Abstract
Clinical studies can be conducted to gather real world evidence (RWE) not available from randomized controlled trials, providing new information and knowledge. Although the concept of RWE emerged relatively recently, numerous clinical studies are utilizing it. However, many researchers are engaging in trial and error that may not overcome the various biases that occur in electronic medical record (EMR)-based RWE studies. While RWE can reflect the real world, there are still limitations to its acceptance. There are many hurdles in using RWE and solutions must be explored. Results based on RWE may be overestimated and it can be difficult to derive good quality results. This paper discusses data quality management, direct chart review, sample size, study design, and the interpretation of EMR-based RWE. More specifically, this paper shares the experience of the various hurdles that occur when conducting RWE studies and discusses the easy-to-false errors. RWE is still in the developmental stage and numerous aspects of RWE use remain unclear. Nonetheless, despite its many limitations, increasing use of RWE is still anticipated. This will require continued experience and effort in using RWE, as well as upgrading RWE research through the accumulation of information on such experiences and efforts.
Graphical Abstract
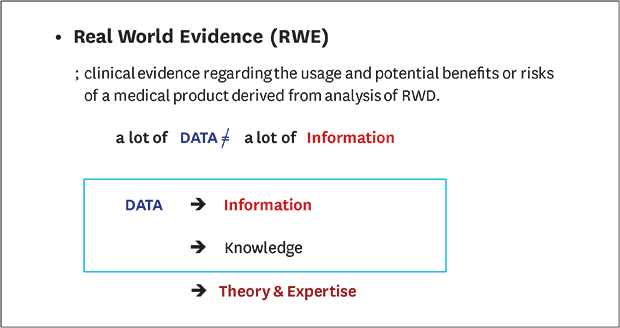
Real world data (RWD) refers to all health data obtained from a variety of sources, not collected for clinical research purposes.1 Real world evidence (RWE) using RWD offers a variety of benefits not available in randomized controlled trials (RCTs).2 At this point, the immediate goals of studies using RWE must be to conduct post marketing surveillance, or those in which conducting an RCT is impossible, as well as studies developing data structures for artificial intelligence such as predictive models.2 However, RWD was not originally conceptualized for traditional clinical research.3 In other words, RWE remains unsuitable for this application. Merely emphasizing that large scale research is possible with less time and cost than a conventional RCT is not enough to uphold the reliability of research results based on RWD. Indeed, despite the apparent potential of RWE, concerns regarding the interpretation of research results obtained using RWE are likely even more numerous than currently anticipated.456 While the number of RWE studies is growing, there are few concrete means of overcoming these hurdles. In studies using RWE, a clear hypothesis regarding the purpose of the study and a clear prior definition of data extraction is required. In this paper, we share our experience of the various hurdles that emerge in conducting RWE studies and comment on easy-to-false errors.
Electronic medical record (EMR) data used in hospitals are the most refined and structured among the various types of RWD.7 It is sometimes thought that structured data are more completely optimized for clinical research. However, this is not always the case, particularly given that extracted EMR data can still be unstable and contain serious errors.8 For laboratory tests, results are expressed as clear numbers and the normal range of the value is clearly defined; hence, interpretation is easy and reliability is high. However, values exceeding the range that the medical equipment can measure may include non-numerical characters (e.g., > 300 mg/dL, under 0.01), which require direct chart reviews and modifications by researchers. Currently, EMR data predominantly consist of free texts and laboratory results. Moreover, numerous stipulative or unformulated abbreviations are used, and different abbreviations are often used by departments and individuals. For example, height and weight may not be properly measured but rather reported verbally by patients. Consequently, different heights may be recorded at each visit for the same patient. Furthermore, whether blood pressure is measured in a stable state is often not confirmed. Errors can also occur when researchers enter values in EMR manually.
One of the most overvalued aspects of studies using RWE is sample size. Unlike RCT, which consists of a homogenous sample, RWE consists of a heterogenous sample. Obtaining as much information as possible from a large sample is a goal of RWE. However, information is not always available from a large sample.9 In fact, even if a large number of subjects are recruited, data from a considerable number of patients often will be excluded depending on the purpose of the study. In other words, even if researchers begin with a large sample, they may encounter a large amount of missing data during the course of the research. While sample size is the largest advantage of RWE, it is also the largest disadvantage in terms of clinical research.
Data from a large sample are advantageous for studies of rare adverse drug reactions.101112 However, unless a structured clear value can define rare diseases or drug side effects, the researcher must go through the chart review process directly. In many cases, however, the actual chart record is insufficient, and identifying a causal relationship using only written data can be tenuous. Data may also be excluded if a patient did not voluntarily stop taking a drug and visited the hospital because of drug side effects, or if a patient was transferred to another hospital. From the researcher's point of view, the latter more often accounts for missing data.
Performing a real chart review is time consuming, especially when the sample size is large. A large sample requires the involvement of more researchers in the DQM process. Moreover, the risk of information bias in this process cannot be ignored. Therefore, a clear protocol must be established before the research to reduce resultant bias. Researchers should start by organizing and researching the DQM process protocol, not simply the inclusion or exclusion criteria. As such, a skilled and experienced clinical researcher is helpful to ensure credible results.
One of the most common misconceptions is the extreme notion that RCTs never reflect real practice, and that only RWE can. On the contrary, RCT is the most reliable form of clinical study13 and is conducted in a real setting. In RCT, patients visit hospitals at regular intervals; the medical institution performs inspections, physical examinations, and blood tests at regular intervals, and there is a high rate of compliance with these tests.3 Evidently, it is possible to measure the effects of certain drugs, as well as their adaptability and short-term side effects. In contrast, in RWE, patients visit the hospital after one month, at 3–6-month intervals, or after more than a year. It is also difficult to confirm whether the drug was taken appropriately in RWE (compliance is determined by calculating the number of drug prescription days and visit intervals).14 The use of RWE in studying the effects of a particular drug has clear limitations and is difficult to trust. The results do not indicate the effect of the drug itself, but rather the actual practice of adding elements of patient compliance and the environment to the drug itself. Thus, relative comparisons between drugs in the same conditions are preferable to the effects of drugs in RWE studies. Using RWE clarifies the causal relationship between certain drugs and various diseases due to the temporal relationship between the onset of the disease and the risk factors of certain drugs. However, actual causality is difficult to discern in many cases due to various risk factors, non-compliance evaluation, and misleading data. Nevertheless, it has advantages over RCT in some respects.
RWE is often based simply on International Classification of Diseases (ICD)-10 to investigate the incidence of a disease under certain conditions or whether it has a comorbid disease. However, there are many cases in which diagnoses or side effects are missing; here, the diagnostic name that is entered (mainly the claim data) can differ from the actual diagnostic name. For severe diseases such as myocardial infarction and cancer, the diagnosis is usually accurate. In addition to the ICD-10 classification, blood tests often complement diagnoses in cases such as diabetes mellitus; however, in cases of diseases defined by clinical symptoms rather than blood tests, a significant number of cases may be underestimated or overestimated.
RWE refers to the analysis of data that have already accumulated. Thus, while most results from RWE studies are easy to interpret and identify, it is not possible to explain causality in many cases. While data are developed into information through technological/statistical techniques in RWE studies, data are not developed into knowledge that includes medically meaningful content. For correct interpretation, researchers with sufficient medical knowledge should have a clear hypothesis and conduct research with adequate protocols developed before the study. After obtaining information from a large amount of data, knowledge should be derived based on the medical knowledge system, and the medical personnel should be able to accept the medical feasibility of this series of processes and results.15 Deriving results using simple and statistical techniques and interpreting them separately makes it difficult to determine whether the correct data leads to correct information. Although RWE can reflect the real world, there are still limitations to its acceptance. Experienced researchers, methodologies, data managers, and experts with interpretation skills are required for the proper interpretation of RWE results.
Notes
References
1. Mahajan R. Real world data: additional source for making clinical decisions. Int J Appl Basic Med Res. 2015; 5(2):82.



2. Kim HS, Lee S, Kim JH. Real-world evidence versus randomized controlled trial: clinical research based on electronic medical records. J Korean Med Sci. 2018; 33(34):e213.


3. Sherman RE, Anderson SA, Dal Pan GJ, Gray GW, Gross T, Hunter NL, et al. Real-world evidence - what is it and what can it tell us? N Engl J Med. 2016; 375(23):2293–2297.


4. Farmer R, Mathur R, Bhaskaran K, Eastwood SV, Chaturvedi N, Smeeth L. Promises and pitfalls of electronic health record analysis. Diabetologia. 2018; 61(6):1241–1248.


5. Lewis JD, Bilker WB, Weinstein RB, Strom BL. The relationship between time since registration and measured incidence rates in the General Practice Research Database. Pharmacoepidemiol Drug Saf. 2005; 14(7):443–451.


6. Sun X, Tan J, Tang L, Guo JJ, Li X. Real world evidence: experience and lessons from China. BMJ. 2018; 360:j5262.


7. Kim HS, Kim H, Jeong YJ, Kim TM, Yang SJ, Baik SJ, et al. Development of clinical data mart of HMG-CoA reductase inhibitor for varied clinical research. Endocrinol Metab (Seoul). 2017; 32(1):90–98.



9. Wierzbicka N, Jahnz-Różyk K. The evolving landscape for real world evidence in Poland: physicians' perspective. J Health Policy Outcome Res. 2015; 1:15–33.
10. Kim H, Baik SY, Yang SJ, Kim TM, Lee SH, Cho JH, et al. Clinical experiences and case review of angiotensin II receptor blocker-related angioedema in Korea. Basic Clin Pharmacol Toxicol. 2019; 124(1):115–122.


11. Corrigan-Curay J, Sacks L, Woodcock J. Real-world evidence and real-world data for evaluating drug safety and effectiveness. JAMA. 2018; 320(9):867–868.


12. Kim HS, Lee SH, Kim H, Lee SH, Cho JH, Lee H, et al. Statin-related aminotransferase elevation according to baseline aminotransferases level in real practice in Korea. J Clin Pharm Ther. 2016; 41(3):266–272.


13. Makady A, de Boer A, Hillege H, Klungel O, Goettsch W. on behalf of GetReal Work Package 1. What is real-world data? a review of definitions based on literature and stakeholder interviews. Value Health. 2017; 20(7):858–865.

