Abstract
Objective
To prospectively evaluate the diagnostic performance of computer-aided diagnosis (CAD) for detection of thyroid cancers via ultrasonography (US).
Materials and Methods
This study included 50 consecutive patients with 117 thyroid nodules on US during the period between June 2016 and July 2016. A radiologist performed US examinations using real-time CAD integrated into a US scanner. We compared the diagnostic performance of radiologist, the CAD system, and the CAD-assisted radiologist for the detection of thyroid cancers.
Results
The sensitivity, specificity, positive predictive value (PPV), negative predictive value (NPV), and accuracy of the CAD system were 80.0, 88.1, 83.3, 85.5, and 84.6%, respectively, and were not significantly different from those of the radiologist (p > 0.05). The CAD-assisted radiologist showed improved diagnostic sensitivity compared with the radiologist alone (92.0% vs. 84.0%, p = 0.037), while the specificity and PPV were reduced (85.1% vs. 95.5%, p = 0.005 and 82.1% vs. 93.3%, p = 0.008). The radiologist assisted by the CAD system exhibited better diagnostic sensitivity and NPV than the CAD system alone (92.0% vs. 80.0%, p = 0.009 and 93.4% vs. 88.9%, p = 0.013), while the specificities and PPVs were not significantly different (88.1% vs. 85.1%, p = 0.151 and 83.3% vs. 82.1%, p = 0.613, respectively).
Thyroid nodules are prevalent in 19–68% of the healthy population (1). Ultrasonography (US) is the primary diagnostic tool to assess the risk of malignancy in patients with a suspected thyroid nodule and facilitate the decision-making for fine-needle aspiration (FNA) (234). However, the diagnostic performance of US varies, with the sensitivity of thyroid cancer detection ranging from 52% to 81% and the specificity from 54% to 83%. Since interobserver variability in interpreting the US characteristics was moderate to substantial in previous studies, unnecessary FNAs, and even diagnostic surgery are common in clinical practice, resulting in a significant burden on healthcare systems and in patient anxiety (5678910).
A computer-aided diagnosis (CAD) system for thyroid nodules on US has been introduced recently for accurate and consistent interpretation of US features and, to potentially reduce unnecessary FNAs by semi-automating the workflow (11121314151617). Several studies reported promising results of the CAD system, suggesting tremendous diagnostic potential. Few studies reported high diagnostic accuracy of the CAD system similar to that of an experienced radiologist. However, it has not been utilized in a clinical setting, because it is not available commercially (11121314151617). A new CAD system, integrated into a commercially available US platform, has recently been proposed. Furthermore, a study reported its potential benefit in clinical practice to date (18). However, no study has evaluated the role of the new CAD system as an adjunct to radiologists for real-time risk assessment of malignancy in patients with thyroid nodules.
The purpose of this study was to prospectively evaluate the diagnostic performance of the CAD system in thyroid cancer and to assess its potential role in decision-making alongside radiologists.
This prospective study was approved by our Institutional Review Board and written informed consent was obtained from all patients before they underwent US. Between June 2016 and July 2016, a total of 50 consecutive patients with 117 thyroid nodules (≥ 5 mm in diameter), who underwent US-guided FNA or US examination prior to scheduled surgery, were enrolled (10 males and 40 females; mean age, 43.2 years; age range, 22–81 years).
A malignant nodule was diagnosed in the surgical specimen. A benign nodule was diagnosed based on any of the following criteria: 1) confirmation of benign status in a surgical specimen; 2) benign core-needle biopsy, histology, or cytologically benign FNA; or 3) benign traits including spongiform or partially cystic nodules with comet tail artifacts, or pure cysts evident on US.
All US examinations were performed using a 5–12 MHz linear probe and a real-time US system (RS80A; Samsung Medison Co., Ltd., Seoul, Korea). The real-time CAD system (S-Detect for Thyroid; Samsung Medison Co., Ltd.) was integrated into the US system. A radiologist specializing in thyroid imaging (with 10 years of clinical experience in the performance and evaluation of thyroid US data) performed all US examinations.
Computer-aided diagnosis data were determined from transverse planes by manually setting a region of interest around the lesion. The software automatically calculated the mass contours and evaluated the US features of the mass including composition (solid, partially cystic, or cystic), shape (oval-to-round or irregular), orientation (parallel or non-parallel), margins (well-defined, ill-defined, or spiculated), and echogenicity (hyperechoic/isoechoic or hypoechoic/markedly hypoechoic); and spongiform status. In terms of margins, the operator selected one of the four options suggested by the software. The nodule was finally diagnosed, in real time, as benign or malignant (Fig. 1).
Grayscale US images were evaluated by the radiologist according to Korean guidelines based on size, internal content, echogenicity, shape, orientation, margin, and calcifications (4). The nodule contents were categorized as solid (no obvious cystic content), predominantly solid (< 50% cystic), predominantly cystic (> 50% cystic), or cystic (pure cyst or almost entirely cystic content). The predominant echogenicity was categorized as hypoechogenicity (marked or mild), isoechogenicity, or hyperechogenicity with reference to the normal portion of the thyroid gland and the anterior neck muscle. Shape was categorized as ovoid-to-round or irregular, with a parallel (when the anteroposterior diameter of the nodule was equal to or less than the transverse or longitudinal diameter) or non-parallel (when the anteroposterior diameter of the nodule was longer than the transverse or longitudinal diameter in the transverse or longitudinal plane, respectively) orientation. The margins were categorized as smooth, spiculated/microlobulated, or ill-defined. Calcification was classified into: none; microcalcification (tiny, punctate echogenic foci of 1 mm or less in diameter, with or without posterior shadowing); macrocalcification (echogenic foci larger than 1 mm in diameter); and rim calcification (peripheral curvilinear or eggshell-like calcification).
Differences in patient demographics, grayscale US features, and CAD diagnoses (benign and malignant) were evaluated using the χ2 or Fisher's exact test. Student's t test was used to compare quantitative variables.
The diagnostic performance of the CAD system, the radiologist, and the CAD-assisted radiologist for thyroid cancer, was evaluated based on the sensitivities, specificities, positive predictive values (PPVs), negative predictive values (NPVs), and accuracy rates; and compared using a generalized equation method. The areas under receiver operating characteristic (ROC) curve (AUC), with 95% confidence intervals (CIs), were calculated. The diagnostic performance of the radiologist assisted by the CAD system was defined as positive when the criteria meet one of the two categories: the radiologist and the CAD system.
The extent of interobserver agreement (the kappa value) between the CAD system and the radiologist in terms of descriptions of the US characteristics was determined. The level of agreement for Cohen's kappa was defined as follows: < 0.20, poor; 0.21–0.40, fair; 0.41–0.60, moderate; 0.61–0.80, substantial; and > 0.80, good agreement.
All statistical analyses were performed using SPSS for Windows (ver. 23.0; IBM Corp., Armonk, NY, USA) and SAS for Windows software (ver. 9.2; SAS Institute, Cary, NC, USA). A significant difference was defined as a p value < 0.05.
The mean nodule diameter was 1.5 ± 1.1 cm (range: 0.5–10.0 cm). The final diagnosis of the 117 nodules was: 67 (57.3%) benign and 50 (42.7%) malignant. All malignant diagnoses were made after surgical resection, and included 41 classical papillary thyroid carcinomas (PTCs), 8 follicular variant PTCs, and 1 hobnail variant PTC. The 53 surgically confirmed benign nodules were all nodular hyperplasias.
The US features of the benign and malignant nodules are summarized in Table 1. The mean diameter of the benign nodules was 1.2 ± 1.0 cm, which was not statistically different from that of the malignant nodules (1.1 ± 0.8 cm; p = 0.616). Alongside the US features, including solid component, marked hypoechogenicity, a non-parallel orientation, spiculated margins, and microcalcification, the “probably malignant” diagnosis based on the CAD system was a significant factor in the detection of thyroid cancers (p < 0.001).
Table 2 summarizes the diagnostic performance of the CAD system, the radiologist, and the CAD-assisted radiologist in thyroid cancer. CAD exhibited statistically insignificant difference in terms of sensitivity and specificity compared with the radiologist (80.0% vs. 84.0%, p = 0.525; 88.1% vs. 95.5%, p = 0.089, respectively); while the radiologist tended to show a higher diagnostic sensitivity and specificity than the CAD system. Diagnostic accuracy did not differ significantly between the CAD system and the radiologist (84.6% vs. 90.6%, p = 0.646) (Figs. 2, 3).
When the CAD system was used to assist the radiologist, the diagnostic sensitivity improved (92.0% vs. 84.0%, p = 0.037) whereas the specificity and the PPV declined (85.1% vs. 95.5%, p = 0.005; 82.1% vs. 93.3%, p = 0.008). However, the radiologist assisted by CAD resulted in a significant increase in the diagnostic sensitivity and NPV compared with that of the CAD system alone (92.0% vs. 80.0%, p = 0.009; 93.4% vs. 85.5%, p = 0.013), while the specificity and PPV were not statistically different (85.1% vs. 88.1%, p = 0.151; 82.1% vs. 83.3%, p = 0.613) (Fig. 4).
Figure 5 shows the ROC curves for the CAD system, the radiologist, and the radiologist assisted by CAD, in terms of differentiation of benign from malignant nodules. The AUCs were 0.840 (95% CI, 0.761–0.901) for the CAD system, 0.898 (0.828–0.946) for the radiologist, and 0.885 (0.813–0.937) for the CAD-assisted radiologist; these values did not differ significantly (p > 0.05).
The extent of agreement between the CAD system and the radiologist was 83.8% (98/117). The extent of interobserver agreement was good (kappa = 0.661) and the extent of interobserver agreement in terms of US characteristics was fair-to-substantial (Table 3). The extent of disagreement was 16.2% (19/117, 10 malignant and 9 benign nodules). Among the 10 malignant nodules, the radiologist missed 4 cancers (2 PTCs and 2 follicular variant PTCs) without suspicious US features. The CAD system missed 6 cancers (5 PTCs and 1 follicular variant PTC) with suspicious US features.
This study demonstrated that the performance of CAD in thyroid cancer was good (80.0% sensitivity and 88.1% specificity) and was not significantly different from that of the radiologist. Although the radiologist assisted by CAD showed an increase in sensitivity of up to 92.0%, the specificity and PPV were lower compared with those of the radiologist alone. The CAD-assisted radiologist exhibited better sensitivity and NPV without significant reductions in specificity and PPV compared with the CAD system alone.
The widespread use of US in thyroid disease diagnosis has greatly increased the detection rate of thyroid nodules. Consistent with this finding, several US features were strongly associated with thyroid cancer such as microcalcifications, spiculated or microlobulated margins, and a taller-than-wide shape (1920). Therefore, the current guidelines suggest that US is indicated primarily for thyroid cancer diagnosis (234). However, US is of limited use since the diagnostic performance of US is mainly affected by physician experience and interobserver variabilities are non-negligible (5678910). The diagnostic performance of less-experienced physicians is less accurate than that of experienced physicians and, unnecessary FNAs are routinely performed in practice. In addition, although human brain is quite adept at matching the patterns of benign and malignant nodules, no single US feature is highly predictive of malignancy. The thyroid CAD system using artificial intelligence might be an option to resolve this problem, with potential ability to handle essentially infinite number of possible sonographic configurations of thyroid nodules. Further investigation is necessary to validate its diagnostic performance in different clinical settings in the future (11).
The CAD system for thyroid nodules on US was initially reported by Lim et al. (11) in 2008. Since the diagnostic performance of the CAD system used an artificial neural network (11), several studies reported that the CAD system yielded an accuracy of up to 98.3% (12131415). However, most of these studies were not conducted in a clinical setting, and they were preclinical in nature without involving radiologists. A recent study by Choi et al. (18) initially reported the utility of this new commercially available CAD system in a clinical setting. They reported that the diagnostic sensitivity of the CAD system was comparable to that of the radiologist (88.4% vs. 90.7%, p > 0.99), but the specificity and AUC curve were lower (specificity: 74.6% vs. 94.9%, p = 0.002; AUC: 0.83 vs. 0.92, p = 0.021). In our study, the diagnostic performance of the radiologist was similar to that of Choi et al. (18), although the specificity and sensitivity of the CAD system was slightly lower than reported. Therefore, the radiologist tended to exhibit higher diagnostic sensitivity, specificity, and accuracy, without any statistically significant difference. The interobserver agreement between the CAD system and the radiologist was substantial for the final diagnosis. However, similar to the study by Choi et al. (18), the interobserver agreement for the description of margin was the lowest and remained fair. The individual US features interpreted by the CAD system require improvement, especially for the margin.
Although the diagnostic performance of the CAD system was not significantly different from that of the radiologist, the extent of disagreement was 16.2% (19/117). The characteristics of nodules that are diagnosed differently by the CAD system and radiologist have yet to be elucidated. However, in our study, the radiologist missed 16.0% (8/50) of cancers that lacked suspicious US features, including 62.5% (5/8) follicular variants of PTCs. On the other hand, the CAD system missed 20.0% (10/50) of cancers, 60% (6/10) of which were classical PTCs. Although three follicular variant PTCs and one classical PTC were missed by both the radiologist and the CAD system, the CAD prevented delayed diagnosis of two follicular variant PTCs and two classical PTCs without suspicious US features. Therefore, when the CAD system detects malignancy without suspicious features, the possibility of follicular variant PTCs may be considered. Further studies are required to validate the role of CAD in detecting follicular variant PTC or follicular neoplasm in large populations.
The study suggests three clinical implications. First, the diagnostic performance of the CAD system was not significantly different from that of the radiologist, which indicates the role of CAD as a potential decision-making aid for a beginner or non-thyroid radiologist. Second, the CAD system-assisted radiologist yielded a higher diagnostic sensitivity than the radiologist alone, although the specificity and PPV were lower. This finding implied that the CAD system allows the radiologist to detect a higher proportion of genuine malignancies. However, the radiological diagnosis is preferable to minimize unnecessary FNAs for the discordant cases, and FNA may be selectively considered for these nodules considering the nodule size and clinical risk factors. Third, the CAD-assisted radiologist showed a higher diagnostic sensitivity and NPV than the CAD system alone, without significant reductions in specificity and PPV. Thus, the performance of CAD system is improved in the hands of a radiologist.
Our study had several limitations. First, in this pilot study, the sample size was small and there may have been a selection bias. Second, we included nodules subjected to US-guided FNA or US examination prior to scheduled surgery. Therefore, the proportion of malignancies was rather high, which may have influenced the diagnostic performance of the CAD system. Third, most of the malignancies were classical PTCs. As the US features of follicular variant PTCs, follicular carcinomas, and other malignancies differ somewhat from those of classical PTC, large population studies are required. Fourth, the CAD system failed to evaluate calcification. Further technical developments are needed to improve the performance of the CAD system. Fifth, we defined the diagnostic performance of the CAD-aided radiologist as positive when the criteria associated with the radiologist or the CAD system, were fulfilled. The actual impact of the CAD system alongside the radiologist should be validated in the future.
In conclusion, the diagnostic performance of the CAD system was not significantly different from that of the radiologist and the CAD-assisted radiologist showed the highest diagnostic sensitivity. Therefore, the CAD system may have a potential supporting role in decision-making alongside radiologists in the thyroid cancer diagnosis.
References
1. Brander A, Viikinkoski P, Nickels J, Kivisaari L. Thyroid gland: US screening in a random adult population. Radiology. 1991; 181:683–687. PMID: 1947082.


2. Haugen BR. 2015 American Thyroid Association management guidelines for adult patients with thyroid nodules and differentiated thyroid cancer: what is new and what has changed? Cancer. 2017; 123:372–381. PMID: 27741354.


3. Camacho PM, Petak SM, Binkley N, Clarke BL, Harris ST, Hurley DL, et al. American Association of Clinical Endocrinologists and American College of Endocrinology clinical practice guidelines for the diagnosis and treatment of postmenopausal osteoporosis - 2016. Endocr Pract. 2016; 22(Suppl 4):1–42.


4. Shin JH, Baek JH, Chung J, Ha EJ, Kim JH, Lee YH, et al. Korean Society of Thyroid Radiology (KSThR) and Korean Society of Radiology. Ultrasonography diagnosis and imaging-based management of thyroid nodules: revised Korean Society of Thyroid Radiology consensus statement and recommendations. Korean J Radiol. 2016; 17:370–395. PMID: 27134526.


5. Ko SY, Kim EK, Sung JM, Moon HJ, Kwak JY. Diagnostic performance of ultrasound and ultrasound elastography with respect to physician experience. Ultrasound Med Biol. 2014; 40:854–863. PMID: 24315394.


6. Kim HG, Kwak JY, Kim EK, Choi SH, Moon HJ. Man to man training: can it help improve the diagnostic performances and interobserver variabilities of thyroid ultrasonography in residents? Eur J Radiol. 2012; 81:e352–e356. PMID: 22137098.


7. Choi SH, Kim EK, Kwak JY, Kim MJ, Son EJ. Interobserver and intraobserver variations in ultrasound assessment of thyroid nodules. Thyroid. 2010; 20:167–172. PMID: 19725777.


8. Park CS, Kim SH, Jung SL, Kang BJ, Kim JY, Choi JJ, et al. Observer variability in the sonographic evaluation of thyroid nodules. J Clin Ultrasound. 2010; 38:287–293. PMID: 20544863.


9. Park SH, Kim SJ, Kim EK, Kim MJ, Son EJ, Kwak JY. Interobserver agreement in assessing the sonographic and elastographic features of malignant thyroid nodules. AJR Am J Roentgenol. 2009; 193:W416–W423. PMID: 19843721.


10. Park SJ, Park SH, Choi YJ, Kim DW, Son EJ, Lee HS, et al. Interobserver variability and diagnostic performance in US assessment of thyroid nodule according to size. Ultraschall Med. 2012; 33:E186–E190. PMID: 23108925.


11. Lim KJ, Choi CS, Yoon DY, Chang SK, Kim KK, Han H, et al. Computer-aided diagnosis for the differentiation of malignant from benign thyroid nodules on ultrasonography. Acad Radiol. 2008; 15:853–858. PMID: 18572120.


12. Chang Y, Paul AK, Kim N, Baek JH, Choi YJ, Ha EJ, et al. Computer-aided diagnosis for classifying benign versus malignant thyroid nodules based on ultrasound images: a comparison with radiologist-based assessments. Med Phys. 2016; 43:554. PMID: 26745948.


13. Li LN, Ouyang JH, Chen HL, Liu DY. A computer aided diagnosis system for thyroid disease using extreme learning machine. J Med Syst. 2012; 36:3327–3337. PMID: 22327384.


14. Acharya UR, Faust O, Sree SV, Molinari F, Garberoglio R, Suri JS. Cost-effective and non-invasive automated benign and malignant thyroid lesion classification in 3D contrast-enhanced ultrasound using combination of wavelets and textures: a class of ThyroScan™ algorithms. Technol Cancer Res Treat. 2011; 10:371–380. PMID: 21728394.
15. Acharya UR, Faust O, Sree SV, Molinari F, Suri JS. ThyroScreen system: high resolution ultrasound thyroid image characterization into benign and malignant classes using novel combination of texture and discrete wavelet transform. Comput Methods Programs Biomed. 2012; 107:233–241. PMID: 22054816.


16. Acharya UR, Sree SV, Krishnan MM, Molinari F, Zieleźnik W, Bardales RH, et al. Computer-aided diagnostic system for detection of Hashimoto thyroiditis on ultrasound images from a Polish population. J Ultrasound Med. 2014; 33:245–253. PMID: 24449727.


17. Acharya UR, Vinitha Sree S, Krishnan MM, Molinari F, Garberoglio R, Suri JS. Non-invasive automated 3D thyroid lesion classification in ultrasound: a class of ThyroScan™ systems. Ultrasonics. 2012; 52:508–520. PMID: 22154208.


18. Choi YJ, Baek JH, Park HS, Shim WH, Kim TY, Shong YK, et al. A computer-aided diagnosis system using artificial intelligence for the diagnosis and characterization of thyroid nodules on ultrasound: initial clinical assessment. Thyroid. 2017; 27:546–552. PMID: 28071987.


19. Ha EJ, Moon WJ, Na DG, Lee YH, Choi N, Kim SJ, et al. A multicenter prospective validation study for the Korean thyroid imaging reporting and data system in patients with thyroid nodules. Korean J Radiol. 2016; 17:811–821. PMID: 27587972.


20. Moon WJ, Jung SL, Lee JH, Na DG, Baek JH, Lee YH, et al. Thyroid Study Group, Korean Society of Neuro- and Head and Neck Radiology. Benign and malignant thyroid nodules: US differentiation--multicenter retrospective study. Radiology. 2008; 247:762–770. PMID: 18403624.


Fig. 1
US image of thyroid nodule acquired via CAD system.
A. Solid hypoechoic nodule with suspicious US features is evident in left thyroid gland. Region of interest is manually drawn around lesion. B. CAD software automatically calculates mass contours and presents US features on right of screen, and possible diagnosis as malignant nodule at bottom. CAD = computer-aided diagnosis, US = ultrasonography
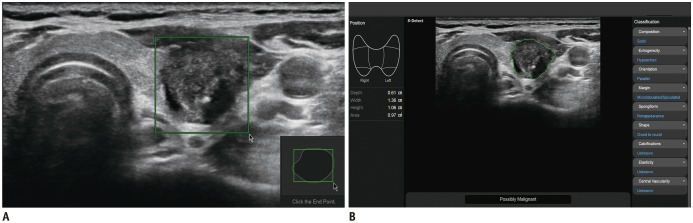
Fig. 2
53-year-old woman with bilateral thyroid nodules.
A. US images show solid isoechoic nodule without suspicious US features in right thyroid gland. Radiological diagnosis suggested benign nodule. B. CAD system presented possible diagnosis of benign nodule. Histology confirmed adenomatous hyperplasia.
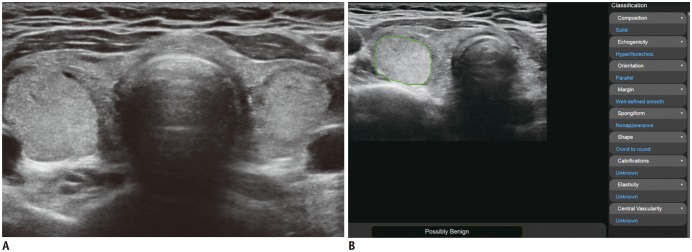
Fig. 3
47-year-old woman with right thyroid nodule.
A. US images show solid hypoechoic nodule with suspicious US features in right thyroid gland. Radiological diagnosis suggested malignant nodule. B. CAD system presented possible diagnosis as malignant nodule. Histology confirmed diagnosis of papillary thyroid carcinoma.
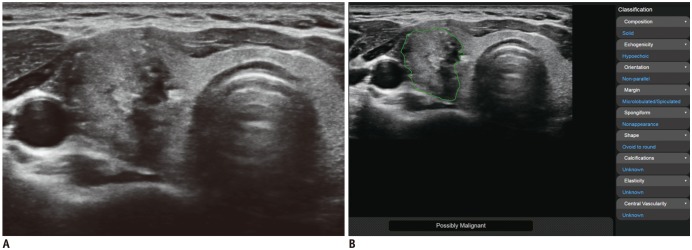
Fig. 4
36-year-old woman with left thyroid nodule.
A. US images show solid isoechoic nodule with thick peripheral halo in left thyroid gland. Radiologist diagnosed it as benign nodule. B. CAD system suggested possible diagnosis of malignant nodule following US misdiagnosis. Histology confirmed diagnosis of adenomatous hyperplasia.
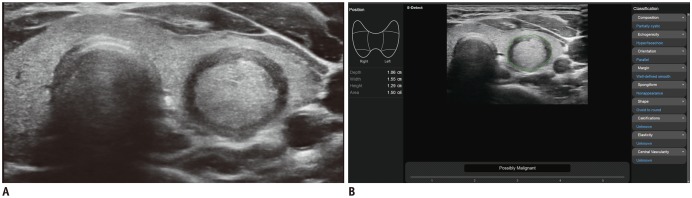
Fig. 5
Comparison of receiver operating characteristic curves for CAD, radiologist, and CAD-assisted radiologist in thyroid cancer diagnosis.
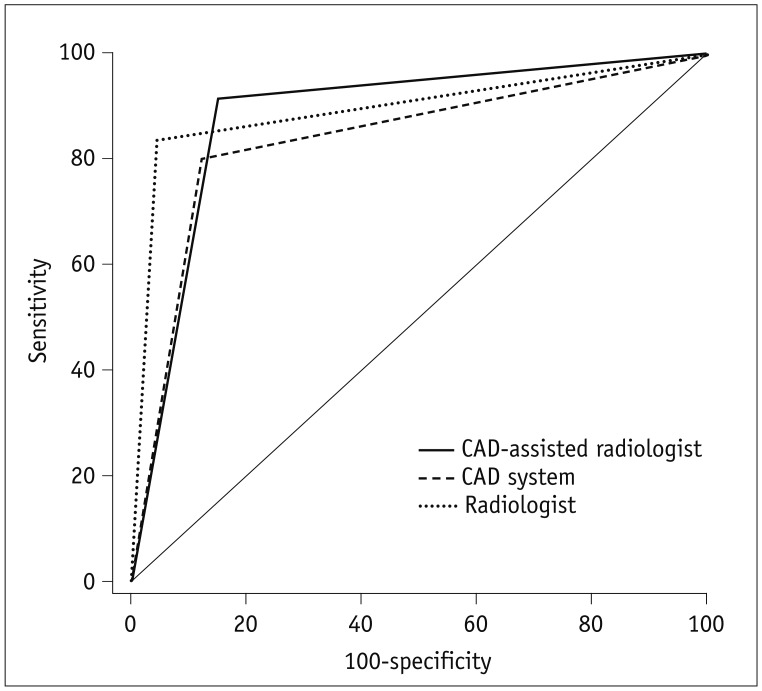
Table 1
Clinical and Sonographic Features of Benign and Malignant Thyroid Nodules
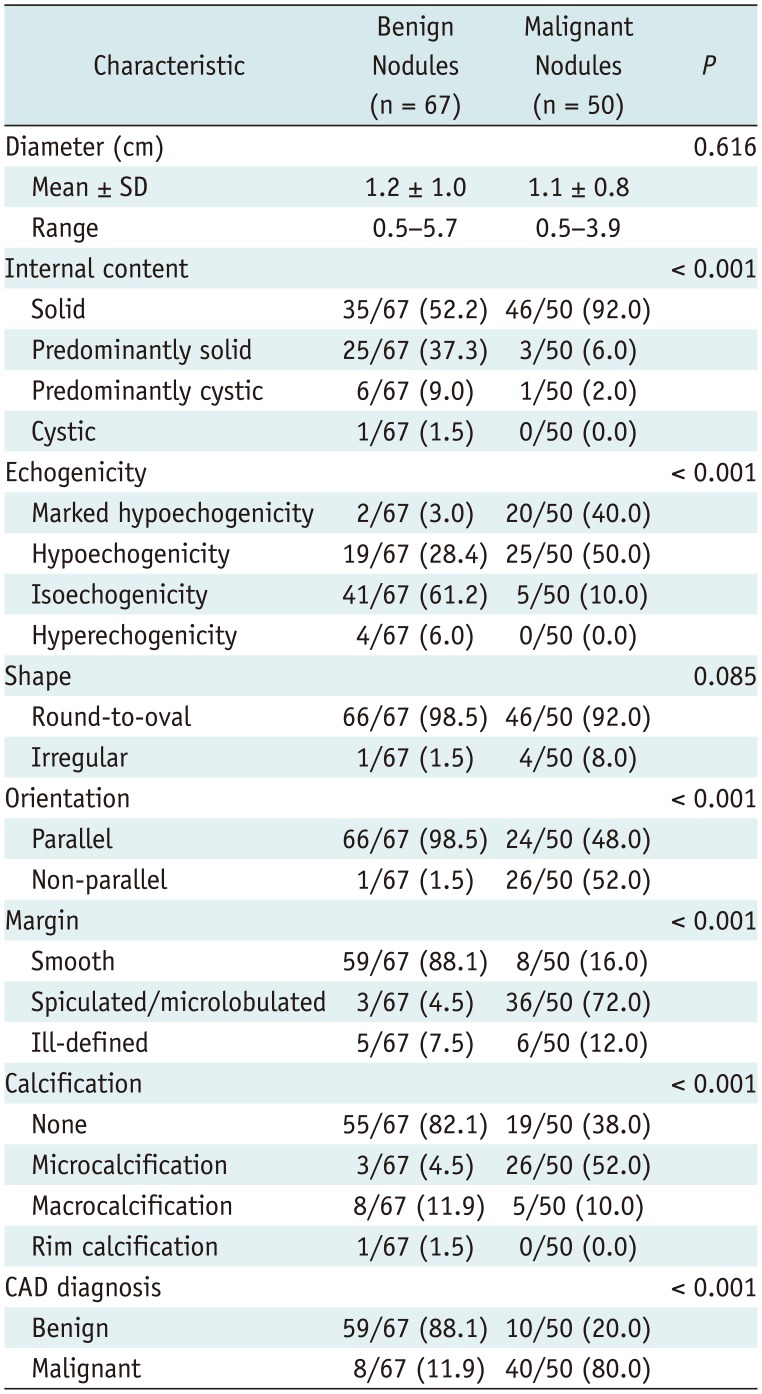
Table 2
Diagnostic Performance of CAD System and Radiologist
