Abstract
Background
This study aimed to investigate the preferences of patients scheduled for carpal tunnel release using conjoint analysis and also introduce an example of how to apply a conjoint analysis to the medical field. The use of conjoint analysis in this study is new to the field of orthopedic surgery.
Methods
A total of 97 patients scheduled for carpal tunnel release completed the survey. The following four attributes were predefined: board certification status, distance from the patient's residency, medical costs, and waiting time for surgery. Two plausible levels for each attribute were assigned. Based on these attributes and levels, 16 scenarios were generated (2 × 2 × 2 × 2). We employed 8 scenarios using a fractional factorial design (orthogonal plan). Preferences for scenarios were then evaluated by ranking: patients were asked to list the 8 scenarios in their order of preference. Outcomes consisted of two results: the average importance of each attribute and the utility score.
Carpal tunnel syndrome (CTS) is the most common type of entrapment neuropathy. According to the Health Insurance Review and Assessment Service Data in Korea, the number of patients who were diagnosed with CTS in 2013 increased by 42% compared with 2009 (from 117,091 to 166,572 individuals) and the total medical expenses for CTS rose by 19% (from $21,956,369 in 2009 to $31,682,541 in 2013). As Korea is a small country with a robust transportation network, the location of a hospital may not be a major consideration when patients choose a hospital. Additionally, the population of Korea is homogeneous and includes few foreigners. Therefore, Korea represents an interesting country to survey factors that affect patient's choice of a hospital for a minor operation, such as carpal tunnel release (CTR). As healthcare market for CTS has recently undergone rapid expansion, studies of patient preferences are warranted and necessary.
Conjoint analysis is a method of identifying preferences that has become popular because it is flexible and easy to understand.1) This type of analysis has been particularly useful in market research and has recently been applied in medical fields. As patients participate in healthcare decision making, it is necessary to know how a patient preference affects their decisions. The use of conjoint analysis in the medical field has been previously reported in plastic surgery and orthodontic services in which patients have a strong role as a consumer.23) Furthermore, it has been used to elicit patient preferences across different racial and/or ethnic groups or to assess patient choices between transport and waiting time for surgery in the UK.456) Our present study of a Korean cohort was the first to assess what patients look for when choosing a hospital for a minor surgery, such as CTR, in a small country in which the population is ethnically homogenous. Additionally, this is the first study to use conjoint analysis in the field of orthopedic surgery. Therefore, it may be interesting to know what patients want when they choose a hospital for CTR, and these findings may be applicable in other similar countries.
A previous study evaluated patient preferences and predictors for satisfaction in CTR.7) However, no study has reported an evaluation of preferences of the patients with CTS when choosing a hospital for CTR. This present study aimed to investigate the preferences of patients scheduled for CTR using conjoint analysis. Moreover, it represents an example of using conjoint analysis in medicine.
After obtaining Asan Medical Center Institutional Review Board approval (No. 20140191), a survey was conducted from January 2014 to May 2014 among 97 patients who were scheduled for CTR at our hospital. Our hospital is located in Seoul and is one of the major hospitals in Korea. Patients younger than 18 years old or those who had a previous carpal tunnel surgery or were unable to complete the questionnaire were excluded. Patients completed the questionnaire in an interviewer's room after visiting the surgeon. Patient characteristics were also assessed as follows: pain score (visual analogue scale [VAS]), income, and living residence.
In this present study, four attributes were predefined through open-ended questions asked to patients and mentioned in a group discussion: board certification status, distance from the patient's residency, medical costs, and waiting time for surgery. Next, two plausible levels for each attribute were assigned (Table 1). Based on these attributes and levels, 16 scenarios were generated (2 × 2 × 2 × 2). Because all possible scenarios can rarely be included in a survey, 8 scenarios were generated using a fractional factorial design (orthogonal plan; IBM SPSS ver. 22, IBM Co., Armonk, NY, USA).38) This method considers the main effect and can reduce the sample size.9) Preferences for scenarios are usually evaluated by one of the following three methods: ranking, rating, or discrete choices.3) In this present study, ranking-based conjoint analysis was used. With ranking, each patient listed the 8 scenarios in their order of preference.
All scenario data were entered into a database and data analysis was performed using IBM SPSS Syntax Editor ver. 22. The outcome of the analysis was shown as the subfile summary, which indicates overall tendency of the respondents. The outcome consists of two results: average importance of the attribute and utility of the attribute.910) Average importance measures how important each attribute is to the overall preference and this value is reported as a percentage. Utility scores (or part-worths) indicate the relative importance (or worth) of each attribute and a higher score denotes a more valuable attribute.9) Calculations were performed separately for each attribute and the outcomes were averaged. Pearson r and Kendall tau statics were used to provide measures of any correlation between the observed and estimated preferences. A p-value less than 0.05 was considered statistically significant. Furthermore, we calculated the average importance and utility according to the pain score (VAS) of patients. Group A was defined as the mild pain group with a VAS 0–3, while group B was the moderate pain group with a VAS 4–6, and group C was the severe pain group with a VAS 7–10.
The mean patient age was 58.3 years (range, 36 to 83 years), 87 of 97 patients were female, and all patients were of the same ethnicity. Patient occupations included 48 housekeepers, 24 small personal business owners, 7 office workers, 6 farm workers, 2 care givers, 1 hair designer, and 9 unemployed persons. There were 37, 54, and 8 patients with a VAS 0–3, VAS 4–6, and VAS 7–10, respectively. Patient demographics are summarized in Table 2.
The average importance of attributes of the patients is summarized in Table 3. The most important attribute was board certificate, followed by distance from patient's residency to the hospital, waiting time, and costs in order. The outcomes according to the VAS score also showed that the board certificate status was the most important attribute. Interestingly, in group C (high VAS score group), waiting time was the second most important attribute.
The utility estimate is summarized in Table 4. Generally, patients showed a greater preference for a hand specialist than a general orthopedic surgeon. Patients in group C reported the lowest score on “more than 4 weeks.” Pearson r and Kendall tau were 0.965 and 0.873, respectively, and both parameters were statistically significant.
The findings of our present study revealed that patients considered the expertise of the doctor as the most important factor for choosing a hospital for CTR. Therefore, patients in Korea prefer to undergo a minor surgery, such as CTR, at a hospital with a hand specialist, even though their residency may be far from the hospital or they may have to wait for more than 4 weeks to undergo surgery. Most patients prefer a well-trained surgeon who is highly recommended by a trusted source who is certified by the board of hand surgery. This is supported by our observation that patients increasingly seek safety without complications as the population becomes more interested in medical malpractice.
The second most important attribute was the overall distance from a patient's home to the hospital; however, waiting time was the second most important attribute in the severe pain group. Additionally, patients in group C reported the lowest utility score on “more than 4 weeks“ and this was predicted to be statistically significant. This indicates that CTS patients with severe pain are less willing to go to a hospital where there is a long waiting time for surgery.
The least important attribute in this present study was cost. Korea has a National Health Insurance (NHI) system, which is compulsory and mandated by law. Payment is made by an individual's employer, unless a person is self-employed, in which case the individual pays for it themselves. Hence, because most patients are covered by insurance, medical costs are not an obstacle to minor surgery. This finding is considered to reflect the situation in our country.
In conjoint analysis, patients must consider trade-offs between attributes and provide information about how they value each scenario. Therefore, this method is more flexible than a simple ranking of attributes.11) Because there are many options to consider for medical decisions, conjoint analysis can be useful to identify patient preferences for use by health care services. However, few previous reports of conjoint analysis exist in the medical field, and it was hard to choose which method was good for establishing preferences among ranking, rating, or discrete choices. We asked the patients to rank the scenarios because we thought it was reasonable for hospital setting.
It is difficult to determine sample size in a conjoint analysis because a conjoint analysis estimates individual worth and there have been few references about determination of the sample size. One paper reported that a minimum of 200–300 were necessary for a general survey and 100 for a small market.12) Another paper reported that a recommended sample size can be based on similar studies.13) We thought that the appropriate sample size of this study was about 100 cases and three cases were excluded because they did not complete the survey. Therefore, total 97 cases were included in this study.
There were several limitations to this study. First, because we enrolled patients from a tertiary care university hospital, this study has a selection bias. Therefore, the outcomes of this study may not represent the general population. If we had enrolled patients scheduled for CTR at a primary or secondary care facility, they could have responded to the survey differently. Second, the scenarios used for our analyses were hypothetical only. Indeed, different levels may provide different results. Thus, we preinterviewed our patients and discussed this factor in sufficient detail to reduce this limitation. Third, as in previous studies, our survey was conducted in a paper-and-pencil format. In this format, patients can become easily fatigued and end the survey without full consideration of all questions. We think that development of a simpler format may be necessary. Finally, the sample size is small.
Figures and Tables
Table 1
Attributes and Levels
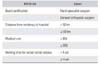
Table 2
Demographics
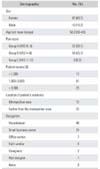
Table 3
Average Importance of the Attributes
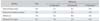
Table 4
Utility Estimates of the Attributes
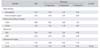
ACKNOWLEDGEMENTS
This survey was conducted under the leadership of Professor Jin Sam Kim who has passed away in early 2016. Completion of this study could not have been possible without his pioneering venture into applying marketing research methods to the clinical orthopedic field. His contributions are gratefully acknowledged.
References
1. Curry J. Understanding conjoint analysis in 15 minutes. Sequim, WA: Sawtooth Software;1996.
2. Waltzman JT, Scholz T, Evans GR. What patients look for when choosing a plastic surgeon: an assessment of patient preference by conjoint analysis. Ann Plast Surg. 2011; 66(6):643–647.
3. Ryan M, Farrar S. Using conjoint analysis to elicit preferences for health care. BMJ. 2000; 320(7248):1530–1533.


4. Ryan M, McIntosh E, Dean T, Old P. Trade-offs between location and waiting times in the provision of health care: the case of elective surgery on the Isle of Wight. J Public Health Med. 2000; 22(2):202–210.


5. Silverman S, Calderon A, Kaw K, et al. Patient weighting of osteoporosis medication attributes across racial and ethnic groups: a study of osteoporosis medication preferences using conjoint analysis. Osteoporos Int. 2013; 24(7):2067–2077.


6. Byrne MM, Souchek J, Richardson M, Suarez-Almazor M. Racial/ethnic differences in preferences for total knee replacement surgery. J Clin Epidemiol. 2006; 59(10):1078–1086.


7. Shifflett GD, Dy CJ, Daluiski A. Carpal tunnel surgery: patient preferences and predictors for satisfaction. Patient Prefer Adherence. 2012; 6:685–689.
8. Bradley M. User's manual for SPEED version 2.1 stated preference experiment editor and designer. Hague: Hague Consulting Group;1991.
9. Koo LC, Tao FK, Yeung JH. Preferential segmentation of restaurant attributes through conjoint analysis. Int J Contemp Hosp Manag. 1999; 11(5):242–253.


10. Levy DS. Modern marketing research techniques and the property professional. Prop Manag. 1995; 13(3):33–40.


11. Pignone MP, Brenner AT, Hawley S, et al. Conjoint analysis versus rating and ranking for values elicitation and clarification in colorectal cancer screening. J Gen Intern Med. 2012; 27(1):45–50.


12. Vilikus O. Optimization of sample size and number of tasks per respondent in conjoint studies using simulated datasets. Stud Ekon Uniw Ekon Katowicach. 2012; (120):77–83.
13. Tang J, Vandale W, Weiner J. Sample planning for CBC models: our experience. In : Proceedings of the Sawtooth Software Conference; 2006 Mar 29-31; Delray Beach, FL. Sequim, WA: Sawtooth Software;2016. p. 111–116.