Abstract
Diffusion-tensor imaging (DTI) is a noninvasive medical imaging tool used to investigate the structure of white matter. The signal contrast in DTI is generated by differences in the Brownian motion of the water molecules in brain tissue. Postprocessed DTI scalars can be used to evaluate changes in the brain tissue caused by disease, disease progression, and treatment responses, which has led to an enormous amount of interest in DTI in clinical research. This review article provides insights into DTI scalars and the biological background of DTI as a relatively new neuroimaging modality. Further, it summarizes the clinical role of DTI in various disease processes such as amyotrophic lateral sclerosis, multiple sclerosis, Parkinson's disease, Alzheimer's dementia, epilepsy, ischemic stroke, stroke with motor or language impairment, traumatic brain injury, spinal cord injury, and depression. Valuable DTI postprocessing tools for clinical research are also introduced.
Diffusion-tensor imaging (DTI) is a relatively new magnetic resonance imaging (MRI) technique that has mostly been used to evaluate microstructural changes in the brain by measuring the motility of water molecules in tissue. Its imaging capabilities are based on the ability to determine the orientation and diffusion characteristics of white matter.1
Recent advances in the resolution of DTI have made it possible to detect pathology-specific details such as microstructural changes in the axons and myelin of brain white matter.2 The main current focus of DTI research is tracking neural fiber pathways in the central nervous system.3
While DTI has been widely applied to various clinical conditions,4 the semiquantitative nature of DTI data analysis remains a significant limitation.5 The measurement protocol and processing of image data need to be standardized in order to generate more-accurate quantitative results. Advanced techniques of DTI have made it possible to visualize potential changes in various neural tracts associated with brain injury and clinical treatments.678
The aim of this review article is to introduce the basic concepts of DTI and various applications for clinical researchers. We introduce DTI scalars, the biological background of DTI, and current DTI postprocessing tools. Further, we summarize the clinical role of DTI in various disease processes, including amyotrophic lateral sclerosis (ALS), multiple sclerosis (MS), Parkinson's disease (PD), Alzheimer's dementia, epilepsy, ischemic stroke, stroke with motor or language impairment, traumatic brain injury (TBI), spinal cord injury, and depression.
Fractional anisotropy (FA), which is the most widely used scalar in DTI, is calculated by dividing the square root of the sum of squares (SRSS) of the diffusivity differences by the SRSS of the diffusivities.9 The FA represents the amount of diffusional asymmetry in a voxel. FA values of 0 and 1 correspond to infinite isotropy (i.e., the ellipsoid is a sphere) and infinite anisotropy (i.e., the ellipsoid is highly elongated), respectively. The ellipsoid itself has three axes called the eigenvectors, which are usually a long axis (λ1) and two small axes (λ2 and λ3) representing the width and depth, respectively. All three axes are perpendicular to each other and cross at the center point of the ellipsoid. The measured lengths of three axes are the eigenvalues. The diffusivity along the principal axis of the neural tract, which is the longest (λ1), is called the axial diffusivity (AD) or parallel diffusivity. The diffusivity of the mean of the two minor axes (λ2 and λ3) is called the radial diffusivity (RD) or perpendicular diffusivity. The mean of the three orthogonal diffusivities (λ1, λ2, and λ3) is known as the mean diffusivity (MD), while the summarized total diffusivity of the three eigenvalues is called a trace. The mode (MO) is a recently developed tensor index that can be used as a probabilistic measure in crossing-fiber tractography. MO specifies the type of anisotropy as a continuous measure that indicates the differences in the shape of the diffusion tensor, which ranges from planar (a flat cylinder) to linear (a tube) (Fig. 1).101112
While FA is the most commonly used DTI scalar, AD, RD, MD, and MO have recently also been used in clinical research. FA is a marker of axonal integrity that presumes degeneration can change the shape of the diffusion ellipsoid, and this makes it highly sensitive to the microstructural integrity of fibers.1 Although AD increases with brain maturation after birth, it is related to axonal injury and thus decreases in cases of axonal damage. RD is a putative myelin marker that increases with myelin damage to white matter tissue. It can also be influenced by the diameter and density of axons. The MD value is an inverse measure of membrane density and is independent of direction, while being sensitive to cellularity, edema, and necrosis.13 Although FA is highly sensitive for detecting microstructural changes, it is not highly specific to the type of changes. FA can theoretically reduce in association with a reduction in AD, increase in RD, or a combination of these two, and so the use of multiple DTI scalars such as AD, RD, MD, and MO is recommended for better characterizing the microstructure of white matter.14 Decreased apparent diffusion coefficient (ADC) values are known to be related to tumors, immune cell infiltration, cytotoxic edema, and hemorrhage, whereas an increased ADC is related to vasogenic edema.15
DTI Studio (https://www.mristudio.org) is an integrated DTI processing program16 that can run on Microsoft Windows systems. It was developed for DTI computation and white matter fiber tracking. Various formats of MRI scanners can input DTI data directly into DTI Studio. The software can apply various processing techniques such as eddy-current correction, tensor calculation, color mapping, and fiber tracking with rapid fiber assignment by a continuous tracking algorithm. Further, it allows the tracked fibers to be visualized in both two-dimensional and three-dimensional (3-D) modes. Most operations of this software can be performed with few simple clicks.
Diffusion MRI image analysis can be performed using diffusion-spectrum MRI (DSI) Studio (http://dsi-studio.labsolver. org) software.17 The main functions of this software include DTI reconstruction, Q-ball imaging, diffusion-spectrum imaging, generalized q-sampling imaging, Q-space diffeomorphic reconstruction, deterministic fiber tracking, and 3-D visualization with a high-level technique. DSI Studio supports a Windows-based interface and can run on Microsoft Windows, macOS, and Linux systems. The main advantage of this software is that it supports statistical analysis of both group and individual connectometry (Fig. 2).
The tract-based spatial statistics (TBSS) toolkit of the FSL (Functional MRI of the Brain Software Library) neuroimaging package allows voxel-based analysis (VBA) of multisubject diffusion data, which is aimed at improving the objectivity, sensitivity, and interpretability of DTI studies.18 The TBSS toolkit can be used to calculate FA, AD, RD, MD, and MO from raw DTI images by fitting a tensor model. FA images of subjects are aligned with the Montreal Neurological Institute (MNI) template using a nonlinear image registration function. The mean FA images are generated from the FA images of subjects and then thinned to create a mean FA skeleton image. The FA images of subjects are projected onto the study-specific mean FA skeleton image, and then a voxel-based permutation statistical test is performed. The TBSS toolkit is more precise than DTI analysis with a voxel-based morphometry approach since it overcomes the problem of spatially aligning the white matter tracts. The TBSS toolkit is the only DTI tool that allows image processing of MO, which is a useful DTI scalar for quantitatively evaluating crossing fibers.19
The Tracts Constrained by Underlying Anatomy (TRACULA) tool of the FreeSurfer software (https://surfer.nmr. mgh.harvard.edu) is a fully automated DTI processing method for reconstructing long pathways of major white matter tracts based on the global probabilistic approach. It uses a prior anatomical information of the pathways from a set of training subjects.20 The TRACULA tool can track 18 major white matter pathways within predefined regions of interest in an individual's anatomical T1-weighted MRI images (i.e., corpus callosum to forceps major, corpus callosum to forceps minor, both corticospinal tracts, inferior longitudinal fasciculus, uncinate fasciculus, anterior thalamic radiation, cingulum cingulate gyrus bundle, cingulum angular bundle, superior longitudinal fasciculus to the parietal bundle, and superior longitudinal fasciculus to the temporal bundle), and extracts the FA, AD, RD, and MD values for these pathways.
MRtrix (http://www.mrtrix.org) is a large set of functional DTI postprocessing tools for performing various analyses and for visualizing white matter fibers.21 The main features of MRtrix include estimating fiber orientation distributions for fiber tractography, anatomically constrained tractography, nonlinear spatial registration of fiber orientation distribution images, fixel (fiber bundle element)-based analysis of the apparent fiber density and fiber cross section, and quantitative structural connectivity analysis. MRtrix can run on Windows, macOS, Linux, and high-performance parallel-processing computing systems. This software efficiently uses Linux pipelines for complex workflows involving the processing of DTI module sets.
A reduction in FA has been reported in diseases associated with structural damage to white matter tracts, such as ALS. FA is therefore a promising DTI biomarker for ALS, as demonstrated by two previous meta-analyses.2223 One meta-analysis of 8 studies involving 143 patients with ALS and 145 healthy control participants demonstrated significant FA reductions in the bilateral frontal white matter, cingulate gyrus, and posterior limb of the bilateral internal capsule, which suggested that ALS is a multisystem disease that encompasses more than motor dysfunction.23 The other meta-analysis revealed that FA was the most useful parameter in the corticospinal tract (CST), but that its diagnostic accuracy as an independent biomarker of ALS was inadequate.22 A recent retrospective study of 253 patients with ALS and 189 control participants from 8 different clinics presented FA maps revealing the most significant alterations in the CSTs, and additional significant changes in white matter tracts in the frontal lobe, brainstem, and hippocampal regions in ALS.24 However, that study also had limitations due to the equipment and DTI protocols varying across the participating centers.
In addition to FA, the AD, RD, and MD parameters have also been used to evaluate ALS in a few previous studies.252627 The change in RD closely matched regions with FA reduction in a study of 24 heterogeneous patients with ALS and well-matched healthy controls.25 However, AD and MD were not sensitive for detecting changes, which might indicate the relative preservation of axonal architecture. This could be attributed to the study involving patients in the early stages of ALS. Conversely, another study found that increases in AD and MD were more widespread than decreases in FA, which were only more evident in the corona radiata and genu of the corpus callosum.26
While the results obtained using FA, AD, RD, and MD in 3-D MRI remain controversial, it has been demonstrated that FA may be a useful tool for researching ALS and other neurodegenerative diseases. Meaningful clinical implementations of DTI will require more studies of patients with ALS in order to overcome the shortcomings of previous studies, such as relatively small samples, low statistical power, lack of control samples, and poorly characterized patient cohorts.
MS lesions are associated with reduced FA values, indicating structural disruption. Although there are some discrepancies between the findings of previous studies, it has been suggested that DTI can be used to detect decreases in FA as a biomarker of acute enhancing lesions, and thus of disease activity. Other DTI parameters such as MD, AD, and RD have also been used to study MS. RD, which is the average value of eigenvalues λ2 and λ3, represents the rate of diffusion of water perpendicular to the axons and is significantly related to demyelination and remyelination.2829 Increased RD values were found to be potentially related to focal T2-weighted lesions and lesional myelin loss. It was also detected in the white matter, where lesions have not been detected using conventional MRI. A relative elevation in AD values was also observed in lesional fibers, consistent with Wallerian degeneration.30 Further, based on the idea that eigenvalues may be more sensitive than FA measurements, acute plaques with greater demyelination can be differentiated from chronic plaques with a mixed histopathology of demyelination and remyelination.
Reduced FA and increased MD are surrogate markers for detecting optic nerve damage in optic neuritis that are more sensitive than conventional MRI.31 Reduced AD values were also suggested to be a predictive factor of clinical outcome.32 This finding was strongly correlated with the physiological functional status indicated by visual evoked potentials. Moreover, changes in DTI parameters in the optic radiation could be a surrogate marker of degenerative changes in the chronic state after optic neuritis.33
The technical difficulties of acquiring qualified images due to the small and mobile optic nerves and partial volume contamination of adjacent structures by fat limit clinical applications of DTI. Nonetheless, recent technical developments in the postprocessing of images has increased the reliability of DTI tractography in evaluating neural fiber connectivity, with a higher sensitivity than conventional MRI for MS.3435 In addition, tractography findings are strongly correlated with the MRI lesion load and clinical disability.36 However, interpretations should be more conservative owing to the likelihood of false-positive findings and the difficulty of obtaining a reliable value for lesions in gray matter and fibers passing through old plaques in white matter.29
DTI has mostly been applied in PD for connectivity analysis to understand the pathomechanisms of various clinical manifestations, including motor symptoms, cognitive dysfunction, and various behavioral symptoms, rather than for diagnosing PD or evaluating its clinical severity. Symptoms related to dysfunction in executive function, attention capacity, and language were correlated with an abnormal diffusivity in a broad area of white matter. However, some symptoms were related to a unique pattern of diffusivity; for example, short-term memory was inversely correlated with MD in the fornix, while long-term memory was inversely correlated with MD in the right anterior corona radiate.37
Some studies have also demonstrated that it is possible to use DTI as a diagnostic tool for differentiating patients with PD or Parkinsonian syndrome from healthy participants, by analyzing changes in white matter fiber connections.38 One study suggested that DTI is useful for evaluating the clinical severity based on an investigation of the correlation between FA in the substantia nigra and clinical motor symptoms.39 A recent meta-analysis of data from 958 patients and 764 controls in 43 studies also suggested that DTI provides promising biomarkers for the clinical manifestations of PD. In addition, a subanalysis using data from nine studies demonstrated that FA was lower in the substantia nigra of patients with PD than in the control subjects, with a notable effect size.38 Another recent study of 36 patients with PD also demonstrated that increased MD values in the putamen, globus pallidus, and thalamus were correlated with higher grades on the Hoehn and Yahr scale and the Schwab and England scale.40
The use of advanced MRI techniques to research how changes in the white matter microstructure may be directly related to the pathophysiology of Alzheimer's dementia has resulted in DTI being used to identify promising Alzheimer's dementia biomarkers, since it has the potential to identify patients with greater white matter pathology. A longitudinal study comparing DTI scalars at 1-year intervals demonstrated significant, widespread reductions in FA and MD with disease progression.41 Recent studies using DTI to investigate alterations of the white matter have suggested that increased cerebrospinal fluid levels of Tau proteins are correlated with microstructural white matter affection prior to the onset of Alzheimer's dementia, representing ongoing axonal damage. Future validation of DTI for detecting biomarkers of Alzheimer's dementia will potentially allow early diagnoses and the differentiation of mild cognitive impairment from early Alzheimer's disease. Moreover, DTI might be useful as a tool for monitoring disease progression.
Patients with refractory temporal-lobe epilepsy exhibit increased diffusivity and reduced FA in a sclerotic hippocampal area, suggesting the presence of structural disorganization.42 There were similar findings in the region with cortical malformation where conventional MRI scans produced normal findings.43 These findings suggest that DTI is promising for studying localized epileptogenic lesions in patients with refractory epilepsy.
Recent studies attempting to understand the pathogenesis of temporal-lobe epilepsy have focused on the integrity of the white matter.4445 A meta-analysis using pooled data from 13 studies demonstrated that FA in the white matter in both hemispheres was lower in patients with temporal-lobe epilepsy who had unilateral hippocampal sclerosis than in healthy participants. Increased MD values were also seen on both sides, but these changes were greater in the white matter connected to the affected temporal lobe. These findings demonstrate that the structural integrity was disturbed in the ipsilateral hemisphere, with the degree of severity varying according to the distance from the epileptogenic foci.46 Although further studies should be performed to validate the role of DTI in evaluating epilepsy, there is general agreement amongst researchers that it is useful for identifying epileptogenic foci.
increased in the acute phase and then decreased markedly in the chronic phase owing to structural disorganization of the ischemic white matter. Although the ADC remained elevated in the chronic phase, FA continued to decrease for up to 6 months after stroke.47 Analyzing both the ADC and FA could therefore be useful for assessing stroke severity and long-term outcome. Although FA was decreased in regions with leukoaraiosis, with increased T2-weighted signals in conventional MRI, the magnitude of the MD increase was significantly smaller than that in the infarct region.48 These findings indicate that DTI can reliably differentiate clinically significant infarction from ischemic leukoaraiosis.
The CST is a major neural tract in the human brain that is crucial for the motor function of distal extremities,49 and so preservation or recovery of the CST is essential to a favorable recovery of impaired motor function in stroke patients. DTI can be used to evaluate the anatomical orientation and integrity of the CST in stroke (Fig. 3).5051 Two recent meta-analyses found a strong correlation between FA values and upperlimb motor recovery in both ischemic and hemorrhagic stroke.5253 Reductions in FA values were inversely correlated with improvements in neurological outcome and motor functions.54 The FA value in the cerebral peduncle can be used to predict the need for orthosis in patients with hemiplegic stroke. When the estimated FA value on the affected side was >0.59, the estimated probability of ambulation without orthosis was approximately 80%.55 Moreover, DTI has been successfully used to evaluate the efficacy of rehabilitation programs such as constraint-induced movement therapy.56 Volumetric data analysis revealed that the number and length of CST fibers increased significantly after 8 weeks of hand training. The cortical thickness of the ventral postcentral gyrus also increased significantly, demonstrating plasticity induced by training with robotic devices.57
The arcuate fasciculus (AF) connects Broca's and Wernicke's areas and is an important neural tract for language function.5859 The FA, ADC, and number of fibers evaluated using DTI were useful for diagnosing aphasia lesions that could not be easily detected in conventional brain MRI.60 Serial DTI scanning allows changes in the injured tract to be estimated, such as regeneration, degeneration, or resolution of peri-AF edema.61 The fiber density of the right AF was increased after intensive and lengthy melodic intonation therapy in chronic stroke patients with Broca's aphasia.62 Further, the evaluation of AF using DTI can help to predict aphasia recovery at the early stage of stroke. The loss of leftward asymmetry in the number of fibers in the AF or difficulty in reconstructing the left AF were correlated with poor outcome.6364 Therefore, the integrity of the AF is important for the language outcome in aphasia, and behavioral changes after language therapy and resultant plastic changes in the white matter tracts can be measured using DTI (Fig. 4).65
In mild TBI, microscopic traumatic axonal injury is not always detectable in conventional brain imaging.66 Unrecognized damage to the white matter is likely to increase the risks of long-term cognitive and functional impairments, and so DTI is not only useful for detecting hidden lesions but also for understanding the pathophysiology of mild TBI.6768 Moreover, DTI is one of the best methods for detecting lesions in areas that are commonly damaged in TBI, such as the anterior corona radiata, uncinate fasciculus, superior longitudinal fasciculus, and corpus callosum.6970 Abnormal structural connectivity both within and between hemispheres involving the prefrontal cortex is an important pathophysiology of mild TBI.68 Reduced FA in these areas has been found to be strongly correlated with deficits in executive, attentional, and memory functions.71
The weak association between MRI results and clinical findings indicated that conventional MRI has a low sensitivity for detecting diffusion abnormalities in the white matter of the spinal cord.72 Although applying DTI in clinical practice remains challenging, it may be useful for defining abnormal white matter fibers that are undetected in routine MRI.73 DTI with fiber tractography can reveal the integrity of the spinal cord and provide information about pathophysiology. Fibers in the spinal cord are known to be displaced in slow-growing processes such as tumors, whereas more-acute changes such as trauma disrupt fiber integrity.74 FA measurements are also useful for predicting patient outcomes (better outcome for FA values of >0.6 in the acute period) in spinal cord lesion.75 Thus, it may soon be possible to use DTI to quantitatively analyze axonal integrity for detecting potential biomarkers that predict locomotor function.76
Functional disruption of neural circuits involved in affective and cognitive processing contributes substantially to the pathophysiology of major depressive disorder (MDD).7778 Numerous DTI studies have found microstructural abnormalities in white matter neural circuits in patients with MDD.79 Changes in the integrity of the white matter in MDD have been analyzed using whole-brain DTI methods, including the TBSS toolkit and VBA.80 In studies that used the TBSS toolkit, patients with MDD exhibited lower FA in white matter tracts including the genu and body of the corpus callosum,8182838485 cingulum (both the cingulate and parahippocampal regions),848586 uncinate fasciculus,85 anterior limb of the internal capsule,87 superior longitudinal fasciculus,848588 inferior longitudinal fasciculus,838589 and anterior thalamic radiation.83 Studies that applied the VBA method suggested that patients with MDD showed reduced FA values in the prefrontal cingulate cortex,8490919293 anterior cingulate cortex,90 temporal lobe,9495 parietal lobe,919396 cerebellum,9697 and thalamus.97
The corpus callosum is a white matter structure that links the bilateral brain hemispheres involved in emotional and cognitive processing, and shows microstructural alterations in MDD.81 The genu of the corpus callosum connects the prefrontal and orbitofrontal cortexes in the bilateral hemispheres, which play a pivotal role in emotion regulation, reward processing, and cognitive tasks.98 The cingulum and uncinate fasciculus, which are main pathways connecting the ventromedial prefrontal cortex to the medial temporal structures (e.g., amygdala and hippocampus), or posterior parietal or temporal regions, also play critical roles in reward circuitry and the corticolimbic circuit of emotion regulation.99100 There is evidence that the microstructural alterations of white matter tracts involved in emotion, reward, and cognitive processing contribute substantially to the pathophysiology of MDD.75 However, there are several inconsistencies or controversies in the results of DTI studies of MDD, which are due to their heterogeneity in size, sociodemographic and clinical characteristics of samples, analysis methods, or DTI imaging parameters.98 These inconsistencies among the previous results highlight the need to perform a meta-analysis of DTI studies of MDD. Five recent meta-analyses of DTI that utilized the TBSS toolkit with or without a VBA data set found that patients with MDD exhibited lower FA values in the genu and body of the corpus callosum, anterior limb of the internal capsule, superior longitudinal fasciculus, uncinate fasciculus, dorsolateral prefrontal cortex, and cerebellum.8098101102103
Several DTI studies have found the clinical characteristics of MDD to be related to disease burden, including the severity or illness duration of MDD, and inversely correlated with the integrity of the white matter as measured using FA. This suggest that DTI measurements could be useful for evaluating biomarkers that define and track the clinical status of MDD.98 The meta-regression analysis of Murphy and Frodl101 demonstrated a significant inverse correlation between the FA of the superior longitudinal fasciculus and the severity of MDD. Another meta-regression analysis, by Jiang et al.,102 also suggested that symptom severity (as measured using the Hamilton Depression Rating Scale) is inversely correlated with the FA value in the genu of the corpus callosum in patients with MDD.
With regard to illness duration, a study that applied linear regression analysis and the TBSS toolkit found that a longer illness duration was inversely correlated with the mean wholebrain FA.84 There are also several lines of evidence that microstructural abnormalities can predict the treatment outcomes when administering antidepressants in MDD. The FA values bilaterally in the hippocampus were lower in patients with treatment-resistant MDD, defined as nonresponsiveness to at least two well-designed trials of antidepressant treatment, than in patients with MDD who responded to 8 weeks of antidepressant treatment.104 A recent study utilizing the TBSS toolkit also found that the FA values in the cingulum and forceps minor were higher in responders to ketamine therapy than in nonresponders.105 Along with recent advances in machine-learning methods, several neuroimaging studies have applied a support-vector machine (SVM) to classifying patients with MDD using DTI data and white matter network patterns. Qin et al.106 applied pattern recognition analysis to white matter brain networks using DTI scans of patients with MDD, and demonstrated that a linear SVM could distinguish patients with MDD from healthy controls with 100% classification accuracy. Another study with a similar methodology also indicated that a linear SVM classifier differentiated patients with depression from healthy controls with 91.7% accuracy, based on changes in anatomical connectivity patterns in the DTI scans of the patients.107 Those authors reported that most-discriminating features were in the frontolimbic network.
DTI is an emerging neuroimaging method for identifying microstructural changes. Various DTI scalars—such as FA, AD, RD, MD, and MO—can be correlated with clinical information in order to reveal abnormalities associated with neurological diseases. Apart from selecting appropriate DTI scalars, sophisticated clinical research also requires suitable DTI postprocessing tools. Advanced robust postprocessing techniques have yielded novel anatomical and structural pathway information about the brain. Improvements in DTI acquisition techniques such as shorter scanning times (to reduce the effects of head motion), high spatial and gradient-direction resolutions, higher signal-to-noise ratios, and standardization of postprocessing methods will guarantee the utilization of DTI in clinical research and even as a diagnostic tool.
Figures and Tables
Fig. 1
DTI scalar images derived from diffusion-tensor images with 20 gradient directions. The FA is a DTI scalar that represents axonal integrity and is strongly related to fiber integrity. The AD is related to axonal damage. The RD is probably a DTI marker of myelin, with an increased RD value suggestive of myelin damage in white matter tissue. The MD is a measure of the average molecular motion. The size and integrity of cells affects the MD, which is known to be related to necrosis, edema, and cellularity. The MO is a probabilistic tractography measure for crossing white matter fibers. AD: axial diffusivity, DTI: diffusion-tensor imaging, FA: fractional anisotropy, MD: mean diffusivity, MO: mode, RD: radial diffusivity.
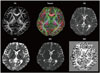
Fig. 2
Frontal-habenula-cerebellar and frontal-cerebellar tracts. The long fiber pathway connecting the frontal cortex via the habenula to the cerebellum (left) and the frontal-cerebellar tracts (right) were tracked using 3-T, 64-direction diffusion-tensor imaging data, analyzed using DSI Studio software.
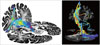
Fig. 3
Diffusion-tensor tractography in a patient (a female aged 74 years) with left hemiparesis after suffering an infarction in the right corona radiata (arrow in lower left figure) and basal ganglia (arrow in upper right figure). The right CST exhibited marked decreases in the number of fibers [n=234 on the right (blue) and n=876 on the left (red)] and in fractional anisotropy (0.4267 and 0.5483, respectively), but the continuity of the right CST was preserved throughout its course. CST: corticospinal tract, L: left, R: right.
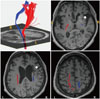
Fig. 4
Three levels of damage to the left AF. DTI of the AF (left column) and T2-weighted magnetic resonance images (right column) show three types of AF (blue, right AF; red, left AF) categorized according to the severity of damage. In type A, fibers of the AF are severely damaged and thus are not visualized in DTI reconstructions. In type B, the AF is disrupted between Wernicke's and Broca's areas. In type C, the AF is preserved around the brain lesion. The arrow indicates disruption of the left AF around the stroke lesion. AF: arcuate fasciculus, DTI: diffusion-tensor imaging.
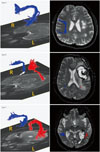
Acknowledgements
This work was supported by the National Research Foundation of Korea (NRF) funded by the Korea government (MSIP) (No. 2016R1A2B400 9206), the National Research Foundation of Korea (NRF) funded by the Korea government (MSIP) (No. 2017R1D1A1B03030280), and the National Research Foundation of Korea (NRF) funded by the Korea government (MSIP) (No. 2017M3C7A1079696).
References
1. Acosta-Cabronero J, Williams GB, Pengas G, Nestor PJ. Absolute diffusivities define the landscape of white matter degeneration in Alzheimer's disease. Brain. 2010; 133:529–539.


2. Zeineh MM, Holdsworth S, Skare S, Atlas SW, Bammer R. Ultra-high resolution diffusion tensor imaging of the microscopic pathways of the medial temporal lobe. Neuroimage. 2012; 62:2065–2082.


3. Abhinav K, Yeh FC, Pathak S, Suski V, Lacomis D, Friedlander RM, et al. Advanced diffusion MRI fiber tracking in neurosurgical and neurodegenerative disorders and neuroanatomical studies: a review. Biochim Biophys Acta. 2014; 1842:2286–2297.


4. Dong Q, Welsh RC, Chenevert TL, Carlos RC, Maly-Sundgren P, Gomez-Hassan DM, et al. Clinical applications of diffusion tensor imaging. J Magn Reson Imaging. 2004; 19:6–18.


5. Cauley KA, Filippi CG. Diffusion-tensor imaging of small nerve bundles: cranial nerves, peripheral nerves, distal spinal cord, and lumbar nerve roots--clinical applications. AJR Am J Roentgenol. 2013; 201:W326–W335.
6. Jiang Q, Zhang ZG, Chopp M. MRI evaluation of white matter recovery after brain injury. Stroke. 2010; 41:S112–S113.


7. Maller JJ, Thomson RH, Lewis PM, Rose SE, Pannek K, Fitzgerald PB. Traumatic brain injury, major depression, and diffusion tensor imaging: making connections. Brain Res Rev. 2010; 64:213–240.


8. Schlaug G, Renga V, Nair D. Transcranial direct current stimulation in stroke recovery. Arch Neurol. 2008; 65:1571–1576.


9. Hagmann P, Jonasson L, Maeder P, Thiran JP, Wedeen VJ, Meuli R. Understanding diffusion MR imaging techniques: from scalar diffusion-weighted imaging to diffusion tensor imaging and beyond. Radiographics. 2006; 26:Suppl 1. S205–S223.


10. Douaud G, Jbabdi S, Behrens TE, Menke RA, Gass A, Monsch AU, et al. DTI measures in crossing-fibre areas: increased diffusion anisotropy reveals early white matter alteration in MCI and mild Alzheimer's disease. Neuroimage. 2011; 55:880–890.


11. Yoncheva YN, Somandepalli K, Reiss PT, Kelly C, Di Martino A, Lazar M, et al. Mode of anisotropy reveals global diffusion alterations in attention-deficit/hyperactivity disorder. J Am Acad Child Adolesc Psychiatry. 2016; 55:137–145.


12. Squarcina L, Bellani M, Rossetti MG, Perlini C, Delvecchio G, Dusi N, et al. Similar white matter changes in schizophrenia and bipolar disorder: a tract-based spatial statistics study. PLoS One. 2017; 12:e0178089.


13. Song SK, Sun SW, Ju WK, Lin SJ, Cross AH, Neufeld AH. Diffusion tensor imaging detects and differentiates axon and myelin degeneration in mouse optic nerve after retinal ischemia. Neuroimage. 2003; 20:1714–1722.


14. Alexander AL, Lee JE, Lazar M, Field AS. Diffusion tensor imaging of the brain. Neurotherapeutics. 2007; 4:316–329.


15. Zhang J, Aggarwal M, Mori S. Structural insights into the rodent CNS via diffusion tensor imaging. Trends Neurosci. 2012; 35:412–421.


16. Jiang H, van Zijl PC, Kim J, Pearlson GD, Mori S. DtiStudio: resource program for diffusion tensor computation and fiber bundle tracking. Comput Methods Programs Biomed. 2006; 81:106–116.


17. Yeh FC, Vettel JM, Singh A, Poczos B, Grafton ST, Erickson KI, et al. Quantifying differences and similarities in whole-brain white matter architecture using local connectome fingerprints. PLoS Comput Biol. 2016; 12:e1005203.


18. Smith SM, Jenkinson M, Johansen-Berg H, Rueckert D, Nichols TE, Mackay CE, et al. Tract-based spatial statistics: voxelwise analysis of multi-subject diffusion data. Neuroimage. 2006; 31:1487–1505.


19. Jbabdi S, Behrens TE, Smith SM. Crossing fibres in tract-based spatial statistics. Neuroimage. 2010; 49:249–256.


20. Yendiki A, Panneck P, Srinivasan P, Stevens A, Zöllei L, Augustinack J, et al. Automated probabilistic reconstruction of white-matter pathways in health and disease using an atlas of the underlying anatomy. Front Neuroinform. 2011; 5:23.


21. Tournier JD, Calamante F, Gadian DG, Connelly A. Direct estimation of the fiber orientation density function from diffusion-weighted MRI data using spherical deconvolution. Neuroimage. 2004; 23:1176–1185.


22. Foerster BR, Dwamena BA, Petrou M, Carlos RC, Callaghan BC, Churchill CL, et al. Diagnostic accuracy of diffusion tensor imaging in amyotrophic lateral sclerosis: a systematic review and individual patient data meta-analysis. Acad Radiol. 2013; 20:1099–1106.


23. Li J, Pan P, Song W, Huang R, Chen K, Shang H. A meta-analysis of diffusion tensor imaging studies in amyotrophic lateral sclerosis. Neurobiol Aging. 2012; 33:1833–1838.


24. Müller HP, Turner MR, Grosskreutz J, Abrahams S, Bede P, Govind V, et al. A large-scale multicentre cerebral diffusion tensor imaging study in amyotrophic lateral sclerosis. J Neurol Neurosurg Psychiatry. 2016; 87:570–579.


25. Filippini N, Douaud G, Mackay CE, Knight S, Talbot K, Turner MR. Corpus callosum involvement is a consistent feature of amyotrophic lateral sclerosis. Neurology. 2010; 75:1645–1652.


26. Metwalli NS, Benatar M, Nair G, Usher S, Hu X, Carew JD. Utility of axial and radial diffusivity from diffusion tensor MRI as markers of neurodegeneration in amyotrophic lateral sclerosis. Brain Res. 2010; 1348:156–164.


27. Sasaki S, Maruyama S. Ultrastructural study of skein-like inclusions in anterior horn neurons of patients with motor neuron disease. Neurosci Lett. 1992; 147:121–124.


28. Song SK, Sun SW, Ramsbottom MJ, Chang C, Russell J, Cross AH. Dysmyelination revealed through MRI as increased radial (but unchanged axial) diffusion of water. Neuroimage. 2002; 17:1429–1436.


29. Rovaris M, Filippi M. Diffusion tensor MRI in multiple sclerosis. J Neuroimaging. 2007; 17:Suppl 1. 27S–30S.


30. Klistorner A, Vootakuru N, Wang C, Yiannikas C, Graham SL, Parratt J, et al. Decoding diffusivity in multiple sclerosis: analysis of optic radiation lesional and non-lesional white matter. PLoS One. 2015; 10:e0122114.


31. Glisson CC, Galetta SL. Nonconventional optic nerve imaging in multiple sclerosis. Neuroimaging Clin N Am. 2009; 19:71–79.


32. van der Walt A, Kolbe SC, Wang YE, Klistorner A, Shuey N, Ahmadi G, et al. Optic nerve diffusion tensor imaging after acute optic neuritis predicts axonal and visual outcomes. PLoS One. 2013; 8:e83825.


33. Kolbe SC, van der Walt A, Butzkueven H, Klistorner A, Egan GF, Kilpatrick TJ. Serial diffusion tensor imaging of the optic radiations after acute optic neuritis. J Ophthalmol. 2016; 2016:2764538.


34. Chen J, Zhou C, Zhu L, Yan X, Wang Y, Chen X, et al. Magnetic resonance diffusion tensor imaging for occult lesion detection in multiple sclerosis. Exp Ther Med. 2017; 13:91–96.


35. Stamile C, Kocevar G, Cotton F, Durand-Dubief F, Hannoun S, Frindel C, et al. A sensitive and automatic white matter fiber tracts model for longitudinal analysis of diffusion tensor images in multiple sclerosis. PLoS One. 2016; 11:e0156405.


36. Pardini M, Yaldizli Ö, Sethi V, Muhlert N, Liu Z, Samson RS, et al. Motor network efficiency and disability in multiple sclerosis. Neurology. 2015; 85:1115–1122.


37. Zheng Z, Shemmassian S, Wijekoon C, Kim W, Bookheimer SY, Pouratian N. DTI correlates of distinct cognitive impairments in Parkinson's disease. Hum Brain Mapp. 2014; 35:1325–1333.


38. Cochrane CJ, Ebmeier KP. Diffusion tensor imaging in parkinsonian syndromes: a systematic review and meta-analysis. Neurology. 2013; 80:857–864.


39. Ofori E, Pasternak O, Planetta PJ, Li H, Burciu RG, Snyder AF, et al. Longitudinal changes in free-water within the substantia nigra of Parkinson's disease. Brain. 2015; 138:2322–2331.


40. Lenfeldt N, Hansson W, Larsson A, Nyberg L, Birgander R, Forsgren L. Diffusion tensor imaging and correlations to Parkinson rating scales. J Neurol. 2013; 260:2823–2830.


41. Mayo CD, Mazerolle EL, Ritchie L, Fisk JD, Gawryluk JR;. Alzheimer's Disease Neuroimaging Initiative. Longitudinal changes in microstructural white matter metrics in Alzheimer's disease. Neuroimage Clin. 2016; 13:330–338.


42. Arfanakis K, Hermann BP, Rogers BP, Carew JD, Seidenberg M, Meyerand ME. Diffusion tensor MRI in temporal lobe epilepsy. Magn Reson Imaging. 2002; 20:511–519.


43. Rugg-Gunn FJ, Eriksson SH, Symms MR, Barker GJ, Duncan JS. Diffusion tensor imaging of cryptogenic and acquired partial epilepsies. Brain. 2001; 124:627–636.


44. Gross DW. Diffusion tensor imaging in temporal lobe epilepsy. Epilepsia. 2011; 52:Suppl 4. 32–34.


45. Kim CH, Chung CK, Koo BB, Lee JM, Kim JS, Lee SK. Changes in language pathways in patients with temporal lobe epilepsy: diffusion tensor imaging analysis of the uncinate and arcuate fasciculi. World Neurosurg. 2011; 75:509–516.


46. Otte WM, van Eijsden P, Sander JW, Duncan JS, Dijkhuizen RM, Braun KP. A meta-analysis of white matter changes in temporal lobe epilepsy as studied with diffusion tensor imaging. Epilepsia. 2012; 53:659–667.


47. Yang Q, Tress BM, Barber PA, Desmond PM, Darby DG, Gerraty RP, et al. Serial study of apparent diffusion coefficient and anisotropy in patients with acute stroke. Stroke. 1999; 30:2382–2390.


48. Jones DK, Lythgoe D, Horsfield MA, Simmons A, Williams SC, Markus HS. Characterization of white matter damage in ischemic leukoaraiosis with diffusion tensor MRI. Stroke. 1999; 30:393–397.


49. Cho SH, Kim DG, Kim DS, Kim YH, Lee CH, Jang SH. Motor outcome according to the integrity of the corticospinal tract determined by diffusion tensor tractography in the early stage of corona radiata infarct. Neurosci Lett. 2007; 426:123–127.


50. Jang SH. The corticospinal tract from the viewpoint of brain rehabilitation. J Rehabil Med. 2014; 46:193–199.


51. Jang SH. A review of diffusion tensor imaging studies on motor recovery mechanisms in stroke patients. NeuroRehabilitation. 2011; 28:345–352.


52. Kumar P, Kathuria P, Nair P, Prasad K. Prediction of upper limb motor recovery after subacute ischemic stroke using diffusion tensor imaging: a systematic review and meta-analysis. J Stroke. 2016; 18:50–59.


53. Kumar P, Yadav AK, Misra S, Kumar A, Chakravarty K, Prasad K. Prediction of upper extremity motor recovery after subacute intracerebral hemorrhage through diffusion tensor imaging: a systematic review and meta-analysis. Neuroradiology. 2016; 58:1043–1050.


54. Eliassen JC, Boespflug EL, Lamy M, Allendorfer J, Chu WJ, Szaflarski JP. Brain-mapping techniques for evaluating poststroke recovery and rehabilitation: a review. Top Stroke Rehabil. 2008; 15:427–450.


55. Maeshima S, Osawa A, Nishio D, Hirano Y, Kigawa H, Takeda H. Diffusion tensor MR imaging of the pyramidal tract can predict the need for orthosis in hemiplegic patients with hemorrhagic stroke. Neurol Sci. 2013; 34:1765–1770.


56. Rickards T, Sterling C, Taub E, Perkins-Hu C, Gauthier L, Graham M, et al. Diffusion tensor imaging study of the response to constraint-induced movement therapy of children with hemiparetic cerebral palsy and adults with chronic stroke. Arch Phys Med Rehabil. 2014; 95:506–514.e1.


57. Lazaridou A, Astrakas L, Mintzopoulos D, Khanicheh A, Singhal AB, Moskowitz MA, et al. Diffusion tensor and volumetric magnetic resonance imaging using an MR-compatible hand-induced robotic device suggests training-induced neuroplasticity in patients with chronic stroke. Int J Mol Med. 2013; 32:995–1000.


58. Dick AS, Tremblay P. Beyond the arcuate fasciculus: consensus and controversy in the connectional anatomy of language. Brain. 2012; 135:3529–3550.


59. Forkel SJ, Thiebaut de, Dell'Acqua F, Kalra L, Murphy DG, Williams SC, et al. Anatomical predictors of aphasia recovery: a tractography study of bilateral perisylvian language networks. Brain. 2014; 137:2027–2039.


60. Kim SH, Lee DG, You H, Son SM, Cho YW, Chang MC, et al. The clinical application of the arcuate fasciculus for stroke patients with aphasia: a diffusion tensor tractography study. NeuroRehabilitation. 2011; 29:305–310.


61. Jang SH. Diffusion tensor imaging studies on arcuate fasciculus in stroke patients: a review. Front Hum Neurosci. 2013; 7:749.


62. Schlaug G, Marchina S, Norton A. Evidence for plasticity in whitematter tracts of patients with chronic Broca’s aphasia undergoing intense intonation-based speech therapy. Ann N Y Acad Sci. 2009; 1169:385–394.


63. Hosomi A, Nagakane Y, Yamada K, Kuriyama N, Mizuno T, Nishimura T, et al. Assessment of arcuate fasciculus with diffusion-tensor tractography may predict the prognosis of aphasia in patients with left middle cerebral artery infarcts. Neuroradiology. 2009; 51:549–555.


64. Kim SH, Jang SH. Prediction of aphasia outcome using diffusion tensor tractography for arcuate fasciculus in stroke. AJNR Am J Neuroradiol. 2013; 34:785–790.


65. Geva S, Correia M, Warburton EA. Diffusion tensor imaging in the study of language and aphasia. Aphasiology. 2011; 25:543–558.


66. Arfanakis K, Haughton VM, Carew JD, Rogers BP, Dempsey RJ, Meyerand ME. Diffusion tensor MR imaging in diffuse axonal injury. AJNR Am J Neuroradiol. 2002; 23:794–802.
67. Strauss S, Hulkower M, Gulko E, Zampolin RL, Gutman D, Chitkara M, et al. Current clinical applications and future potential of diffusion tensor imaging in traumatic brain injury. Top Magn Reson Imaging. 2015; 24:353–362.


68. Aoki Y, Inokuchi R. A voxel-based meta-analysis of diffusion tensor imaging in mild traumatic brain injury. Neurosci Biobehav Rev. 2016; 66:119–126.


69. Niogi SN, Mukherjee P. Diffusion tensor imaging of mild traumatic brain injury. J Head Trauma Rehabil. 2010; 25:241–255.


70. Aoki Y, Inokuchi R, Gunshin M, Yahagi N, Suwa H. Diffusion tensor imaging studies of mild traumatic brain injury: a meta-analysis. J Neurol Neurosurg Psychiatry. 2012; 83:870–876.


71. Kraus MF, Susmaras T, Caughlin BP, Walker CJ, Sweeney JA, Little DM. White matter integrity and cognition in chronic traumatic brain injury: a diffusion tensor imaging study. Brain. 2007; 130:2508–2519.


72. Ellingson BM, Ulmer JL, Kurpad SN, Schmit BD. Diffusion tensor MR imaging in chronic spinal cord injury. AJNR Am J Neuroradiol. 2008; 29:1976–1982.


73. Facon D, Ozanne A, Fillard P, Lepeintre JF, Tournoux-Facon C, Ducreux D. MR diffusion tensor imaging and fiber tracking in spinal cord compression. AJNR Am J Neuroradiol. 2005; 26:1587–1594.
74. Vargas MI, Delavelle J, Jlassi H, Rilliet B, Viallon M, Becker CD, et al. Clinical applications of diffusion tensor tractography of the spinal cord. Neuroradiology. 2008; 50:25–29.


75. Ducreux D, Fillard P, Facon D, Ozanne A, Lepeintre JF, Renoux J, et al. Diffusion tensor magnetic resonance imaging and fiber tracking in spinal cord lesions: current and future indications. Neuroimaging Clin N Am. 2007; 17:137–147.


76. Kelley BJ, Harel NY, Kim CY, Papademetris X, Coman D, Wang X, et al. Diffusion tensor imaging as a predictor of locomotor function after experimental spinal cord injury and recovery. J Neurotrauma. 2014; 31:1362–1373.


77. Rive MM, van Rooijen G, Veltman DJ, Phillips ML, Schene AH, Ruhé HG. Neural correlates of dysfunctional emotion regulation in major depressive disorder. A systematic review of neuroimaging studies. Neurosci Biobehav Rev. 2013; 37:2529–2553.


78. Elliott R, Zahn R, Deakin JF, Anderson IM. Affective cognition and its disruption in mood disorders. Neuropsychopharmacology. 2011; 36:153–182.


79. Liao Y, Huang X, Wu Q, Yang C, Kuang W, Du M, et al. Is depression a disconnection syndrome? Meta-analysis of diffusion tensor imaging studies in patients with MDD. J Psychiatry Neurosci. 2013; 38:49–56.


80. Wise T, Radua J, Nortje G, Cleare AJ, Young AH, Arnone D. Voxel-based meta-analytical evidence of structural disconnectivity in major depression and bipolar disorder. Biol Psychiatry. 2016; 79:293–302.


81. Han KM, Choi S, Jung J, Na KS, Yoon HK, Lee MS, et al. Cortical thickness, cortical and subcortical volume, and white matter integrity in patients with their first episode of major depression. J Affect Disord. 2014; 155:42–48.


82. Choi S, Han KM, Won E, Yoon BJ, Lee MS, Ham BJ. Association of brain-derived neurotrophic factor DNA methylation and reduced white matter integrity in the anterior corona radiata in major depression. J Affect Disord. 2015; 172:74–80.


83. Olvet DM, Peruzzo D, Thapa-Chhetry B, Sublette ME, Sullivan GM, Oquendo MA, et al. A diffusion tensor imaging study of suicide attempters. J Psychiatr Res. 2014; 51:60–67.


84. de Diego-Adeliño J, Pires P, Gómez-Ansón B, Serra-Blasco M, Vives-Gilabert Y, Puigdemont D, et al. Microstructural white-matter abnormalities associated with treatment resistance, severity and duration of illness in major depression. Psychol Med. 2014; 44:1171–1182.


85. Murphy ML, Carballedo A, Fagan AJ, Morris D, Fahey C, Meaney J, et al. Neurotrophic tyrosine kinase polymorphism impacts white matter connections in patients with major depressive disorder. Biol Psychiatry. 2012; 72:663–670.


86. Seok JH, Choi S, Lim HK, Lee SH, Kim I, Ham BJ. Effect of the COMT val158met polymorphism on white matter connectivity in patients with major depressive disorder. Neurosci Lett. 2013; 545:35–39.


87. Zhu X, Wang X, Xiao J, Zhong M, Liao J, Yao S. Altered white matter integrity in first-episode, treatment-naive young adults with major depressive disorder: a tract-based spatial statistics study. Brain Res. 2011; 1369:223–229.


88. Zuo N, Fang J, Lv X, Zhou Y, Hong Y, Li T, et al. White matter abnormalities in major depression: a tract-based spatial statistics and rumination study. PLoS One. 2012; 7:e37561.


89. Versace A, Almeida JR, Quevedo K, Thompson WK, Terwilliger RA, Hassel S, et al. Right orbitofrontal corticolimbic and left corticocortical white matter connectivity differentiate bipolar and unipolar depression. Biol Psychiatry. 2010; 68:560–567.


90. Blood AJ, Iosifescu DV, Makris N, Perlis RH, Kennedy DN, Dougherty DD, et al. ; Phenotype Genotype Project on Addiction and Mood Disorders. Microstructural abnormalities in subcortical reward circuitry of subjects with major depressive disorder. PLoS One. 2010; 5:e13945.
91. Ma N, Li L, Shu N, Liu J, Gong G, He Z, et al. White matter abnormalities in first-episode, treatment-naive young adults with major depressive disorder. Am J Psychiatry. 2007; 164:823–826.


92. Srivastava S, Bhatia MS, Bhargava SK, Kumari R, Chandra S. A Diffusion tensor imaging study using a voxel-based analysis, region-of-interest method to analyze white matter abnormalities in first-episode, treatment-naïve major depressive disorder. J Neuropsychiatry Clin Neurosci. 2016; 28:131–137.


93. Wu F, Tang Y, Xu K, Kong L, Sun W, Wang F, et al. Whiter matter abnormalities in medication-naive subjects with a single short-duration episode of major depressive disorder. Psychiatry Res. 2011; 191:80–83.


94. Ouyang X, Tao HJ, Liu HH, Deng QJ, Sun ZH, Xu L, et al. White matter integrity deficit in treatment-naïve adult patients with major depressive disorder. East Asian Arch Psychiatry. 2011; 21:5–9.
95. Steele JD, Bastin ME, Wardlaw JM, Ebmeier KP. Possible structural abnormality of the brainstem in unipolar depressive illness: a tran scranial ultrasound and diffusion tensor magnetic resonance imaging study. J Neurol Neurosurg Psychiatry. 2005; 76:1510–1515.


96. Jia Z, Huang X, Wu Q, Zhang T, Lui S, Zhang J, et al. High-field magnetic resonance imaging of suicidality in patients with major depressive disorder. Am J Psychiatry. 2010; 167:1381–1390.


97. Tha KK, Terae S, Nakagawa S, Inoue T, Kitagawa N, Kako Y, et al. Impaired integrity of the brain parenchyma in non-geriatric patients with major depressive disorder revealed by diffusion tensor imaging. Psychiatry Res. 2013; 212:208–215.


98. Lichenstein SD, Bishop JH, Verstynen TD, Yeh FC. Diffusion capillary phantom vs. human data: outcomes for reconstruction methods depend on evaluation medium. Front Neurosci. 2016; 10:407.


99. Bracht T, Linden D, Keedwell P. A review of white matter microstructure alterations of pathways of the reward circuit in depression. J Affect Disord. 2015; 187:45–53.


100. Steffens DC, Taylor WD, Denny KL, Bergman SR, Wang L. Structural integrity of the uncinate fasciculus and resting state functional connectivity of the ventral prefrontal cortex in late life depression. PLoS One. 2011; 6:e22697.


101. Murphy ML, Frodl T. Meta-analysis of diffusion tensor imaging studies shows altered fractional anisotropy occurring in distinct brain areas in association with depression. Biol Mood Anxiety Disord. 2011; 1:3.


102. Jiang J, Zhao YJ, Hu XY, Du MY, Chen ZQ, Wu M, et al. Microstructural brain abnormalities in medication-free patients with major depressive disorder: a systematic review and meta-analysis of diffusion tensor imaging. J Psychiatry Neurosci. 2017; 42:150–163.


103. Wen MC, Steffens DC, Chen MK, Zainal NH. Diffusion tensor imaging studies in late-life depression: systematic review and meta-analysis. Int J Geriatr Psychiatry. 2014; 29:1173–1184.


104. Zhou Y, Qin LD, Chen J, Qian LJ, Tao J, Fang YR, et al. Brain microstructural abnormalities revealed by diffusion tensor images in patients with treatment-resistant depression compared with major depressive disorder before treatment. Eur J Radiol. 2011; 80:450–454.


105. Vasavada MM, Leaver AM, Espinoza RT, Joshi SH, Njau SN, Woods RP, et al. Structural connectivity and response to ketamine therapy in major depression: a preliminary study. J Affect Disord. 2016; 190:836–841.

