Abstract
Background
Bone mineral density (BMD) is used as a sole parameter in the diagnosis of osteoporosis. Due to the ease of acquirement of BMD, clinical diagnosis still involves its usage although the limitations of BMD are quite well-established. Therefore, this preliminary study hoped to reduce the errors introduced by BMD alone by incorporating geometric and structural predictors simultaneously to observe if strength was implicitly dependent on the geometry and BMD. Hence, we illustrated the triadic relationship between BMD, buckling ratio (BR) and critical fracture load (Fcr).
Methods
The geometric predictor was the BR as it involves both the changes in the periosteum and the cortical thickness. Also, structural changes were monitored by finite element (FE) analysis-predicted Fcr. These BR and Fcr measurements were plotted with their respective femoral neck BMD values in elderly female patients (n=6) in a 3-year follow-up study, treated with ibandronate.
Results
In all the three-dimensional plots (baseline, mid and final year), high Fcr values were found at regions containing high BMD and low BR values. Quantitatively, this was also proven where an averaged highest Fcr across the three years had a relatively higher BMD (46%) and lower BR (19%) than that of the averaged lowest Fcr. The dependence of FE predicted strength on both the geometry and bone density was illustrated.
At present, osteoporosis is one of the leading diseases amongst the elderly, resulting in an increase in morbidity and mortality.[1] This skeletal disease has profound implications on the society in terms of social costs and economic burden. The current diagnosis is based on the obtainment of bone mineral density (BMD) from dual energy X-ray absorptiometry (DXA), where a scans are taken in a single plane and an average BMD is obtained for the site of interest.[2] There are several problems with the diagnosis of osteoporosis and consequently the prediction of hip fractures currently. Firstly, the etiology of fractures is multi-factorial [3] and no one predictor can fully encompass the complex and time-dependent nature of bone loss.[4] Secondly, BMD is an averaged parameter. Thus, it could mask critical regions of bone loss, since there could be localized regions of accelerated bone loss [5] that if gone unnoticed, could have serious implications on the risk of fractures. Lastly, skeletal diseases like osteoporosis have a profound influence on the bone geometry and strength. Excluding these parameters is a serious drawback in terms of diagnosis and progression of these diseases.
Several studies have studied the accuracy with which finite element (FE) models were able to reproduce experimentally measured surface strains.[6,7,8] While they maybe an idealized representation described in mathematical expressions, it offers the best source of information on bone morphology thus far.[9] FE analysis (FEA) derived critical fracture load (Fcr) captures the macroscopic compressive strength of the femur under compression loading. Bone strength is difficult to measure in vivo [10] and is influenced by the amount of bone, the spatial distribution of bone mass and the intrinsic properties of the materials that comprise the bone.[11] Buckling ratio (BR), an originally mechanical engineering concept, introduced by Beck et al.[10] in his earlier works, is the ratio of the outer radius to cortical thickness and is a measure of instability. BR captures the compensation mechanism of the femur by which it remodels by redistributing bone mass to counteract the loss in bone mass in order to preserve bending strength.
The use of BR and Fcr will be useful in the clinical scenario as both predictors capture the geometric and structural changes respectively. So a more accurate analysis of the hip can be provided to doctors, to make a more informed diagnosis. Therefore, there is a need to include other predictors, with or without BMD, to better diagnose osteoporosis and improve the accuracy of hip fracture prediction. This study proposes a novel method of incorporating both Fcr and BR with BMD to explore possible influences of one predictor on the other two. This could lay the foundation for future work on using triadic representations as a tool for predicting osteoporosis and consequently hip fractures.
Quantitative computed tomography (QCT) scans were used to extract the femoral neck BMD values as the focus of this study was on osteoporosis at the femoral neck region. These QCT scans were generated using a General Electric (GE) Medical Systems Scanner (GE Medical Systems, Milwaukee, WI, USA) with settings as follows: 120 kVp, 219 mAs, contiguous, 2.5 mm-thick slices, 0.703 mm pixels, 512×512 matrix and standard reconstruction. Patients treated with ibandronate (n=6) over three years (2008, 2009, and 2010) were analyzed in this study, with their detailed information provided in Table 1. Following methodology adopted by Carpenter et al.,[12] geometric parameter, BR, was obtained from the extraction of femoral neck, followed by drawing of profile rays at 30° intervals and finally obtainment of outer radius and cortical thickness values from the profile rays (Fig. 1).[12] Non-linear FEA was performed as post-failure material behaviour was defined. In our study, displacements are implemented incrementally and the Fcr is represented by the peak total reaction force, which is the sum of all the reaction forces obtained over the 3 cm loaded region on the femoral head.[13] A simple fall in the frontal plane was simulated at 10° to the horizontal and a surface load was applied on the femoral head, as mentioned above. Fcr and BR values obtained were then plotted with respect to BMD in a triadic plot for each year for all patients combined (n=6). This was done using MATLAB® (Mathworks, Natick, MA, USA) for each year.
Three-dimensional (3D) plots were generated for each year (Fig. 2A, C). With BR and BMD plotted in the horizontal axes, Fcr was the dependent variable plotted on the vertical axis. As such, the coloured contour gradient was mapped to the changes in Fcr with respect to BR and BMD. As can be observed, the range of values of Fcr had increased from baseline (Fig. 2A) to final year (Fig. 2C), depicted by the increase in intensity of colours (blue to red).
While some observations are easy to determine, interpretation of meaningful findings are lost in these 3D representations (Fig. 2A, C). Hence, it might be more useful to study the changes in the two-dimensional (2D) surface plot instead (Fig. 2D, F) where both the ranges of predictors and their coupled changes can be observed with relative ease. In Figures 2D to 2F, high Fcr values are observed at the low BR and high BMD regions. In all other regions, low Fcr values were exhibited at regions of high BR and/or low BMD values.
This was also validated quantitatively where an average of the lowest Fcr values obtained was approximately 25,000 N, which was associated with a BMD value of 0.621 and a BR value of 5.17 (Table 2). This was in contrast to an average of the highest Fcr values obtained (86,000 N approximately) which was associated with a BMD value of 0.905 and BR value of 4.18. The averaged highest Fcr value was more than double the averaged lowest Fcr value, while the BMD was 46% higher and BR was 19% lower in the final year than in the baseline year (Table 2).
In this study, FE derived Fcr was strongly associated with both BR and BMD after adjustments for age and body mass index (BMI). Since this preliminary study has only n=6 subjects, it may be small to compare the FE outcomes against BMD in terms of predictive ability. Nevertheless, with the use of material properties and boundary conditions already validated previously, FE derived Fcr is sufficient for this comparative study. The most critical finding in this study was that high Fcr values were associated with high BMD and low BR values. This finding is concomitant with literature as previous studies have established a linear increasing relationship between Fcr and BMD.[14,15] Whereas, a higher BR value means greater instability and thus makes sense that greater strength is found at regions with greater stability (low BR). This goes to show the dependence of strength on both geometry and BMD and that isolating BMD, or any other parameter alone, could mean a critical loss of information.
There are many factors such as loading conditions, weight and age reasonable for changes in geometry. And geometry itself could be analyzed in terms of various parameters such as hip axis length (HAL), neck shaft angle (NSA), femoral neck axis length (FNAL) and femoral neck width (FNW). Identifying these factors and geometrical parameters singularly for a more accurate prediction of fractures is analogous to finding the most significant factor that is majorly responsible for fractures. However, we are well aware that bone remodelling is a dynamic process which is elicited by numerous signals.[16] This patient-specific analysis will pave the way for a more accurate diagnosis of osteoporosis and also for better treatment suggestions. For example, if the patient falls in the critical region as illustrated earlier, then the patient would need to take a higher dosage of drug treatment coupled with strength exercises to strengthen the hip. This leads us to believe that patient-specific analysis could be vital to clinical diagnosis of osteoporosis and fracture prediction.
Therefore, this is the first prospective evaluation of the combination of FEAs with radiology-derived parameters and bone density. Larger studies are required to determine the reproducibility of the triadic relationship and future studies can also focus on the refinement of the FE method to obtain optimized results.
Figures and Tables
Fig. 1
Obtainment of outer radius and cortical thickness from profile rays drawn on narrowest neck slice. After extraction of femoral neck region, profile rays were drawn at 30° intervals, measured from the centroid of the femoral neck slice. A sample profile ray at 0° is shown where respective regions of cortical bone, trabecular bone and soft tissues are identified.
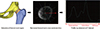
Fig. 2
Yearly three-dimensional surface plots of bone mineral density (BMD), buckling ratio and fracture load (Fcr). High Fcr values were found at regions containing high BMD and low BR values.
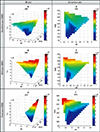
References
1. Melton LJ 3rd. Hip fractures: a worldwide problem today and tomorrow. Bone. 1993; 14:Suppl 1. S1–S8.


2. Kanis JA, Melton LJ 3rd, Christiansen C, et al. The diagnosis of osteoporosis. J Bone Miner Res. 1994; 9:1137–1141.


3. Geusens P, van Geel T, van den Bergh J. Can Hip Fracture Prediction in Women be Estimated beyond Bone Mineral Density Measurement Alone? Ther Adv Musculoskelet Dis. 2010; 2:63–77.


4. Schuit SC, van der Klift M, Weel AE, et al. Fracture incidence and association with bone mineral density in elderly men and women: the Rotterdam Study. Bone. 2004; 34:195–202.


5. Anitha D, Kim KJ, Lim SK, et al. Implications of local osteoporosis on the efficacy of anti-resorptive drug treatment: a 3-year follow-up finite element study in risedronate-treated women. Osteoporos Int. 2013; 24:3043–3051.


6. Lotz JC, Cheal EJ, Hayes WC. Fracture prediction for the proximal femur using finite element models: part I--Linear analysis. J Biomech Eng. 1991; 113:353–360.


7. Bessho M, Ohnishi I, Matsuyama J, et al. Prediction of strength and strain of the proximal femur by a CT-based finite element method. J Biomech. 2007; 40:1745–1753.


8. Schileo E, Taddei F, Malandrino A, et al. Subject-specific finite element models can accurately predict strain levels in long bones. J Biomech. 2007; 40:2982–2989.


9. Cristofolini L, Schileo E, Juszczyk M, et al. Mechanical testing of bones: the positive synergy of finite-element models and in vitro experiments. Philos Trans A Math Phys Eng Sci. 2010; 368:2725–2763.


10. Beck TJ, Oreskovic TL, Stone KL, et al. Structural adaptation to changing skeletal load in the progression toward hip fragility: the study of osteoporotic fractures. J Bone Miner Res. 2001; 16:1108–1119.


11. Bouxsein ML. Determinants of skeletal fragility. Best Pract Res Clin Rheumatol. 2005; 19:897–911.


12. Carpenter RD, Sigurdsson S, Zhao S, et al. Effects of age and sex on the strength and cortical thickness of the femoral neck. Bone. 2011; 48:741–747.


13. Keyak JH. Improved prediction of proximal femoral fracture load using nonlinear finite element models. Med Eng Phys. 2001; 23:165–173.


14. Johnell O, Kanis JA, Oden A, et al. Predictive value of BMD for hip and other fractures. J Bone Miner Res. 2005; 20:1185–1194.


15. Cawthon PM, Ewing SK, Mackey DC, et al. Change in hip bone mineral density and risk of subsequent fractures in older men. J Bone Miner Res. 2012; 27:2179–2188.


16. Parfitt AM. Skeletal heterogeneity and the purpose of bone remodeling: implications for the understanding of osteoporosis. In : Marcus R, Feldman D, Kelsey JL, editors. Osteoporosis. 2nd ed. San Diego, CA: Academic Press;2001. p. 433–447.