Abstract
Background
Effective treatment and monitoring of tuberculosis (TB) requires biomarkers that can be easily evaluated in blood samples. The aim of this study was to analyze the serum proteome of patients with TB and to identify protein biomarkers for TB.
Methods
Serum samples from 26 TB patients and 31 controls were analyzed by using nano-flow ultra-performance liquid chromatography coupled to quadrupole time-of-flight mass spectrometry in data-independent mode, and protein and peptide amounts were calculated by using a label-free quantitative approach. The generated data were analyzed by using principal component analysis and partial least squares discriminant analysis, a multivariate statistical method.
Results
Of more than 500 proteins identified, alpha-1-antitrypsin was the most discriminative, which was 4.4 times higher in TB patients than in controls. Peptides from alpha-1-antitrypsin and antithrombin III increased in TB patients and showed a high variable importance in the projection scores and coefficient in partial least square discriminant analysis.
Tuberculosis (TB) is one of the oldest infectious diseases known. Approximately one-third of the world's population has been infected by Mycobacterium tuberculosis, and 1.7 million people died from TB in 2009. The global incidence rate of TB is falling, but too slowly (http://www.who.int/tb/publications/2011/factsheet_tb_2011.pdf). Moreover, the increase in the numbers of immunocompromised individuals, owing to cancer, transplantation, human immunodeficiency virus infection, or other conditions, has increased the incidence of infection by organisms previously considered nonpathogenic, such as nontuberculous mycobacteria [1].
Acid-fast bacilli cultures and subsequent tests with DNA are used to confirm the existence of pathogenic mycobacteria and are regarded as the gold standard for the laboratory diagnosis of TB [2]. However, the slow growth of the bacteria may delay diagnosis and medical intervention. At least 2 weeks are required to obtain final results; during this interval, some patients may be discharged or treated without identification of the TB infection. Therefore, serum biomarkers that are sensitive and specific can shorten the time to diagnosis. Recently, panel biomarkers, which combine multiple biomarkers to increase diagnostic efficiency, have been investigated, and some have been approved by the US Food and Drug Administration [3]. OVA1 is the first in vitro Diagnostic Multivariate Index (IVDMIA) of protein biomarkers developed from clinical proteomics approach. Like OVA1, the combination of multiple biomarkers can increase the clinical sensitivity and/or specificity of the diseases for which biomarkers has been of limited value [3]. Profiling of serum proteins is the initial step in identifying such biomarkers, followed by integration with genomics and clinical proteomics research [4]. In this study, we used a mass spectrometry-based proteomic analysis to identify candidate biomarkers in the serum of patients with TB.
This study was reviewed and approved by the Institutional Review Board of Seoul National University Bundang Hospital. Informed consent was obtained from the 26 TB patients and 31 controls enrolled in the study. The patients with first diagnosis of TB and before treatment were enrolled. Patients were diagnosed with TB when one or more of the following criteria were met: positivity for acid-fast bacilli culture, chronic granulomatous inflammation with or without central necrosis and good treatment response to TB medication, positivity for TB polymerase chain reaction in tissue or body samples, and chest X-rays compatible with TB and good clinical response to TB medication. Serum samples from TB patients and controls were collected and frozen at -70℃ for subsequent analysis.
The average ages of the TB patients and controls were 50.6±15.9 and 49.0±16.8 yr, respectively. Males comprised 51.7% and 53.6% of the TB patients and controls, respectively. The health status of the controls was variable. One patient visited the hospital for a routine health examination, and the others were diagnosed with gastritis, cancer, back pain, cataract, or diabetes. The controls had no respiratory symptoms or active lung lesions visible in chest X-rays.
Serum samples were thawed at room temperature and filtered using a 0.22-µm filter spin kit. The most abundant six serum proteins (albumin, IgG, IgA, transferrin, haptoglobin, and antitrypsin) were depleted by using an immunoaffinity MARS Human 6 column (Agilent Technologies, Santa Clara, CA, USA). The protein concentration of the flow-through was measured by using the Bradford method. One hundred microliters of the flow-through was mixed with 20 µL of 0.5% RapiGest SF (Waters, Milford, MA, USA) in 50 mM ammonium bicarbonate and heated at 80℃ for 15 min. Ten microliters of 50 mM dithiothreitol was added and heated at 60℃ for 60 min. After cooling at room temperature for 5 min, 10 µL of 150 mM iodoacetamide was added and incubated in the dark for 30 min. Trypsin (Promega, Madison, WI, USA) was added at an enzyme-to-substrate ratio of 1:50. After overnight incubation at 37℃, the same amount of 1M HCl was added and incubated at 37℃ for 30 min. The tryptic peptide solution was desalted on an Oasis HLB cartridge (Waters). The solvent was dried using a SpeedVac concentrator (Thermo Fisher Scientific, Waltham, MA, USA).
The dried concentrate was resolved in water/acetonitrile [95:5 (v/v)] in 0.2% formic acid, and 250 fmol digested enolase from Saccharomyces cerevisiae (P00924) was added as an internal standard for absolute quantification. The final solution was loaded onto a liquid chromatography vial and analyzed with a nanoACQUITY Ultra Performance Liquid Chromatography system connected to a Synapt Mass Spectrometer (MS; Waters). The peptides were eluted with a gradient of 0.1% formic acid in water and 0.1% formic acid in acetonitrile. An ACQUITYBEH C18 column (1.7 µm, 75 µm×150 mm) was used as the analytical column, and a C18 column (5 µm, 180 µm×20 mm) was used as the pre-column. The running time was 120 min. For correction of the m/zvalues, 500 µM of [Glu1]-Fibrinopeptide B human (Sigma-Aldrich, St. Louis, MO, USA) was sprayed at 1 µL per 30 sec. The MS was run in positive ion mode with the data-independent analysis method (MSE). Tandem mass spectrometry (MS/MS) data was simultaneously obtained by a cone voltage ramp.
The raw data files were processed with PLGS 2.4 software (Waters). The Universal Protein Resource (UniProt) reviewed human protein database was used for protein identification. One missed cleavage by trypsin was allowed. The search was performed with carbamidomethylation of cysteine as a fixed modification and acetylation of the N-terminus, deamidation of asparagine, and oxidation of methionine as variable modifications. Mass tolerance was automatically calculated but was set to approximately 10 ppm for precursor ions and 20 ppm for fragment ions.
The false-positive rate allowed was 4%. Each identified protein was quantitated by summing the peak intensities of the most abundant three peptides, as previously described [5]. The amount on the column was calculated by comparing the summed intensities to that of the added enolase. To compare the averaged column amounts of each identified protein, t-tests were performed by using IBM SPSS Statistics 19 software (IBM Corporation, Armonk, NY, USA). P values less than 0.05 were considered statistically significant.
After normalization to the total amount of protein or peptide on the column in each analysis, lists of the identified proteins and peptides and their amounts were exported to SIMCA-P+ 12.0 (Umetrics, Umeå, Sweden). Principal component analysis and partial least square-discriminant analysis (PLS-DA) were used to evaluate the discriminative ability of proteins and peptides in patient and control sera and thereby to identify candidate serum biomarkers of TB. The significance of the identified proteins and peptides was further analyzed with the Ingenuity Pathway Analysis tool (IPA; QIAGEN, Redwood City, CA, USA).
In total, 518 proteins were identified. Of these, 28 proteins were identified in all TB patients and controls. The most abundant five proteins were apolipoprotein A1 (P02647), alpha 2 macroglobulin (P01023), complement C3 (P01024), hemopexin (P02790), and alpha-1-antichymotrypsin (P01011). No proteins were identified exclusively in TB patients or controls. As shown in Table 1, the average amounts of 21 proteins were significantly different between TB patients and controls. Of these, 2 proteins were increased and 19 proteins were decreased in TB patients. The average amounts of alpha-1-antitrypsin (P01009) and complement component C6 flags precursor (P13671) were 4.4 and 1.9 times higher, respectively, in TB patients than in controls. For the 19 proteins significantly decreased in TB patients, the ratios of the average amount in TB patients to that in controls were 0.58-0.89.
When the identified proteins from the serum samples of TB patients and controls were analyzed with PLS-DA, 55.4% of the variation could be discriminated and 49.5% of the variation could be predicted after cross-validation with two components. As shown in the score scatter plot and loading scatter plot from PLS-DA (Fig. 1), the proteins closest to TB patients and controls (discriminative) were zinc alpha 2 glycoprotein (P25311), transthyretin (P02766), prothrombin (P00734), alpha-1-acid glycoprotein (P02763), alpha-1-antitrypsin (P01009), and complement component C6 flags precursor (P13671).
Because fragmentation of tryptic peptides produces multiple types of peptides, such as peptides with missed cleavage, tryptic peptides shorter than expected, and peptides with modifications, the intensities of the different types of peptides from the expected large peptides were summed before statistical analysis. In total, 2,617 peptides were identified, and their amino acid sequences were used as identifiers. The five components from PLS-DA explained 27.8% of the variation of the X variable (peptides) and predicted 80.9% of the variation. The controls and TB patients were well separated on the score scatter plot (Fig. 2). The peptides identified in more than 70% of both controls and TB patients, with a P value less than 0.05 and a high rank of variable importance in the projection (VIP) score, are listed in Table 2. Of 18 peptides selected based on these criteria, 2 peptides were higher and 16 peptides were lower in TB patients than in controls. The two peptides that increased in TB patients were from alpha-1-antitrypsin (P01009) and antithrombin III (P01008). The 16 peptides that decreased in TB patients were from alpha-1-microglobulin (P02760), complement C3 (P01024), plasminogen (P00747), zinc alpha 2 glycoprotein (P25311), gelsolin (P06396), plasma protease C1 inhibitor (P05155), serum amyloid P component (P02743), inter-alpha-trypsin inhibitor heavy chain H4 and H1 (Q14624 and P19827), complement C4 (P0C0L4), and hemopexin (P02790).
The two top functions associated with the serum proteins in TB patients were humoral immune response and inflammatory response (P=4.44E-27). The two top canonical pathways involved were acute phase response signaling (P=2.32E-58) and LXR/RXR activation (P=1.15E-54). These top functions and canonical pathways were also identified, when peptides were analyzed.
The discriminative power of proteins and peptides whose levels differed significantly in TB patients and controls was evaluated by ROC analysis. As shown in Fig. 3, alpha-1-antitrypsin (P01009) performed better than complement component C6 flags precursor (P13671). The areas under the curve (AUC) of the two proteins were 0.947 (0.883-1.000) and 0.681 (0.507-0.856), respectively. When the amounts of the two proteins were summed in ROC analysis, the AUC did not exceed that of alpha-1-antitrypsin. The remaining proteins did not show larger AUC than alpha-1-antitrypsin.
For peptides, VFSNGADLSGVTEEAPLKLSK from alpha-1-antitrypsin performed better than EQLQDMGLVDLFSPEKSK from antithrombin III (Fig. 3). The AUC of the two peptides were 0.885 (0.792-0.924) and 0.811 (0.699-0.924), respectively. Summation of the amounts of the two peptides increased the AUC to 0.914 (0.842-0.987).
In the present study, we analyzed the protein and peptide profiles of sera from patients with TB using a quadrupole time-of-flight (Q-TOF) MS system. Despite progress in the treatment of TB and advances in diagnostic tools such as genetic analysis and the use of liquid media, biomarker development for TB is needed, specifically to provide prognostic information for patient classification and for the prediction of reactivation and cure [6]. Profiling of proteins in sera from TB patients using mass spectrometry was reported in 2006 [7]. In that study, surface-enhanced laser desorption/ionization time-of-flight (SELDI-TOF) MS and support vector machines were used. SELDI-TOF can analyze many samples in a short time, which is attractive and appropriate for a large-scale case-control study. However, the drawbacks of SELDI-TOF (low resolution and accuracy and the need to use other tools for identification of candidate peptides) have made most researchers reluctant to use SELDI-TOF for biomarker discovery. Q-TOF MS, used in this study, has a higher resolution and accuracy, and the MS/MS data enable analysis of both proteins and peptides.
Only a few studies have investigated serum protein changes in TB patients. Most were conducted more than 20 yr ago through targeted analysis of immunoglobulin, acute phase reactants, and other candidate proteins using antibody-based techniques such as immunoelectrophoresis [8, 9] or nephelometry [10]. IgG, IgA, alpha-1-antitrypsin, haptoglobin, alpha 2 macroglobulin, and complement C3 were the major proteins reported to change in TB at initial diagnosis. Although the findings were not consistent, most studies reported that IgG, IgA, and alpha-1-antitrypsin increased. Our findings confirm previous reports. In various statistical analyses of TB patients and controls, alpha-1-antitrypsin was consistently higher in TB patients and diagnostic for TB. On average, the serum concentration of alpha-1-antitrypsin was 4.41 times higher in TB patients than in controls. This degree of elevation was similar to that detected in a previous study, which reported an average increase of 4.47-fold [8]. Alpha-1-antitrypsin and its peptide had high VIP scores and coefficients on multivariate analysis, which contributed to the differentiation between TB patients and controls. ROC analyses of the candidate proteins and peptides also showed the largest AUC for alpha-1-antitrypsin. Alpha-1-antitrypsin is a single chain glycoprotein of 418 amino acids that belongs to the serpin family. It is a major plasma serine protease inhibitor that protects the lower respiratory tract by binding and inhibiting granulocyte elastase, which degrades alveolar walls [11]. Alpha-1-antitrypsin deficiency is associated with the development of emphysema [12], and alpha-1-antitrypsin is used to diagnose [13] and treat the condition [14]. Alpha-1-antitrypsin is also a well-known acute phase protein that is upregulated in various inflammatory conditions and disorders [15, 16]. The increase in plasma alpha-1-antitrypsin in TB may protect against elastase-mediated destruction in the lower respiratory tract.
The increases in other plasma proteins, such as complement C6 or antithrombin III, were not consistent with previous studies. Complement C6 is a member of the membrane attack complex, which plays a key role in the innate and adaptive immune response by forming pores in the plasma membrane of target cells [17]. Alterations in the complement system have been reported previously [18], but studies that analyzed the targeting of C6 in TB treatment are not available. A decrease in antithrombin III in TB has been observed in several studies, and the development of deep vein thrombosis may be associated with hypercoagulability in pulmonary TB [19, 20]. In our study, however, plasma antithrombin III levels were not significantly different between TB patients and controls. Instead, a peptide derived from antithrombin III, with the amino acid sequence EQLQDMGLVDLFSPEKSK, increased 1.4-fold in TB patients and greatly contributed to discriminate the two groups. The change in this specific peptide in plasma suggested that antithrombin III metabolism or degradation products might be different in TB patients.
Of note, humoral immune response was a major pathway associated with proteins, whose levels were significantly different in the sera of TB patients. This finding is unexpected, because the primary immune response to TB infection involves cell-mediated immunity. However, increasing evidence supports the involvement of the humoral immune response in the defense against intracellular organisms, including M. tuberculosis. Even a brief extracellular period may expose intracellular pathogens to antibody-mediated defense mechanisms, which can play an important role in disease control and clearance [21].
In this study, we used PLS-DA to determine the discriminative power of serum proteins and peptides. PLS-DA was more useful for the analysis of peptides than proteins and identified candidate peptides that could differentiate TB patients from controls on the basis of VIP score and coefficient.
The major drawback of this study is the lack of control for pulmonary diseases other than TB. Biomarkers that can differentiate TB from nontuberculous mycobacterial infection and other pulmonary diseases are more practical in a clinical setting. The candidate proteins and peptides identified in this study may also change in pulmonary diseases. Further studies with well-designed patient and control groups are required to validate the usefulness of the identified proteins and peptides as biomarkers for TB.
In summary, sera from patients with TB had higher levels of alpha-1-antitrypsin than sera from controls. This difference may aid in the diagnosis of TB. The combination of multiple proteins and peptides by adopting multivariate data analyses can enhance the discriminatory power for TB patients.
Acknowledgments
This study was supported by grant No. 02-2007-019 from the Seoul National University Bundang Hospital Research Fund.
References
1. Wayne LG, Sramek HA. Agents of newly recognized or infrequently encountered mycobacterial diseases. Clin Microbiol Rev. 1992; 5:1–25.


2. Neonakis IK, Gitti Z, Krambovitis E, Spandidos DA. Molecular diagnostic tools in mycobacteriology. J Microbiol Methods. 2008; 75:1–11.


3. Zhang Z, Chan DW. The road from discovery to clinical diagnostics: lessons learned from the first FDA-cleared in vitro diagnostic multivariate index assay of proteomic biomarkers. Cancer Epidemiol Biomarkers Prev. 2010; 19:2995–2999.
4. Boja ES, Rodriguez H. The path to clinical proteomics research: integration of proteomics, genomics, clinical laboratory and regulatory science. Korean J Lab Med. 2011; 31:61–71.


5. Cheng FY, Blackburn K, Lin YM, Goshe MB, Williamson JD. Absolute protein quantification by LC/MS(E) for global analysis of salicylic acid-induced plant protein secretion responses. J Proteome Res. 2009; 8:82–93.


6. Wallis RS, Pai M, Menzies D, Doherty TM, Walzl G, Perkins MD, et al. Biomarkers and diagnostics for tuberculosis: progress, needs, and translation into practice. Lancet. 2010; 375:1920–1937.


7. Agranoff D, Fernandez-Reyes D, Papadopoulos MC, Rojas SA, Herbster M, Loosemore A, et al. Identification of diagnostic markers for tuberculosis by proteomic fingerprinting of serum. Lancet. 2006; 368:1012–1021.


8. Wong CT, Saha N. Changes in serum proteins (albumin, immunoglobulins and acute phase proteins) in pulmonary tuberculosis during therapy. Tubercle. 1990; 71:193–197.


9. Emmett M, Miller JL, Crowle AJ. Protein abnormalities in adult respiratory distress syndrome, tuberculosis, and cystic fibrosis sera. Proc Soc Exp Biol Med. 1987; 184:74–82.


10. Grange JM, Kardjito T, Setiabudi I. A study of acute-phase reactant proteins in Indonesian patients with pulmonary tuberculosis. Tubercle. 1984; 65:23–39.


11. Schaller J, Gerber S, editors. Human blood plasma proteins:structure and function. Chichester, West Sussex: John Wiley & Sons;2008.
12. Laurell CB, Eriksson S. The electrophoretic α1-globulin pattern of serum in α1-antitrypsin deficiency. COPD. 2013; 10(Suppl 1):3–8.


14. Miravitlles M. Alpha-1-antitrypsin and other proteinase inhibitors. Curr Opin Pharmacol. 2012; 12:309–314.


15. Cylwik B, Chrostek L, Gindzienska-Sieskiewicz E, Sierakowski S, Szmitkowski M. Relationship between serum acute-phase proteins and high disease activity in patients with rheumatoid arthritis. Adv Med Sci. 2010; 55:80–85.


16. Wu W, Juan WC, Liang CR, Yeoh KG, So J, Chung MC. S100A9, GIF and AAT as potential combinatorial biomarkers in gastric cancer diagnosis and prognosis. Proteomics Clin Appl. 2012; 6:152–162.


17. Morgan BP. Regulation of the complement membrane attack pathway. Crit Rev Immunol. 1999; 19:173–198.


18. Sai Baba KS, Moudgil KD, Jain RC, Srivastava LM. Complement activation in pulmonary tuberculosis. Tubercle. 1990; 71:103–107.


19. Turken O, Kunter E, Sezer M, Solmazgul E, Cerrahoglu K, Bozkanat E, et al. Hemostatic changes in active pulmonary tuberculosis. Int J Tuberc Lung Dis. 2002; 6:927–932.
20. Robson SC, White NW, Aronson I, Woollgar R, Goodman H, Jacobs P. Acute-phase response and the hypercoagulable state in pulmonary tuberculosis. Br J Haematol. 1996; 93:943–949.


21. Kozakiewicz L, Phuah J, Flynn J, Chan J. The role of B cells and humoral immunity in Mycobacterium tuberculosis infection. Adv Exp Med Biol. 2013; 783:225–250.


22. Kirwan GM, Johansson E, Kleemann R, Verheij ER, Wheelock AM, Goto S, et al. Building multivariate systems biology models. Anal Chem. 2012; 84:7064–7071.


23. Fan L, Zhang W, Yin M, Zhang T, Wu X, Zhang H, et al. Identification of metabolic biomarkers to diagnose epithelial ovarian cancer using a UPLC/QTOF/MS platform. Acta Oncol. 2012; 51:473–479.


24. Liu Y, Sun X, Di D, Quan J, Zhang J, Yang X. A metabolic profiling analysis of symptomatic gout in human serum and urine using high performance liquid chromatography-diode array detector technique. Clin Chim Acta. 2011; 412:2132–2140.


25. Wang X, Zhang A, Han Y, Wang P, Sun H, Song G, et al. Urine metabolomics analysis for biomarker discovery and detection of jaundice syndrome in patients with liver disease. Mol Cell Proteomics. 2012; 11:370–380.


Fig. 1
PLS-DA of proteins from tuberculosis patients and controls. (A) The tuberculosis patients and controls were separated on the score scatter plot, with some outliers and overlapping scores. Almost all of the proteins clustered around the diagonal line of the plot. Proteins (black) close to the points representing the two groups (red) show the strongest correlation. (B) Proteins such as zinc alpha 2 glycoprotein (P25311), transthyretin (P02766), prothrombin (P00734), alpha-1-acid glycoprotein (P02763), alpha-1-antitrypsin (P01009), and complement component C6 flags precursor (P13671) mainly contributed to the separation of tuberculosis patients and controls.
Abbreviations: PLS-DA, partial least square-discriminant analysis; C, controls; P, tuberculosis patients.

Fig. 2
PLS-DA of peptides from tuberculosis patients and controls. (A) The tuberculosis patients and controls were well separated on the score scatter plot, with some outliers and overlapping scores. (B) Most of the peptides clustered around the diagonal line of the plot. Proteins (black) close to the points representing the two groups (red) show the strongest correlation.
Abbreviations: PLS-DA, partial least square-discriminant analysis; C, controls; P, tuberculosis patients.
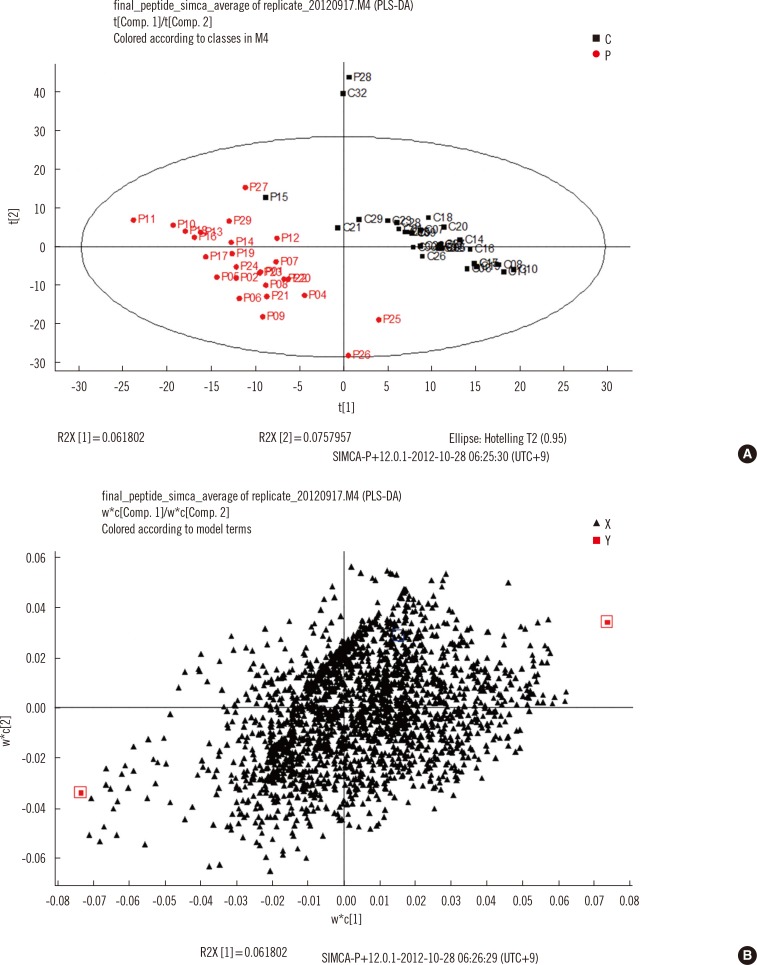
Fig. 3
ROC curves of the proteins (A) and peptides (B) that were significantly higher in tuberculosis patients than in controls. (A) Alpha-1-antitrypsin (P01009) was best able to diagnose tuberculosis patients. The tryptic peptide VFSNGADLSGVTEEAPLKLSK, digested from alpha-1-antitrypsin, performed better than EQLQDMGLVDLFSPEKSK, digested from antithrombin III.
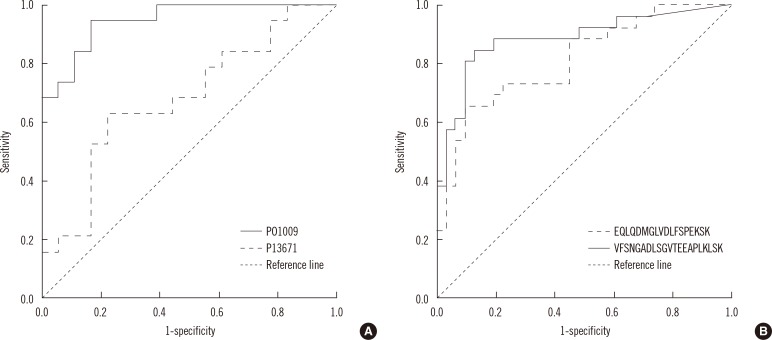
Table 1
Proteins that showed statistically significant differences between tuberculosis patients and controls
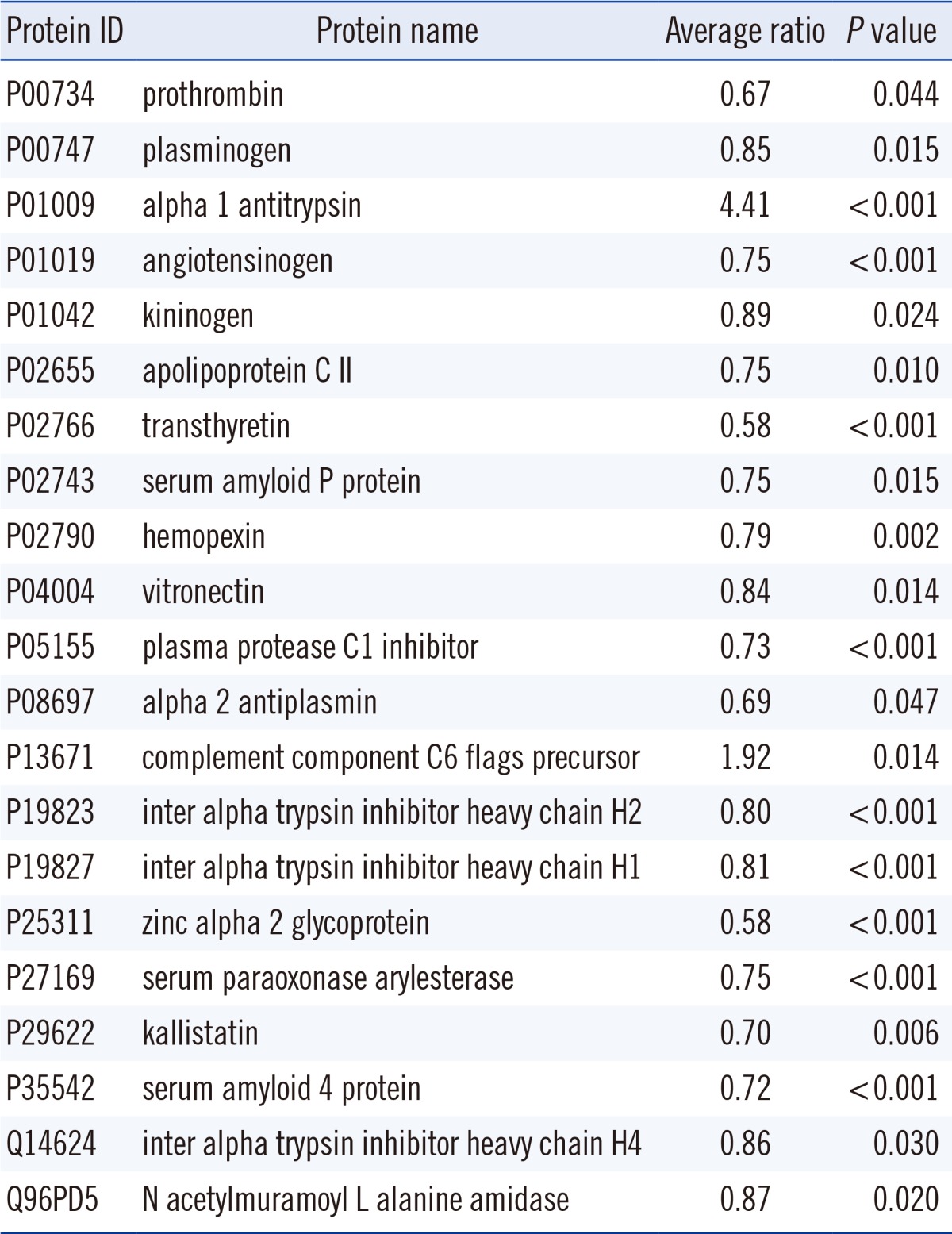