Abstract
Glucose variability has been identified as a potential risk factor for diabetic complications; oxidative stress is widely regarded as the mechanism by which glycemic variability induces diabetic complications. However, there remains no generally accepted gold standard for assessing glucose variability. Representative indices for measuring intraday variability include calculation of the standard deviation along with the mean amplitude of glycemic excursions (MAGE). MAGE is used to measure major intraday excursions and is easily measured using continuous glucose monitoring systems. Despite a lack of randomized controlled trials, recent clinical data suggest that long-term glycemic variability, as determined by variability in hemoglobin A1c, may contribute to the development of microvascular complications. Intraday glycemic variability is also suggested to accelerate coronary artery disease in high-risk patients.
Diabetic patients with similar mean glucose levels often exhibit differences in terms of both the number and degree of glucose excursions. This phenomenon, termed glucose variability, has been suggested as a factor associated with diabetic complications. The development of continuous glucose monitoring (CGM) systems has revolutionized the monitoring of short-term glucose variability, and understanding of the role of glucose fluctuations is in progress. Here, we provide an overview of the methods used to measure glucose variability and review the literatures on clinical implications associated with glucose variability.
Table 1 describes the formulas underlying commonly used measurements and their characteristics. There is no generally accepted gold standard and little consensus regarding which method offers the most meaningful assessment of glucose variability. Among the most commonly used methods is the standard deviation (SD). It can be calculated from self-monitoring of blood glucose (SMBG) levels. Since CGM was available, the mean amplitude of glycemic excursions (MAGE) has been widely used, too.
To avoid distortions in variability due to glycemic exposure, calculations of glucose variability should be devoid of a time component: glucose excursion×time=glycemic exposure, but not variability. Similarly, the formula glucose excursion/time=slope is the rate of glucose change, but not its magnitude [1]. Unlike the integrated measurement of long-term glucose control provided by hemoglobin A1c (HbA1c), glycemic variability requires comprehensive assessment of glycemia. CGM systems provide a more accurate measure of glycemia than does SMBG; however, issues of reproducibility limit the utility of this approach [2].
The simplest method of assessing intra-day variability of serum glucose is to calculate the SD or coefficient of variation (CV) of multiple SMBG readings taken over the course of a day. Usually 7-point glucose measures are used, although important fluctuations can be missed simply because they occur between two measurements (Fig. 1). Furthermore, it is difficult to obtain information on nocturnal glycemic patterns. CGM systems are able to overcome many of the issues with SMBG glucose curves, though the data are still not normally distributed, a condition necessary for calculating the SD. However, the SD correlates well with other variability measures and is the only measurement identified to date where a relationship between glucose variability and mortality in intensive care unit can be demonstrated [34]. For these reasons, some groups have suggested the SD as the preferred method for assessing intraday glucose variability [5].
MAGE was originally developed using hourly glucose samples taken from venous blood [6], and it has emerged as the preferred method for assessing CGM data. In the example presented in Fig. 2, 1 SD of the mean glucose level for each 24-hour period acts as an individualized standard; only one limb of the excursion >1 SD, either ascending or descending, is used to calculate subsequent excursions. The arithmetic mean of these glycemic excursions over the period of study (24 hours or longer) is then used to calculate the MAGE; an automated algorithm has been created for this calculation [7]. Using this method, the mean glucose value becomes the reference point for glycemic variability. However, because the MAGE represents only major excursions from the mean and ignores excursions of <1 SD, this approach disregards smaller excursions that may be important.
The M-value was developed to quantify glycemic control of patients with type 1 diabetes mellitus (T1DM) using a reference value of six blood sugar measurements taken over a 24-hour period [8]. This approach measures the stability of glucose excursions relative to a user-defined "ideal" value ranging from 80 to 120 mg/dL. As a result, differences in user-defined "ideal" values have limited the ability to compare results between studies. In addition, M-values rise in response to increasing glycemic variability and poor glycemic control, making it difficult to distinguish between patients with high mean glucose levels and high glucose variability. Because hypoglycemia has a greater impact on the M-value than does hyperglycemia, this method is more relevant as a clinical, rather than a mathematical, indicator of glycemic control. A similar index which takes into account both the mean glucose level and variability of glycemia is the J-index [9].
In 1999, a new method, continuous overall net glycemic action (CONGA)-n, was developed specifically for use with CGM data [10]. This method calculates the difference between current glucose levels and that of glucose levels n hours previously, with n varying from 1 to 8 hours; readouts are then calculated using the SD of these differences. Because CONGA does not require arbitrarily defined glucose cutoffs or changes in glucose levels, this approach provides an objective assessment of glycemic variability over short time intervals. However, glucose differences are not normally distributed, limiting the applicability of this approach. Furthermore, which time segments produce the most useful results has yet to be determined.
Outside of measuring glucose itself, serum 1,5-anhydroglucitol (1,5-AG) levels have been suggested as a means of assessing glycemic excursions [11]. 1,5-AG is a polyol maintained within stable limits in subjects with healthy glucose levels. Its reabsorption in the kidney is inhibited by excessive excretion of urinary glucose; the higher the plasma glucose concentration, the lower the concentration of plasma 1,5-AG [12]. Urinary glucose only appears at plasma glucose concentrations over 160 mg/dL; therefore, 1,5-AG level seems of little use in detecting glucose fluctuations below this range. In addition, because both chronic and intermittent hyperglycemia are characterized by low concentrations of 1,5-AG, the correlation between glucose variability and 1,5-AG is poor when mean glucose levels remain high persistently. Use of the 1,5-AG concentration as a clinical marker is therefore limited to patients with postprandial hyperglycemia with HbA1c levels below 8% [1314]. Even though, 1,5-AG is not able to provide a direct measurement of glucose fluctuations, limiting the ability of this method to measure both the size and frequency of glycemic excursions.
The easiest way to measure interday variability of serum glucose is by calculating the SD of the fasting blood glucose concentration [15]. The absolute mean of daily differences (MODD) using hourly blood sampling was developed as a supplement to MAGE and mean blood glucose testing; this approach calculates the mean absolute value of the differences between glucose values on two consecutive days at the same time [16]. However, the timing of meals can greatly affect MODD scores, making a strict dietary regimen essential for accurate monitoring.
It has been suggested that glucose variability may have been responsible for differences in microvascular outcomes between intensively and conventionally treated T1DM patients with the same mean HbA1c in the Diabetes Control and Complications Trial (DCCT) [17]. Although this hypothesis has since been refuted by the study authors themselves [18], interest in the relationship between glucose variability and chronic complications of diabetes has persisted. This interest has yet to progress to the point of randomized clinical trials, making the connection between these two factors largely speculative.
Hyperglycemia is thought to induce oxidative stress, which has been shown to exacerbate diabetic complications through a variety of molecular mechanisms [19]. The proposed contribution of glucose variability is supported by reports that oxidative stress is greater for intermittent as opposed to sustained hyperglycemia [202122], though contradictory evidence has also been reported [132324].
In the case of T1DM, a large proportion of studies have been secondary analyses of either the DCCT or the follow-up Epidemiology of Diabetes Interventions and Complications (EDIC) study. In these studies, the SD of SMBG results revealed no relationship between glucose variability and the risk of development or progression of microvascular complications (retinopathy and/or nephropathy) [18252627]. Glucose variability did not contribute to the development of neuropathy, although neuropathy data were more limited than other clinical covariates [28]. However, according to a prospective observational study that followed 100 patients with T1DM for 11 years, the SD of SMBG was found to be significantly related only with peripheral neuropathy and a borderline predictor of incidence (hazard ratio, 1.73; P=0.07) [29]. Therefore, these data suggest that the nervous system may be particularly vulnerable to glycemic variability. However, these studies relied on SMBG data, which may miss fluctuations occurring between the measurements.
A similar study assessing glycemic variability in T1DM patients using both CGM and SMBG readings revealed a strong correlation between microvascular complications and higher glycemic variability by CGM, but not by SMBG [30]. However, reproducibility remains an issue, with a conflicting report demonstrating no significant association between retinopathy and measures of glucose variability using CGM data in either T1DM or type 2 diabetes mellitus (T2DM) [31]. More studies are therefore necessary to determine the relationship between intraday glycemic variability and microvascular complications. In the meantime, long-term fluctuations such as HbA1c variability and the CV/SD of fasting blood glucose have been implicated in the development of retinopathy or nephropathy in T1DM [32] and T2DM [3334] by retrospective analyses. However, even these associations remain controversial [35].
In terms of the DCCT study, the SD was not a predictor of cardiovascular events in patients with T1DM [36]. As for T2DM, some evidence of long-term variability in fasting plasma glucose as a prognostic factor for cardiovascular mortality has been seen in elderly patients [37]. Recent 7-point glucose data from the HEART2D study comparing basal insulin and prandial insulin after acute myocardial infarction (Fig. 3) reported no association between the mean absolute glucose (MAG), an intraday assessment of glucose variability, and the endpoint of combined cardiovascular events [38]. Because neither SD nor MAGE was significantly different between the two treatment groups, this interpretation has been challenged in part due to the reliance on MAG, with no other methods used to assess variability [39]. In the meanwhile, in patients with acute myocardial infarction, MAGE from CGM data collected at the time of admission independently predicted major adverse cardiac events [4041]. A subsequent cross-sectional study also showed that short-term glucose variability, as determined by CGM, was associated with the severity of coronary artery disease [42]. When we look at studies of surrogate endpoints such as subclinical atherosclerosis, more supportive evidence for associations with glucose variability can be found both in T1DM and T2DM [43444546]. However, a substantial proportion of the T2DM studies included mixed populations of patients treated with diet alone, diet and oral antidiabetic medications, and insulin. As such, this may have been a confounding factor in many of the findings, requiring further subgroup analyses to more accurately assess risk factors in this population.
While the data remain very heterogeneous, some conclusions can be drawn from this literature review. First, in the absence of a true gold standard for determining glucose variability, MAGE and SD have become the go-to methods for variability testing. CGM systems appear to be preferable to SMBG for capturing variability because of the larger number of data points possible with this approach. Second, little supporting evidence linking short-term glucose variability and microvascular complications exists, with full confirmation requiring use of a CGM system, particularly in patients with T1DM. The bulk of the T1DM data were derived from a single study (DCCT), limiting the generalizability of some conclusions; further studies are necessary to determine whether these findings are consistent across different populations. Prospective analyses examining the effects of long-term variability on microvascular complications are also needed. As for macrovascular complications, although the HEART2D trial suggested that lowering glucose variability does not improve cardiovascular outcomes in T2DM patients after acute myocardial infarction, subsequent studies demonstrated that glycemic variability has a probability of accelerating coronary artery disease in high-risk patients with T2DM. Large-scale randomized controlled trials are necessary to establish such a risk.
Figures and Tables
![]() | Fig. 1Twenty-four-hour glycemic curves of two patients with diabetes (red and blue lines). The two patients exhibit different patterns of glycemic variation; however, standard deviations calculated across all four points, before each meal and at bedtime (arrows), do not reflect this because the glucose measures are similar between the two patients at those points. |
![]() | Fig. 2Continuous glucose monitoring in a patient with type 1 diabetes mellitus. Qualifying excursions are shown as blue arrows (only the inflection components in this case). Each inflection incorporates several excursions smaller than 1 standard deviation (SD) within a given day (44 mg/dL for day 1 and 65 mg/dL for day 2). The averaged excursion (that is, mean amplitude of glycemic excursion [MAGE]) is (A) 85.0 mg/dL for day 1 and (B) 156.5 mg/dL for day 2. MAGE calculated from the entire 48-hour time course (SD, 56.5 mg/dL) was 131.5 mg/dL; this level was similar across each day of the study period (120.7 mg/dL). Similar MAGE values could also be calculated from the descending limbs. |
![]() | Fig. 3Glycemic measures in a randomized controlled trial comparing prandial and basal insulin in patients with cardiovascular disease (HEART2D study). Seven-point mean self-monitoring of blood glucose profiles at baseline (dotted line) and throughout the study (solid line) are indicative of the treatment strategy. Only the change in the mean absolute glucose level, an alleged measure of glucose variability, was significantly different between treatments, with no observable differences in standard deviation or mean amplitude of glycemic excursion. Therefore, accurate interpretation of the relationship between glycemic variability and the endpoint of combined cardiovascular events in this trial is prudent. Adapted from Raz et al. [47], with permission from American Diabetes Association. aP<0.05 between treatment. |
Table 1
Formulas Used to Describe Glucose Variability
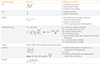
Units are in mmol/L or mg/dL depending on the unity of the glucose values measured. To convert glucose values from mg/dL to mmol/L multiply by 0.0555. Adapted from Siegelaar et al. [5], with permission from Endocrine Society.
SD, standard deviation; CV, coefficient of variation; MAGE, mean amplitude of glycemic excursion; MBG, mean blood glucose; CONGA, continuous overall net glycemic action; MODD, mean of daily difference.
ACKNOWLEDGMENTS
This study was supported by a grant from the Innovative Research Institute for Cell Therapy (A062260) by the Ministry of Health and Welfare, Republic of Korea.
References
1. Service FJ. Glucose variability. Diabetes. 2013; 62:1398–1404.
2. Facchinetti A, Sparacino G, Cobelli C. Modeling the error of continuous glucose monitoring sensor data: critical aspects discussed through simulation studies. J Diabetes Sci Technol. 2010; 4:4–14.
3. Krinsley JS. Glycemic variability: a strong independent predictor of mortality in critically ill patients. Crit Care Med. 2008; 36:3008–3013.
4. Egi M, Bellomo R, Stachowski E, French CJ, Hart G. Variability of blood glucose concentration and short-term mortality in critically ill patients. Anesthesiology. 2006; 105:244–252.
5. Siegelaar SE, Holleman F, Hoekstra JB, DeVries JH. Glucose variability; does it matter? Endocr Rev. 2010; 31:171–182.
6. Service FJ, Molnar GD, Rosevear JW, Ackerman E, Gatewood LC, Taylor WF. Mean amplitude of glycemic excursions, a measure of diabetic instability. Diabetes. 1970; 19:644–655.
7. Baghurst PA. Calculating the mean amplitude of glycemic excursion from continuous glucose monitoring data: an automated algorithm. Diabetes Technol Ther. 2011; 13:296–302.
8. Schlichtkrull J, Munck O, Jersild M. The M-valve, an index of blood-sugar control in diabetics. Acta Med Scand. 1965; 177:95–102.
9. Moberg E, Kollind M, Lins PE, Adamson U. Estimation of blood-glucose variability in patients with insulin-dependent diabetes mellitus. Scand J Clin Lab Invest. 1993; 53:507–514.
10. McDonnell CM, Donath SM, Vidmar SI, Werther GA, Cameron FJ. A novel approach to continuous glucose analysis utilizing glycemic variation. Diabetes Technol Ther. 2005; 7:253–263.
11. Kishimoto M, Yamasaki Y, Kubota M, Arai K, Morishima T, Kawamori R, Kamada T. 1,5-Anhydro-D-glucitol evaluates daily glycemic excursions in well-controlled NIDDM. Diabetes Care. 1995; 18:1156–1159.
12. Buse JB, Freeman JL, Edelman SV, Jovanovic L, McGill JB. Serum 1,5-anhydroglucitol (GlycoMark): a short-term glycemic marker. Diabetes Technol Ther. 2003; 5:355–363.
13. Kim MJ, Jung HS, Hwang-Bo Y, Cho SW, Jang HC, Kim SY, Park KS. Evaluation of 1,5-anhydroglucitol as a marker for glycemic variability in patients with type 2 diabetes mellitus. Acta Diabetol. 2013; 50:505–510.
14. Dungan KM, Buse JB, Largay J, Kelly MM, Button EA, Kato S, Wittlin S. 1,5-anhydroglucitol and postprandial hyperglycemia as measured by continuous glucose monitoring system in moderately controlled patients with diabetes. Diabetes Care. 2006; 29:1214–1219.
15. Shima K, Tanaka R, Morishita S, Tarui S, Kumahara Y. Studies on the etiology of "brittle diabetes". Relationship between diabetic instability and insulinogenic reserve. Diabetes. 1977; 26:717–725.
16. Molnar GD, Taylor WF, Ho MM. Day-to-day variation of continuously monitored glycaemia: a further measure of diabetic instability. Diabetologia. 1972; 8:342–348.
17. Brownlee M, Hirsch IB. Glycemic variability: a hemoglobin A1c-independent risk factor for diabetic complications. JAMA. 2006; 295:1707–1708.
18. Lachin JM, Genuth S, Nathan DM, Zinman B, Rutledge BN. DCCT/EDIC Research Group. Effect of glycemic exposure on the risk of microvascular complications in the diabetes control and complications trial: revisited. Diabetes. 2008; 57:995–1001.
19. Brownlee M. Biochemistry and molecular cell biology of diabetic complications. Nature. 2001; 414:813–820.
20. Ceriello A, Esposito K, Piconi L, Ihnat MA, Thorpe JE, Testa R, Boemi M, Giugliano D. Oscillating glucose is more deleterious to endothelial function and oxidative stress than mean glucose in normal and type 2 diabetic patients. Diabetes. 2008; 57:1349–1354.
21. Monnier L, Colette C, Mas E, Michel F, Cristol JP, Boegner C, Owens DR. Regulation of oxidative stress by glycaemic control: evidence for an independent inhibitory effect of insulin therapy. Diabetologia. 2010; 53:562–571.
22. Monnier L, Mas E, Ginet C, Michel F, Villon L, Cristol JP, Colette C. Activation of oxidative stress by acute glucose fluctuations compared with sustained chronic hyperglycemia in patients with type 2 diabetes. JAMA. 2006; 295:1681–1687.
23. Siegelaar SE, Barwari T, Kulik W, Hoekstra JB, DeVries JH. No relevant relationship between glucose variability and oxidative stress in well-regulated type 2 diabetes patients. J Diabetes Sci Technol. 2011; 5:86–92.
24. Wentholt IM, Kulik W, Michels RP, Hoekstra JB, DeVries JH. Glucose fluctuations and activation of oxidative stress in patients with type 1 diabetes. Diabetologia. 2008; 51:183–190.
25. Kilpatrick ES, Rigby AS, Atkin SL. The effect of glucose variability on the risk of microvascular complications in type 1 diabetes. Diabetes Care. 2006; 29:1486–1490.
26. Kilpatrick ES, Rigby AS, Atkin SL. Effect of glucose variability on the long-term risk of microvascular complications in type 1 diabetes. Diabetes Care. 2009; 32:1901–1903.
27. Service FJ, O'Brien PC. The relation of glycaemia to the risk of development and progression of retinopathy in the Diabetic Control and Complications Trial. Diabetologia. 2001; 44:1215–1220.
28. Siegelaar SE, Kilpatrick ES, Rigby AS, Atkin SL, Hoekstra JB, Devries JH. Glucose variability does not contribute to the development of peripheral and autonomic neuropathy in type 1 diabetes: data from the DCCT. Diabetologia. 2009; 52:2229–2232.
29. Bragd J, Adamson U, Backlund LB, Lins PE, Moberg E, Oskarsson P. Can glycaemic variability, as calculated from blood glucose self-monitoring, predict the development of complications in type 1 diabetes over a decade? Diabetes Metab. 2008; 34(6 Pt 1):612–616.
30. Soupal J, Skrha J Jr, Fajmon M, Horova E, Mraz M, Skrha J, Prazny M. Glycemic variability is higher in type 1 diabetes patients with microvascular complications irrespective of glycemic control. Diabetes Technol Ther. 2014; 16:198–203.
31. Sartore G, Chilelli NC, Burlina S, Lapolla A. Association between glucose variability as assessed by continuous glucose monitoring (CGM) and diabetic retinopathy in type 1 and type 2 diabetes. Acta Diabetol. 2013; 50:437–442.
32. Kilpatrick ES, Rigby AS, Atkin SL. A1C variability and the risk of microvascular complications in type 1 diabetes: data from the Diabetes Control and Complications Trial. Diabetes Care. 2008; 31:2198–2202.
33. Gimeno-Orna JA, Castro-Alonso FJ, Boned-Juliani B, Lou-Arnal LM. Fasting plasma glucose variability as a risk factor of retinopathy in type 2 diabetic patients. J Diabetes Complications. 2003; 17:78–81.
34. Takao T, Ide T, Yanagisawa H, Kikuchi M, Kawazu S, Matsuyama Y. The effects of fasting plasma glucose variability and time-dependent glycemic control on the long-term risk of retinopathy in type 2 diabetic patients. Diabetes Res Clin Pract. 2011; 91:e40–e42.
35. Zoppini G, Verlato G, Targher G, Casati S, Gusson E, Biasi V, Perrone F, Bonora E, Muggeo M. Is fasting glucose variability a risk factor for retinopathy in people with type 2 diabetes? Nutr Metab Cardiovasc Dis. 2009; 19:334–339.
36. Kilpatrick ES, Rigby AS, Atkin SL. Mean blood glucose compared with HbA1c in the prediction of cardiovascular disease in patients with type 1 diabetes. Diabetologia. 2008; 51:365–371.
37. Muggeo M, Verlato G, Bonora E, Zoppini G, Corbellini M, de Marco R. Long-term instability of fasting plasma glucose, a novel predictor of cardiovascular mortality in elderly patients with non-insulin-dependent diabetes mellitus: the Verona Diabetes Study. Circulation. 1997; 96:1750–1754.
38. Siegelaar SE, Kerr L, Jacober SJ, Devries JH. A decrease in glucose variability does not reduce cardiovascular event rates in type 2 diabetic patients after acute myocardial infarction: a reanalysis of the HEART2D study. Diabetes Care. 2011; 34:855–857.
39. Monnier L, Colette C. Glycemic variability: can we bridge the divide between controversies? Diabetes Care. 2011; 34:1058–1059.
40. Su G, Mi SH, Tao H, Li Z, Yang HX, Zheng H, Zhou Y, Tian L. Impact of admission glycemic variability, glucose, and glycosylated hemoglobin on major adverse cardiac events after acute myocardial infarction. Diabetes Care. 2013; 36:1026–1032.
41. Wang X, Zhao X, Dorje T, Yan H, Qian J, Ge J. Glycemic variability predicts cardiovascular complications in acute myocardial infarction patients with type 2 diabetes mellitus. Int J Cardiol. 2014; 172:498–500.
42. Su G, Mi S, Tao H, Li Z, Yang H, Zheng H, Zhou Y, Ma C. Association of glycemic variability and the presence and severity of coronary artery disease in patients with type 2 diabetes. Cardiovasc Diabetol. 2011; 10:19.
43. Esposito K, Giugliano D, Nappo F, Marfella R. Campanian Postprandial Hyperglycemia Study Group. Regression of carotid atherosclerosis by control of postprandial hyperglycemia in type 2 diabetes mellitus. Circulation. 2004; 110:214–219.
44. Snell-Bergeon JK, Roman R, Rodbard D, Garg S, Maahs DM, Schauer IE, Bergman BC, Kinney GL, Rewers M. Glycaemic variability is associated with coronary artery calcium in men with type 1 diabetes: the Coronary Artery Calcification in Type 1 Diabetes study. Diabet Med. 2010; 27:1436–1442.
45. Barbieri M, Rizzo MR, Marfella R, Boccardi V, Esposito A, Pansini A, Paolisso G. Decreased carotid atherosclerotic process by control of daily acute glucose fluctuations in diabetic patients treated by DPP-IV inhibitors. Atherosclerosis. 2013; 227:349–354.
46. Mo Y, Zhou J, Li M, Wang Y, Bao Y, Ma X, Li D, Lu W, Hu C, Li M, Jia W. Glycemic variability is associated with subclinical atherosclerosis in Chinese type 2 diabetic patients. Cardiovasc Diabetol. 2013; 12:15.
47. Raz I, Wilson PW, Strojek K, Kowalska I, Bozikov V, Gitt AK, Jermendy G, Campaigne BN, Kerr L, Milicevic Z, Jacober SJ. Effects of prandial versus fasting glycemia on cardiovascular outcomes in type 2 diabetes: the HEART2D trial. Diabetes Care. 2009; 32:381–386.