Abstract
ACKNOWLEDGEMENTS
References








































Fig. 1
Area under receiver operator characteristic (ROC) curve values predictive of a hypothetical condition carry modest (1.5), sizeable (10), and large (50) odds ratios (ORs), showing false-positive fractions at 80% sensitivity (dotted line; fractions are >75, >25, and <10%, respectively). The graph demonstrates that very large ORs are needed to provide acceptably low false-positive fractions. Reprinted from Jakobsdottir, et al. PLoS Genet 2009;5:e1000337, with permission of Jakobsdottir, et al.30
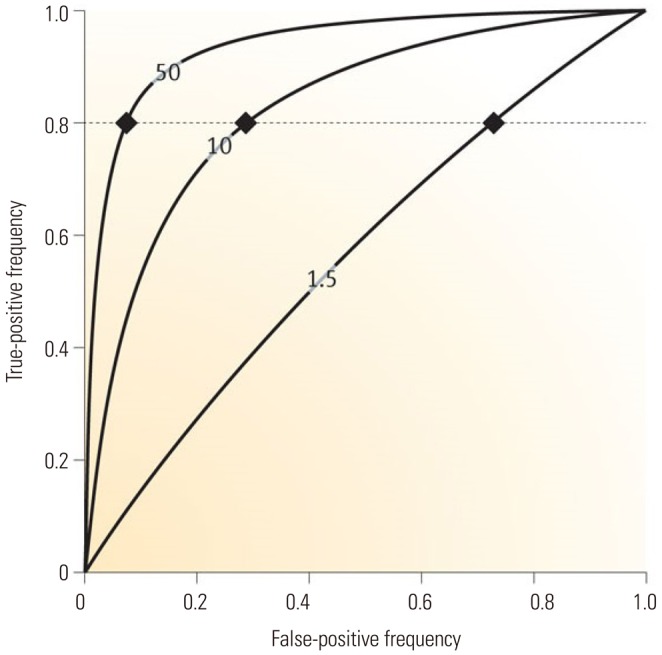
Fig. 2
ROC curves used to devise the best combinatorial model using the 10 most statistically significant SNPs. Values were taken from the first genomewide association study, which used 100K Bead Chips, to examine 80 NERD and 100 ATA subjects. RR was calculated for each individual subject using a multiple logistic regression analysis examining all 1023 combinations (210−1) of the 10 SNPs described in reference 34. A model consisting of eight SNPs shows the highest area under the ROC curve of 0.9 with an accuracy of 82.01%. The sensitivity and specificity are 78% and 88%, respectively, with an odds ratio of 20.74 (p=3.24E^−19). The eight SNPs are listed in Table 2. Reprinted from Shin, et al. DNA Cell Biol 2012;31:1604–9, with permission of Mary Ann Liebert, Inc.34 ROC, receiver operator characteristic; SNP, single nucleotide polymorphism; NERD, nonsteroidal antiinflammatory drug-exacerbated respiratory diseases; ATA, aspirin-tolerant asthma; RR, relative risk.
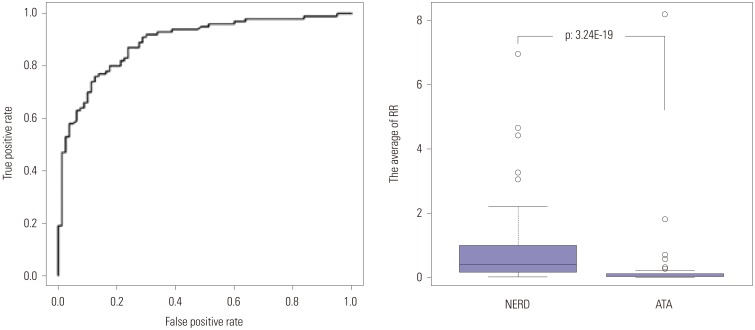
Fig. 3
Linkage disequilibrium (LD) between the six exonic SNPs in the HLA genes. The number in the box indicates LDr2. Reprinted from Shin, et al. PLoS One 2014;9:e111887, with permission of Shin, et al.36
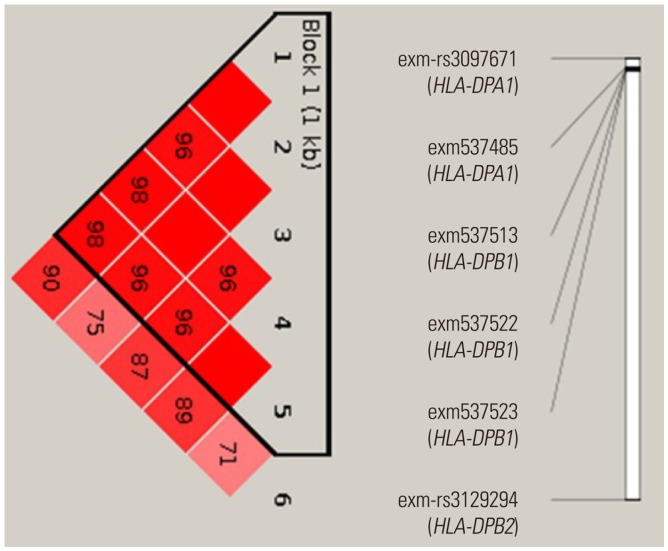
Table 1
Classification of Asthma Phenotypes according to Etiologic Agent, Clinical and Inflammatory Patterns, and Molecular Mechanisms
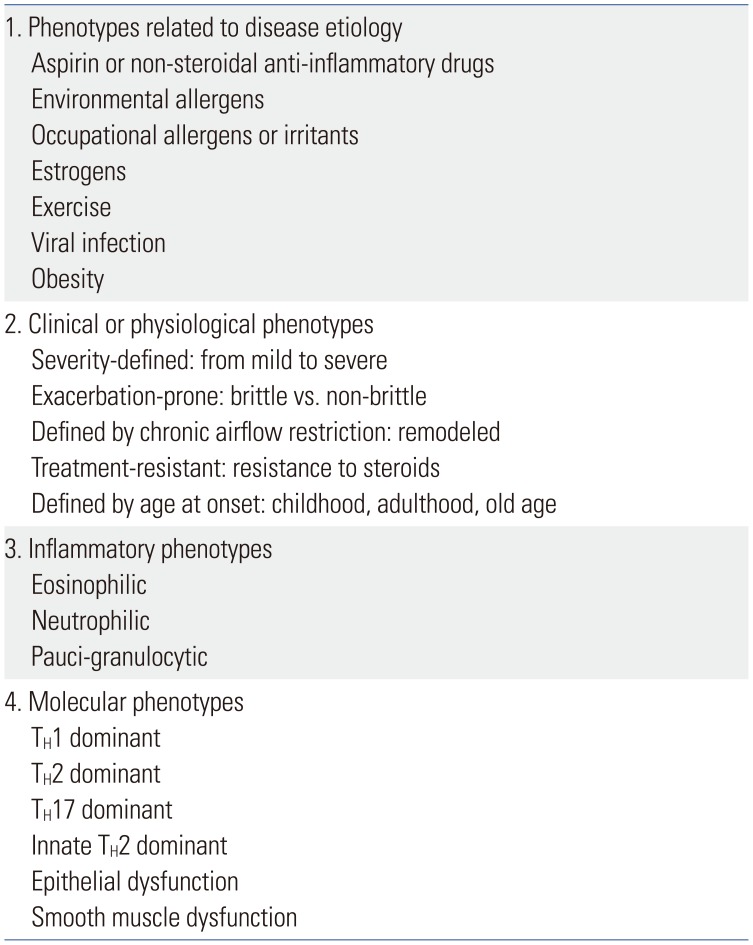
Table 2
List of the Eight SNPs in Fig. 2 and their RRs for Diagnosis of Nonsteroidal Anti-Inflammatory Drug-Exacerbated Respiratory Diseases
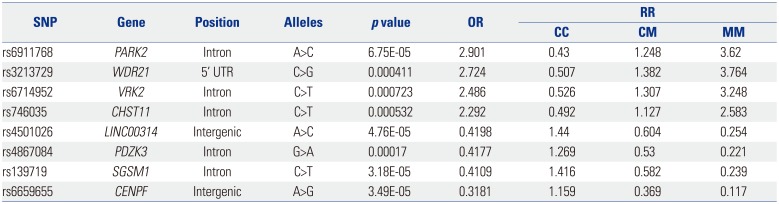
SNP, single nucleotide polymorphism; RR, relative risk; OR, odds ratio; PARK2, Parkin RBR E3 Ubiquitin Protein Ligase; WDR21, DDB1 and CUL4 Associated Factor 4; VRK2, Vaccinia Related Kinase 2; CHST11, Carbohydrate Sulfotransferase 11; LINC00314, Long Intergenic Non-Protein Coding RNA 314; PDZK3, PDZ Domain Containing 2; SGSM1, Small G Protein Signaling Modulator 1; CENPF, Centromere Protein F.
Reprinted from Shin, et al. DNA Cell Biol 2012;31:1604–9, with permission of Mary Ann Liebert, Inc.34
Table 3
List of the Top 30 SNPs Associated with NERD
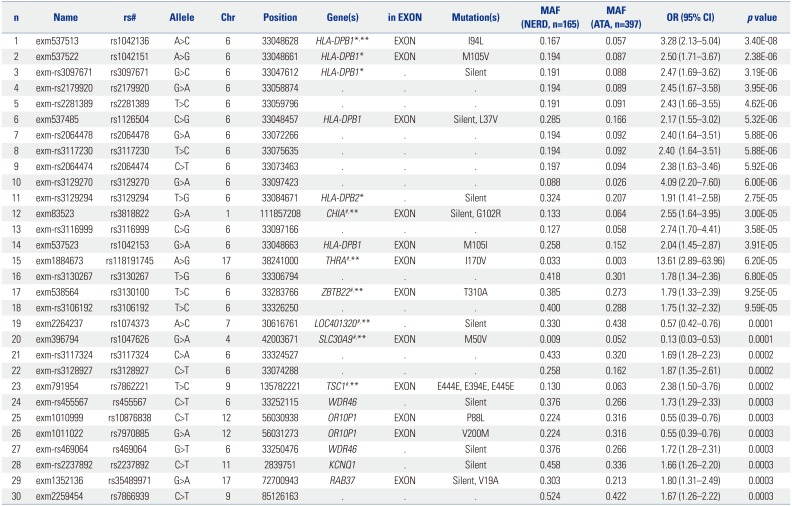
NERD, nonsteroidal antiinflammatory drug-exacerbated respiratory diseases; MAF, minor allele frequency; ATA, aspirin-tolerant asthma; SNP, single nucleotide polymorphism; AUC, area under the curve; OR, odds ratio. An exonic SNP (exm537513 in HLA-DPB1, rs1042136) exhibited the strongest association with NERD (p=3.4×10−8, OR=3.28). Among six exonic SNPs within the HLA genes, a combination of four SNPs (marked with *) had the best AUC value of 0.629, with an OR=3.677, 68.4% accuracy, 29.8% sensitivity, and 90.3% specificity. The other six SNPs (marked with #) were then added to the four HLA SNPs, and multiple logistic regression analysis was performed. Relative risk was calculated for each individual subject using a multiple logistic regression analysis examining all 1023 combinations (210−1) of the 10 SNPs. A combination model of seven SNPs marked with ** showed the best AUC of 0.75 (p=7.94×10−21), with OR=7.291, 40.8% sensitivity, 88.0% specificity, and 75% accuracy for discrimination between NERD from ATA. Reprinted from Shin, et al. PLoS One 2014;9:e111887, with permission of Shin, et al.36
Table 4
List of the 14 Top SNPs Predictive of NERD from the 660K GWAS
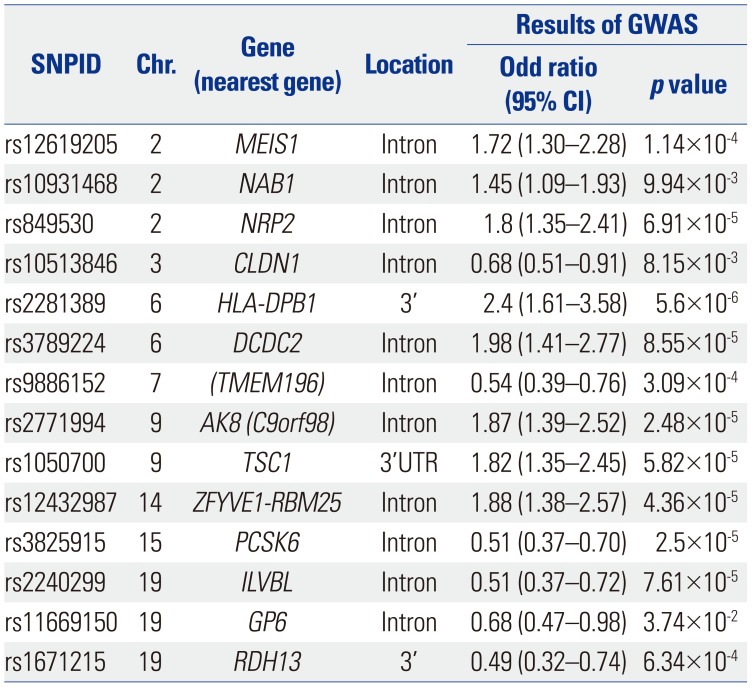
SNP, single nucleotide polymorphism; NERD, nonsteroidal antiinflammatory drug-exacerbated respiratory diseases; GWAS, genome-wide association studies; CI, confidence interval.
Using the 14 SNPs listed in the table, combinatorial analysis was performed, and the results are summarized in Table 5. Reprinted from Chang, et al. Pharmacogenomics J 2015;15:316–21, with permission of Springer Nature.37
Table 5
Diagnostic Value of the Summed Risk Scores Using the Top 14 SNPs to Predict NERD

SNP, single nucleotide polymorphism; NERD, nonsteroidal antiinflammatory drug-exacerbated respiratory diseases; ATA, aspirin-tolerant asthma; +PV, positive predictive value; −PV, negative predictive value; LR+, positive likelihood ratio; LR−, negative likelihood ratio; OR, odds ratio; RR, relative risk.
To calculate the genetic risk score of each of the subjects, ORs were assigned to the risk alleles in contrast to the value of 1 assigned to reference alleles. ORs from multiple variants in different genetic loci were summed into a single RR value. The summed risk scores of the 14 SNPs in the study subjects ranged from 0.774 to 1.142. The summed risk scores were significantly higher in the NERD group than in the ATA group (1.033±0.005 vs. 0.963±0.002, p=8.58×10−37). A higher proportion of NERD subjects showed summed risk scores above the cutoff value [64.66% vs. 15.04%, p=1.38×10−32, OR: 10.34 (6.69–15.96)]. The sensitivity and specificity of the scores were 64.7% and 85.0%, respectively, with 42.1% positive and 93.4% negative predictive values. The overall accuracy of the test was 82.0%. Reprinted from Chang, et al. Pharmacogenomics J 2015;15:316–21, with permission of Springer Nature.37
Table 6
Diagnostic Accuracy of SNPs Identified in GWAS and Exon-Wide Association Studies for NERD
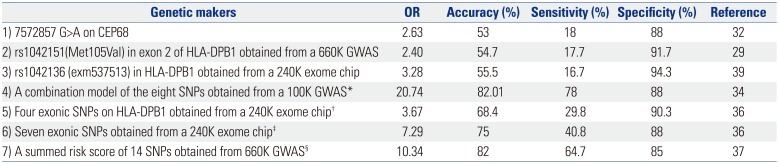
SNP, single nucleotide polymorphism; GWAS, genome-wide association studies; NERD, nonsteroidal antiinflammatory drug-exacerbated respiratory diseases; OR, odds ratio.
*A combination set of eight SNPs listed in Table 2, †A combination of four exonic SNPs (exm537513, exm537522, exm-rs3097671, and exmrs3129294) listed in Table 3, ‡A combination model of seven SNPs (exm537513, exm83523, exm1884673, exm538564, exm2264237, exm396794, and exm791954) listed in Table 3, §14 SNPs on the genes [HLA-DPB1, MEIS1, NAB1, NRP2, CLDN1, DCDC2, TMEM196, AK8(C9orf98), TSC1, ZFYVE1-RBM25, PCSK6, ILVBL, GP6 and RDH13] listed in Table 4.