Helicobacter pylori is a microaerophilic gram-negative flagellate associated with gastritis, peptic ulcer disease, mucosa-associated lymphoid tissue lymphoma, and gastric cancer [
12]. Over half of the world's population, including Korean population, has been infected by
H. pylori [
34]. Although endoscopic intervention and eradication of
H. pylori has decreased the seroprevalence rate of
H. pylori infection from 66.9% (1998) to 51.0% (2015), the Korean national prevalence is similar to the average worldwide prevalence [
4].
H. pylori infection is diagnosed using both invasive methods, such as culturing, histology, and rapid urease tests, and non-invasive methods, including the urea breath test, serological tests, and stool antigen test [
56]. Non-invasive diagnostic methods are considered adequate to reflect the global infection state as they cover ≥0.5 m
2 of the gastric mucosa [
7]. Histology is regarded as the reference standard for diagnosing
H. pylori infection; however, its accuracy is affected by biopsy site, size, number of biopsy specimens, staining method, and drug history [
58]. We therefore believe that a reference standard created using statistical methods would be more accurate than the current reference standard.
Latent class analysis (LCA) can be used to evaluate diagnostic tests without reference standards, by creating a reference standard using a combination of observed and estimated results [
9]. LCA reveals hidden groups or disease states in multivariate dichotomous or categorical data [
1011]. One limitation of LCA is the underlying assumption that the tests are independent of each other, raising the possibility that there are more than two latent classes in the data.
We examined whether LCA can be used to construct a reference standard to diagnose H. pylori infection through a combination of results from histology, a stool antigen test using an immunochromatographic method (Ag-ICA, BioTracer H. pylori Ag Rapid Card; NanoEntek, Seoul, Korea), and a serum antibody test using an immunochromatographic method (Ab-ICA, BioTracer H. pylori Rapid Card; NanoEntek). We compared the performance of these tests under LCA and under the conventional method, in which histology is the reference standard.
This retrospective study was approved by the Institutional Review Board of Incheon St. Mary's Hospital, Incheon, Korea (XC15DDME0103U). Informed consent was waived as the study posed only minimal risk to the subjects. A cohort of 96 healthy subjects (median age: 63 years [range: 51–83 years]; 50 men and 46 women) undergoing a routine health check-up were enrolled from January to July 2016 at Incheon St. Mary's Hospital. Stool and remnant serum specimens after blood tests with a volume of more than 1 mL were collected in the same day that the subjects underwent endoscopy and biopsy and were stored at −20℃. The serum was isolated in the same day of blood collection. The stool was thawed for the H. pylori Ag-ICA and was then re-stored at −20℃, in case of future re-testing. H. pylori IgG, IgM, and IgA antibody tests were performed. Endoscopic and histology findings were reviewed from electronic medical records.
The H. pylori Ag-ICA was performed according to the manufacturer's instructions. The test utilizes a monoclonal antibody against H. pylori antigens. A swab was dipped into the stool specimen and then mixed with the 100 mM Tris buffer in the container. Three to five drops (120–150 µL) of the buffer-diluted stool specimen mixture were passed through a filtered tip and then placed in the specimen port of the test cassette. The appearance of a red line in the interpretation window after 10 minutes at room temperature (18–25℃) indicated a positive control band; an additional red band appeared if the specimen contained H. pylori. To determine seroprevalence, we examined anti-H. pylori IgG, IgA, and IgM antibodies, using the Ab-ICA, wherein three drops (120 µL) of serum specimen were applied to the port of the test cassette and interpreted after 10 minutes.
The positive or negative test result of biopsy, Ag-ICA, and Ab-ICA was entered in the model. Unknown disease state, that is, the subclass with and without
H. pylori infection, could be a hidden or latent class. LCA assumes that tests are conditionally independent, and the data fit the model. A two-class model with two latent variables,
H. pylori infection and
H. pylori non-infection, and a three-class model with infection, non-infection, and intermediate state (indeterminate state), were considered. Model fit was evaluated using the Bayesian information criterion (BIC), Akaike information criterion (AIC), and Pearson goodness of fit and likelihood ratios. The BIC and AIC values are unitless, and lower values are considered for model selection. For the Pearson goodness of fit and likelihood ratios, a higher
P is considered for model selection. This analysis was performed using the R package, poLCA. The sensitivity, specificity, positive predictive values, and negative predictive values and accuracies with 95% confidence interval (CI) were calculated using the randomLCA package [
121314].
The overall prevalence of H. pylori infection based on biopsy was 57.3% (55/96): 62.0% (31/50) in men and 52.1% (24/46) in women. It did not differ significantly between subjects <60 years and those ≥60 years (51.3% [19/37] vs 61.0% [36/59], P=0.868). The seroprevalence of H. pylori infection was 46.8% (45/96).
The two-class model had better values (BIC, 384.5; AIC, 366.6;
P from Pearson goodness of fit, 0.000019;
P from likelihood ratio, 0.000019) than the three-class model (BIC, 402.8; AIC, 374.6;
P from Pearson goodness of fit, 1.377×10
−10;
P from likelihood ratio, 1.379×10
−10). Accordingly, we selected the two-class model. The observed and estimated distributions based on results of three tests are listed in
Table 1. When LCA was used, histology had the highest sensitivity, followed by the Ag-ICA and Ab-ICA. Histology exhibited the highest specificity, followed by the serum antibody and stool antigen tests (
Table 2). Unexpectedly, sensitivity and specificity were higher for all tests under LCA than the conventional method, except for sensitivity of the serum antibody test.
Table 1
Tested data, expected values, assignment to latent class, and probability of the assignment class based on a two-class model
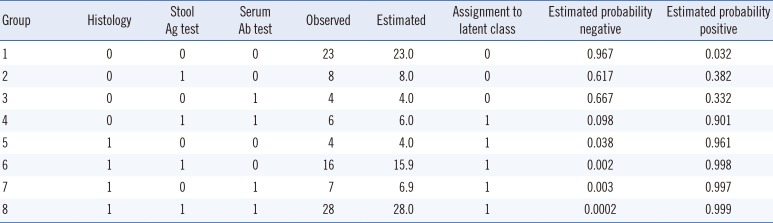
Group |
Histology |
Stool Ag test |
Serum Ab test |
Observed |
Estimated |
Assignment to latent class |
Estimated probability negative |
Estimated probability positive |
1 |
0 |
0 |
0 |
23 |
23.0 |
0 |
0.967 |
0.032 |
2 |
0 |
1 |
0 |
8 |
8.0 |
0 |
0.617 |
0.382 |
3 |
0 |
0 |
1 |
4 |
4.0 |
0 |
0.667 |
0.332 |
4 |
0 |
1 |
1 |
6 |
6.0 |
1 |
0.098 |
0.901 |
5 |
1 |
0 |
0 |
4 |
4.0 |
1 |
0.038 |
0.961 |
6 |
1 |
1 |
0 |
16 |
15.9 |
1 |
0.002 |
0.998 |
7 |
1 |
0 |
1 |
7 |
6.9 |
1 |
0.003 |
0.997 |
8 |
1 |
1 |
1 |
28 |
28.0 |
1 |
0.0002 |
0.999 |

Table 2
Performance of diagnostic tests for Helicobacter pylori infection according to latent class analysis based on a two-class model (performed without a reference standard) and the conventional method
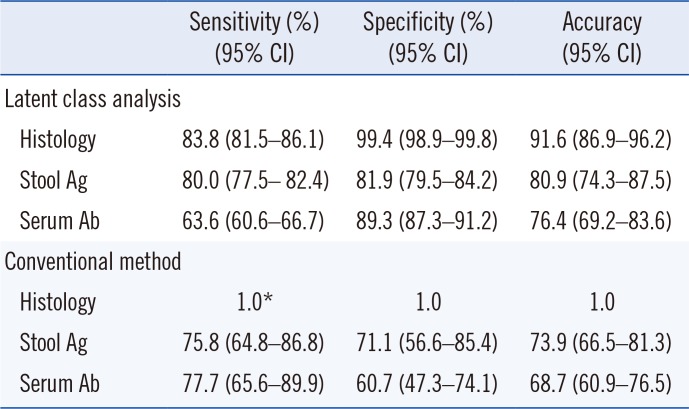
|
Sensitivity (%) (95% CI) |
Specificity (%) (95% CI) |
Accuracy (95% CI) |
Latent class analysis |
|
|
|
Histology |
83.8 (81.5–86.1) |
99.4 (98.9–99.8) |
91.6 (86.9–96.2) |
Stool Ag |
80.0 (77.5– 82.4) |
81.9 (79.5–84.2) |
80.9 (74.3–87.5) |
Serum Ab |
63.6 (60.6–66.7) |
89.3 (87.3–91.2) |
76.4 (69.2–83.6) |
Conventional method |
|
|
|
Histology |
1.0*
|
1.0 |
1.0 |
Stool Ag |
75.8 (64.8–86.8) |
71.1 (56.6–85.4) |
73.9 (66.5–81.3) |
Serum Ab |
77.7 (65.6–89.9) |
60.7 (47.3–74.1) |
68.7 (60.9–76.5) |

Our results showed that diagnostic capability was 5–10% higher for the LCA two-class model than for the conventional method. Thus, using LCA could support diagnosis in the absence of a reference standard. The results of our two-class LCA model are in line with a study showing that the sensitivity and specificity of histology are 85–95% and almost 100%, respectively [
5]. The sensitivity and specificity of Ag-ICA vary widely: 48.9–92.2% and 88.9–94.4%, respectively [
515]. Sensitivity and specificity of Ab-ICAs are 55.6–97.8% and 60.3–96.8%, respectively [
5]. The low specificity of Ag-ICA (71.1%) in our study was due to the use of histology as the reference standard; most previous studies used a composite reference standard [
56]. Despite its low specificity, Ag-ICA can be used in combination with the Ab-ICA in LCA. If the serum antibody test had concordant results with the stool antigen test, the LCA might show higher specificity than the conventional method, whereas discordant results might result in similar or lower specificity.
In previous studies, histology results were combined with those of other tests to construct a composite reference standard [
6]. A combination of tests has been used to classify definite infection or probable infection based on the number of positive test results [
616]. LCA and the conventional method provided similar results, as shown in
Table 2. However, the 5–10% increase in sensitivity and specificity indicates that LCA has improved ability to evaluate
H. pylori infection diagnostic tests. Thus, LCA might provide a reliable reference standard in the absence of invasive methods for diagnosing
H. pylori infection.
A limitation of this study was that the sample size was relatively small. Further, information on the number and site of the biopsy specimens was not available, which might affect the positive rate of H. pylori infection.
In conclusion, LCA could be applied to evaluate diagnostic tests that lack a reference standard. Sensitivity and specificity increased using the LCA, except for the sensitivity of serum antigen tests.