Abstract
Purpose
The aim of this study was to evaluate the diagnostic performance of breast ultrasound (US) computer-aided diagnosis (CAD) to distinguish between benign and malignant lesions and analyze features of lesions interpreted with errors retrospectively.
Materials and Methods
Three hundred and sixteen women with 375 breast lesions were enrolled. We assessed the accuracy, sensitivity, specificity, positive predictive value (PPV) and negative predictive value (NPV). Additionally, we evaluated the causes and patterns of the misinterpretation in the false positive and negative groups.
Results
The accuracy, sensitivity, specificity, PPV, and NPV of breast US-CAD were 80.3%, 83.3%, 79.8%, 37.7%, and 97.0%, respectively. There were 8 false negative lesions that were oval in shape and in parallel orientation. There were 66 false positive lesions. The greatest number of errors entailed inappropriate demarcation due to heterogeneous echogenicity, etc. The second exhibited suspicious features with good demarcation and description but were confirmed as benign histologically. The third entailed a benign lesion with suspicious features, such as abscesses. The smallest portion with good demarcations and descriptions indicating benign status exhibited possible malignancy as a final conclusion.
REFERENCES
1.Wang X., Guo Y., Wang Y. Automatic detection of regions of interest in breast ultrasound images based on local phase information. Biomed Mater Eng. 2015. 26(Suppl 1):S1265-S1273.


2.Jung KW., Won YJ., Oh CM., Kong HJ., Lee DH., Lee KH. Cancer statistics in Korea: incidence, mortality, survival, and prevalence in 2014. Cancer Res Treat. 2017. 49:292–305.


3.Chabi ML., Borget I., Ardiles R., Aboud G., Boussouar S., Vilar V, et al. Evaluation of the accuracy of a computer-aided diagnosis (CAD) system in breast ultrasound according to the radiologist's experience. Acad Radiol. 2012. 19:311–319.


4.Kim K., Song MK., Kim EK., Yoon JH. Clinical application of S-Detect to breast masses on ultrasonography: a study evaluating the diagnostic performance and agreement with a dedicated breast radiologist. Ultrasonography. 2017. 36:3–9.


5.Chen DR., Chien CL., Kuo YF. Computer-aided assessment of tumor grade for breast cancer in ultrasound images. Comput Math Methods Med. 2015. 2015:914091.


6.Moon WK., Huang YS., Lo CM., Huang CS., Bae MS., Kim WH, et al. Computer-aided diagnosis for distinguishing between triple-negative breast cancer and fibroadenomas based on ultrasound texture features. Med Phys. 2015. 42:3024–3035.


7.Song SE., Seo BK., Cho KR., Woo OH., Son GS., Kim C, et al. Computer-aided detection (CAD) system for breast MRI in assessment of local tumor extent, nodal status, and multifo-cality of invasive breast cancers: preliminary study. Cancer Imaging. 2015. 15:1.


8.Shan J., Alam SK., Garra B., Zhang Y., Ahmed T. Computer-aided diagnosis for breast ultrasound using computerized BI-RADS features and machine learning methods. Ultrasound Med Biol. 2016. 42:980–988.


9.Cho E., Kim EK., Song MK., Yoon JH. Application of computer-aided diagnosis on breast ultrasonography: evaluation of diagnostic performances and agreement of radiologists according to different levels of experience. J Ultrasound Med. 2018. 37:209–216.


10.Choi JH., Kang BJ., Baek JE., Lee HS., Kim SH. Application of computer-aided diagnosis in breast ultrasound interpretation: improvements in diagnostic performance according to reader experience. Ultrasonography. 2017. 37:217–225.


11.Lee SE., Moon JE., Rho YH., Kim EK., Yoon JH. Which supplementary imaging modality should be used for breast ultrasonography? Comparison of the diagnostic performance of elastography and computer-aided diagnosis. Ultrasonography. 2017. 36:153–159.


12.Wang Y., Jiang S., Wang H., Guo YH., Liu B., Hou Y, et al. CAD algorithms for solid breast masses discrimination: evaluation of the accuracy and interobserver variability. Ultrasound Med Biol. 2010. 36:1273–1281.


13.Dromain C., Boyer B., Ferré R., Canale S., Delaloge S., Balleygui-er C. Computed-aided diagnosis (CAD) in the detection of breast cancer. Eur J Radiol. 2013. 82:417–423.


14.Landis JR., Koch GG. The measurement of observer agreement for categorical data. Biometrics. 1977. 33:159-174.


15.Tan T., Platel B., Twellmann T., van Schie G., Mus R., Grivegnée A, et al. Evaluation of the effect of computer-aided classification of benign and malignant lesions on reader performance in automated three-dimensional breast ultrasound. Acad Radiol. 2013. 20:1381–1388.
16.Lehman CD., Wellman RD., Buist DS., Kerlikowske K., Tosteson AN., Miglioretti DL. Diagnostic accuracy of digital screening mammography with and without computer-aided detection. JAMA Intern Med. 2015. 175:1828–1837.


Fig. 1
Imaging findings from a 56-year-old woman who had ductal carcinoma in situ, an example of a false negative interpretation of CAD. CAD interpretation of this lesion as possibly benign with oval parallel, circumscribed hypoechoic features. CAD misinterpretation of ‘well-circumscribed’ breast carcinomas as benign lesions. CAD = computer-aided diagnosis
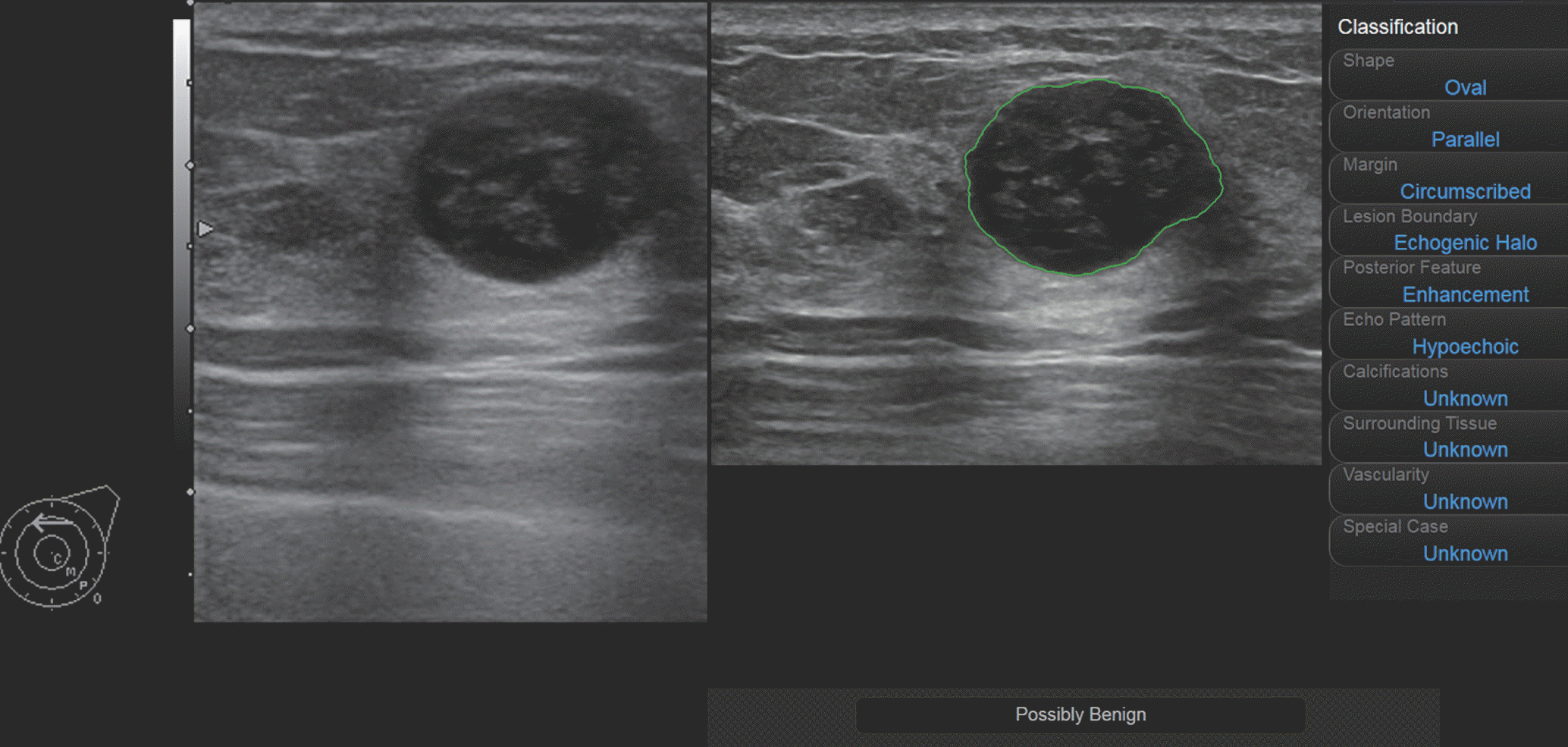
Fig. 2
Imaging findings from a 60-year-old woman who had an involuting fibroadenoma, an example of first type error of a false positive interpretation of CAD. This breast lesion was known as an involuting fibroadenoma. Heterogeneous echogenicity of this mass is applied to CAD, and due to its heterogeneity, the lesion shape is read as an irregular shape with a microlobulated margin, and the final assessment is possibly malignancy. CAD = computer-aided diagnosis
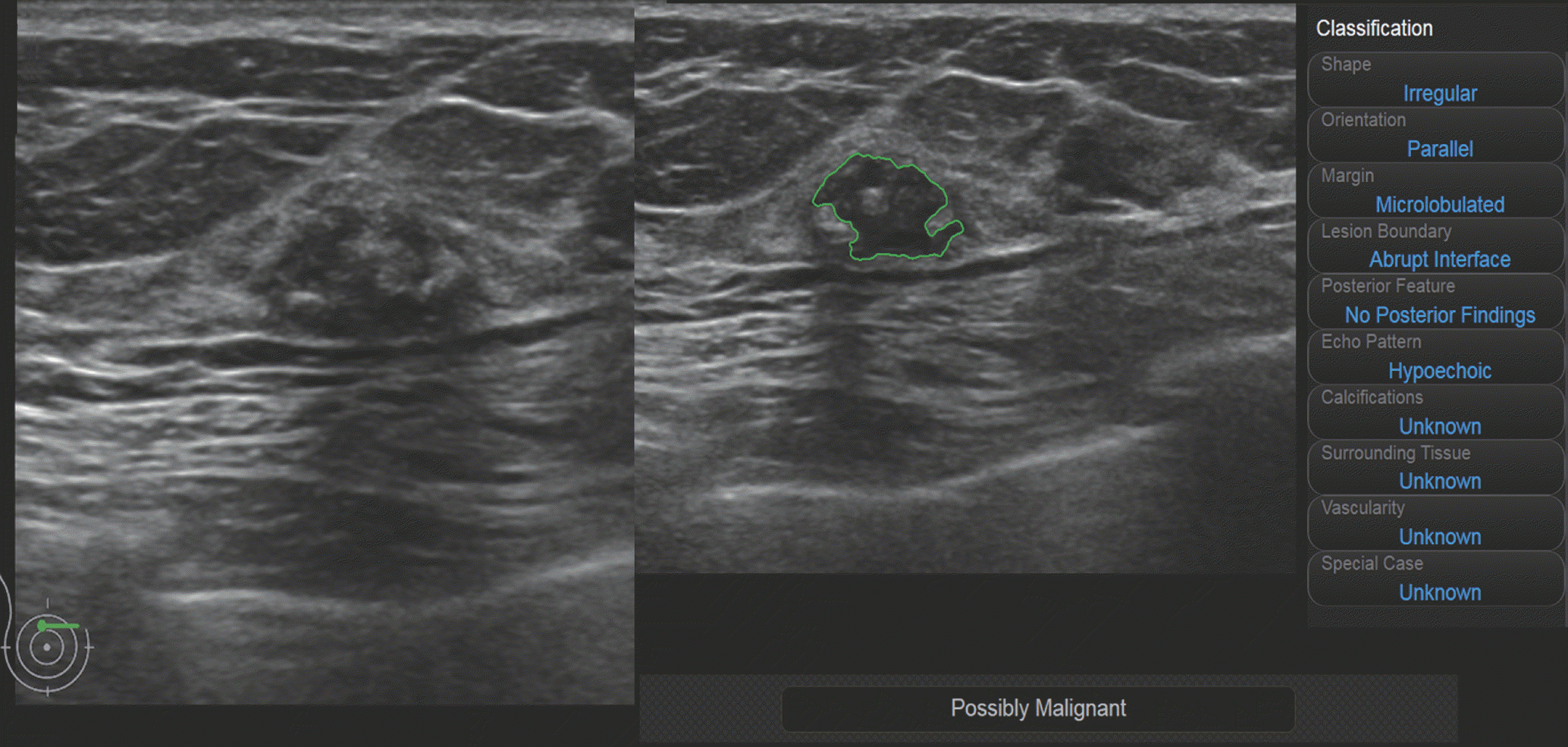
Fig. 3
Imaging findings from a 41-year-old woman who had fibrocystic change, an example of second type error of false positive interpretation of CAD. An irregular-shaped not parallel hypoechoic lesion is found on US. CAD reveals proper demarcation with irregular, not parallel, spiculated hypoechoic description with possible malignant conclusion. Due to its suspicious features, US-guided biopsy is found on US performed, and fibrocystic change is found on US confirmed. It is found on US an inevitable false positive case in the clinical setting. CAD = computer-aided diagnosis, US = ultrasound
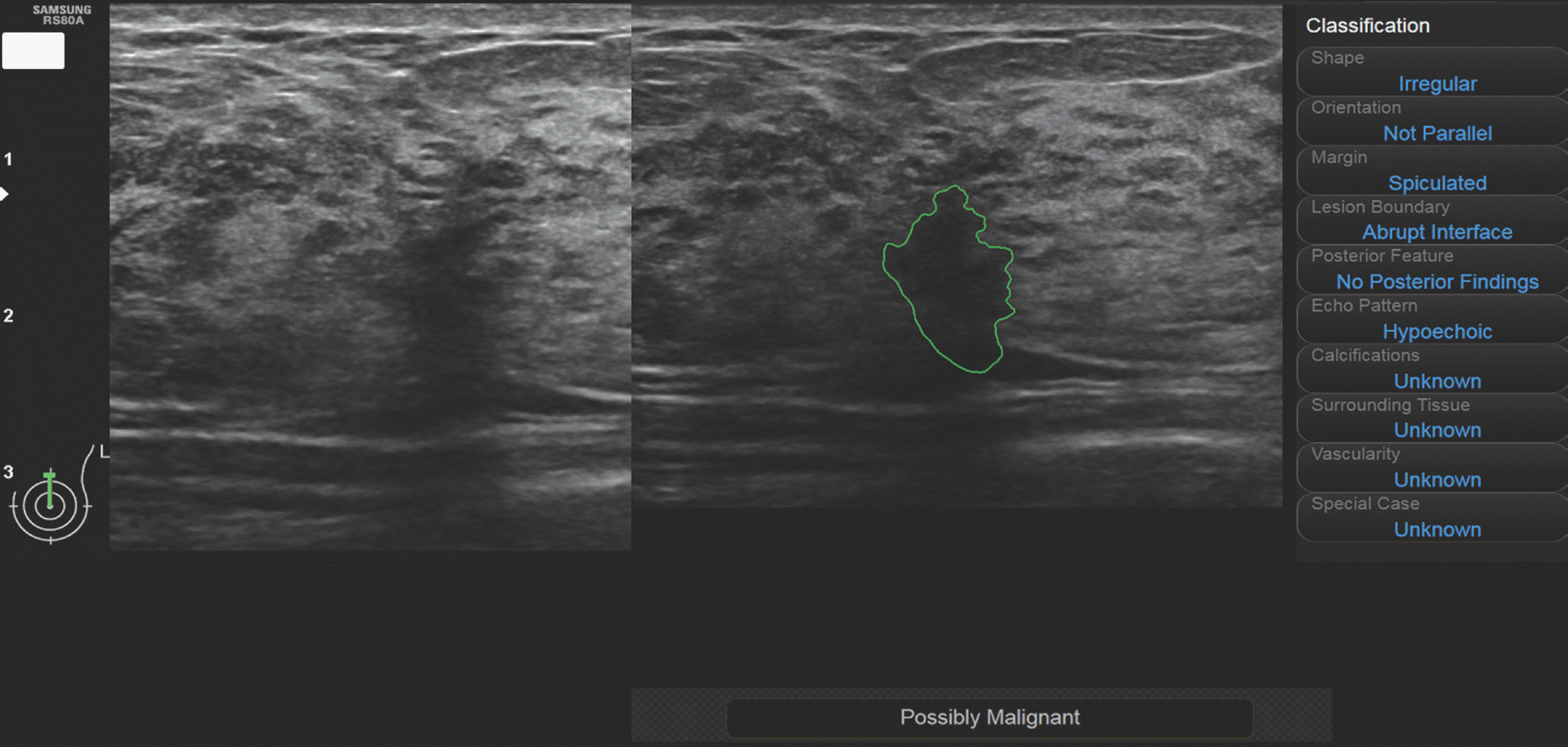
Fig. 4
Imaging findings from a 61-year-old woman who had fibrocystic change, which is an example of fourth type error of false positive interpretation on CAD. US reveals an oval parallel circumscribed hypoechoic mass. CAD also describes this lesion as an oval parallel circumscribed hypoechoic mass without posterior features. However, the final assessment was possible malignancy. A US-guided biopsy was performed and fibrocystic change was confirmed. This was a real error in the interpretation of CAD. CAD = computer-aided diagnosis, US = ultrasound
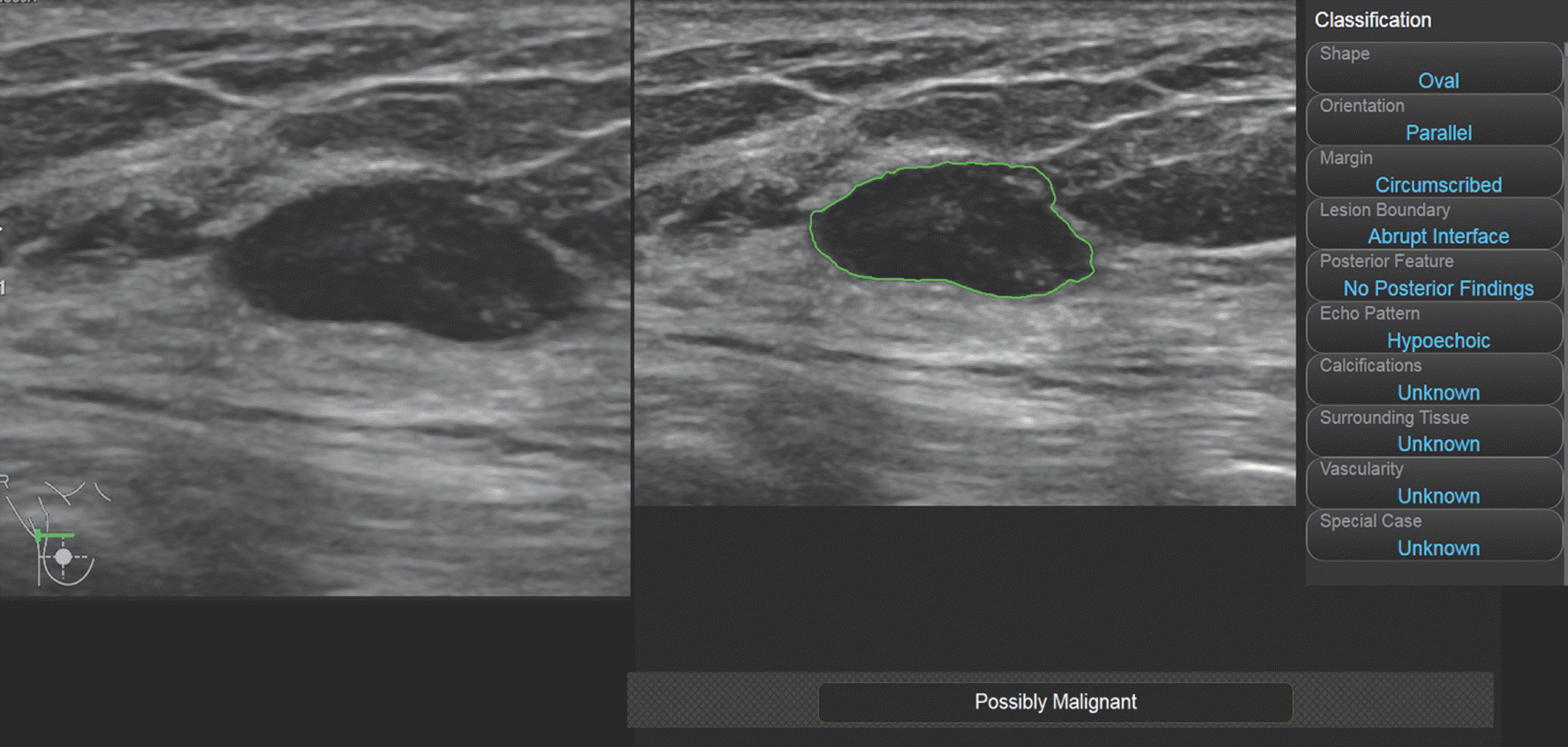
Table 1.
Characteristics of 327 Benign Lesions and 48 Malignant Lesions
Table 2.
Interobserver Agreement between Radiologists and CAD System
Table 3.
Diagnostic Performance of CAD System and Radiologists
Table 4.
Patterns of False Negative Interpretations of CAD System