Abstract
Background
Methods
Results
Conclusions
Acknowledgments
References
SUPPLEMENTARY MATERIALS
Supplemental Data Table S1
Supplemental Data Table S4
Supplemental Data Table S5
Supplemental Data Table S6
Supplemental Data Table S8
Supplemental Data Table S9
Supplemental Data Table S10
Supplemental Data Table S11
Fig. 1
Workflow for diagnosing inherited metabolic diseases. The study population represented about 22% of births (120,700/540,200) in Korea during the designated period. Using the integrated screening model, results were interpreted and divided into three groups: Association-Positive Cases (Cases with mutations in genes relevant to metabolites), Positive Cases with Discrepancy (Cases with mutations in genes irrelevant to metabolites), and Presumptive Positive Cases (Cases with only metabolite abnormalities). The numbers in brackets indicate the number of samples.
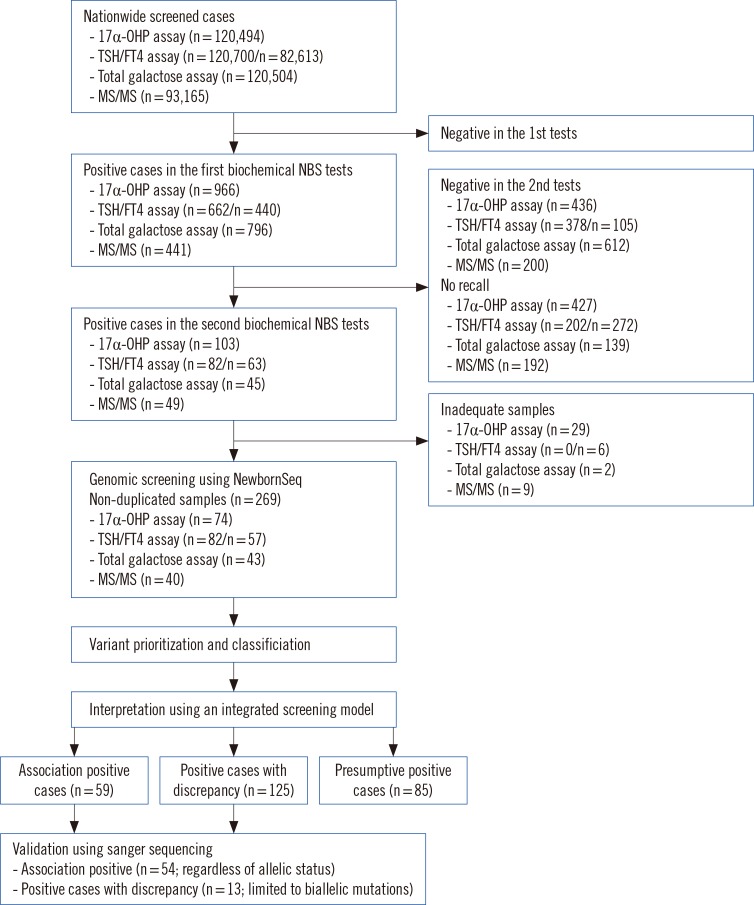
Fig. 2
Putative variant prioritization and pathogenicity classification. Pathogenic variants were prioritized based on conventional methods and the American College of Medical Genetics and Genomics criteria in (A) total samples (n=269), (B) association-positive cases (n=125), and (C) in positive cases with discrepancy (n=85). The numbers in brackets indicate the number of different types of variants.
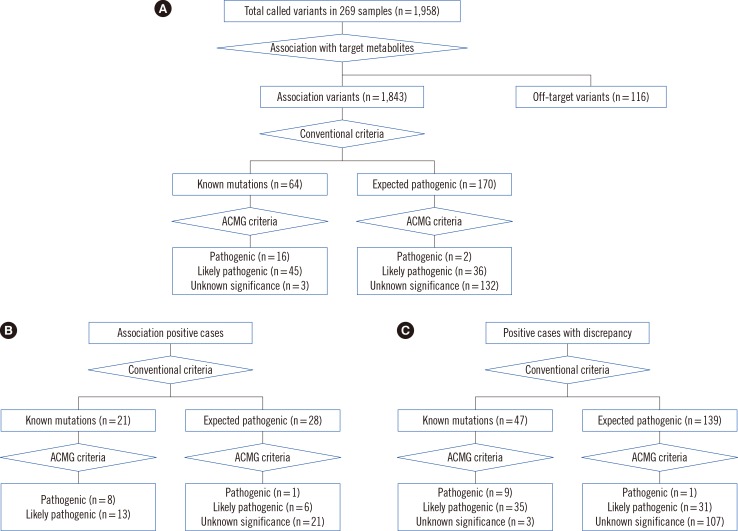
Table 1
Mutation incidence and frequency of inherited metabolic diseases detected using an integrated screening model

Table 2
Diagnosis of inherited metabolic diseases using an integrated screening model
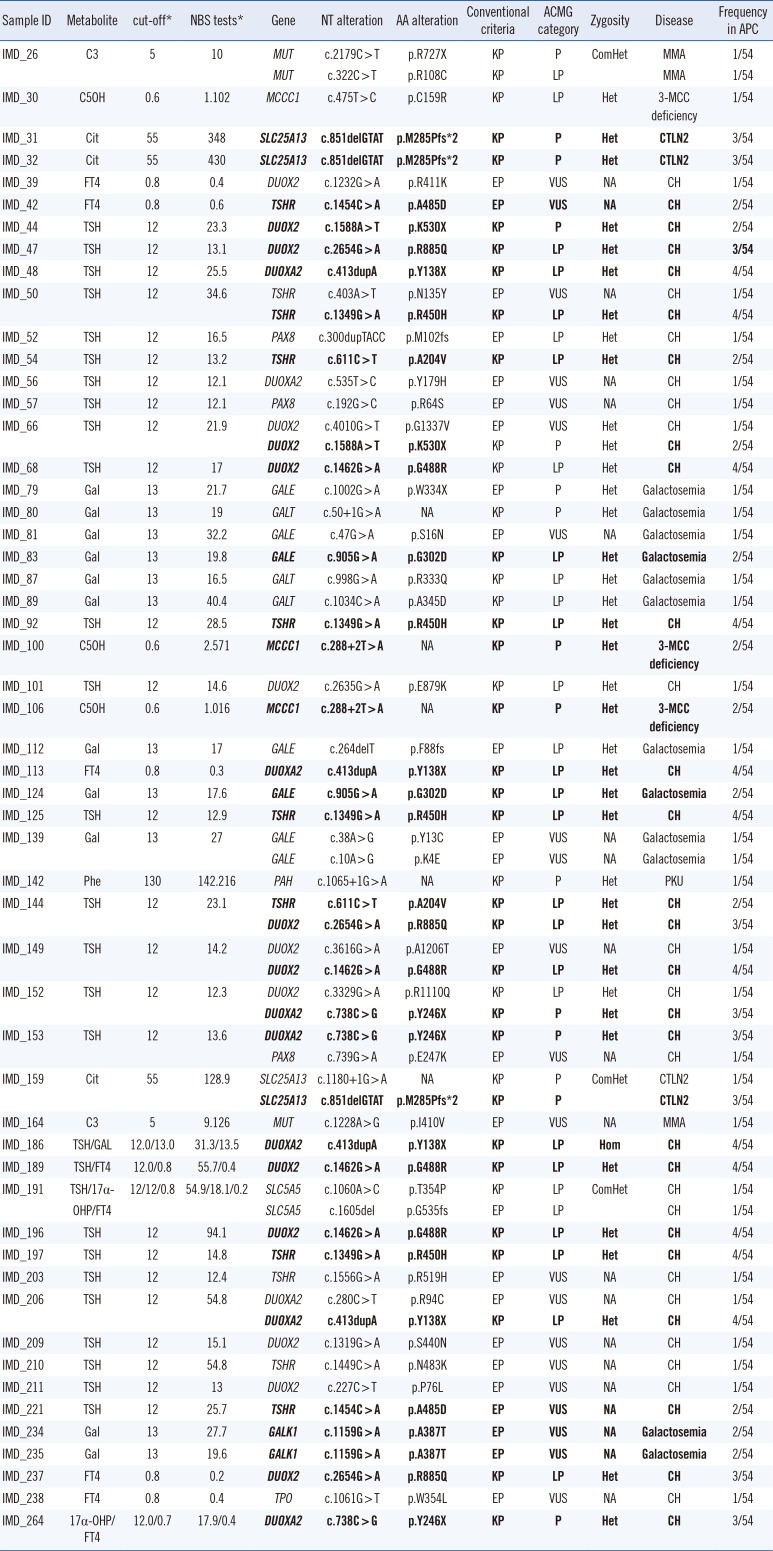
Reference sequences of MUT, MCCC1, SLC25A13, DUOX2, TSHR, DUOXA2, PAX8, GALE, GALT, GALT, PAH, SLC5A5, GALK1, and TPO were NM_000255, NM_001293273, NM_001160210, NM_014080, NM_000369, NM_207581, NM_003466, NM_001127621, NM_001258332, NM_000155, NM_000277, NM_000453, NM_000154, and NM_175722, respectively.
*The metabolite units of C3, C5OH, Phe, Cit, Gal, TSH, FT4 and 17α-OHP were µmol/L, µmol/L, µmol/L, µmol/L, µmol/L, mU/L, ng/dL, ng/mL, respectively. Recurrent mutations are in bold.
Abbreviations: KP, known pathogenic mutation based on the Human Genome Mutation Database (DM) or ClinVar (pathogenic) databases; EP, expected pathogenic mutation based on population frequency, in silico prediction, and mutation type (loss of function mutations); P, pathogenic; LP, likely pathogenic; VUS, variant of unknown significance; NA, not applicable; Het, heterozygous; ComHet, compound heterozygous; Hom, homozygous; Cit, citrulline; GAL, galactose; TSH, thyroid stimulating hormone; FT4, free T4; MMA, Methylmalonic aciduria; 3-MCC deficiency, 3-methylcrotonyl-CoA carboxylase deficiency; PKU, Phenylketonuria; CTLN2, Type II citrullinemia; CH, Congenital hypothyroidism.
Table 3
Unexpected detection of cases with biallelic mutations in genes irrelevant to metabolite abnormalities
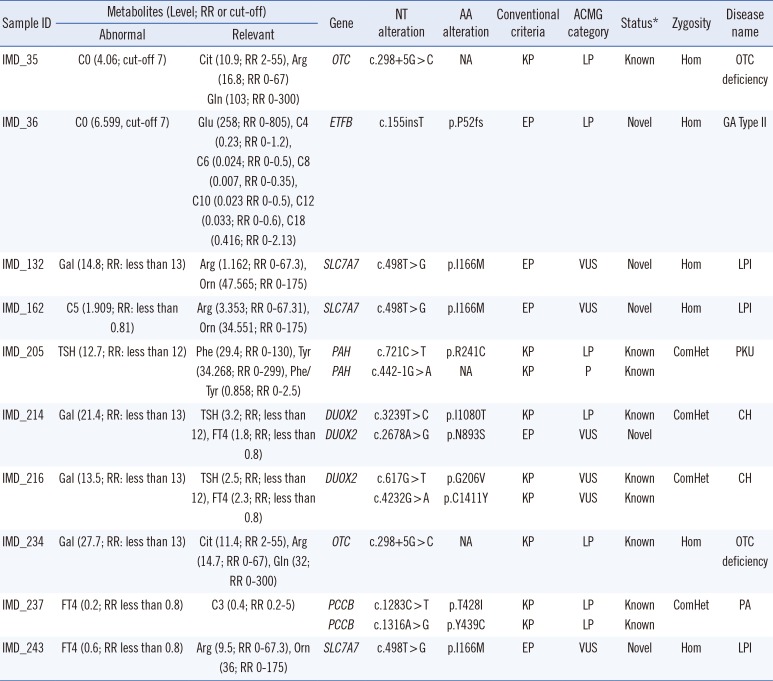
Reference sequences of OTC, ETFB, HAL, SLC7A7, PAH, DUOX2, and PCCB were NM_000531.5, NM_001014763, NM_001258333, NM_001126105, NM_000277, NM_014080, and NM_000532, respectively. The metabolites units of TSH and FT4 were mU/L, ng/dL, ng/mL, respectively. The unit of the other metabolites was µmol/L.
*The mutation status was assessed based on the Human Genome Mutation Database (DM) or ClinVar (pathogenic) databases.
Abbreviations: AA, amino acid; NT, nucleotide; KP, known pathogenic; EP, expected pathogenic based on population frequency, in silico prediction, and mutation type (loss of function mutations); P, pathogenic; LP, likely pathogenic; VUS, variant of unknown significance; RR, reference range; Gal, galactose; TSH, thyroid-stimulating hormone; FT4, free thyroxine; Cit, citrulline; Arg, arginine; Gln, glutamine; Glu, glutamate; Orn, ornithine; Phe, phenylalanine; Tyr, tyrosine; NA, not applicable; Het, heterozygous; ComHet, compound heterozygous; Hom, homozygous; OTC deficiency, Ornithine carbamoyltransferase deficiency; LPI, Lysinuric protein intolerance; CH, Congenital hypothyroidism; PA, Propionic academia, GA type II, Glutaric acidemia type II; PKU, Phenylketonuria.
Table 4
Comparison of mutation-containing haplotypes between cases and controls
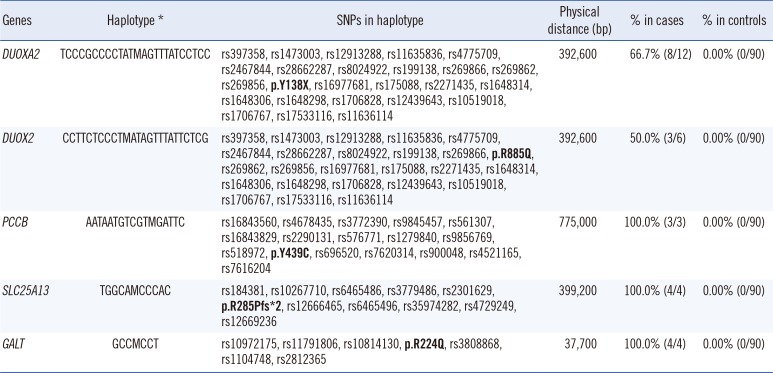
The haplotype frequencies in mutation-positive cases were compared with those in 90 control individuals from the Korean HapMap.
*M represents recurrent mutations (p.Y138X in DUOXA2, p.R885Q in DUOX2, p.Y439C in PCCB, p.R285Pfs*2 in SLC25A13, p.R224Q in GALT).
Abbreviation: SNP, single nucleotide polymorphism.