Abstract
Objective
For localization of the motor cortex, seed-based resting-state functional MRI (rsfMRI) uses the contralateral motor cortex as a seed. However, research has shown that the location of the motor cortex could differ according to anatomical variations. The purpose of this study was to compare the results of rsfMRI using two seeds: a template seed (the anatomically expected location of the contralateral motor cortex) and a functional seed (the actual location of the contralateral motor cortex determined by task-based functional MRI [tbfMRI]).
Materials and Methods
Eight patients (4 with glioma, 3 with meningioma, and 1 with arteriovenous malformation) and 9 healthy volunteers participated. For the patients, tbfMRI was performed unilaterally to activate the healthy contralateral motor cortex. The affected ipsilateral motor cortices were mapped with rsfMRI using seed-based and independent component analysis (ICA). In the healthy volunteer group, both motor cortices were mapped with both-hands tbfMRI and rsfMRI. We compared the results between template and functional seeds, and between the seed-based analysis and ICA with visual and quantitative analysis.
Results
For the visual analysis, the functional seed showed significantly higher scores compared to the template seed in both the patients (p = 0.002) and healthy volunteers (p < 0.001). Although no significant difference was observed between the functional seed and ICA, the ICA results showed significantly higher scores than the template seed in both the patients (p = 0.01) and healthy volunteers (p = 0.005). In the quantitative analysis, the functional seed exhibited greater similarity to tbfMRI than the template seed and ICA.
Accurate preoperative localization of the motor cortex is critical for minimizing postoperative neurological deficits (12). Intraoperative electrical stimulation in awake surgery is currently the gold standard method for motor cortex localization. However, it is an invasive method and does not provide neurosurgeons with preoperative guidance (34).
Functional MRI (fMRI) is a useful imaging method for localizing the motor cortex using a blood-oxygen-level-dependent (BOLD) signal. It is noninvasive and provides preoperative information for mapping the motor cortex (5). fMRI can be divided into task-based fMRI (tbfMRI) and resting-state fMRI (rsfMRI); recently, rsfMRI has been identified as a promising method for motor cortex localization (6). Compared to tbfMRI, rsfMRI studies observe the brain in the absence of overt task performance or stimulus. Therefore, rsfMRI can be used in patients with neurological deficits or in uncooperative patients, including children or anesthetized patients (47).
Many studies have demonstrated that rsfMRI could be clinically effective for presurgical mapping of the eloquent motor cortex (489101112). Functional connectivity has been found between the left and right sensorimotor cortices and between language areas (9101113). Furthermore, the reliability of this connectivity has been found to be consistent within the same patients and across patients (11114). The results of tbfMRI and rsfMRI have been compared and found to be comparable (8911141516). Among the various analytical methods of rsfMRI, seed-based analysis uses the contralateral motor cortex of the healthy hemisphere as a seed and has been widely used to localize the motor cortex in hemiplegic or uncooperative tumor patients (9101114). In these studies, the contralateral hand area of the motor cortex was determined based on the anatomy of a knob-like structure in the precentral gyrus (14).
However, previous studies have shown that the location of the motor cortex could differ according to anatomical variations and distortions or shifting caused by mass effects in patients with tumors (581718). Although anatomical distortion or shifting might be less prominent in the contralateral motor cortex than on the affected ipsilateral side, it could cause inaccuracies in rsfMRI results obtained using a seed based on the anatomically expected location of the contralateral motor cortex. We hypothesized that the contralateral motor cortex determined by tbfMRI could be used as a seed in rsfMRI, and it might provide a more accurate map of the affected ipsilateral motor cortex. Our purpose was to identify an effective seed for rsfMRI for localizing the affected ipsilateral motor cortex in patients with brain pathologies that were unable to perform tbfMRI. We compared the results of template seed rsfMRI based on the anatomically expected location of the contralateral motor cortex with those of functional seed rsfMRI based on the location of the contralateral motor cortex determined by tbfMRI.
The drawback of seed-based analysis is that it relies on the operator's assumption for region of interest (ROI) selection (119). To solve this problem, we also compared the results between seed-based analysis and independent component analysis (ICA), which is a data-driven analytic method without the need for an operator's assumption.
This study was approved by our Institutional Review Board and the requirement for informed consent was waived in accordance with the requirements of a retrospective study. A total of 17 patients were enrolled in this study. Eight patients (7 men and 1 woman; mean age, 45.5 years; age range, 34–79 years) and 9 healthy volunteers (6 men and 3 women; mean age, 34.7 years; age range, 22–44 years) participated in the study. Pathologies included glioma in 4 patients, meningioma in 3 patients, and arteriovenous malformation in 1 patient. All of the patients suffered from lesion-induced motor weakness in the affected hand.
We used a 3T MRI scanner (Magnetom Verio, Siemens, Erlangen, Germany) with an 8-channel head coil. A magnetization-prepared gradient echo (MPRAGE) volumetric T1-weighted sequence was performed to assess anatomical localization with the following parameters: repetition time (TR), 1900 ms; echo time (TE), 2.93 ms; flip angle, 9°; field of view (FOV), 250 × 250 mm; matrix size, 256 × 256; slice thickness, 1 mm.
For tbfMRI, single-shot gradient-echo echo-planar imaging (EPI) was used with the following parameters: TR, 2000 ms; TE, 30 ms; flip angle, 90°; FOV, 240 × 240 mm; matrix size, 96 × 96; slice thickness, 3 mm. tbfMRI consisted of 5 cycles of blocked trial runs of hand motor movements for 30 seconds using finger tapping and rest runs for 30 seconds. For the patient group, tbfMRI was performed only with ipsilateral hand motor movements to activate the healthy contralateral motor cortex. For the healthy volunteer group, tbfMRI was performed bilaterally.
Single-shot gradient-echo EPI was used for rsfMRI: TR, 2000 ms; TE, 30 ms; flip angle, 90°; FOV, 240 × 240 mm; matrix size, 96 × 96; slice thickness, 3 mm. The patients were instructed to keep their eyes closed and to refrain from any cognitive, language, or motor tasks for 8 minutes during the resting-state acquisition.
Image preprocessing was performed with statistical parametric mapping 12 (https://www.fil.ion.ucl.ac.uk/spm). Time- and motion-correction were performed. Structural images using T1-weighted MPRAGE were co-registered and spatial normalization was performed based on the Montreal Neurologic Institute template. Images were smoothed using a spatial Gaussian kernel with a full width at half maximum of 8 mm to increase the signal-to-noise ratio (19). Linear detrending, bandpass filtering (0.01–0.08 Hz), and nuisance regression were performed. Statistical maps of activations were generated using cross-correlation analysis and the threshold for statistical significance was set to p < 0.05.
For the patient group, seeds were localized to the healthy contralateral hand motor cortex area because it was not possible to determine an affected ipsilateral seed for rsfMRI because of displacement and distortion of the motor cortex caused by the lesion. Two kinds of seeds were used: a template seed, which was indicated by a built-in anatomical template in CONN software (https://www.nitrc.org/projects/conn) (i.e., the anatomically expected location of the motor cortex), and a functional seed, which was determined by tbfMRI of the healthy contralateral hand motor cortex. A spherical seed ROI with a radius of 6 mm was drawn in each seed region to avoid noise.
rsfMRI was also analyzed with single-patient ICA using the Group ICA of fMRI Toolbox software (GIFT; http://mialab.mrn.org/software/gift/). ICA was performed separately for each patient using the InfoMax algorithm with the ICASSO (http://research.ics.aalto.fi/ica/icasso/) toolbox implemented in GIFT. The number of independent components (ICs) was estimated individually for each patient and a total of 30 ICs were performed in this study.
Two neuroradiologists reviewed the results of rsfMRI with template seeds, functional seeds, and ICA. For the patient group, we first evaluated the ability of rsfMRI in mapping the eloquent motor cortex location, and assessed the activation map to determine the strength of the signal in the motor cortex. For qualitative analysis, we used a scoring system based on signal intensity and visibility. The scoring criteria were as follows: score of 0 (no signal), score of 1 (detectable signal), and score of 2 (stronger signal) (Fig. 1).
In the healthy volunteer group, both motor cortex locations were mapped using both-hand tbfMRI and the participants also underwent rsfMRI. Seed-based rsfMRI was performed for the patient group as described previously. The two seed types, template and functional, were also used. Contralateral tbfMRI maps were used as functional seeds, whereas ipsilateral tbfMRI maps were used as a reference for comparison with the rsfMRI results obtained using the template and functional seed approaches. The scoring systems were based on signal intensity, visibility, and location overlap using the tbfMRI results. The same scoring system used in the patient group was applied.
For the quantitative assessment of the overlap of the activation maps of the two different methods, we calculated Dice coefficients at an optimal threshold which was determined by two neuroradiologists by consensus. Dice coefficients indicate the degree of similarity or concordance between two data sets, ranging from 0 to 1 (2021). To compute the coefficients, masks were extracted from the activation maps from which Dice coefficients were calculated by counting the number of voxels for the individual masks and overlapped regions. For comparisons between functional and template seeds, Dice coefficients were obtained from the two data sets: functional seed rsfMRI vs. tbfMRI, and template seed rsfMRI vs. tbfMRI. For comparisons between seed-based analysis and ICA, Dice coefficients were obtained from the values between functional seed rsfMRI-tbfMRI and ICA-tbfMRI or template seed rsfMRI-tbfMRI and ICA-tbfMRI. In comparisons between seed-based analysis and ICA, adopting tbfMRI as a reference allowed the results of Dice coefficients to indicate which method was more similar to tbfMRI.
The Wilcoxon rank-sum test was used to determine whether a significant difference existed between functional and template seeds and between seed-based analysis and ICA for the patient and healthy volunteer groups. For the healthy volunteer group, the Dice coefficients were compared between functional and template seeds and between seed-based analysis and ICA using the Wilcoxon rank-sum test. Statistical analyses were performed using commercially available software (SPSS, version 18 for Windows, SPSS Inc., Chicago, IL, USA). A value of p < 0.016 (0.05/3) indicated statistical significance for multiple comparisons correction.
In the rsfMRI results of the patient group, all motor cortices were identified using the functional seed approach but only half were identified using the template seed approach. Table 1 summarizes the patients' characteristics. Detailed analysis revealed that functional seed rsfMRI attained significantly higher scores than template seed analysis (p = 0.002); functional seed rsfMRI maps attained a score of 2 in 6 cortices (75%) and a score of 1 in 2 cortices (25%) (Table 2). However, template seed rsfMRI attained a score of 1 in 4 cortices (50%) and a score of 0 in 4 cortices (50%) (Table 2). ICA attained a score 2 in 5 cortices (62.5%) and a score of 1 in 3 cortices (37.5%). For the comparisons between seed-based analysis and ICA, no significant difference was observed between functional seed and ICA, whereas ICA showed significantly higher scores than template seed analysis (p = 0.01). Figure 2 shows an example of a patient with meningioma in the left parasagittal area; the template seed (Fig. 2B) attained a score of 1, whereas the functional seed (Fig. 2C) and ICA (Fig. 2D) attained a score of 2.
In the healthy volunteer group, the motor cortices were identified by both template and functional seed rsfMRI, and these rsfMRI maps were similar in locations compared with the reference tbfMRI. For the comparisons between functional and template seed rsfMRI, detailed analysis indicated that functional seed rsfMRI attained a score of 2 in 13 cortices (72.2%) and a score of 1 in 5 cortices (27.8%) (Table 2), whereas template seed rsfMRI attained a score of 2 in 4 cortices (22.2%) and a score of 1 in 14 cortices (77.8%) (Table 2). The functional seed analysis attained significantly higher scores than template seed analysis (p = 0.003). ICA attained a score of 2 in 12 cortices (66.7%) and a score of 1 in 6 cortices (33.3%). For the comparisons between seed-based analysis and ICA, no significant difference was observed between functional seed analysis and ICA, whereas ICA attained significantly higher scores than template seed analysis (p = 0.005). Figure 3 shows that template seed analysis (Fig. 3B) attained a score of 1 in the right motor cortex compared to tbfMRI (Fig. 3A), whereas the score of functional seed analysis (Fig. 3C) and ICA (Fig. 3D) was 2 in the right motor cortex.
In this study, Dice coefficients were calculated only for the healthy volunteer group because the patient group lacked tbfMRI data as a reference. In the healthy volunteer group, the mean Dice coefficient values were 0.40, 0.48, and 0.42 for the template seed analysis, functional seed analysis, and ICA, respectively, with reference to tbfMRI. Functional seed analysis was the most similar to tbfMRI among the three different methods. However, no significant difference was observed in Dice coefficient values between template seed and functional seed analysis. In addition, no significant differences were observed in Dice coefficient values between template seed analysis and ICA or between functional seed analysis and ICA (Table 3).
In this study, we determined a more effective method for selecting seeds when performing rsfMRI. Functional and template seeds can both be commonly used for delineating contralateral motor cortex imaging and the locations they identify are in close proximity. However, we found that functional seed rsfMRI could enhance visual delineation of the motor cortex compared to that of template seed analysis. The difference in results could be attributable to functional seed analysis being derived from an actual seed in tbfMRI. Thus, rsfMRI with functional seeds could reflect the distortion or shifting of normal brain architecture resulting from mass effects or obscuration caused by edema or pathologies. By contrast, because template seed analysis is reliant on the anatomically expected location of the motor cortex, it is more difficult for this approach to reflect the actual location of the motor cortex. Therefore, using the location of contralateral motor cortex obtained from tbfMRI as a seed could be an effective method for seed determination in rsfMRI.
Through comparisons between seed-based analysis and ICA, we found no significant difference between functional seed analysis and ICA. Although statistically nonsignificant, we observed a tendency toward higher scores with functional seed analysis than ICA in the visual assessment. Functional seed analysis was found to be the most similar to tbfMRI with a Dice coefficient value of 0.48, followed by ICA and template seed analysis. Furthermore, no significant difference was found between functional seed analysis and ICA in quantitative analysis. Regarding the comparisons between seed-based analysis and ICA, a previous study by Rosazza et al. (22) obtained results from the two methods that were similar with moderate correspondence, but not exactly identical. Joel et al. (23) reported that seed-based analysis provides a single metric of functional connectivity, whereas ICA provides total functional connectivity and decomposes into connectivity within each network and between networks. They demonstrated that seed-based analysis reveals the connectivity of the specific experimental state, whereas ICA provides metrics of connectivity between networks. Accordingly, functional seed analysis might be more accurate than ICA in delineating the motor cortex, particularly by showing a single metric of connectivity between the bilateral motor cortex in a patient with a brain tumor.
In the visual and quantitative analysis, tbfMRI was used as the reference only in the healthy volunteer group but not in the patient group. For the visual analysis, rsfMRI maps with functional seed analysis, template seed analysis, and ICA were compared with ipsilateral tbfMRI maps in the healthy volunteer group. By contrast, no reference was available for the patient group because tbfMRI could not be performed to localize the affected ipsilateral motor cortex. tbfMRI was performed in the patient group only to localize the healthy contralateral motor cortex. Therefore, we compared the results of rsfMRI without tbfMRI as the reference in the patient group. For the quantitative analysis, the Dice coefficients between rsfMRI and tbfMRI were obtained to determine the degree of similarity with respect to the reference tbfMRI. Because the patient group did not have reference tbfMRI of the affected ipsilateral side, Dice coefficients were calculated only in the healthy volunteer group.
Since Biswal et al. (24) reported rsfMRI as a useful diagnostic tool, many studies (8101114) have also demonstrated its usefulness. The rsfMRI method focuses on spontaneous low-frequency fluctuation (< 0.1 Hz) and uses the BOLD technique without task performance. Therefore, rsfMRI has been demonstrated as a highly useful tool for presurgical mapping of the motor cortex in disabled, anesthetized, or uncooperative patients and children (1237). Zhang et al. (11) and Liu et al. (14) reported that rsfMRI was effective for presurgical motor cortex mapping. They applied seed-based analysis using the contralateral motor cortex as a seed. They used the anatomically expected location of the motor cortex, which was defined as a presumptive template seed in our study. To the best of our knowledge, no studies have reported a more reliable method for selecting seeds in rsfMRI.
Hou et al. (17) quantitatively compared tbfMRI, rsfMRI, and anatomically expected locations to determine the location of the motor cortex in patients and healthy volunteer groups. In the healthy volunteer group, the results showed a gap of less than 2 cm between the anatomically expected location of the motor cortex and the motor cortex location determined by tbfMRI. This result supports our hypothesis of a difference between the anatomically expected location of the motor cortex (template seed) and the tbfMRI-derived motor cortex location (the functional seed).
The most common analytical methods for rsfMRI are seed-based analysis and ICA (1). Several studies have shown that these two methods produce similar and complementary results (2223). Seed-based analysis has several advantages and disadvantages compared to the ICA method. For seed-based analysis, the results are straightforward and easy to interpret; however, it requires an assumption by the operator to determine the seed area. Conversely, ICA does not require assumption-driven determination of the seed area. Instead, because the ICA is a data-driven extraction method, the results of ICA could differ depending on the number of components selected. Moreover, problems can be encountered when interpreting noise (16892526).
This study had some limitations. First, the number of enrolled patients was small. Second, the disease spectrum in the patient group was heterogeneous. According to Fraga de Abreu et al. (27), the BOLD signal is affected by tumor types in tbfMRI, with a markedly decreased signal in glioblastomas but not in metastasis and meningiomas. Therefore, the BOLD signal might have differed because of tumor types in our study. Accordingly, further studies must account for the effect of different tumor types in image analysis. Third, we performed tbfMRI only for the hand paradigm and, therefore, tailored design is necessary for tbfMRI using the foot and face in the future. Additionally, our results did not provide correlations with electrical cortical stimulation, which is the gold standard for motor cortex localization. In the visual analysis of activation maps, demonstration of larger correlated areas may improve visual sensitivity but may conversely increase false-positives. To overcome the disadvantages of visual analysis, we applied quantitative analysis with Dice coefficients. Nevertheless, an appropriate threshold is necessary for the acquisition of activation maps in the future.
In conclusion, preserving the motor cortex is essential to maintaining quality of life and ensuring functional outcomes for patients undergoing surgery. Therefore, dedicated and precise mapping is crucial. We postulated that seed-based analysis for rsfMRI with a functional seed in which the location of the contralateral motor cortex is derived from tbfMRI could enhance pre-surgical mapping of the motor cortex.
Figures and Tables
Fig. 1
Patient group scoring criteria.
Classification is divided into scores of 0-2, as follows.
A. Score of 0: rsfMRI reveals no signal on left motor cortex. B. Score of 1: rsfMRI reveals detectable signal in left motor cortex. C. Score of 2: rsfMRI reveals strong signal in left motor cortex. All images are demonstrated left-on-left (neurologic convention). rsfMRI = resting-state functional MRI
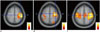
Fig. 2
Patient group representative case.
Axial contrast-enhanced T1-weighted image (A) showing well-enhanced extra-axial mass, which was confirmed to be meningioma of left parasagittal area. Template seed (B) rsfMRI attained score of 1 in left motor cortex using seed based on anatomically expected location of contralateral motor cortex. Functional seed (C) rsfMRI attained score of 2 in left motor cortex using seed based on contralateral motor cortex location determined by tbfMRI. Functional seed analysis attained stronger signal than template seed analysis. Furthermore, ICA map (D) of rsfMRI attained score of 2 in left motor cortex. All images are demonstrated left-on-left (neurologic convention). ICA = independent component analysis, tbfMRI = task-based functional MRI

Fig. 3
Healthy volunteer representative case.
tbfMRI map (A) demonstrating results of left hand finger tapping to activate right motor cortex. tbfMRI map (A) was used as reference. When compared with tbfMRI (A), template seed (B) rsfMRI attained score of 1 in right motor cortex using anatomically expected left motor cortex location. When compared with tbfMRI (A), functional seed (C) rsfMRI attained score of 2 in right motor cortex using left motor cortex location determined by tbfMRI. ICA map (D) of rsfMRI attained score 2 in right motor cortex. All images are demonstrated left-on-left (neurologic convention).
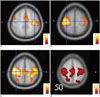
Table 1
Patient Group Characteristics

Table 2
Visual Analysis Results in Patient and Healthy Volunteer Groups
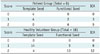
Score | Patient Group (Total = 8) | ICA | |
---|---|---|---|
Template Seed | Functional Seed | ||
0 | 4 | 0 | 0 |
1 | 4 | 2 | 3 |
2 | 0 | 6 | 5 |
Score | Patient Group (Total = 8) | ICA | |
---|---|---|---|
Template Seed | Functional Seed | ||
0 | 0 | 0 | 0 |
1 | 14 | 5 | 6 |
2 | 4 | 13 | 12 |
References
1. Lee MH, Smyser CD, Shimony JS. Resting-state fMRI: a review of methods and clinical applications. AJNR Am J Neuroradiol. 2013; 34:1866–1872.



2. Kamran M, Hacker CD, Allen MG, Mitchell TJ, Leuthardt EC, Snyder AZ, et al. Resting-state blood oxygen level-dependent functional magnetic resonance imaging for presurgical planning. Neuroimaging Clin N Am. 2014; 24:655–669.



3. Lang S, Duncan N, Northoff G. Resting-state functional magnetic resonance imaging: review of neurosurgical applications. Neurosurgery. 2014; 74:453–464. discussion 464-465.

4. Schneider FC, Pailler M, Faillenot I, Vassal F, Guyotat J, Barral FG, et al. Presurgical assessment of the sensorimotor cortex using resting-state fMRI. AJNR Am J Neuroradiol. 2016; 37:101–107.



5. Wengenroth M, Blatow M, Guenther J, Akbar M, Tronnier VM, Stippich C. Diagnostic benefits of presurgical fMRI in patients with brain tumours in the primary sensorimotor cortex. Eur Radiol. 2011; 21:1517–1525.



6. Fox MD, Greicius M. Clinical applications of resting state functional connectivity. Front Syst Neurosci. 2010; 4:19.



7. Tyndall AJ, Reinhardt J, Tronnier V, Mariani L, Stippich C. Presurgical motor, somatosensory and language fMRI: technical feasibility and limitations in 491 patients over 13 years. Eur Radiol. 2017; 27:267–278.

8. Kokkonen SM, Nikkinen J, Remes J, Kantola J, Starck T, Haapea M, et al. Preoperative localization of the sensorimotor area using independent component analysis of resting-state fMRI. Magn Reson Imaging. 2009; 27:733–740.


9. Quigley M, Cordes D, Wendt G, Turski P, Moritz C, Haughton V, et al. Effect of focal and nonfocal cerebral lesions on functional connectivity studied with MR imaging. AJNR Am J Neuroradiol. 2001; 22:294–300.

10. Shimony JS, Zhang D, Johnston JM, Fox MD, Roy A, Leuthardt EC. Resting-state spontaneous fluctuations in brain activity: a new paradigm for presurgical planning using fMRI. Acad Radiol. 2009; 16:578–583.


11. Zhang D, Johnston JM, Fox MD, Leuthardt EC, Grubb RL, Chicoine MR, et al. Preoperative sensorimotor mapping in brain tumor patients using spontaneous fluctuations in neuronal activity imaged with functional magnetic resonance imaging: initial experience. Neurosurgery. 2009; 65:6 Suppl. 226–236.



12. Otten ML, Mikell CB, Youngerman BE, Liston C, Sisti MB, Bruce JN, et al. Motor deficits correlate with resting state motor network connectivity in patients with brain tumours. Brain. 2012; 135(Pt 4):1017–1026.



13. Cordes D, Haughton VM, Arfanakis K, Wendt GJ, Turski PA, Moritz CH, et al. Mapping functionally related regions of brain with functional connectivity MR imaging. AJNR Am J Neuroradiol. 2000; 21:1636–1644.

14. Liu H, Buckner RL, Talukdar T, Tanaka N, Madsen JR, Stufflebeam SM. Task-free presurgical mapping using functional magnetic resonance imaging intrinsic activity. J Neurosurg. 2009; 111:746–754.



15. Mannfolk P, Nilsson M, Hansson H, Ståhlberg F, Fransson P, Weibull A, et al. Can resting-state functional MRI serve as a complement to task-based mapping of sensorimotor function? A test-retest reliability study in healthy volunteers. J Magn Reson Imaging. 2011; 34:511–517.


16. Rosazza C, Aquino D, D'Incerti L, Cordella R, Andronache A, Zacà D, et al. Preoperative mapping of the sensorimotor cortex: comparative assessment of task-based and resting-state FMRI. PLoS One. 2014; 9:e98860.


17. Hou BL, Bhatia S, Carpenter JS. Quantitative comparisons on hand motor functional areas determined by resting state and task BOLD fMRI and anatomical MRI for pre-surgical planning of patients with brain tumors. Neuroimage Clin. 2016; 11:378–387.



18. Rutten GJ, Ramsey NF. The role of functional magnetic resonance imaging in brain surgery. Neurosurg Focus. 2010; 28:E4.


19. Belyaev AS, Peck KK, Brennan NM, Holodny AI. Clinical applications of functional MR imaging. Magn Reson Imaging Clin N Am. 2013; 21:269–278.


20. Yahyavi-Firouz-Abadi N, Pillai JJ, Lindquist MA, Calhoun VD, Agarwal S, Airan RD, et al. Presurgical brain mapping of the ventral somatomotor network in patients with brain tumors using resting-state fMRI. AJNR Am J Neuroradiol. 2017; 38:1006–1012.



21. Sair HI, Yahyavi-Firouz-Abadi N, Calhoun VD, Airan RD, Agarwal S, Intrapiromkul J, et al. Presurgical brain mapping of the language network in patients with brain tumors using resting-state fMRI: comparison with task fMRI. Hum Brain Mapp. 2016; 37:913–923.

22. Rosazza C, Minati L, Ghielmetti F, Mandelli ML, Bruzzone MG. Functional connectivity during resting-state functional MR imaging: study of the correspondence between independent component analysis and region-of-interest-based methods. AJNR Am J Neuroradiol. 2012; 33:180–187.



23. Joel SE, Caffo BS, van Zijl PC, Pekar JJ. On the relationship between seed-based and ICA-based measures of functional connectivity. Magn Reson Med. 2011; 66:644–657.



24. Biswal B, Yetkin FZ, Haughton VM, Hyde JS. Functional connectivity in the motor cortex of resting human brain using echo-planar MRI. Magn Reson Med. 1995; 34:537–541.


25. Margulies DS, Böttger J, Long X, Lv Y, Kelly C, Schäfer A, et al. Resting developments: a review of fMRI post-processing methodologies for spontaneous brain activity. MAGMA. 2010; 23:289–307.

