Abstract
Purpose
To investigate structural brain changes and their relationship with cognitive function by determining the alterations of white matter integrity and changes in hippocampal volume that occur during chronic obstructive pulmonary disease (COPD).
Materials and Methods
Diffusion tensor images and 3D-T1 MR images were acquired in 13 male nonhypoxemic COPD patients and 13 age- and gender-matched healthy controls. Global probabilistic tractography was used to assess a total of 18 major tracts. We examined the association between the hippocampal volume and diffusivity parameters of white matter tracts.
Results
A significant difference in diffusion parameters between groups was identified for 11 white matter bundles (p < 0.05). No association was demonstrated between the normalized hippocampus volume and diffusion parameters among the participants. Significant associations between Korean version of the Mini-Mental State Examination (K-MMSE) scores and diffusivities were found for six tracts (p < 0.05). Of note, the mean diffusivity (MD) and redial diffusivity (RD) of the left superior longitudinal fasciculus parietal segment showed a significant negative correlation with the K-MMSE score (MD: r = −0.623, p = 0.001; RD: r = −0.408, p = 0.048).
Chronic obstructive pulmonary disease (COPD) is a leading cause of morbidity and mortality, and it is considered a multi-systemic disease that includes brain pathology (12). Hypoxemia resulting from irreversible airflow limitation and neuronal damage caused by systemic inflammation leads to structural brain damage (3). Many studies have investigated structural brain damage or dysfunction in COPD patients. Several studies have suggested regional structural brain changes in COPD patients (45). Dodd et al. (4) reported that white matter integrity is reduced in COPD patients, which is independent of smoking and cerebrovascular comorbidity, but the mechanisms remain unclear.
Magnetic resonance imaging (MRI) has been used for the evaluation of structural brain changes. While providing anatomical information on the brain, conventional MRI techniques have limitations in spatial resolution and contrast that do not allow demonstration of microstructural damage (6). Diffusion tensor imaging (DTI) enables visualization and characterization of white matter bundles through diffusion MRI measurements (78). DTI parameters include fractional anisotropy (FA), which provides a measure of tissue structural integrity, and mean diffusivity (MD), which reflects the tissue microstructure.
TRActs Constrained by UnderLying Anatomy (TRACULA) is an automated probabilistic reconstruction of a set of major white matter pathways from diffusion-weighted MR images. It utilizes prior information on the anatomy of the pathways from a set of training subjects (1) and provides information on the specific tract involved in a disease and the type of damage. The robustness of TRACULA has been validated in prior studies, which have shown meaningful results (19101112131415).
Cognitive impairment is an important extrapulmonary manifestation in COPD patients (1617). However, it has not been fully determined whether structural brain changes are associated with cognitive dysfunction in COPD patients. Some studies using neuroimaging have found that adults with severe COPD may develop alterations in brain perfusion as a result of hypoxemia, and this change has been hypothesized to affect cognitive performance and cause cognitive impairment (18). The hippocampus plays a critical role in cognitive function (19) and it is particularly vulnerable to hypoxemia (20). A pattern of cognitive dysfunction specific to COPD may exist (17). Therefore, the question arises as to whether detectable changes in the volume of the hippocampus occur in COPD patients.
The purpose of this study was to investigate alterations in the microstructural integrity of the white matter and the volume of the hippocampus in stable nonhypoxemic COPD patients compared to those in age- and gender-matched control subjects. We hypothesized that TRACULA would detect altered white matter integrity in COPD patients versus control subjects, and the alteration of white matter integrity would be associated with structural changes in the hippocampus.
This prospective study was approved by our institution's research Ethics Board, and written informed consent was obtained from all patients. Stable nonhypoxemic male COPD patients between 50 to 80 years of age who had not experienced any exacerbations for 8 weeks at the time of data collection were recruited from our outpatient respiratory clinic in 2013. The patients were clinically diagnosed according to the diagnostic criteria of the Global Initiative for Chronic Obstructive Lung Disease [GOLD; post-bronchodilator forced expiratory volume 1/forced vital capacity (FEV1/FVC) < 0.7] and categorized according to the GOLD criteria. Patients were excluded if they had a history of any other pulmonary disease, cerebrovascular disease, brain trauma, psychiatric disorders, or any other neurological or medical conditions that could result in cognitive changes. All patients underwent pulmonary function tests. Sixteen COPD patients were recruited, and three patients withdrew from the study because they decided to discontinue their participation before image acquisition. A total of 13 male, stable, nonhypoxemic patients (age 66.0 years, IQR 63.0–67.0) with COPD were included. Age- and gender-matched control participants were recruited from our respiratory and neurology outpatient clinics. A history of COPD was an exclusionary criterion for control participants, and the other exclusion criteria were the same as in the patient group. Thirteen male participants (age 66.0 years, IQR 58.1–71.0) were enrolled in the control group. The Korean version of the Mini-Mental State Examination (K-MMSE) was used to evaluate general cognitive functions.
DTI and three-dimensional (3D) T1 MRIs were acquired with a 3.0 T Achieva (Philips Medical Systems, Best, the Netherlands) MRI system. A 3D structural MRI was acquired using a T1-weighted turbo field echo sequence [repetition time/echo time (TR/TE) = 9.9 ms/4.6 ms, field of view (FOV) = 240 × 240 × 195 mm3, matrix = 256 × 256, number of excitations (NEX) = 1, slice thickness = 1.0 mm]. A DTI pulse sequence with single shot diffusion-weighted echo planar imaging (TR/TE = 8380 ms/94 ms, FOV = 224 × 224 × 130 mm3, matrix = 112 × 110, NEX = 1, slice thickness = 2.0 mm) was applied in 32 directions (b-value = 1000 s/mm2). At the time of MRI acquisition, two experts performed visual scans to determine whether participants had gross brain abnormalities and to evaluate the adequacy of the data for fiber reconstruction.
Eighteen major fiber bundles were constructed by TRACULA, which is part of the FreeSurfer module (121). TRACULA is a method for automatic reconstruction of a set of major white matter pathways from diffusion-weighted MRIs. It uses global probabilistic tractography with prior anatomical information from a set of 18 manually labeled tracts (1).
The acquired 32-direction DTI data were preprocessed to correct for head motion and distortions induced by eddy current by aligning the diffusion-weighted images to non-diffusion-weighted b = 0 images. The b = 0 images were registered to the same subject's T1-weighted images using a registration method that seeks to maximize the intensity contrast of the b = 0 image across the cortical gray/white boundary (22). Each subject's own T1-weighted image was registered to the 1 mm-resolution MNI-152 atlas (23) by applying affine registration (24). Using FreeSurfer 5.3 (http://surfer.nmr.mgh.harvard.edu), cortical parcellations and subcortical segmentations were generated from each individual's 3D T1 MR images (2526272829).
Using FSL's DTIFIT, which fits a diffusion tensor model at each voxel of the DTI gradient data (http://www.fmrib.ox.ac.uk/fsl), FA, MD, axial diffusivity (AD), and radial diffusivity (RD) were calculated and images showing these measures were generated. FSL's bedpostX applied the ball-and stick model of diffusion to attain the participant's local diffusion orientations (30). TRACULA uses each subject's ball-and-stick model of local diffusion orientations and the labels of the same subject's cortical and subcortical segmentation. The volumetric distributions of the 18 major white matter pathways were reconstructed by combining them with prior information on each tract's position relative to these labels. Then, the tensor-based measures (FA, AD, RD, and MD) for the reconstructed pathways were separately extracted. The 18 reconstructed white matter pathways included the corpus callosum-forceps major (FMAJ) and corpus callosumforceps minor (FMIN) in the corpus callosum, inferior longitudinal fasciculus (ILF), superior longitudinal fasciculus-parietal endings (SLFP), superior longitudinal fasciculus-temporal endings (SLFT), corticospinal tract, uncinate fasciculus (UF), anterior thalamic radiation, cingulum-cingulate gyrus bundle (CCG), and cingulum-angular bundle (CAB). The intracranial volume (ICV), which was measured manually, was used to normalize the hippocampal volume [(sum of both hippocampus volumes divided by the ICV) ×100] (31).
Statistical analyses were performed using SAS ver. 9.4 (SAS Institute Inc., Cary, NC, USA). A non-parametric Mann-Whitney U-test was used to analyze age, K-MMSE scores, ICV, and normalized hippocampal volume. Diffusion parameters (FA, AD, MD, and RD) were extracted for each of the reconstructed pathways. A group difference in the diffusion parameters was found using ranked ANCOVA.
We analyzed the partial correlation between normalized hippocampal volume and DTI parameters of white matter bundles with age and ICV as covariates using the Spearman partial correlation. Correlations between K-MMSE scores and diffusion parameters were also analyzed.
Demographic data are summarized in Table 1. No significant differences were found in age, ICV, and normalized hippocampal volume between the controls and COPD patients. COPD patients did not exhibit significant hypoxemia (SpO2 = 96 ± 1.88%). K-MMSE scores were significantly lower in the COPD group (p = 0.010). The 18 reconstructed tracts used in the data set are shown in Fig. 1.
Significant group differences in DTI parameters were identified using ranked ANCOVA for the FMAJ (FA: p = 0.012, RD: p = 0.013), FMIN (MD: p = 0.047, RD: p = 0.047), left ILF (FA: p = 0.023, AD: p = 0.047), right ILF (FA: p = 0.008, RD: p = 0.018), left SLFP (FA: p = 0.047, MD: p = 0.005, RD: p = 0.035), right SLFP (AD: p = 0.038), left SLFT (MD: p = 0.035), left UF (AD: p = 0.007), right UF (FA: p = 0.023, RD: p = 0.042), left CAB (MD: p = 0.007, AD: p = 0.011, RD: p = 0.035), and right CAB (MD: p = 0.017, RD: p = 0.005), as shown in Table 2.
Table 3 shows the association between diffusion parameters and normalized hippocampus volume and the association between diffusion parameters and K-MMSE scores. No association was demonstrated between the normalized hippocampus volume and DTI parameters among the participants. Significant associations between K-MMSE scores and diffusivities were found for several tracts, including the left SLFP (MD: p = 0.001, RD: p = 0.048), left SLFT (AD: p = 0.006), right SLFT (AD: p = 0.032), and left CCG (AD: p = 0.014). Of note, the MD and RD of the left SLFP showed a significant negative correlation with the K-MMSE score (MD: r = −0.623, p = 0.001; RD: r = −0.408, p = 0.048).
COPD is a leading cause of morbidity and mortality and it has been recognized as a multi-component disease. Cognitive impairment is a significant extrapulmonary manifestation that is associated with increased mortality and disability in COPD patients (1617). A major mechanism proposed for cognitive decline in COPD patients is the neuronal damage mediated through hypoxia (17). However, the mechanism of cognitive dysfunction is unclear (17).
Diffuse white matter changes in COPD patients have been noted in conventional MR images. Recent advances in neuroimaging technology allow for a finer description of structural brain changes. DTI plays a critical role in the study of diseases that affect the brain, which allows prediction of the integrity of white matter tracts (4). TRACULA is an automated probabilistic reconstruction of a set of major white matter pathways from diffusion-weighted MR images. This technique provides information on the specific tract involved in a disease and the type of damage (1). This study is one of the first studies to investigate white matter integrity in COPD patients using TRACULA.
Our study demonstrated an increased number of white matter lesions with altered diffusivity in COPD patients, including the FMAJ, the FMIN, both ILFs, both SLFPs, the left SLFT, both UFs, and both CABs. The K-MMSE scores in COPD patients were significantly lower than those in control participants. These results suggest that alterations of regional white matter integrity are related to cognitive function in COPD patients.
The K-MMSE scores of the participants showed a negative correlation in several white matter tracts, including the left SLFP, both SLFTs, and the left CCG. Of note, the MD and RD of the left SLFP showed a significant negative correlation (MD: r = −0.623, p = 0.001; RD: r = −0.408, p = 0.048) with the K-MMSE score. In addition, COPD patients showed increased MD and RD in the left SLFP relative to healthy controls (MD: p = 0.005, RD: p = 0.035).
Based on the subdivision of the SLF that has been suggested in the literature (32), the SLFP corresponded most closely to the superior longitudinal fasciculus III (SLF III) (1). The SLF III is located in the opercular white matter of the parietal and frontal lobes, extending from the rostral inferior parietal lobule to the ventral part of the premotor and prefrontal cortex. The SLF III links frontal area 44 (the pars opercularis) and the supramarginal gyrus (area 40), which are involved in the articulatory component of language. The connection between the inferior parietal lobule and ventral prefrontal area 46 via this fiber tract has been shown to play a role in working memory (32). The altered diffusivities of the SLFP during COPD may be associated with cognitive changes in COPD patients.
The hippocampus plays a significant role in cognitive function, and a prior study showed that hippocampal atrophy leads to cognitive impairment in COPD patients (5). Structural changes in the hippocampus and altered white matter integrity may cause cognitive dysfunction in COPD patients. Therefore, informative visualization of the relationship between changes in hippocampal volume and white matter integrity is important. In our study, no significant correlation was found between hippocampal volume and diffusion parameters of the white matter tracts, and no volume difference was observed between the control and COPD groups, which is inconsistent with the findings of a previous study (5). Li and Fei (5) proposed that hippocampal atrophy leads to cognitive impairment in COPD, and the degree of atrophy is proportional to arterial oxygen saturation. In this study, we enrolled COPD patients who did not have hypoxemia and had not experienced an acute exacerbation. It is possible that we did not demonstrate the relationship between hippocampal volume changes and COPD in this study. Therefore, further studies with large cohorts should be performed.
The limitations of the study include the small number of patients and the lack of a full assessment of the effect of aging. However, we compared COPD patients with age-matched control individuals. DTI parameters are highly sensitive to motion artifacts, which can be caused by the patient's respiration. To minimize this problem, we recruited only stable nonhypoxemic patients without cardiovascular comorbidities. We also performed visual inspection at the time of MRI acquisition and head motion correction at the time of post-processing.
Interpretation of diffusion parameters is exceedingly complicated, and the specific biological concepts of diffusion metrics are still poorly understood. Although increased MD has been indicated in previous studies as a potential indicator of edema, elevated RD is suggestive of axonal damage or demyelination, and changes in FA describe the degree of directional diffusion organization (33), the manner in which different diffusion metrics are related to one another and define a disease remains to be investigated. Therefore, the precise mechanism by which FA, AD, RD, and MD contribute to characterizing COPD remains to be investigated.
In conclusion, COPD is a systemic disease, and the brain is affected by the disease process. This study demonstrated the alterations of white matter integrity, including those in the SLFP, in COPD patients. This altered white matter integrity may be related to cognitive changes. Hippocampal change, which is important for cognitive function, should be investigated in further studies with larger cohorts.
Figures and Tables
Fig. 1
Eighteen automatically reconstructed white matter tracts from TRACULA [sagittal (A) and axial projective view (B)].
ATR = anterior thalamic radiation, CAB = cingulum-angular bundle, CCG = cingulum-cingulate gyrus bundle, CST = corticospinal tract, FMAJ = corpus callosum-forceps major, FMIN = corpus callosum-forceps minor, ILF = inferior longitudinal fasciculus, SLFP = superior longitudinal fasciculus-parietal endings, SLFT = superior longitudinal fasciculus-temporal endings, TRACULA = TRActs Constrained by UnderLying Anatomy, UF = uncinate fasciculus
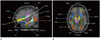
Table 1
Demographic Information, K-MMSE Scores, ICV, and Hippocampal Volume of Controls and COPD Participants
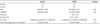
Table 2
Median (IQR) of Diffusion Parameters in Controls and COPD Participants
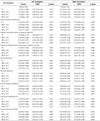
Table 3
Partial Correlation with Normalized Hippocampus Volume and K-MMSE Score in All Participants
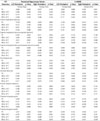
References
1. Yendiki A, Panneck P, Srinivasan P, Stevens A, Zöllei L, Augustinack J, et al. Automated probabilistic reconstruction of white-matter pathways in health and disease using an atlas of the underlying anatomy. Front Neuroinform. 2011; 5:23.
2. Song SK, Sun SW, Ramsbottom MJ, Chang C, Russell J, Cross AH. Dysmyelination revealed through MRI as increased radial (but unchanged axial) diffusion of water. Neuroimage. 2002; 17:1429–1436.
3. Wouters EF, Creutzberg EC, Schols AM. Systemic effects in COPD. Chest. 2002; 121:5 Suppl. 127S–130S.
4. Dodd JW, Chung AW, van den Broek MD, Barrick TR, Charlton RA, Jones PW. Brain structure and function in chronic obstructive pulmonary disease: a multimodal cranial magnetic resonance imaging study. Am J Respir Crit Care Med. 2012; 186:240–245.
5. Li J, Fei GH. The unique alterations of hippocampus and cognitive impairment in chronic obstructive pulmonary disease. Respir Res. 2013; 14:140.
6. Mori S, Zhang J. Principles of diffusion tensor imaging and its applications to basic neuroscience research. Neuron. 2006; 51:527–539.
7. Basser PJ, Mattiello J, LeBihan D. MR diffusion tensor spectroscopy and imaging. Biophys J. 1994; 66:259–267.
8. Basser PJ. Inferring microstructural features and the physiological state of tissues from diffusion-weighted images. NMR Biomed. 1995; 8:333–344.
9. Lee SH, Coutu JP, Wilkens P, Yendiki A, Rosas HD, Salat DH. Alzheimer's disease Neuroimaging Initiative (ADNI). Tract-based analysis of white matter degeneration in Alzheimer's disease. Neuroscience. 2015; 301:79–89.
10. Chong CD, Schwedt TJ. Migraine affects white-matter tract integrity: a diffusion-tensor imaging study. Cephalalgia. 2015; 35:1162–1171.
11. Sarica A, Cerasa A, Vasta R, Perrotta P, Valentino P, Mangone G, et al. Tractography in amyotrophic lateral sclerosis using a novel probabilistic tool: a study with tract-based reconstruction compared to voxel-based approach. J Neurosci Methods. 2014; 224:79–87.
12. Wozniak JR, Mueller BA, Lim KO, Hemmy LS, Day JW. Tractography reveals diffuse white matter abnormalities in myotonic dystrophy type 1. J Neurol Sci. 2014; 341:73–78.
13. Storsve AB, Fjell AM, Yendiki A, Walhovd KB. Longitudinal changes in white matter tract integrity across the adult lifespan and its relation to cortical thinning. PLoS One. 2016; 11:e0156770.
14. Pfuhl G, King JA, Geisler D, Roschinski B, Ritschel F, Seidel M, et al. Preserved white matter microstructure in young patients with anorexia nervosa? Hum Brain Mapp. 2016; 37:4069–4083.
15. Christodoulou JA, Murtagh J, Cyr A, Perrachione TK, Chang P, Halverson K, et al. Relation of white-matter microstructure to reading ability and disability in beginning readers. Neuropsychology. 2016; 03. 07. DOI: 10.1037/neu0000243. [Epub].
16. Incalzi RA, Gemma A, Marra C, Muzzolon R, Capparella O, Carbonin P. Chronic obstructive pulmonary disease. An original model of cognitive decline. Am Rev Respir Dis. 1993; 148:418–424.
17. Dodd JW, Getov SV, Jones PW. Cognitive function in COPD. Eur Respir J. 2010; 35:913–922.
18. Ortapamuk H, Naldoken S. Brain perfusion abnormalities in chronic obstructive pulmonary disease: comparison with cognitive impairment. Ann Nucl Med. 2006; 20:99–106.
19. Eichenbaum H. Hippocampus: cognitive processes and neural representations that underlie declarative memory. Neuron. 2004; 44:109–120.
20. Choi DW, Rothman SM. The role of glutamate neurotoxicity in hypoxic-ischemic neuronal death. Annu Rev Neurosci. 1990; 13:171–182.
21. Yendiki A, Koldewyn K, Kakunoori S, Kanwisher N, Fischl B. Spurious group differences due to head motion in a diffusion MRI study. Neuroimage. 2014; 88:79–90.
22. Greve DN, Fischl B. Accurate and robust brain image alignment using boundary-based registration. Neuroimage. 2009; 48:63–72.
23. Marino-Neto J, Sabbatini RM. A stereotaxic atlas for the telencephalon of the Siamese fighting fish (Betta splendens). Braz J Med Biol Res. 1988; 21:971–986.
24. Jenkinson M, Bannister P, Brady M, Smith S. Improved optimization for the robust and accurate linear registration and motion correction of brain images. Neuroimage. 2002; 17:825–841.
25. Dale AM, Fischl B, Sereno MI. Cortical surface-based analysis. I. Segmentation and surface reconstruction. Neuroimage. 1999; 9:179–194.
26. Fischl B, Sereno MI, Dale AM. Cortical surface-based analysis. II: Inflation, flattening, and a surface-based coordinate system. Neuroimage. 1999; 9:195–207.
27. Fischl B, Salat DH, Busa E, Albert M, Dieterich M, Haselgrove C, et al. Whole brain segmentation: automated labeling of neuroanatomical structures in the human brain. Neuron. 2002; 33:341–355.
28. Fischl B, Dale AM. Measuring the thickness of the human cerebral cortex from magnetic resonance images. Proc Natl Acad Sci U S A. 2000; 97:11050–11055.
29. Fischl B, Salat DH, van der Kouwe AJ, Makris N, Ségonne F, Quinn BT, et al. Sequence-independent segmentation of magnetic resonance images. Neuroimage. 2004; 23:Suppl 1. S69–S84.
30. Behrens TE, Berg HJ, Jbabdi S, Rushworth MF, Woolrich MW. Probabilistic diffusion tractography with multiple fibre orientations: what can we gain? Neuroimage. 2007; 34:144–155.
31. Tae WS, Kim SS, Lee KU, Nam EC, Kim KW. Validation of hippocampal volumes measured using a manual method and two automated methods (FreeSurfer and IBASPM) in chronic major depressive disorder. Neuroradiology. 2008; 50:569–581.
32. Makris N, Kennedy DN, McInerney S, Sorensen AG, Wang R, Caviness VS Jr, et al. Segmentation of subcomponents within the superior longitudinal fascicle in humans: a quantitative, in vivo, DT-MRI study. Cereb Cortex. 2005; 15:854–869.
33. Alexander AL, Lee JE, Lazar M, Field AS. Diffusion tensor imaging of the brain. Neurotherapeutics. 2007; 4:316–329.