INTRODUCTION
METHODS
Participants and study design
Table 1
Clinical characteristics of participants
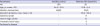
![]() | Fig. 1Study design and word-specific HMM training. Separate HMMs were generated for each of the 37 words in the Assessment of Phonology and Articulation for Children test and HMMs were trained through recordings from 100 healthy individuals. Recordings from 28 patients were applied to the developed dysarthria assessment software to yield aLL. Subsequently, two SLP listen to the recordings and calculate the CPA. Two repeated recordings in the patient group were applied to the software and obtain aLL1 and aLL2 for test-retest reliability.HMM = hidden Markov model, aLL = average log likelihood, CPA = consonant production accuracy.
|
Speech examination by SLPs
Word-specific HMMs
Statistical analysis
RESULTS
Assessment of dysarthria by SLPs
![]() | Fig. 2CPA from two different SLPs. (A) The scatter plot for the CPA value of each patient with both measurements from both SLPs (B) Bland-Altman plot for the CPA value of each patient with measurements from both SLPs. Mean differences are indicated by the solid line and 95% limits of agreement (mean differences ± 1.96 standard deviation of the difference) are shown by the dashed line. The dotted line shows regression lines for proportional biases.CPA = consonant production accuracy, SLPs = speech-language pathologists.
|
Assessment of dysarthria using HMMs
![]() | Fig. 3Comparison between CPA values measured by SLPs and aLL values calculated by word-specific HMMs. (A-D) The scatter plot comparing CPA values from SLPs and aLL values from word-specific HMMs in repetition. (E) The scatter plot of the aLL value obtained by applying the repeated recordings to the HMM.CPA = consonant production accuracy, SLPs = speech-language pathologists, aLL = average log likelihood, HMM = hidden Markov model.
|