Abstract
Background
The Achutha Menon Centre Diabetes Risk Score (AMCDRS), which was developed in rural Kerala State, South India, had not previously been externally validated. We examined the performance of the AMCDRS in urban and rural areas in the district of Vellore in the South Indian state of Tamil Nadu, and compared it with other diabetes risk scores developed from India.
Methods
We used the data from 4,896 participants (30 to 64 years) of a cross-sectional study conducted in Vellore (2010 to 2012), to calculate the AMCDRS scores using age, family history, and waist circumference. Sensitivity, specificity, positive predictive value (PPV), and negative predictive values (NPV), and the area under the receiver operating characteristic curve (AROC) were calculated for undiagnosed and total diabetes.
Results
Of the 4,896 individuals surveyed, 274 (5.6%) had undiagnosed diabetes and 759 (15.5%) had total diabetes. The AMCDRS, with an optimum cut-point of ≥4, identified 45.0% for further testing with 59.5% sensitivity, 60.5% specificity, 9.1% PPV, 95.8% NPV, and an AROC of 0.639 (95% confidence interval [CI], 0.608 to 0.670) for undiagnosed diabetes. The corresponding figures for total diabetes were 75.1%, 60.5%, 25.9%, 93.0%, and 0.731 (95% CI, 0.713 to 0.750), respectively. The AROC for the AMCDRS was not significantly different from that of the Indian Diabetes Risk Score, the Ramachandran or the Chaturvedi risk scores for total diabetes, but was significantly lower than the AROC of the Chaturvedi score for undiagnosed diabetes.
Type 2 diabetes mellitus (T2DM) is now a major cause of morbidity and mortality worldwide, particularly in low and middle-income countries such as India [1]. Currently India is home to an estimated 69.2 million people with T2DM and an estimated 52% of these cases are undiagnosed [1]. Undiagnosed diabetes is frequently associated with complications affecting the heart, blood vessels, kidneys, and nerves [1]. Furthermore, several randomised controlled trials have unequivocally shown that the onset of T2DM can be delayed or prevented through lifestyle and pharmacological interventions [234]. For these reasons, early identification and treatment of individuals with T2DM is essential.
Universal blood testing for screening for T2DM is not feasible, especially in resource constrained settings such as in India. Simple, low-cost screening tools such as diabetes risk scores have therefore been developed for use in various populations throughout the world, including four in India [567891011]. The first of these, the Indian Diabetes Risk Score (IDRS) [9], collects information on age, family history of diabetes, waist circumference, and physical activity. The second, the Ramachandran risk score [10], includes body mass index (BMI) in addition to the four variables used for the IDRS. The third, the Chaturvedi risk score [11], includes information on age, family history of diabetes, waist circumference, and hypertension. All three have proved useful in identifying undiagnosed T2DM. Variables such as BMI, blood pressure (BP), and physical activity may, however, be cumbersome to measure in low-resource primary care settings such as in some parts of India. This is because measurements such as BMI and BP require expensive instruments and standardization [12], while measuring physical activity using self-reported questionnaires takes time and has low validity and reliability [13].
The fourth diabetes risk score, the Achutha Menon Centre Diabetes Risk Score (AMCDRS), is a simple diabetes risk score developed using data from a cross-sectional study conducted in rural Kerala, India, to identify people with total diabetes (diagnosed and undiagnosed) [1415]. The AMCDRS includes three simple variables—age, family history of diabetes, and waist circumference—which are relatively easy to measure in primary care settings in India.
In the original study using the AMCDRS, the number of individuals with undiagnosed diabetes was low and therefore the AMCDRS's performance in identifying undiagnosed cases of diabetes could not be assessed [14]. Furthermore, the AMCDRS has not been externally validated. The study that is the subject of this article therefore aims to validate the AMCDRS by examining its performance in identifying undiagnosed diabetes, as well as total diabetes, in urban and rural Vellore district in the South Indian state of Tamil Nadu. For this study, we compared the performance of other Indian risk scores—IDRS, Ramachandran risk score, and the Chaturvedi risk score [91011]—with that of the AMCDRS. We also estimated the number needed to screen (NNS), in order to identify one case of undiagnosed diabetes, and the cost per case detected using a two-step screening procedure (risk score followed by fasting plasma glucose [FPG]). The results of these estimates were compared with universal screening using FPG.
All procedures performed in the study were approved by the Institutional Review Board of the Christian Medical College, Vellore (IRB. Min. No. 7020 dated 16-12-2009) and were in accordance with the ethical standards specified by the 1964 Helsinki Declaration and its later amendments. Written informed consent was obtained from all participants.
A cross sectional survey of 6,196 individuals aged 30 to 64 years was conducted in 2010 to 2012 in urban and rural Vellore using the World Health Organisation's (WHO) STEPwise approach to surveillance (STEPS) [16]. The aim of this survey was to study the prevalence of coronary heart disease and its risk factors [1718].
For the rural sample, all adults aged 30 to 64 years in nine randomly selected villages in Kaniyambadi, a rural block, were invited to participate in the study. For the urban sample, all adults aged 30 to 64 years in one randomly selected street in 48 different wards in Vellore city were surveyed for the study [17]. Eligible adults were interviewed at home and then invited to local study clinics where physical and biochemical measurements (FPG and lipids) were taken. Physical activity was assessed using the Global Physical Activity Questionnaire, which is a part of the STEPS methodology [16].
The data from this study was used to compute the AMCDRS for both the rural and urban subjects [17]. The maximum score possible for the AMCDRS is 6, with maximum weightage for age (age 55 to 64 years acquires a score of 4, 45 to 54 years a score of 3, 35 to 44 years a score of 2, and <35 years a score of 0). A score of 1 each was given for positive family history of diabetes (parents or siblings) and abdominal obesity (waist circumference ≥80 cm in women and ≥90 cm in men) [14]. T2DM was defined as either FPG ≥126 mg/dL or being on anti-diabetic medications [16]. Sensitivity, specificity, positive predictive value (PPV), and negative predictive value (NPV) for undiagnosed diabetes and total diabetes were calculated for various possible cut-off values of the AMCDRS. Area under the receiver operating characteristic curve (AROC) was used to determine the optimum cut-off value for undiagnosed diabetes and total diabetes. Other risk scores—IDRS, Ramachandran, and Chaturvedi—were also computed, and sensitivity, specificity, PPV, NPV, and the AROC were calculated for the published cut-off values [91011]. The AROC for the AMCDRS was compared with the three other risk scores for undiagnosed diabetes and total diabetes.
We estimated the NNS for identifying one case of undiagnosed diabetes and the cost per case detected using a two-step screening process (risk score followed by FPG among those who were screen positive on the risk score) and for universal screening with FPG using a previously reported methodology [19]. The number of people eligible for screening in this costing analysis were those who were not on anti-diabetic medication, for whom data was available to calculate the AMCDRS (n=4,411 participants). The costs of FPG testing (material and personnel costs) and questionnaires (printing and supplies), were taken from figures used for a recent economic analysis on a simulated national population, which used inputs from the WHO CHOICE (CHOosing Interventions that are Cost-Effective) guide [1920]. Additional costs, such as payments for personnel (from national level managers to district health workers) and operational costs (transport, office rent, and utilities) were not measured.
Of the 6,196 study participants, data on diabetes status as well as risk scores were available for 4,896 (3,051 rural and 1,845 urban), comprising 79% of the study population. The mean age of the sample was 45 years, with 43% males and literacy rates of 65% in the rural area and 77% in the urban areas. While 46% of the rural population was engaged in agriculture or non-agricultural manual labour, only 13% of the urban sample was involved in manual labour.
The study identified 759 participants (15.5%) with total diabetes. Of these 759 diabetics, 274 were previously undiagnosed and the remaining 485 were known diabetics. The optimum cut-off value of the AMCDRS was 4 and above for both undiagnosed diabetes and total diabetes. The proportion of individuals who were screen positive was 45.0% (47.8% in the urban sample and 36.9% in the rural sample).
A higher AMCDRS was associated with a higher total diabetes prevalence (chi-square for trend 478.63, P<0.001). The prevalence of T2DM was 47.3% among those with a score of 6, as compared to 1.5% among those with a score of 0. A similar relationship was observed for undiagnosed diabetes (chi-square for trend 65.47, P<0.001), where the prevalence was 9.3% among those with a score of 6, as compared to 1.2% among those with a score of 0.
Sensitivity, specificity, and predictive values for the AMCDRS in identifying undiagnosed diabetes and total diabetes are given in Table 1. Table 2 shows the sensitivity, specificity, and predictive values of the IDRS, Ramachandran, and Chaturvedi scores for predicting undiagnosed diabetes. A comparison of the two tables shows that the AMCDRS's sensitivity and specificity were closest to those of the IDRS.
Figs. 1 and 2 show the AROC curves for the AMCDRS and other Indian risk scores for undiagnosed diabetes and total diabetes. The AROC of the AMCDRS for undiagnosed diabetes was 0.638 (95% confidence interval [CI], 0.624 to 0.652), which was significantly lower than that of the Chaturvedi risk score (P<0.001) and similar to the AROC of the other risk scores. The AROC of the AMCDRS for total diabetes was 0.731 (95% CI, 0.713 to 0.750) which was significantly lower than the Chaturvedi score (P=0.001), but higher than the other scores (P<0.01).
The NNS to detect one case of undiagnosed diabetes was lower for universal screening with FPG (NNS, 16), as compared to 21 to 27 for the two-step screening procedure (risk score followed by confirmation with FPG) (Table 3). The IDRS and the AMCDRS had similar NNS values (NNS, 27) and these were lower than the values for the Chaturvedi score (NNS, 24) and Ramachandran score (NNS, 21). The cost per case detected, however, was highest (US$ 39.2) for universal screening with FPG and lowest (US$ 24.1) for the two-step screening procedure with the Chaturvedi score. The IDRS and the AMCDRS had similar values for cost per case detected, US$ 28.3 and 28.8, respectively (Table 3).
Our study confirmed that the AMCDRS's best cut-off for identifying undiagnosed diabetes and total diabetes was 4, as shown in the original study by Sathish et al. [14]. The original AMCDRS study conducted in Kerala showed a sensitivity and specificity of 81% and 68% with an AROC of 0.812 (95% CI, 0.765 to 0.860), for an optimal cut-off value of 4 [14]. The sensitivity of 75.1% and specificity of 60.5% obtained from this study in the state of Tamil Nadu confirms the score's usefulness for screening for diabetes.
The original study in Kerala was probably not powered to assess the validity of the AMCDRS for identifying undiagnosed diabetes, as the number of participants with undiagnosed diabetes was low (n=42) [14]. This study from Tamil Nadu which included 274 cases of undiagnosed diabetes, showed a lower performance of AMCDRS for undiagnosed diabetes (AROC, 0.638) as compared to total diabetes (AROC, 0.731). The study for which the Chaturvedi risk score was developed showed a similar finding, where the risk score had a lower AROC (0.69) for undiagnosed diabetes as compared to total diabetes (AROC, 0.74) [11]. This discrepancy in the performance of the risk scores in identifying undiagnosed and total diabetes might be because older participants or those with a family history of diabetes are more likely to develop diabetes at an earlier stage, and thus already be diabetic at the time of screening [112122].
The AMCDRS's performance in identifying total diabetes was similar to that of other Indian risk scores, which include variables such as BMI, BP, and physical activity that are relatively difficult to measure in low-resource primary care settings in India, due to the need for expensive instruments [23]. It should be acknowledged however, that measuring waist circumference requires adequate training to ensure measurement accuracy [16]. For detecting undiagnosed diabetes, the AMCDRS did not perform as well as the Chaturvedi risk score which also includes hypertension along with the three other variables included in the AMDRS—age, family history, and waist circumference. This could be explained by the fact that hypertension has a strong association with diabetes [24]. Measuring BP accurately in primary care settings is not easy however, as it requires at least three readings to confirm a diagnosis of hypertension with a calibrated BP apparatus [16].
In this study, the NNS was higher when the risk score based approach was used as compared to universal FPG testing. The previous microsimulation study by Basu et al. [19] had also found that the NNS was higher with risk score based screening or random glucometer based screening (NNS ranging from 15.2 to 21.7) as compared to FPG (NNS, 11), due to the low sensitivity of the risk score based approaches.
The cost per case detected, however, was lower for the risk score based approach than for universal FPG testing in this study, for all risk scores including the AMCDRS. The microsimulation study had shown that the lowest cost per case detected would be for random glucometer based screening (US$ 5.28) as compared to risk score based two-step screening (US$ 13.01 to 17.06) [19]. As we did not obtain random glucometer values, we were unable to compare costs per case detected between risk scores and random point of care glucometer testing. Thus, when random glucometer based screening is impractical (due to lack of resources), a two-step screening using an initial risk score could be considered as an alternative screening method.
Our study had certain limitations. Firstly, as mentioned earlier, we could only calculate the AMCDRS and other risk scores for 79% of the study population. However, the sample size was large enough to validate a diabetes risk score. Secondly, the diagnosis of T2DM was made using FPG as recommended for the WHO STEPS survey [16], and not by a 75-g oral glucose tolerance test, the current gold standard for diagnosing T2DM [25]. Thirdly, our study findings may not be able to be generalised to other states in India owing to differences in diabetes prevalence and the distribution of risk factors. The states of Tamil Nadu and Kerala, for example, have a much higher burden of diabetes as compared to that of other states in India [151726]. Finally, the cost analysis was a simplified analysis as only actual screening tool costs were considered and not those additional costs associated with personnel and operations.
To conclude, the AMCDRS is a simple diabetes risk score that field workers and volunteers can use in low-resource primary care settings to screen for undiagnosed diabetes and total diabetes. If the AMCDRS was recalibrated, its performance in identifying undiagnosed diabetes would probably improve. If there are sufficient resources, additional parameters such as BP and BMI for calculating risk would improve prediction. Cohort studies are required to assess the performance of the AMCDRS in predicting incident T2DM.
ACKNOWLEDGMENTS
The cross-sectional survey in Vellore was funded by the Indian Council of Medical Research (grant no. 50/3/TF/DV/06-NCD-II).
The authors thank Kavumpurathu Raman Thankappan from the Achutha Menon Centre for Health Science Studies at the Sree Chitra Tirunal Institute for Medical Sciences and Technology, Trivandrum, Kerala, for his valuable advice and suggestions. Sathish was supported by the ASCEND Program, funded by the Fogarty International Centre of the National Institutes of Health (NIH), Bethesda, MD, USA (Award Number: D43TW008332). We also thank the ENCORE (Excellence in NonCoOmmunicable disease REsearch) program funded by the International Research and Research Training Fund (IRRTF), University of Melbourne, Melbourne, Australia, for enabling this collaborative work.
References
1. International Diabetes Federation. IDF diabetes atlas. 7th ed. Brussels: International Diabetes Federation;2015.
2. Knowler WC, Barrett-Connor E, Fowler SE, Hamman RF, Lachin JM, Walker EA, Nathan DM. Diabetes Prevention Program Research Group. Reduction in the incidence of type 2 diabetes with lifestyle intervention or metformin. N Engl J Med. 2002; 346:393–403. PMID: 11832527.


3. Ramachandran A, Snehalatha C, Mary S, Mukesh B, Bhaskar AD, Vijay V. Indian Diabetes Prevention Programme (IDPP). The Indian Diabetes Prevention Programme shows that lifestyle modification and metformin prevent type 2 diabetes in Asian Indian subjects with impaired glucose tolerance (IDPP-1). Diabetologia. 2006; 49:289–297. PMID: 16391903.


4. Lindstrom J, Louheranta A, Mannelin M, Rastas M, Salminen V, Eriksson J, Uusitupa M, Tuomilehto J. Finnish Diabetes Prevention Study Group. The Finnish Diabetes Prevention Study (DPS): lifestyle intervention and 3-year results on diet and physical activity. Diabetes Care. 2003; 26:3230–3236. PMID: 14633807.
5. Herman WH, Smith PJ, Thompson TJ, Engelgau MM, Aubert RE. A new and simple questionnaire to identify people at increased risk for undiagnosed diabetes. Diabetes Care. 1995; 18:382–387. PMID: 7555482.


6. Bang H, Edwards AM, Bomback AS, Ballantyne CM, Brillon D, Callahan MA, Teutsch SM, Mushlin AI, Kern LM. Development and validation of a patient self-assessment score for diabetes risk. Ann Intern Med. 2009; 151:775–783. PMID: 19949143.


7. Lindstrom J, Tuomilehto J. The diabetes risk score: a practical tool to predict type 2 diabetes risk. Diabetes Care. 2003; 26:725–731. PMID: 12610029.
8. Glumer C, Carstensen B, Sandbaek A, Lauritzen T, Jorgensen T, Borch-Johnsen K. Inter99 study. A Danish diabetes risk score for targeted screening: the Inter99 study. Diabetes Care. 2004; 27:727–733. PMID: 14988293.
9. Mohan V, Deepa R, Deepa M, Somannavar S, Datta M. A simplified Indian Diabetes Risk Score for screening for undiagnosed diabetic subjects. J Assoc Physicians India. 2005; 53:759–763. PMID: 16334618.
10. Ramachandran A, Snehalatha C, Vijay V, Wareham NJ, Colagiuri S. Derivation and validation of diabetes risk score for urban Asian Indians. Diabetes Res Clin Pract. 2005; 70:63–70. PMID: 16126124.


11. Chaturvedi V, Reddy KS, Prabhakaran D, Jeemon P, Ramakrishnan L, Shah P, Shah B. Development of a clinical risk score in predicting undiagnosed diabetes in urban Asian Indian adults: a population-based study. CVD Prev Control. 2008; 3:141–151.
12. John J, Muliyil J, Balraj V. Screening for hypertension among older adults: a primary care “high risk” approach. Indian J Community Med. 2010; 35:67–69. PMID: 20606923.


13. Mathews E, Salvo D, Sarma PS, Thankappan KR, Pratt M. Adapting and validating the Global Physical Activity Questionnaire (GPAQ) for Trivandrum, India, 2013. Prev Chronic Dis. 2016; 13:E53. PMID: 27103263.


14. Sathish T, Kannan S, Sarma PS, Thankappan KR. Achutha Menon Centre Diabetes Risk Score: a type 2 diabetes screening tool for primary health care providers in rural India. Asia Pac J Public Health. 2015; 27:147–154. PMID: 22865719.
15. Thankappan KR, Shah B, Mathur P, Sarma PS, Srinivas G, Mini GK, Daivadanam M, Soman B, Vasan RS. Risk factor profile for chronic non-communicable diseases: results of a community-based study in Kerala, India. Indian J Med Res. 2010; 131:53–63. PMID: 20167974.
16. World Health Organization. STEP wise approach to surveillance. cited 2017 Jun 18. Available from: http://www.who.int/chp/steps/en/.
17. Oommen AM, Abraham VJ, George K, Jose VJ. Rising trend of cardiovascular risk factors between 1991-1994 and 2010-2012: a repeat cross sectional survey in urban and rural Vellore. Indian Heart J. 2016; 68:263–269. PMID: 27316476.


18. Oommen AM, Abraham VJ, George K, Jose VJ. Prevalence of coronary heart disease in rural and urban Vellore: a repeat cross-sectional survey. Indian Heart J. 2016; 68:473–479. PMID: 27543468.


19. Basu S, Millett C, Vijan S, Hayward RA, Kinra S, Ahuja R, Yudkin JS. The health system and population health implications of large-scale diabetes screening in India: a microsimulation model of alternative approaches. PLoS Med. 2015; 12:e1001827. PMID: 25992895.


20. World Health Organization. Cost effectiveness and strategic planning (WHO-CHOICE). cited 2017 Jun 18. Available from: http://www.who.int/choice/cost-effectiveness/en/.
21. Erasmus RT, Blanco Blanco E, Okesina AB, Mesa Arana J, Gqweta Z, Matsha T. Importance of family history in type 2 black South African diabetic patients. Postgrad Med J. 2001; 77:323–325. PMID: 11320276.


22. Turi KN, Buchner DM, Grigsby-Toussaint DS. Predicting risk of type 2 diabetes by using data on easy-to-measure risk factors. Prev Chronic Dis. 2017; 14:E23. PMID: 28278129.


23. Sathish T, Kannan S, Sarma SP, Thankappan KR. Screening performance of diabetes risk scores among Asians and Whites in rural Kerala, India. Prev Chronic Dis. 2013; 10:E37. PMID: 23517580.


24. Izzo R, de Simone G, Chinali M, Iaccarino G, Trimarco V, Rozza F, Giudice R, Trimarco B, De Luca N. Insufficient control of blood pressure and incident diabetes. Diabetes Care. 2009; 32:845–850. PMID: 19223610.


25. World Health Organization. Definition and diagnosis of diabetes mellitus and intermediate hyperglycemia: report of a WHO/IDF consultation. cited 2017 Jun 18. http://www.who.int/diabetes/publications/Definition%20and%20diagnosis%20of%20diabetes_new.pdf.
26. Mohan V, Sandeep S, Deepa R, Shah B, Varghese C. Epidemiology of type 2 diabetes: Indian scenario. Indian J Med Res. 2007; 125:217–230. PMID: 17496352.
Fig. 1
Receiver operating characteristic curves of various risk scores for diagnosis of undiagnosed diabetes. AMCDRS, Achutha Menon Centre Diabetes Risk Score; IDRS, Indian Diabetes Risk Score.
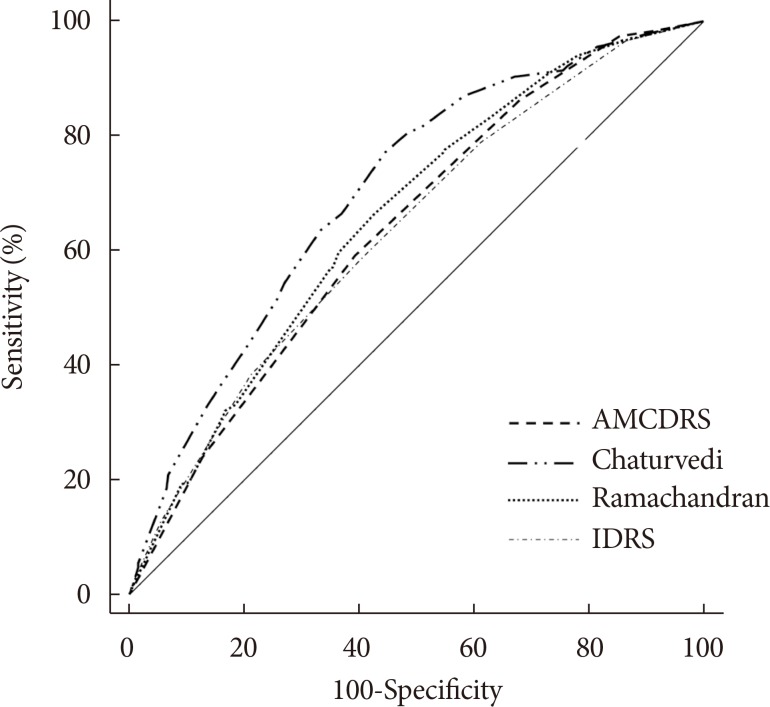
Fig. 2
Receiver operating characteristic curves of various risk scores for diagnosis of total diabetes. AMCDRS, Achutha Menon Centre Diabetes Risk Score; IDRS, Indian Diabetes Risk Score.
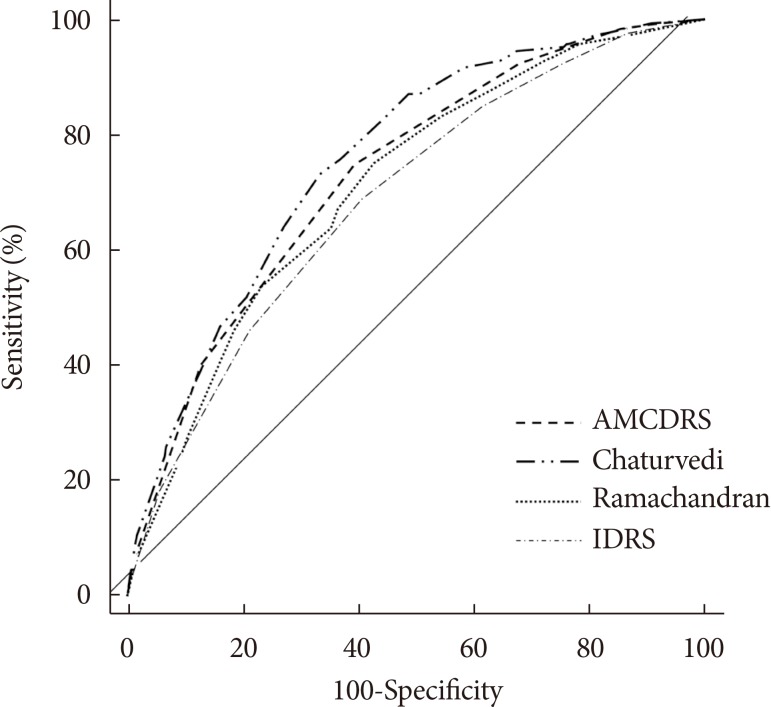
Table 1
Validity of the Achutha Menon Centre Diabetes Risk Score for predicting prevalent diabetes
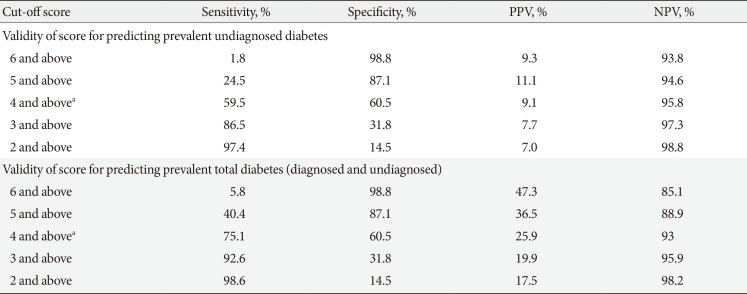
PPV, positive predictive value; NPV, negative predictive value.
aOptimal score both in this study as well as the study by Sathish et al. [14].
Table 2
Validity of other risk scores from India, in predicting prevalent diabetes
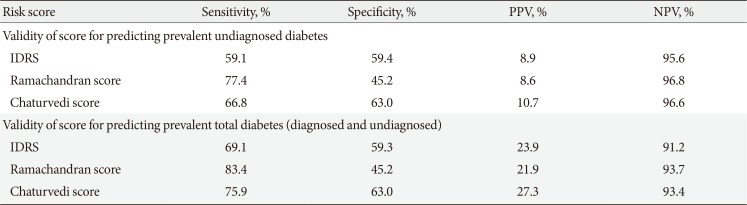
Table 3
Cost per case detected for universal screening versus risk score followed by confirmation
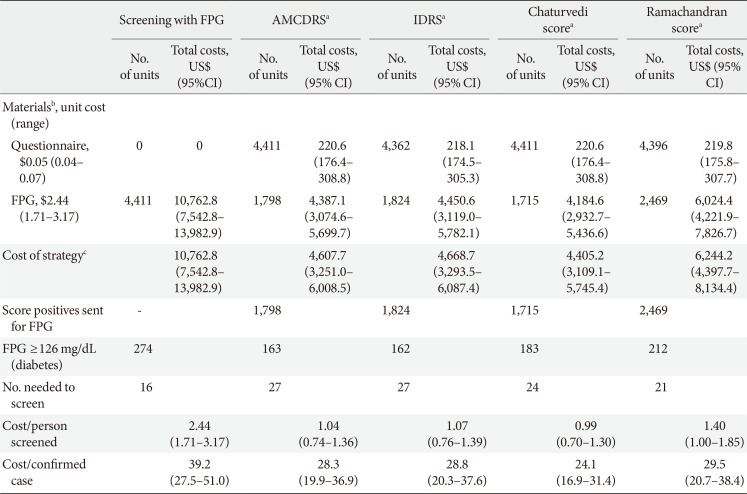
FPG, fasting plasma glucose; AMCDRS, Achutha Menon Centre Diabetes Risk Score; IDRS, Indian Diabetes Risk Score; CI, confidence interval.
aAll risk score based screening followed by a confirmatory FPG, bCosts in 2014 US$ obtained from a modelling study by Basu et al. [19], cOperation costs and personnel costs (training for glucometer use or questionnaire based survey) assumed to be the same for both strategies.