This article has been
cited by other articles in ScienceCentral.
Abstract
PURPOSE
Accurate information is essential in dentistry. The image information of missing teeth is used in optically based medical equipment in prosthodontic treatment. To evaluate oral scanners, the standardized model was examined from cases of image recognition errors of linear discriminant analysis (LDA), and a model that combines the variables with reference to ISO 12836:2015 was designed.
MATERIALS AND METHODS
The basic model was fabricated by applying 4 factors to the tooth profile (chamfer, groove, curve, and square) and the bottom surface. Photo-type and video-type scanners were used to analyze 3D images after image capture. The scans were performed several times according to the prescribed sequence to distinguish the model from the one that did not form, and the results confirmed it to be the best.
RESULTS
In the case of the initial basic model, a 3D shape could not be obtained by scanning even if several shots were taken. Subsequently, the recognition rate of the image was improved with every variable factor, and the difference depends on the tooth profile and the pattern of the floor surface.
CONCLUSION
Based on the recognition error of the LDA, the recognition rate decreases when the model has a similar pattern. Therefore, to obtain the accurate 3D data, the difference of each class needs to be provided when developing a standardized model.
Keywords: 3D scanner, Linear discriminant analysis (LDA), Standardization model, Image recognition
INTRODUCTION
Various needs for the digitalization of teeth have come to the fore in the fields of dental medicine, including the production of tooth implants, the production of braces, and identification. Optical-based medical devices form a new paradigm of digitization and have firm positions as the next-generation medical devices. Digital information of the teeth allows even non-experts to produce dental impression easily, facilitating tooth defect recovery and performing a dental prosthesis treatment in one day. Without the need for a separate impression process, an intraoral scan can shorten the patients' chairtime and, at the same time, allow the design of a tooth implant in realtime and provide integrated solutions for dental care through computer aided engineering (CAE).
12 The performance of a 3D scanner, which is the basis of these solutions, has direct impact on dental care.
A 3D scanner reproduces the shapes of the actual objects based on the recognition method presented on the computer, by producing a database of the positional information and the 3D surface information with pattern and stereo methods. Principal Component Analysis (PCA), Linear Discriminant Analysis (LDA), Independent Component Analysis (ICA), and Probabilistic Decision-Based Neural Networks (PDBNN) are well-known recognition methods. The PCA used in the present study is a statistical method that shows the degree of the dispersion of each data set for the proper direction of the largest vector of the distributed data. Turk and Pentland in 1991 developed an image recognition technique for face recognition, which is a technique of connecting images by representing the pixels of the images as vector values, removing noise-like information using the difference in the intensity of each position, and expressing only the vector values of the principal components as the original data. This technique reacts sensitively to external lighting or changes in the environment, but has been improved gradually as a basic statistical method of image recognition and is applied to several methods.
3 LDA classifies each group through linear deformation, in which the Fisher discriminant becomes the maximum. The recognition is better than the result of a tendency analysis of all data, which does not consider the difference between classes in PDA, because it considers the dispersion of the class as well as the dispersion among the classes. In other words, LDA can collect data from the same class together and has sufficient strength for preserving the information on that class (
Fig. 1).
4 On the other hand, it has a weakness in that it is nonlinear to the data or that it is difficult to separate the same value of each class. Furthermore, in LDA, the dispersion of each class is differentiated to the maximum and the dispersion in the same class is differentiated on the projected surface to the minimum.
5
The LDA techniques presented above are the most widely used methods in face recognition. Therefore, in the case of a dental scanner, the model is photographed continuously. In case of a program that combines images, the order that the photographer takes the model from one place to the next cannot be confirmed. Therefore, if the shape of each section is similar during shooting, the image cannot be reproduced because it is impossible to perform the upper, lower, left, and right gauges. To solve this problem, the model can be reproduced by avoiding the errors of the LDA, given the variable factors. In this study, we designed a model that modified ISO 12836:2015 and applied variable factors to the abutment teeth in order to implement a standardized model cast that can evaluate the accuracy of the scanner for the entire arch. In addition, we want to present the results of the analysis based on the case of image recognition errors of LDA method obtained data after capturing the models.
MATERIALS AND METHODS
This study prepared specimens on dentiform, imitating the shapes of the teeth and mandibular arch. First, a basic model was prepared using Solidworks 2014 (Dassault Systems SolidWorks Corp., MA, USA), designing tooth profiles of No. 1, 3, 5, and 7 of Teeth No. 1 – 7, as shown in
Fig. 2. Based on the designed data, metal model was then fabricated using a CNC device. The metal model was replicated using silicon (Deguform, DeguDent GmbH, Hanau-Wolfgang, Germany) and the model was completed by pouring dental stone (Fujirock, GC Corp, Leuven, Belgium). Two types of equipment were prepared to scan the dental stone model: AEGIS.PO (DDS, Seoul, Korea) and Trios (3 shape, Copenhagen, Denmark). The AEGIS.PO equipment is a method of scanning in a photo type, and the trios is a method of the video type.
With the basic plaster model (general cylinder) in
Fig. 2, four variable factors were selected: ⓐ chamfer cylinder in the form of a cutting surface on the axial surface, ⓑ groove cylinder in the form with a groove and bevel on the occlusal surface, ⓒ curve on the base, and ⓓ square curve. Eight cases were made (
Table 1) and the plaster model was manufactured (
Fig. 3).
To assess the precision of the scanner, this study designed the base, imitating the shape of the mandibular arch and considered shapes, including circle, triangle, square, and curve, as the conditions of the variables of the specimen and produced a model meeting the ISO regulations, judging whether there was initial model recognition. The errors in each image were then analyzed. Images of the dental stone model were collected in the following sequence: ① occlusal, ② buccal, and ③ lingual, as shown in
Fig. 4. The direction of progress was decided in the order of No. 7 to No. 1. Each model was photographed 10 times using a photo scanner and a video scanner. Subsequently, it was judged difficult to derive the result through the quantitative value of the data.
RESULTS
A DDS scanner and 3shape scanner were used for image analysis of each shape;
Fig. 5 and
Fig. 6 present the findings from the basic model and cases. As a result of the scan of the basic model, the occlusal shape was recognized, but the model was not recognized after scanning in the buccal direction, as shown in
Fig. 5.
Fig. 6 presents the experimental findings in the eight cases with variable factors based on the initial model. Each case was scanned 10 times using each intraoral scanner. The number of data collected in a complete shape was 10 in case 8 with AEGIS PO and 10 each in cases 5, 7, and 8 with a 3D shape. The data was not collected in a complete shape for cases other than those mentioned above. Case 1 is a model with a spline curve on the base surface. An analysis of the image for Case 1(a) for the single model recognized the occlusal shape, but for the two figures in Case 1(a) and (b), the model recognition after scanning in the buccal direction was imperfect between the individuals. Case 2 is a model spline square curve on the base surface, and as a result of image analysis, the occlusal shape was consistent with the model in Case 2(a). On the other hand, the direction was lost when the measurements were taken in the buccal and lingual directions and overlapped on the side opposite to the scan direction. In Case 2(b), as in 2(a), the occlusal shape information was similar to the model, but when scanning in the buccal and lingual directions, recognition was not achieved and the 3D shape was incomplete. Case 3 is a model with a chamfered cylinder. As a result of image analysis, as in the figure in Case 3(a), the shape was recognized in the occlusal direction, but the coordinates were not recognized during the buccal and lingual work performance. The Trios scanner images did not recognize more than two occlusal surfaces, as in Case 3(b). Case 4 is a model with a chamfer on No. 1 and 3 and a groove at the top of No. 5 and 7. As a performance result of a DDS scanner, each occlusal shape was embodied as the same as in the model, but when the buccal and lingual images were collected to obtain 3D data, they were not recognized, as in Case 4(a). Case 4(b) showed the same imperfections as Case (a). Case 5 is a model with a chamfer after a spline curve on the base surface, and as a result of image analysis, the occlusal shape was consistent with the model like Case 5(a). On the other hand, as a result of combining the entire image to center on the position where the curve slope became 0, the left shape was opposite to the right shape, but it was incomplete as 3D data. In contrast, in Case 5(b), the shape of the model was consistent with the image file. Case 6 is a model with a chamfer with a spline square curve on the base surface. As a result of image analysis, in Case 6(a), 3D data could be obtained, but polymerization of the image at the edge between the square curve and chamfer was poor. In Case 6(b), the image file in three directions was obtained accurately, but when each image was combined to center around No. 7, the buccal and lingual directions were not differentiated. Case 7 presents a model with a chamfer on No. 1 and 3 and a groove at the top of No. 5 and 7 with a spline curve on the base surface. In Case 7(a), the occlusal image was realized, but in No. 5 and 3 image recognition process, image overlap appeared in the direction opposite to the existing model, centering around No. 5; hence, it could not be used as 3D data. In Case 7(b), the shape of the model was consistent with the image file, which can be used as 3D data. Case 8 is a model with a chamfer on No. 1 and 3 and a groove on top of No. 5 and 7 with spline square curve on the base surface. As a result of image analysis, the 3D data of Cases (a) and (b) were consistent with the model.
DISCUSSION
The digital dental impression technique using an oral scanner has attracted considerable attention as a technology that can solve the weakness of analog impression taking. On the other hand, there are still various difficulties in replacing the traditional way completely, such as the resolution of the scanner, software algorithms, and the miniaturization of equipment. Fortunately, the development of oral scanners is becoming gradually faster with continuous research and development, and many new products are being developed. In this research and development process, an accurate assessment of the many new products available necessitates the development of a standardized evaluation method. For research on the standardization model, this experiment would analyze the interpretation of the non-recognition of an image in each case for the cause of recognition rate decline often occurring in LDA methods.
LDA is a method of recognizing the changes in the elements by separating the class of data, in which the set of data is non-linear and there is a difficulty in separating each class if the mean value of each class is the same. When an analysis is performed with the causes of LDA recognition rate decline through this experiment, a few factors can be found. First, there is a problem of recognition errors in that the section an oral scanner can shoot at once is not large. For a video or photo of each section in the basic model, when the tooth profiles of No. 1 – 7 are the same, the occlusal image is recognized as a circle and a rectangle in the process of combining images. However, later, the buccal and lingual shapes would be recognized as the same shape in the narrow shooting sectors. In other words, in the process of merging images in the LDA method, a set of similar class is formed with the same tooth profile on the left and right and the images are not merged precisely, as shown in
Fig. 7(a). The second factor is that a problem of connection between the tooth profiles occurs. The main reason is that the interpretation is lacking directionality for x1 and x2. As shown in
Fig. 7(b), in the experimental process in the connection to another tooth profile after performing a 3-face scan of one tooth profile, an error occurs between the direction that the user scans and the direction that the computer recognizes. That is, it is difficult for the user to distinguish to which position the measured value changed from the reference point when completing an image of Tooth Profile No. 7 and handling to obtain Image No. 5.
Fig. 7(c) shows the errors combining (a) and (b); the section taken at once is small. Therefore, for a simple tooth profile, it is difficult to recognize the distinction of right and left and top and bottom because of the error in differentiation between the base and tooth profile.
67
This study compared the cases with regard to how images are recognized for the variable factors, including chamfer, groove, curve, and square curve applied to solve the errors in image recognition. First, in the basic model and Case 1, there is a difference in the curve on the base surface. The basic model did not make any connection of images in shooting a buccal surface after capturing an occlusal image. On the other hand, in Case 1, the 3D image could not be connected, but it represented the shape of the mandibular arch compared to the basic model. Similarly, because of the formation of patterns on the base surface, recognition is possible at the front and back of the tooth profile. To compare Cases 1 and 2, regarding the type of pattern of the base surface, the square curve with an edge was better than the shape of the general curve in the acquisition of an image. On the other hand, due to the lack of direction between the tooth profiles, it is difficult for both cases to obtain 3D data. Therefore, the regularity of patterns acted as a variable unfavorable to the scanner's image recognition, and it is judged that it would be necessary to utilize the formation that can produce an irregular pattern or a difference in the opposite direction to the scanning direction when designing a standardization model. Second, to compare Cases 3 with 4, concerning the tooth profile, it is easy to balance centering on the circle rather than the shape of the general cylinder for Tooth Profile No. 7 in both cases because of the effects of the chamfer and groove. Therefore, it appears that one tooth profile can obtain 3D data. On the other hand, when designing a tooth profile, the same shape on both sides may cause an error of an image overlap when selecting a standardization model in the future. Therefore, it will be necessary to implement each shape differently, and it would be necessary to make a column to differ the intensity between the base surface and tooth profile in the buccal and lingual directions. Third, in Cases 5 to 8, the first and second errors were supplemented to obtain similar 3D images. Similarly, applying variable factors to the base and tooth profiles increases the recognition rate of the image, and complex elements should be considered when designing a standardization model.
Currently, the standardization model for an oral scanner is considered before enactment. Therefore, considering the shape of the standardized model, it is expected that the model will be effective in measuring the performance of the oral scanner if the model is selected to avoid the recognition errors of LDA.
CONCLUSION
This study considered a basic model, tooth profiles, and variable factors on the base surface and analyzed each model through the cases of errors in image recognition with LDA. Because the actual model was not recognized in similar sections in scanning with a scanner, it is essential to avoid the formation of the same pattern. Differentiating each class when producing a standardization model is a method that can avoid errors in image recognition. In addition, because the model reported in this paper refers to ISO13836, it will be possible to complete the standardization model of an oral scanner and desktop scanner with a single model.
Figures and Tables
Fig. 1
Comparison of data projection of Principal Component Analysis (PCA) and Linear Discriminant Analysis (LDA).
Fig. 2
Schematic of dentiform and base models: (A) definitive model; (B) gypsum model with tooth number; (C) gypsum model with four variable factors.
Fig. 3
Dentiform specimen geometry of Cases 1 – 8.
Fig. 4
Scanning and recognition direction: ① occlusal; ② buccal; ③ lingual.
Fig. 5
Scanned image results of basic model: (A) DDS scanner AEGIS.PO; (B) 3Shape Trios.
Fig. 6
Scanned image results of specimen: (a) DDS scanner AEGIS.PO; (b) 3Shape Trios.
Fig. 7
Types of LDA recognition errors: (A) duplicate images, (B) position error in one axis, (C) position error on two axes.
Table 1
Specimen shape of test materials
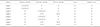
Name |
General cylinder |
Chamfer cylinder |
Groove cylinder |
Curve |
Square curve |
Case 1 |
①,③,⑤,⑦ |
X |
X |
O |
X |
Case 2 |
①,③,⑤,⑦ |
X |
X |
X |
O |
Case 3 |
X |
①,③,⑤,⑦ |
X |
X |
X |
Case 4 |
X |
①,③ |
⑤,⑦ |
X |
X |
Case 5 |
X |
①,③,⑤,⑦ |
X |
O |
X |
Case 6 |
X |
①,③,⑤,⑦ |
X |
X |
O |
Case 7 |
X |
①,③ |
⑤,⑦ |
O |
X |
Case 8 |
X |
①,③ |
⑤,⑦ |
X |
O |