of tumor cells have been well documented [121314151617]. Blocking channel activity affects the growth of certain tumors both in vitro and in vivo [131819].
Among all the voltage-gated ion channels, potassium channels (K+ channels) have attracted most of the attentions in regard to the relationship with human cancers because K+ channels constitute the largest heterogeneous family, such as voltage-gated K+ (Kv) channels, calcium-activated K+ (KCa) channels, inwardly rectifying K+ (Kir) channels, and two-pore K+ (K2p) channels, with more than eighty genes encoding membrane proteins that control membrane potential [20]. More importantly, K+ channels play a pivotal role in the proliferation, migration, and invasion of various tumor cells and have been considered as new targets for designing cancer treatment strategies [2021]. For example, Kv channels have been found to be involved in the proliferation of uterine and breast cancer cells [2223]. Similarly, the activation of KCa channels has been shown to drive tumor cell proliferation in astrocytoma and glioma [2425]. Actually, increasing evidences indicate that, instead of a single subfamily of K+ channel being present in tumor cells from tissues such as colon, prostate, and breast, a variety of K+ channels are found in these tissues [17]. For example, the expression of Kv, KCa, and K2p channels has been reported in prostate cancer cells [262728].
Lung cancer is the most common cause of cancer-related death in human worldwide [2930]. Several molecular signatures have been developed to predict survival in lung cancer [313233343536]. However, little is known regarding the role of K+ channels in promoting tumor growth and its influence on the prognosis of lung cancer compared with other cancers. In this study, we analyzed high-throughput gene expression data to identify K+ channel genes implicated in lung cancer. A K+ channel gene signature was sought that differentiates lung tumor tissues from normal ones. This molecular signature predicted clinical prognosis in human lung cancer effectively, which is independent of, but cooperative with, standard clinical and pathological prognostic factors including patient age, lymph node involvement, tumor size, and tumor grade. We also indicated that this gene signature potentially may be used to predict outcome in human breast and colon cancers.
The definition of ion channel gene for human was obtained from IUPHAR-DB [37]. In total, we collected eighty-eight K+ channel genes, including 47 Kv channels, 12 KCa channels, 15 Kir channels, and 14 K2p channels (Supplementary Table 1).
Two independent gene expression datasets on lung cancer (GSE10072 and GSE19804) were downloaded from the Gene Expression Omnibus (GEO) database. These two datasets were chosen based on the availability of both normal and tumor tissue specimens, as well as having a large number of samples available. We used these two datasets to filter out the differentially expressed genes between normal and tumor tissues.
Besides the two GEO datasets we mentioned above, we also downloaded the gene expression datasets representing human lung, breast, and colon cancers from publicly available repositories. These datasets were chosen based on the large number of samples, the availability of clinical outcome data, and the diversity of tumor types. For each tumor type, training and validation cohorts were constructed. For lung cancer, we obtained three datasets (n = 359) which were available from a single study [31]. Two datasets were combined as training cohort (n = 257) and the other one was used as validation cohort (n = 102). The dataset for breast cancer (n = 295) was available from previous study [38]. Lastly, for colon cancer, we downloaded the dataset (n = 232) from GEO database (GSE17538). In breast and colon cancer, the tumor samples were randomly separated into two parts (1/2 for training and 1/2 for validation). Clinical characteristics of the patients used for training and validation are listed in Supplementary Table 2.
For the datasets GSE10072 and GSE19804, two-tailed t-test was used to identify the genes that differentially expressed between normal and tumor tissues. Genes with adjusted p-value < 0.01 after Bonferroni procedure were deemed dysregulated. The common differentially expressed genes shared by the two datasets were saved for further analysis.
Next, we tested the power of the differentially expressed genes in predicting the clinical outcome in lung, breast, and colon cancers. For each cancer training/validation dataset, we normalized the gene expression level into the scale of [−1, 1] by probability of expression (POE) algorithm [3940] implemented in the metaArray library of the R Statistical Package (R Foundation for Statistical Computing, Vienna, Austria) [41]. Based on the gene expression and clinical outcome data from the training dataset, we can assign a Wald statistic generated by Cox proportional-hazard regression to each gene as a weight. A risk score was calculated for each patient using a linear combination of weighted gene expression as below:
Here, s is the risk score of the patient; n is the number of differentially expressed genes; wi denotes the weight of gene i; ei denotes the expression level of gene i; and µi and τi are the mean and standard deviation of the gene expression values for gene i across all samples, respectively. Patients were then divided into high-score and low-score groups with the median of the risk score as the threshold value. A high score indicated a poor outcome. The weight of each gene was fixed, based on the training groups, and then tested in the validation groups [34].
Two independent lung cancer datasets (GSE10072 and GSE19804) were obtained from GEO database. Both datasets contain the gene expression data from both normal and tumor lung tissues. For the dataset GSE10072, all the subjects (59 normal and 58 tumor tissues) were collected in the Lombardy region of Italy [42]. Affymetrix Human Genome U133A Array was used to measure the gene expression levels in this dataset. In contrast, the subjects in the dataset GSE19804 (60 normal and 60 tumor tissues) were from Taiwan [43]. All the subjects in this cohort are female. The gene expression levels were assayed by Affymetrix Human Genome U133 Plus 2.0 Array (Affymetrix, Santa Clara, CA, USA).
In the dataset GSE10072, all the K+ channel genes were represented by 97 probesets. Among these probesets, 7 probe sets representing 6 genes were found be to up-regulated in tumor tissue while 12 probesets coding for 9 genes were down-regulated in tumor tissue, at the specified significance level (Bonferroni-adjusted p < 0.01) (Supplementary Table 3). Most of these results can be reproduced in the dataset GSE19804. 5 out of 6 up-regulated K+ channel genes in the dataset GSE10072 were validated in the dataset GSE19804 (Fig. 1 and Supplementary Fig. 1). Similarly, 5 out of 9 down-regulated genes in the dataset GSE10072 were confirmed by the dataset GSE19804 (Fig. 1 and Supplementary Fig. 1). Therefore, we designated the ten common dysregulated genes (5 up-regulated and 5 down-regulated in tumor tissue) shared by both datasets as K-10 signature (Table 1). This K-10 signature accurately distinguished tumor tissue specimens from normal ones in both Italian and Taiwanese cohorts (Fig. 2).
We hypothesized that K-10 signature is predictive of tumor outcome in lung cancer patients. We constructed a risk scoring system that combined gene expression of K-10 with risk for death in the training dataset. High-score patients were defined as those having a risk score greater than or equal to the group median score. In independent validation cohorts, we tested the ability of the risk score to stratify patients into prognostic groups. We performed Kaplan-Meier survival analysis comparing the high-score and low-score groups and determined statistical significance by log-rank tests. The K-10 signature was able to identify patients with poor overall survival in lung cancer in both the training (p < 0.001) and testing (p = 0.033) cohorts (Fig. 3). This association between K-10 risk score and survival was also confirmed by univariate Cox proportional hazard analysis of overall survival. High-score patients had an increased risk for death of 1.78-fold in the training cohort (p < 0.001) and 2.08-fold in the testing cohort (p = 0.036).
We investigated the performance of the K-10 signature in comparison with clinicopathologic variables associated with prognosis in lung cancer. A multivariate Cox analysis of overall survival indicated that K-10 status remained a significant covariate in relation to the standard clinicopathologic factors in lung cancer, including patient age, lymph node status, tumor size, and tumor grade (Table 2). We next stratified the patients according to the factors significant on multivariate analysis, i.e., age, lymph node status, and tumor size, respectively. For patients with age < 65 and ≥ 65, the high-score patients had a significantly increased risk for death of 1.31-fold (p = 0.013) and 1.30-fold (p = 0.006), respectively. For patients with and without lymph node involvement, the high-score patients had a significantly increased risk for death of 1.31-fold (p = 0.005) and 1.31-fold (p = 0.014), respectively. For patients with tumor size < T3 and ≥ T3, the high-score patients had a significantly increased risk for death of 1.25-fold (p = 0.005) and 1.41-fold (p = 0.066), respectively. Kaplan-Meier survival analysis also demonstrated a significantly reduced overall survival for high-score patients in each subset grouped by age, lymph node status, and tumor size (Fig. 4). Taken together, these results suggest that the expression of K-10 signature is associated with clinical outcomes and is an independent prognostic factor.
We further tested the performance of K-10 in predicting clinical outcome in breast and colon cancers. A risk score based on the expression of K-10 signature was assigned to each patient with either breast or colon cancer. Kaplan-Meier survival analysis indicated that the K-10 signature was also able to identify patients with poor overall survival in breast and colon cancers in both the training (p = 0.004 for breast cancer and p = 0.016 for colon cancer) and testing (p = 0.047 in breast cancer and p = 0.007 in colon cancer) cohorts (Fig. 5). Univariate Cox proportional hazard regression on overall survival demonstrated that, in the training cohorts, high-score patients had an increased risk for death of 2.59-fold in breast cancer (p = 0.005) and 2.29-fold in colon cancer (p = 0.019). In the validation cohorts, high-score patients had an increased risk for death of 1.97-fold in breast cancer (p = 0.052) and 2.05-fold in colon cancer (p = 0.009).
We also conducted multivariate analyses using standard clinical and pathological factors available for the breast and colon cancer datasets. Cox proportional hazards regression indicated that K-10 status was still a significant covariate in colon cancer but not in breast cancer (Supplementary Table 4). In breast cancer with poor prognosis (age < 40, tumor size ≥ T2, tumor grade ≥ 2, or ER-negative), we further stratified the patients by using the K-10 risk score. Kaplan-Meier survival analysis demonstrated a significantly reduced overall survival for the high-score patients when compared to the low-score patients except for the ER-negative group (Supplementary Fig. 2). A similar analysis for the colon cancer patients with poor prognosis (age ≥ 60 or with stage 3, 4) also demonstrated an increased risk for death in the high-score patients (Supplementary Fig. 3).
The major aim of this work is to identify the differentially expressed K+ channel genes in lung cancer. Leveraging microarray expression data in two independent cohorts, we identified a gene signature comprised of K-10 for differentiating lung tumor tissue from normal ones. K-10 signature shows a considerable power to predict clinical outcome in human lung cancer. Multivariate analysis indicates that K-10 is independent of, but cooperative with, standard clinical and pathological prognostic factors including patient age, lymph node involvement, tumor size, and tumor grade. Further analysis suggests that K-10 is also potential to serve as a novel biomarker to assess the risk in breast and colon cancers.
The differentially expressed K+ channel genes were first derived from the Italian lung cancer cohort. At this step, we in total identified 15 dysregulated genes between normal and tumor tissue. We next validated these genes in the Taiwanese female cohort. Despite racial and gender difference, we found 10 common dysregulated genes shared by the two groups. Heatmap of expression level of these genes demonstrates that K-10 signature clearly separates the tumor samples from the normal ones in both datasets, which suggests that K-10 signature is not likely to be racial and gender specific.
These findings suggest that K+ channel is a mediator of tumor growth and progression. K+ channels participate in the regulation of membrane potential and electrochemical driving force for Ca2+ entry and both mechanisms are associated with mitotic cell cycling, proliferation, cell volume and development of cancer [444546]. Previous studies have described that alteration in expression and function of diverse types of K+ channels, including KCa, Kv, and K2p, in colon, breast, and pancreatic cancers [474849]. Kv channels are widely implicated in the development of different tumors [5051]. Specifically, we found that KCNA5, KCNAB1, and KCNAB2 were downregulated in lung tumor tissue. KCa channels are gated by calcium and contribute to cell proliferation by change of intracellular calcium during tumor cell migration [4852]. Based on our K-10 signature, both KCNMB3 and KCNN4 among KCa channels were upregulated in lung tumor tissue. K2p channels are background channels that open at rest and affect membrane potential, which also play a critical role in both cell apoptosis and tumorigenesis [4953]. We also found that altered expression of K2p channels in lung tumor tissue, in particular, KCNK1, KCNK12 , and KCNK5, were overexpressed in tumor tissue, while KCNK3 was down-regulated.
The mutation of channels is also critical factor for functional dysregulation of K+ channels. For example, insertion/deletion of 12 nucleotides in the 5' untranslated region (5'UTR) of KCNK1 was identified in long QT syndrome patients [54]. The missense variants in KCNK5 have been observed among patients with Balkan-onset nephropathy [55]. Lorenz et al. [56] reported an allele association between the KCNMB3 delA750- mutant and idiopathic systemic epilepsy syndrome. Genomic DNA analysis revealed a nonsense mutation in KCNA5 in patients with idiopathic atrial fibrillation, and two mutations, G182R and E211D, localized to the KCNA5, were identified in idiopathic pulmonary arterial hypertension (IPAH) patients [5758]. Moreover, homozygous mutations of KCNK3 have been identified in PAH patients [59]. The finding in our study, however, the mutations of K+ channels are not expected to correspond to the K-10 signature genes.
Molecular signatures discovered through gene expression profiling have been shown to add prognostic value to clinical and pathological findings in several human cancers [313233343536]. There is also accumulated evidence indicating that differentially expression of K+ channels have correlated with tumorigenesis in lung, breast and colon cancer pathology. Previous reports suggesting that K2p channel may be novel molecular-markers with differential expression related to breast cancer. For example, KCNK5, KCNK9, and KCNK12 were highly expressed, and KCNK6 and KCNK15 were down-regulated in breast cancer [60]. The dysregulation of KCNA5 has also identified in colorectal cancer [51] and breast cancer [61]. Similar to our results, increased KCNN4 expression was identified with aggressive features of lung carcinoma [62]. However, four genes among our K-10 signature, KCNMB3, KCNAB1, KCNAB2 , and KCNJ8 , have been less researched, with only few studies related to cancer [63].
In this study, we analyzed the gene expression profiles in human lung cancer and developed gene signature to predict cancer. However, the additional molecular studies are required to validate K-10 signature in human lung cancer. Identifying prognostic variables that work cooperatively with known factors may improve the identification of patients at higher risk for relapse and death.
Figures and Tables
Fig. 1
Distribution of expression level for the K-10 signature in the Italian cohort (GSE10072).
The 10 dysregulated genes were verified in the Taiwanese cohort (GSE19804), among which 5 genes (6 probe sets, the upper row) were up-regulated while 5 genes (7 probe sets, the lower row) were down-regulated in tumor tissue. Each panel was labelled with the corresponding gene symbol and probeset ID. Y-axis: log2 transformed expression level. The solid line depicts the mean of expression value for normal or tumor tissue. The dot line indicates the overall mean of expression value across all individual samples.
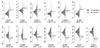
Fig. 2
Heatmap of expression level for the K-10 signature.
(A) Heatmap of expression level for the K-10 signature in the Italian cohort (GSE10072). 6 probe sets represent 5 up-regulated genes and 7 probe sets represent 5 down-regulated genes. Each row was labelled with the corresponding gene symbol and probe set ID. “++”: tumor tissue; “−”: normal tissue. (B) Heatmap of expression level for the K-10 signature in the Taiwanese cohort (GSE19804). 15 probe sets encoding 5 up-regulated and 5 down-regulated genes were displayed. Each row was labelled with the corresponding gene symbol and probe set ID. “++”: tumor tissue; “−”: normal tissue.
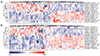
Fig. 3
Kaplan-Meier curves for the patients from the training and validation cohorts.
The expression of K-10 signature predicts poor clinical outcome in lung cancer. High-score patients were defined as those having a K-10 risk score greater than or equal to the group median score. p-value were calculated by log-rank tests for the differences in overall survival between the high-score and low-score groups.
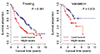
Fig. 4
Kaplan-Meier survival curves of the lung cancer patients grouped by clinicopathologic factors.
K-10 is independent from other clinicopathologic factors in lung cancer. (A) Patients were stratified by age; (B) Patients were stratified by lymph node status; and (C) Patients were stratified by tumor size. High-score patients were defined as those having a K-10 risk score greater than or equal to the group median score. p-value were calculated by log-rank tests for the differences in overall survival between the high-score and low-score groups.
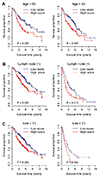
Fig. 5
Kaplan-Meier curves for the patients from the training and validation cohorts in breast and colon cancers.
The expression-of K-10 signature predicts poor clinical outcome in both (A) breast and (B) colon cancers. High-score patients were defined as those having a K-10 risk score greater than or equal to the group median score. p-values were calculated by log-rank tests for the differences in overall survival between the high-score and low-score groups.
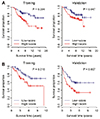
ACKNOWLEDGEMENTS
This research was supported by Basic Science Research Program through the National Research Foundation of Korea(NRF) funded by the Ministry of Education (no. NRF-2017R1D1A1B06035273, NRF-2017R1A2B4002052), and this work was supported by Korea Institute of Planning and Evaluation for Technology in Food, Agriculture and Forestry (IPET) through Animal Disease Management Technology, Development Program, funded by Ministry of Agriculture, Food and Rural Affairs (MAFRA) (119052-3).
Notes
References
1. Thorneloe KS, Nelson MT. Ion channels in smooth muscle: regulators of intracellular calcium and contractility. Can J Physiol Pharmacol. 2005; 83:215–242.


2. Krasznai Z. Ion channels in T cells: from molecular pharmacology to therapy. Arch Immunol Ther Exp (Warsz). 2005; 53:127–135.
3. Mason WT, Rawlings SR, Cobbett P, Sikdar SK, Zorec R, Akerman SN, Benham CD, Berridge MJ, Cheek T, Moreton RB. Control of secretion in anterior pituitary cells--linking ion channels, messengers and exocytosis. J Exp Biol. 1988; 139:287–316.


5. Jentsch TJ, Hübner CA, Fuhrmann JC. Ion channels: function unravelled by dysfunction. Nat Cell Biol. 2004; 6:1039–1047.


6. Lehmann-Horn F, Jurkat-Rott K. Voltage-gated ion channels and hereditary disease. Physiol Rev. 1999; 79:1317–1372.


7. Hübner CA, Jentsch TJ. Ion channel diseases. Hum Mol Genet. 2002; 11:2435–2445.
8. Curran ME. Potassium ion channels and human disease: phenotypes to drug targets? Curr Opin Biotechnol. 1998; 9:565–572.


9. Cooper EC, Jan LY. Ion channel genes and human neurological disease: recent progress, prospects, and challenges. Proc Natl Acad Sci U S A. 1999; 96:4759–4766.


10. Hanna MG, Wood NW, Kullmann DM. Ion channels and neurological disease: DNA based diagnosis is now possible, and ion channels may be important in common paroxysmal disorders. J Neurol Neurosurg Psychiatry. 1998; 65:427–431.


11. Bloch M, Ousingsawat J, Simon R, Schraml P, Gasser TC, Mihatsch MJ, Kunzelmann K, Bubendorf L. KCNMA1 gene amplification promotes tumor cell proliferation in human prostate cancer. Oncogene. 2007; 26:2525–2534.


12. Le Guennec JY, Ouadid-Ahidouch H, Soriani O, Besson P, Ahidouch A, Vandier C. Voltage-gated ion channels, new targets in anti-cancer research. Recent Pat Anticancer Drug Discov. 2007; 2:189–202.
13. Fiske JL, Fomin VP, Brown ML, Duncan RL, Sikes RA. Voltage-sensitive ion channels and cancer. Cancer Metastasis Rev. 2006; 25:493–500.


14. Roger S, Potier M, Vandier C, Besson P, Le Guennec JY. Voltage-gated sodium channels: new targets in cancer therapy? Curr Pharm Des. 2006; 12:3681–3695.


15. Pardo LA, Stühmer W. Eag1: an emerging oncological target. Cancer Res. 2008; 68:1611–1613.
16. Pardo LA, Contreras-Jurado C, Zientkowska M, Alves F, Stühmer M. Role of voltage-gated potassium channels in cancer. J Membr Biol. 2005; 205:115–124.


18. Gómez-Varela D, Zwick-Wallasch E, Knötgen H, Sánchez A, Hettmann T, Ossipov D, Weseloh R, Contreras-Jurado C, Rothe M, Stühmer W, Pardo LA. Monoclonal antibody blockade of the human Eag1 potassium channel function exerts antitumor activity. Cancer Res. 2007; 67:7343–7349.


19. Pillozzi S, Masselli M, De Lorenzo E, Accordi B, Cilia E, Crociani O, Amedei A, Veltroni M, D'Amico M, Basso G, Becchetti A, Campana D, Arcangeli A. Chemotherapy resistance in acute lymphoblastic leukemia requires hERG1 channels and is overcome by hERG1 blockers. Blood. 2011; 117:902–914.


20. Villalonga N, Ferreres JC, Argilés JM, Condom E, Felipe A. Potassium channels are a new target field in anticancer drug design. Recent Pat Anticancer Drug Discov. 2007; 2:212–223.


21. Li M, Xiong ZG. Ion channels as targets for cancer therapy. Int J Physiol Pathophysiol Pharmacol. 2011; 3:156–166.
22. Suzuki T, Takimoto K. Selective expression of HERG and Kv2 channels influences proliferation of uterine cancer cells. Int J Oncol. 2004; 25:153–159.
23. Brevet M, Haren N, Sevestre H, Merviel P, Ouadid-Ahidouch H. DNA methylation of Kv1.3 potassium channel gene promoter is associated with poorly differentiated breast adenocarcinoma. Cell Physiol Biochem. 2009; 24:25–32.


24. Basrai D, Kraft R, Bollensdorff C, Liebmann L, Benndorf K, Patt S. BK channel blockers inhibit potassium-induced proliferation of human astrocytoma cells. Neuroreport. 2002; 13:403–407.


25. Weaver AK, Liu X, Sontheimer H. Role for calcium-activated potassium channels (BK) in growth control of human malignant glioma cells. J Neurosci Res. 2004; 78:224–234.


26. Abdul M, Hoosein N. Expression and activity of potassium ion channels in human prostate cancer. Cancer Lett. 2002; 186:99–105.


27. Gessner G, Schönherr K, Soom M, Hansel A, Asim M, Baniahmad A, Derst C, Hoshi T, Heinemann SH. BKCa channels activating at resting potential without calcium in LNCaP prostate cancer cells. J Membr Biol. 2005; 208:229–240.


28. Voloshyna I, Besana A, Castillo M, Matos T, Weinstein IB, Mansukhani M, Robinson RB, Cordon-Cardo C, Feinmark SJ. TREK-1 is a novel molecular target in prostate cancer. Cancer Res. 2008; 68:1197–1203.


29. Ferlay J, Shin HR, Bray F, Forman D, Mathers C, Parkin DM. Estimates of worldwide burden of cancer in 2008: GLOBOCAN 2008. Int J Cancer. 2010; 127:2893–2917.


30. Jemal A, Bray F, Center MM, Ferlay J, Ward E, Forman D. Global cancer statistics. CA Cancer J Clin. 2011; 61:69–90.


31. Director's Challenge Consortium for the Molecular Classification of Lung Adenocarcinoma. Shedden K, Taylor JM, Enkemann SA, Tsao MS, Yeatman TJ, Gerald WL, Eschrich S, Jurisica I, Giordano TJ, Misek DE, Chang AC, Zhu CQ, Strumpf D, Hanash S, Shepherd FA, Ding K, Seymour L, Naoki K, Pennell N, Weir B, et al. Gene expression-based survival prediction in lung adenocarcinoma: a multi-site, blinded validation study. Nat Med. 2008; 14:822–827.


32. Sun Z, Wigle DA, Yang P. Non-overlapping and non-cell-type-specific gene expression signatures predict lung cancer survival. J Clin Oncol. 2008; 26:877–883.


33. Landi MT, Zhao Y, Rotunno M, Koshiol J, Liu H, Bergen AW, Rubagotti M, Goldstein AM, Linnoila I, Marincola FM, Tucker MA, Bertazzi PA, Pesatori AC, Caporaso NE, McShane LM, Wang E. MicroRNA expression differentiates histology and predicts survival of lung cancer. Clin Cancer Res. 2010; 16:430–441.


34. Pitroda SP, Zhou T, Sweis RF, Filippo M, Labay E, Beckett MA, Mauceri HJ, Liang H, Darga TE, Perakis S, Khan SA, Sutton HG, Zhang W, Khodarev NN, Garcia JG, Weichselbaum RR. Tumor endothelial inflammation predicts clinical outcome in diverse human cancers. PLoS One. 2012; 7:e46104.


35. Perumal D, Singh S, Yoder SJ, Bloom GC, Chellappan SP. A novel five gene signature derived from stem-like side population cells predicts overall and recurrence-free survival in NSCLC. PLoS One. 2012; 7:e43589.


36. Kossenkov AV, Dawany N, Evans TL, Kucharczuk JC, Albelda SM, Showe LC, Showe MK, Vachani A. Peripheral immune cell gene expression predicts survival of patients with non-small cell lung cancer. PLoS One. 2012; 7:e34392.


37. Sharman JL, Mpamhanga CP, Spedding M, Germain P, Staels B, Dacquet C, Laudet V, Harmar AJ. NC-IUPHAR. IUPHAR-DB: new receptors and tools for easy searching and visualization of pharmacological data. Nucleic Acids Res. 2011; 39(Database issue):D534–D538.


38. van de Vijver MJ, He YD, van't Veer LJ, Dai H, Hart AA, Voskuil DW, Schreiber GJ, Peterse JL, Roberts C, Marton MJ, Parrish M, Atsma D, Witteveen A, Glas A, Delahaye L, van der Velde T, Bartelink H, Rodenhuis S, Rutgers ET, Friend SH, et al. A gene-expression signature as a predictor of survival in breast cancer. N Engl J Med. 2002; 347:1999–2009.


39. Choi JK, Yu U, Kim S, Yoo OJ. Combining multiple microarray studies and modeling interstudy variation. Bioinformatics. 2003; 19 Suppl 1:i84–i90.


40. Parmigiani G, Garrett ES, Anbazhagan R, Gabrielson E. A statistical framework for expression-based molecular classification in cancer. J R Stat Soc Series B Stat Methodol. 2002; 64:717–736.


41. R_Development_Core_Team. R: A language and environment for statistical computing. Vienna: R Foundation for Statistical Computing;2005.
42. Landi MT, Dracheva T, Rotunno M, Figueroa JD, Liu H, Dasgupta A, Mann FE, Fukuoka J, Hames M, Bergen AW, Murphy SE, Yang P, Pesatori AC, Consonni D, Bertazzi PA, Wacholder S, Shih JH, Caporaso NE, Jen J. Gene expression signature of cigarette smoking and its role in lung adenocarcinoma development and survival. PLoS One. 2008; 3:e1651.


43. Lu TP, Tsai MH, Lee JM, Hsu CP, Chen PC, Lin CW, Shih JY, Yang PC, Hsiao CK, Lai LC, Chuang EY. Identification of a novel biomarker, SEMA5A, for non-small cell lung carcinoma in nonsmoking women. Cancer Epidemiol Biomarkers Prev. 2010; 19:2590–2597.


44. Breuer EK, Fukushiro-Lopes D, Dalheim A, Burnette M, Zartman J, Kaja S, Wells C, Campo L, Curtis KJ, Romero-Moreno R, Littlepage LE, Niebur GL, Hoskins K, Nishimura MI, Gentile S. Potassium channel activity controls breast cancer metastasis by affecting β-catenin signaling. Cell Death Dis. 2019; 10:180.


45. Ru Q, Tian X, Wu YX, Wu RH, Pi MS, Li CY. Voltage-gated and ATP-sensitive K+ channels are associated with cell proliferation and tumorigenesis of human glioma. Oncol Rep. 2014; 31:842–848.
46. Sundelacruz S, Levin M, Kaplan DL. Role of membrane potential in the regulation of cell proliferation and differentiation. Stem Cell Rev Rep. 2009; 5:231–246.


47. Spitzner M, Ousingsawat J, Scheidt K, Kunzelmann K, Schreiber R. Voltage-gated K+ channels support proliferation of colonic carcinoma cells. FASEB J. 2007; 21:35–44.
48. Jiang S, Zhu L, Yang J, Hu L, Gu J, Xing X, Sun Y, Zhang Z. Integrated expression profiling of potassium channels identifys KCNN4 as a prognostic biomarker of pancreatic cancer. Biochem Biophys Res Commun. 2017; 494:113–119.


49. Williams S, Bateman A, O'Kelly I. Altered expression of two-pore domain potassium (K2P) channels in cancer. PLoS One. 2013; 8:e74589.


50. Comes N, Bielanska J, Vallejo-Gracia A, Serrano-Albarrás A, Marruecos L, Gómez D, Soler C, Condom E, Ramón Y, Hernández-Losa J, Ferreres JC, Felipe A. The voltage-dependent K+ channels Kv1.3 and Kv1.5 in human cancer. Front Physiol. 2013; 4:283.
51. Hauptman N, Jevšinek Skok D, Spasovska E, Boštjančič E, Glavač D. Genes CEP55, FOXD3, FOXF2, GNAO1, GRIA4, and KCNA5 as potential diagnostic biomarkers in colorectal cancer. BMC Med Genomics. 2019; 12:54.


52. Cheng YY, Wright CM, Kirschner MB, Williams M, Sarun KH, Sytnyk V, Leshchynska I, Edelman JJ, Vallely MP, McCaughan BC, Klebe S, van Zandwijk N, Lin RC, Reid G. KCa1.1, a calcium-activated potassium channel subunit alpha 1, is targeted by miR-17-5p and modulates cell migration in malignant pleural mesothelioma. Mol Cancer. 2016; 15:44.


53. Mu D, Chen L, Zhang X, See LH, Koch CM, Yen C, Tong JJ, Spiegel L, Nguyen KC, Servoss A, Peng Y, Pei L, Marks JR, Lowe S, Hoey T, Jan LY, McCombie WR, Wigler MH, Powers S. Genomic amplification and oncogenic properties of the KCNK9 potassium channel gene. Cancer Cell. 2003; 3:297–302.


54. Iwasa H, Kurabayashi M, Nagai R, Nakamura Y, Tanaka T. Genetic variations in five genes involved in the excitement of cardiomyocytes. J Hum Genet. 2001; 46:549–552.


55. Toncheva D, Mihailova-Hristova M, Vazharova R, Staneva R, Karachanak S, Dimitrov P, Simeonov V, Ivanov S, Balabanski L, Serbezov D, Malinov M, Stefanovic V, Čukuranovič R, Polenakovic M, Jankovic-Velickovic L, Djordjevic V, Jevtovic-Stoimenov T, Plaseska-Karanfilska D, Galabov A, Djonov V, et al. NGS nominated CELA1, HSPG2, and KCNK5 as candidate genes for predisposition to Balkan endemic nephropathy. Biomed Res Int. 2014; 2014:920723.
56. Lorenz S, Heils A, Kasper JM, Sander T. Allelic association of a truncation mutation of the KCNMB3 gene with idiopathic generalized epilepsy. Am J Med Genet B Neuropsychiatr Genet. 2007; 144B:10–13.
57. Olson TM, Alekseev AE, Liu XK, Park S, Zingman LV, Bienengraeber M, Sattiraju S, Ballew JD, Jahangir A, Terzic A. Kv1.5 channelopathy due to KCNA5 loss-of-function mutation causes human atrial fibrillation. Hum Mol Genet. 2006; 15:2185–2191.
58. Remillard CV, Tigno DD, Platoshyn O, Burg ED, Brevnova EE, Conger D, Nicholson A, Rana BK, Channick RN, Rubin LJ, O'connor DT, Yuan JX. Function of Kv1.5 channels and genetic variations of KCNA5 in patients with idiopathic pulmonary arterial hypertension. Am J Physiol Cell Physiol. 2007; 292:C1837–C1853.
59. Navas Tejedor P, Tenorio Castaño J, Palomino Doza J, Arias Lajara P, Gordo Trujillo G, López Meseguer M, Román Broto A, Lapunzina Abadía P, Escribano Subía P. An homozygous mutation in KCNK3 is associated with an aggressive form of hereditary pulmonary arterial hypertension. Clin Genet. 2017; 91:453–457.
60. Dookeran KA, Zhang W, Stayner L, Argos M. Associations of two-pore domain potassium channels and triple negative breast cancer subtype in The Cancer Genome Atlas: systematic evaluation of gene expression and methylation. BMC Res Notes. 2017; 10:475.


61. Qu C, Sun J, Liu Y, Wang X, Wang L, Han C, Chen Q, Guan T, Li H, Zhang Y, Wang Y, Liu J, Zou W, Liu J. Caveolin-1 facilitated KCNA5 expression, promoting breast cancer viability. Oncol Lett. 2018; 16:4829–4838.


62. Bulk E, Ay AS, Hammadi M, Ouadid-Ahidouch H, Schelhaas S, Hascher A, Rohde C, Thoennissen NH, Wiewrodt R, Schmidt E, Marra A, Hillejan L, Jacobs AH, Klein HU, Dugas M, Berdel WE, Müller-Tidow C, Schwab A. Epigenetic dysregulation of KCa 31 channels induces poor prognosis in lung cancer. Int J Cancer. 2015; 137:1306–1317.
SUPPLEMENTARY MATERIALS
Supplementary data including three figures and four tables can be found with this article online at http://pdf.medrang.co.kr/paper/pdf/Kjpp/Kjpp2019-23-6-11-s001.pdf.
Supplementary Fig. 1
Distribution of expression level for the K-10 signature in the Taiwanese cohort (GSE19804). 5 genes (6 probe sets) were up-regulated while 5 genes (9 probe sets) were down-regulated in tumor tissue. Each panel was labelled with the corresponding gene symbol and probeset ID. Y-axis: log2 transformed expression level.
Supplementary Fig. 2.
Kaplan-Meier survival curves of the breast cancer patients grouped by clinicopathologic factors associated with poor prognosis. High-score patients were defined as those having a K-10 risk score greater than or equal to the group median score. p-values were calculated by log-rank tests for the differences in overall survival between the high-score and low-score groups.
Supplementary Fig. 3.
Kaplan-Meier survival curves of the colon cancer patients grouped by clinicopathologic factors associated with poor prognosis. High-score patients were defined as those having a K-10 risk score greater than or equal to the group median score. p-values were calculated by log-rank tests for the differences in overall survival between the high-score and low-score groups.