Abstract
Methods
We utilized the keywords, as the main variables, of the research papers that were presented in the journal and symposia of the Korean Society of Medical Informatics, and we used, as cases, the English titles and abstracts of the papers (n = 915) published from 1995 through 2008. N-grams (bigram to 5-gram) were extracted from the corpora using the BiKE Text Analyzer, and their cooccurrence networks were generated via a cosine correlation coefficient, and then the networks were analyzed and visualized using Pajek.
Characterizing a domain of study presupposes the understanding of its knowledge structure. Since its inception in 1987, there have been few efforts to understand Korean medical informatics in quantitative way. The purpose of this paper was to quantitatively analyze the knowledge structure of Korean medical informatics.
There are a number of qualitative studies on biomedical informatics [1-3]. These research efforts heavily relied on experts' knowledge, experience, and intuition. Thus they may lack objective and quantitative understanding of the structure of the field. Mainstream approaches exploring the knowledge structure of a domain are quantitative studies such as co-citation analysis (CCA) and co-word analysis (CWA) which gauge topical closeness, similarity in the level of authors, papers, journals, or disciplines.
The most extensively used method, CWA, can reveal the overall picture and broad landscape and boundaries of a given field. CWA uses patterns of co-occurrence of pairs of words or phrases in a corpus of texts to identify the relationships between ideas within the subject areas presented in texts [4]. Since their introduction [5], CWA techniques have been used to explore knowledge structure [6-8], analyze research trends [4,7,9-11], and generate hypothesis and discover knowledge [12-16]. In this study, we utilize CWA for exploring the knowledge structure of Korean medical informatics.
The following questions guided this research: 1) What are the important topics of Korean medical informatics? 2) What are the newly emerging research topics?
This paper begins with data and analysis methods to find the knowledge structure of Korean medical informatics. Then research findings such as important research topics and their contexts, and newly emerging topics are covered. Finally, we discuss the major findings and conclude the paper.
In this study, we adopted a well established co-word analysis protocol. It involves the following steps: 1) select the text corpus for the study; 2) extract and normalize the terms and get term weights; 3) get a term co-occurrence frequency matrix for the corpus; 4) get term-term relatedness; 5) analyze the term-term relatedness matrix, and visualize it. For this study BiKE Text Analyzer (BTA), a Java application was used.
The time window for the target data was set as the 14 years from 1995 to 2008. We collected 1,075 papers' titles and abstracts published in the journal and symposia of Korean Society of Medical Informatics. Abstract-free papers were excluded from the corpus to have 915 for analysis. For consistency, Korean titles and abstracts of 295 papers was translated into English through Google Translator Toolkit. Then, the English terms were corrected, which have different meanings from original ("화상진료시스템" ==> "Burn care system" --> "telemedicine system"), were Romanized as pronounced ("기록지"==> "girokji" --> "record"), and have not been marked as medical terminology ("검사" ==> "inspection" --> "lab test").
In a co-word analysis, the critical step is to create a list of terms that constitute the variables for analysis. Our variables were created from a combination of the sources including: 1) symposium topic lists; 2) author keywords and biomedical informatics keywords from Thomson Web of Science; and 3) MeSH descriptors. Research topics were collected from call for paper topics and session titles in all symposia. The collected terms were appended to the Vocabulary Manager of BTA. The Vocabulary Manager automatically erases duplicate terms and manages n-grams (up to 5-grams), and it allows users to load new vocabularies and input new terms (Figure 1).
Topics are difficult to represent with single words because they often have more than one meaning. In most cases, topics are appropriately described in multi-word phrases, which, especially in research domains, are much more interpretable [17]. In this study, we view a topic as a multi-word phrase rather than a single word. Before we proceeded to extract phrases and obtain precise variables, tokenized words' plural forms were singularized and their synonyms controlled. We adopted a less strict normalization strategy for words: tokenized words' plural forms were singularized (eg, records to record) and abbreviations were controlled with synonym lists (eg, HER = electronic health record). From paper corpora, we extracted n-gram terms (from 2-grams to 5-grams) as variables using BTA (total number of n-gram terms = 2,954). The most frequently occurring term was "information system" (term frequency = 533). After excluding the terms that occurred less than 5 times and inappropriate to be a variable (eg, "two type"), the term variables for analysis became 748.
Since not all terms may have the same importance in a document, the weight of each term was calculated by multiplying term frequencies (TF) by the inverse document frequency (IDF) for that term.
where fi,j is the number of times the term i appears in the document j, Σknk,j is the total number of terms in the document d, N is the total number of documents, and ni is the total number of documents containing the term i.
The co-occurrence analysis approach quantifies term co-occurrences in documents. It assumes that the more frequently two terms appear together in the same document, the sooner they will be identified as being closely related [18]. BTA generates a term co-occurrence frequency matrix (748 × 748), and then transforms the matrix into a cosine correlation matrix, where each cell indicates the relative closeness of each term pair with a 0-1 range. The cosine measure is defined as the cosine of the angle enclosed between two term vectors x and y:
The term-term closeness matrix was converted to a social network showing the binary relationships between any two terms. This network provides a useful medium for representing the topical structure of Korean medical informatics in a concise and intuitive manner. Pajek software was used for network visualization and analysis. The node size equals to the logarithm of the term frequency, and the thickness of the lines indicates the cosine value (closeness) between a pair of terms.
Some terms have links with many terms; their network of co-occurrences is quite extensive and occupies a central position in a field. To identify the important research topics in Korean medical informatics, authority and hub scores were calculated for each topic. In social network analysis, if a vertex points to many good authorities, it is a good hub. And if a vertex is pointed to by many good hubs, it is a good authority [19]. The authority scores and hub scores of topics are rendered as:
The authority score of a topic i (Ti) equals the sum of the hub scores of all topics (Tj) that point to it. The hub score (Ti) of a topic i (Ti) equals to the sum of the authority scores of all topics that it points to. Authority scores mutually reinforce hub scores. As shown in Table 1, we extracted the 50 most important topics in Korean medical informatics during the past 14 years (1995-2008). Table 2 shows that top 19 topics with high authority score occupy about 3.5% of 748 topics.
As shown in Figure 2, the 50 most important topics were grouped into 12 clusters: information system, decision support system, picture archiving and communication system, electronic health record, electronic medical record, XML, university hospital, healthcare, and so on. It is comparable to the findings of a study in global scale, in which physician order entry and practice guideline are one of the major topics as shown in Figure 3. It is also interesting that the information system group (upper right corner of Figure 2) is closely associated with nursing science topics such as nursing record, nursing process, nursing activity, nursing information, and so on.
To investigate newly emerging topics, for each 3-year period (2 years for 2007 and 2008), we calculated term frequencies and identified the topics which represented the lowest 10% in the low frequency group in the preceding period(s), and which also remained in the highest 10% (5% in the years 2007-2008) in the high frequency group in the following periods.
During the past 14 years (1995-1998), information system, medical record, hospital information, management system, hospital information system, health information, web based, and information technology have been occupying top 5% of Korean medical informatics research topics. Some of the newly emerging research topics during the years 1998-2000 are nursing informatics and electronic medical record, during the years 2001-2003 are consumer health. Electronic health record system, information extraction, and ubiquitous healthcare are newly popular topics during 2004-2006. During 2007-2008 oriental nursing, bioinformatics, ubiquitous computing, personal health device are some of newly emerging topics (Figures 4, 5, 6).
The social network analysis of research topics communicated through the KOSMI journal and symposia provides a systematic overview on the knowledge structure of Korean medical informatics. From our analysis it is supposed that Korean medical informatics has been paying attention to the information artifacts such as EHR (EMR), CDSS, PACS and so on, but less to methodological topics (eg, machine learning, natural language processing, support vector machine) and their applications (eg, computerized physician order entry, clinical practice guideline) which are some of major topics in the global scale. Since the early 2000s, bioinformatics related topics (eg, expression data) have been emerging in the global scale [20], whereas in Korean only since the years of 2007-2008 (eg, bioinformatics data). These suggest that Korean medical informatics should be equipped with more rigorous methodologies and pay more attention to bioinformatics.
Several contributions of this study are notable. This study provides topic networks for systematic understanding of Korean medical informatics, and helps to gain a first insight into the main research interest in Korea. Our research trend analysis also helps to decide which technologies and themes should be included in medical informatics curriculum to meet ever-changing learners' needs. In addition, the methodology used for this study has implications in hypothesis generation and knowledge discovery which were demonstrated in many studies [21]. One may analyze, for example, the relationship between chief complaint and disease.
There are technical limitations to our study. Several advantage of using the N-grams as text analysis unit can also be viewed as disadvantage; N-gram may not catch important topics with single word topics (ie, ontology). In our study, however, even the combination of single-word and N-gram topics did not result in desirable product since they hardly bear context. Sooner they loosed the fine-grained spectacles of topics, and the highly pre-coordinated meaning of the topics. We do not regard topics covered in this study as wholly definitive topics of Korean medical informatics in Korea, nor does corpus used in this study encompass all research efforts in Korea. We would simply claim that the corpus represents those of studies and should suffice for our main purpose.
Further research may include comparative analysis of Chinese, Japanese and Korean medical informatics.
Figures and Tables
Figure 2
Top 50 important topics of the Korean medical informatics. The edge values lower than cosine 0.15 in the original network were removed and clustered with component (tf ≥ 5; N = 50; cosine ≥ 0.15; k-component ≥ 1; component = 9). Nine components generate 12 groups. Contour lines were drawn by hand.
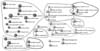
Figure 3
Top 100 important topics of medical informatics in global scale (tf ≥10; N=100; cosine ≥ 0.1; κ-component ≥1; component= 40). Adapted from [20].
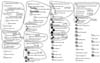
Acknowledgements
This paper is an extended version of our previous work [22]. This work was supported by the Korean Research Foundation Grant funded by the Korean Government (KRF-2008-562-D00035).
References
1. Hasman A, Haux R. Modeling in biomedical informatics: an exploratory analysis part 1. Methods Inf Med. 2006. 45:638–642.


2. Hasman A, Haux R. Modeling in biomedical informatics: an exploratory analysis part 2. Int J Med Inform. 2007. 76:96–102.
3. Maojo V, Kulikowski CA. Bioinformatics and medical informatics: collaboration on the road to genomic medicine? J Am Med Inform Assoc. 2003. 10:515–522.


4. He Q. Knowledge discovery through co-word analysis. Lib Trends. 1999. 48:133–159.
5. Callon M, Courtial JP, Turner WA, Bauin S. From translations to problematic networks: an introduction to coword analysis. Soc Sci Inform. 1983. 22:191–235.


6. Morris TA. Structural relationships within medical informatics. Proc AMIA Symp. 2000. 590–594.
7. Bansard JY, Rebholz-Schuhmann D, Cameron G, Clark D, van Mulligen E, Beltrame E, Barbolla E, Martin-Sanchez Fdel H, Milanesi L, Tollis I, van der Lei J, Coatrieux JL. Medical informatics and bioinformatics: a bibliometric study. IEEE Trans Inf Technol Biomed. 2007. 11:237–243.


8. Mane KK, Börner K. Mapping topics and topic bursts in PNAS. Proc Natl Acad Sci U S A. 2004. 101:Suppl 1. 5287–5290.


9. Garfield E. Mapping the world of biomedical engineering: Alza lecture (1985). Ann Biomed Eng. 1986. 14:97–108.


10. Pickens J, MacFarlane A. Term context models for information retrieval. Proceedings of 15th ACM International Conference on Information and Knowledge Management. 2006. Nov 5-11; Arlington, VA. 559–560.


11. Rebholz-Schuhman D, Cameron G, Clark D, van Mulligen E, Coatrieux JL, Del Hoyo Barbolla E, Martin-Sanchez F, Milanesi L, Porro I, Beltrame F, Tollis I, Van der Lei J. SYMBIOmatics: synergies in medical informatics and bioinformatics - exploring current scientific literature for emerging topics. BMC Bioinformatics. 2007. 8:Suppl 1. S18.


12. Stegmann J, Grohmann G. Hypothesis generation guided by co-word clustering. Scientometrics. 2003. 56:111–135.
14. Swanson DR. Fish oil, Raynaud's syndrome, and undiscovered public knowledge. Perspect Biol Med. 1986. 30:7–18.


15. Stegmann J, Grohmann G. Transitive text mining for information extraction and hypothesis generation [Internet]. 2005. cited 2008 Jul 10. Available from: http://arxiv.org/abs/cs/0509020.
16. Swanson DR. Migraine and magnesium: eleven neglected connections. Perspect Biol Med. 1988. 31:526–557.


17. Mann GS, Mimno D, McCallum A. Bibliometric impact measures leveraging topic analysis. Proceedings of the 6th ACM/IEEE-CS Joint Conference on Digital Libraries. 2006. June 11-15; Chapel Hill, NC. 65–74.


18. Noyons E. Bibliometric mapping of science in a policy context. Scientometrics. 2001. 50:83–98.
20. Jeong S, Kim HG. Intellectual structure of biomedical informatics reflected in scholarly events. Scientometrics. Epub 2010 Feb 11. DOI: 10.1007/s11192-010-0166-z.


21. Bekhuis T. Conceptual biology, hypothesis discovery, and text mining: Swanson's legacy. Biomed Digit Libr. 2006. 3:2.


22. Jeong S, Lee SK, Kim HG. Knowledge structure of Korean medical informatics. Proceedings of CJKMI Fall Conference. 2009. Oct 30-31; Daejeon, KR. 49–51.