Abstract
Objective
To measure the accuracy and usability of an the image-processing based pill identifier application that we have developed.
Methods
The subjects selected were medical residents and nurses. Five nurses and five physicians were randomly assigned to use either an the image-processing based pill identifier application (n=10), or the conventional pill identifier application (n=10). They were instructed to examine 10 pills using the application assigned to them, and searches that took <3 minutes to find candidate drugs were recognized as successes. Among these successful searches, the accuracy was defined to identify the correct names of the drugs and the times needed in the correctly identifications were also measured. After using one application the subjects were instructed to use the other one and repeat the same process. Finally, they answered a questionnaire on the usability of the applications.
Results
The average proportion searches completed within 3 minutes was 91% for the the image-processing based pill identifier application, slightly, but not significantly, higher than that for the conventional pill identifier application (85%). The accuracies of the the image-processing based and conventional pill identifier applications were similar, 89% and 83%, respectively. In the usability examination, the the image-processing based pill identifier application yielded higher scores for the desirable, usable, findable and useful qualities than the conventional pill identifier application.
REFERENCES
1. Smolen JS, Landewé R, Bijlsma J, Burmester G, Chatzidionysiou K, Dougados M, et al. EULAR recommendations for the management of rheumatoid arthritis with synthetic and biological disease-modifying antirheumatic drugs: 2016 update. Ann Rheum Dis. 2017; 76:960–77.
2. Treharne GJ, Douglas KM, Iwaszko J, Panoulas VF, Hale ED, Mitton DL, et al. Polypharmacy among people with rheumatoid arthritis: the role of age, disease duration and comorbidity. Musculoskeletal Care. 2007; 5:175–90.


3. Wolf MS, Curtis LM, Waite K, Bailey SC, Hedlund LA, Davis TC, et al. Helping patients simplify and safely use complex prescription regimens. Arch Intern Med. 2011; 171:300–5.


4. Kantor ED, Rehm CD, Haas JS, Chan AT, Giovannucci EL. Trends in prescription drug use among adults in the United States from 1999-2012. JAMA. 2015; 314:1818–31.


5. Spiller HA, Griffith JR. Increasing burden of pill identification requests to US Poison Centers. Clin Toxicol (Phila). 2009; 47:253–5.


6. Hellier E, Tucker M, Kenny N, Rowntree A, Edworthy J. Merits of using color and shape differentiation to improve the speed and accuracy of drug strength identification on over-the-counter medicines by laypeople. J Patient Saf. 2010; 6:158–64.


7. Greene JA, Kesselheim AS. Why do the same drugs look different? Pills, trade dress, and public health. N Engl J Med. 2011; 365:83–9.


8. Steinhubl SR, Muse ED, Topol EJ. Can mobile health technologies transform health care? JAMA. 2013; 310:2395–6.


10. World Health Organization. From innovation to implementation: eHealth in the WHO European region [Internet]. Copenhagen: World Health Organization;2016. [cited 2017 Aug 22]. Available from:. http://www.euro.-who.int/__data/assets/pdf_file/0012/302331/From-Innovation-to-Implementation-eHealth-Report-EU.xml?ua=1.
11. Whitehead L, Seaton P. The effectiveness of self-management mobile phone and tablet apps in long-term condition management: a systematic review. J Med Internet Res. 2016; 18:e97.


12. Hamine S, Gerth-Guyette E, Faulx D, Green BB, Ginsburg AS. Impact of mHealth chronic disease management on treatment adherence and patient outcomes: a systematic review. J Med Internet Res. 2015; 17:e52.


13. Woo KH. Comparison of the usability of microblog – focused on the comparison between the Twitter and me2DAY. J Digit Design. 2010; 10:463–73.
14. Kwon GM, Kim AR, Kim SI. The activation methods of mobile service through usability testing – focus on comparison mobile application YES24 and KYOBO Bookstores. J Digit Design. 2014; 14:392–9.
15. Collins TR. Tech talk: apps put more rheumatology information at fingertips [Internet]. Atlanta: American College of Rheumatology;2012. Jun 10 [cited 2017 Aug 22]. Available from:. https://www.the-rheumatologist.org/article/tech-talk-apps-put-more-rheumatology-information-at-fingertips/.
Figure 2.
Pill identification by the image-processing based pill identifier application. 1. The main page displays three menu items (camera, gallery, history). 2. When the camera screen is on, users choose the tablet or capsule form and shape of the pill they want to search. 3. The progress of extraction of imprints is displayed in the color bar, and when the bar turns orange (meaning finished), users click the OK button to see the search results. 4. Candidate drugs are listed on the search result screen. 5. When a drug is selected, detailed information on it is presented on the screen.
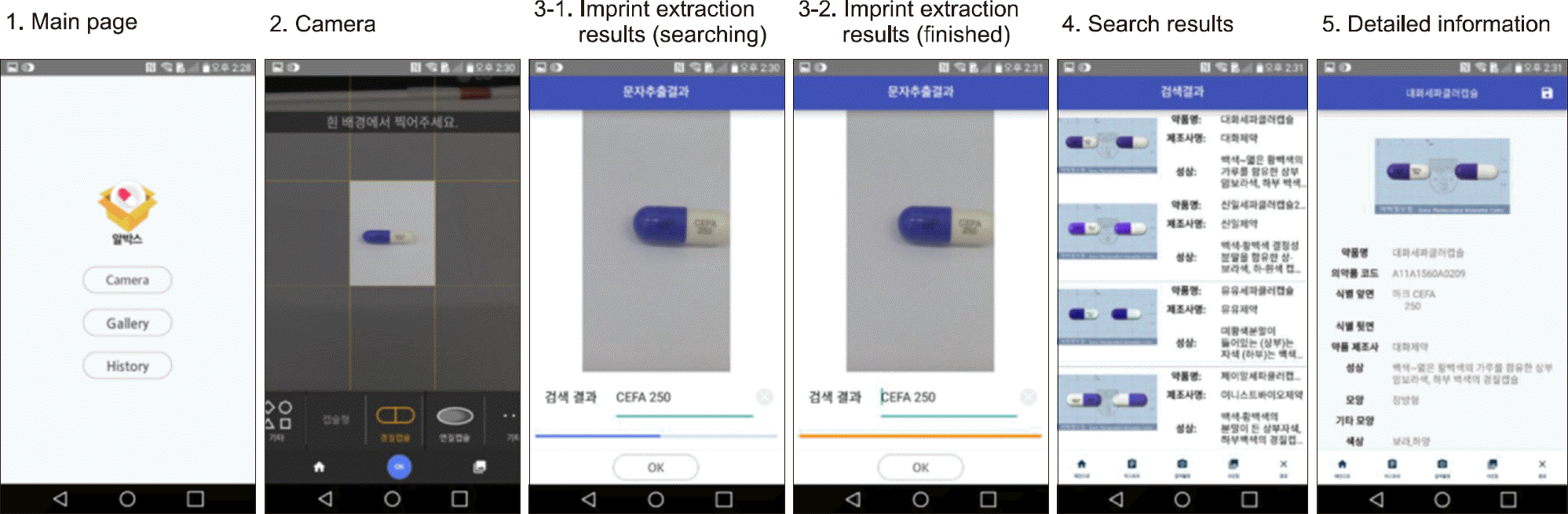
Supplementary Figure 1.
System working module of image-processing based pill identifier application. OCR: optical character recognition, API: application programming interface.
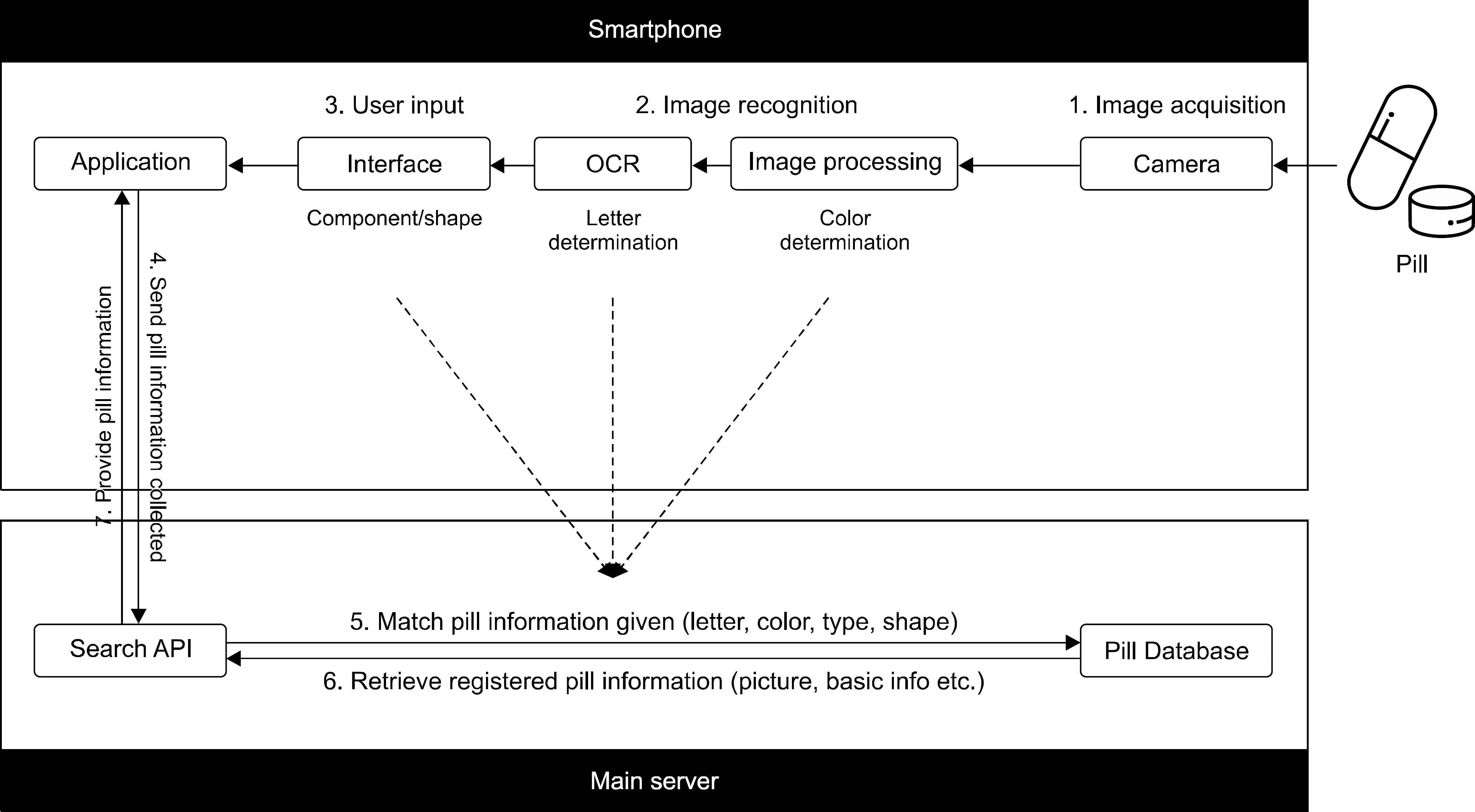
Supplementary Figure 2.
The ten drugs of different types selected for the test (ready for the test).
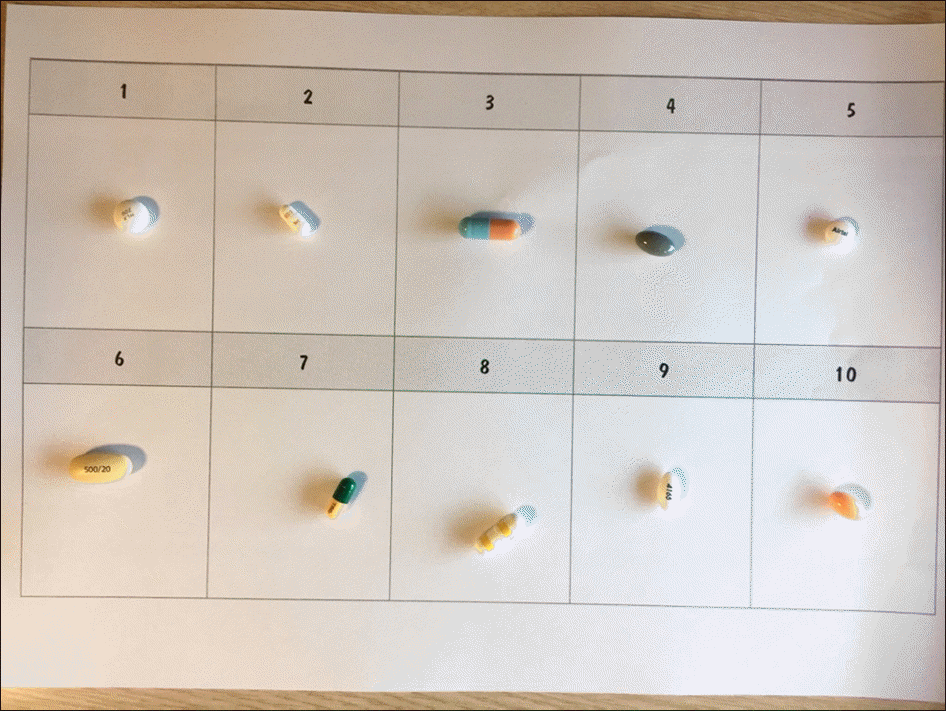
Table 1.
Recog Pill identification application
Pill identification application | Recognition rate* | Accuracy rate† | Time spent to find accurate drug names‡ | ||||||
---|---|---|---|---|---|---|---|---|---|
Image-processing based | Conventional | p-value | Image-processing based C | Conventional | p-value | Image-processing based (s) | Conventional (s) | p-value | |
Drug 1 | 100 | 80 | 0.45 | 100 | 100 | 0.47 | 74.2±49.9 | 70.5±49.3 | 0.83 |
Drug 2 | 80 | 80 | 1.00 | 100 | 100 | 1.00 | 53.9±45.0 | 41.1±21.9 | 0.80 |
Drug 3 | 90 | 80 | 1.00 | 100 | 100 | 1.00 | 48.9±32.2 | 61.6±41.9 | 0.89 |
Drug 4 | 70 | 90 | 0.58 | 85.7 | 100 | 0.27 | 66.3±43.2 | 53.7±47.2 | 0.36 |
Drug 5 | 100 | 90 | 1.00 | 100 | 100 | 1.00 | 29.8±12.1 | 41.5±16.3 | 0.08 |
Drug 6 | 100 | 100 | - | 90 | 80 | 1.00 | 21.8±12.8 | 27.6±11.1 | 0.02 |
Drug 7 | 90 | 70 | 0.58 | 100 | 100 | 0.58 | 36.7±18.9 | 64.6±45.5 | 0.25 |
Drug 8 | 90 | 70 | 0.58 | 100 | 100 | 0.58 | 35.7±12.6 | 40.6±34.3 | 0.25 |
Drug 9 | 100 | 100 | - | 100 | 100 | - | 25.5±12.8 | 18.6±7.9 | 0.06 |
Drug 10 | 90 | 90 | 1.00 | 100 | 100 | 1.00 | 46.0±26.9 | 39.2±21.8 | 0.67 |
Supplementary Table 1.
The comparison between the conventional pill identifier and image-processing based pill identifier application
Supplementary Table 2.
Ten drugs of different types selected for the test
Supplementary Table 3.
Questionnaire for evaluating usability based on the classification system of the honeycomb model