Abstract
Purpose
Materials and Methods
Results
Figures and Tables
Fig. 1
Flowchart of patient selection. DWI = diffusion-weighted imaging; MR = magnetic resonance; T = tesla; WHO = World Health Organization
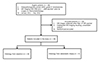
Fig. 2
Flowchart of manual and semiautomatic segmentation based on T2WI and CE-T1WI as structural images. A 70-year-old man with WHO grade IV glioblastoma confirmed via resection, underwent MR imaging with DWI before surgery or chemoradiotherapy. (Top) The axial image of T2-weighted turbo spin echo sequence demonstrated a T2 high signal intensity mass in the left temporal lobe with definite perilesional edema. The mass measured approximately 4.8 cm at its largest diameter. The reformatted axial image of CE-T1WI demonstrates a well-enhanced, solid, and cystic mass with a partially poorly demarcated outer tumor border, as well as a poorly demarcated inner margin of the necrotic portion. Intralesional macrovessels were also noted. (Left column) Coregistrations between structural images (T2WI and CE-T1WI) and the ADC map were performed using the manual segmentation method. Thereafter, the ROI was depicted manually by the reviewers on the axial planes of both T2WI and CE-T1WI. (Right column) Structural images (T2WI and CE-T1WI) and the ADC map were coregistered using the semiautomatic segmentation method. The reviewers manually defined the elliptical VOI, including the entire mass, on structural images. The software automatically segmented the tumor solely within the defined VOI using clustering analysis. Finally, the reviewers depicted appropriate combinations of clusters for tumor segmentation. ADC = apparent diffusion coefficient; CE-T1WI = contrast-enhanced T1-weighted imaging; DWI = diffusion-weighted imaging; MR = magnetic resonance; ROI = region of interest; T2WI = T2-weighted imaging; VOI = volume of interest; WHO = World Health Organization
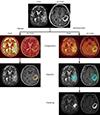
Fig. 3
Bland-Altman plots showing intraobserver reliability between the 1st and 2nd measurements in (a) the manual segmentation method with CE-T1WI for structural imaging; (b) the manual segmentation method with T2WI for structural imaging; (c) the semiautomatic segmentation method with CE-T1WI for structural imaging; and (d) the semiautomatic segmentation method with T2WI for structural imaging. CE-T1WI = contrast-enhanced T1-weighted imaging; T2WI = T2-weighted imaging
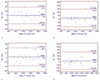
Table 1
Mean ADC Values Obtained by the 2 Radiologists Using Manual and Semiautomatic Segmentation Methods
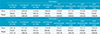
Table 2
Interobserver Reliability in ADC Measurement
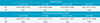
Manual method | ||||
---|---|---|---|---|
1st* CE-T1WI | 1st* T2WI | 2nd* CE-T1WI | 2nd* T2WI | |
ICC† | 0.950 (0.895-0.976) | 0.728 (0.496-0.864) | 0.929 (0.854-0.966) | 0.651 (0.377-0.821) |
CV‡ | 4.25 | 9.30 | 5.11 | 10.59 |
Semiautomatic segmentation method | ||||
---|---|---|---|---|
1st* CE-T1WI | 1st* T2WI | 2nd* CE-T1WI | 2nd* T2WI | |
ICC† | 0.988 (0.975-0.995) | 0.572 (0.264-0.775) | 0.994 (0.987-0.997) | 0.624 (0.338-0.806) |
CV‡ | 2.05 | 13.52 | 1.44 | 11.84 |
All of the numbers in brackets indicate the 95% confidence interval.
ADC = apparent diffusion coefficient; CE-T1WI = contrast-enhanced T1-weighted imaging; CV = coefficient of variance; ICC = intraclass correlation coefficient; T2WI = T2-weighted imaging
*1st and 2nd indicate the first and second measurement, respectively.
†ICC values were categorized as follows: < 0.40, poor agreement; 0.40-0.59, fair agreement; 0.60-0.74, good agreement; and ≥ 0.75, high agreement.
‡Numbers are expressed as percentages.
Table 3
Intraobserver Reliability in ADC Measurement
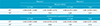
Observer 1 | Observer 2 | |||
---|---|---|---|---|
Manual method | ||||
CE-T1WI | T2WI | CE-T1WI | T2WI | |
ICC* | 0.996 (0.991-0.998) | 0.947 (0.889-0.975) | 0.986 (0.970-0.994) | 0.981 (0.959-0.991) |
CV† | 1.15 | 4.35 | 2.28 | 2.33 |
Semiautomatic segmentation method | ||||
---|---|---|---|---|
CE-T1WI | T2WI | CE-T1WI | T2WI | |
ICC* | 0.995 (0.990-0.998) | 0.826 (0.659-0.916) | 0.992 (0.982-0.996) | 0.968 (0.931-0.985) |
CV† | 1.27 | 9.36 | 1.70 | 3.14 |
All of the numbers in brackets indicate the 95% confidence interval.
ADC = apparent diffusion coefficient; CV = coefficient of variance; CE-T1WI = contrast-enhanced T1-weighted imaging; ICC = intraclass correlation coefficient; T2WI = T2-weighted imaging
*ICC values were categorized as follows: < 0.40, poor agreement; 0.40-0.59, fair agreement; 0.60-0.74, good agreement; and ≥ 0.75, high agreement.
†Numbers are expressed as percentages.