Abstract
Background and Purpose
The claims data of the Korean National Health Insurance (NHI) system can be useful in stroke research. The aim of this study was to validate the accuracy of hospital discharge data used for NHI claims in identifying acute stroke and use of thrombolytic therapy.
Methods
The hospital discharge data of 1,811 patients with stroke-related diagnosis codes were obtained from Jeju National University Hospital (JNUH) and Seoul Medical Center (SMC). Three algorithms were tested to identify discharges with acute stroke [ischemic stroke (IS), intracranial hemorrhage (ICH), or subarachnoid hemorrhage (SAH)]: 1) all diagnosis codes up to nine positions, 2) one primary diagnosis and one secondary diagnosis, and 3) only one primary diagnosis code. Reviews of medical records were considered the gold standards.
Results
Overall, the degree of agreement (κ) was higher for algorithms 1 and 2 than for algorithm 3, and the sensitivity and specificity of the first two algorithms for IS and SAH were both >90%, with almost perfect agreement (κ=0.83-0.84) in the JNUH data set. Regarding ICH, only algorithm 1 yielded an almost perfect agreement (κ=0.82). In the SMC data set, almost perfect agreement was found for both ICH and SAH in all three algorithms. In contrast, the three algorithms yielded a range of agreement levels, though all substantial, for IS. Almost perfect agreement was obtained for use of thrombolytic therapy in both data sets (κ=0.91-0.99).
Administrative health information, such as hospital discharge data, is acquired routinely in the health service and is often used for health insurance claims. These data are beneficial to clinical research since they are readily available and inexpensive to acquire, and can frequently be obtained on a large scale.1 Administrative data have been used for research into the incidence, prevalence, and mortality of stroke, to explore the utilization trends of thrombolytic therapies for stroke, and to estimate the cost associated with stroke care.2345678 Since disease or procedure codes within the administrative data have been frequently used to identify the stroke or the use of thrombolytic therapy in those research, validations of such codes or algorithms used to identify the disease or the procedure are indispensable for stroke research using administrative data. The accuracy of International Classification of Diseases, 9th Revision, Clinical Modification (ICD-9 CM) codes for identifying acute stroke has improved with the introduction of modifier codes and the application of specific algorithms according to the type of stroke.19 Nonetheless, the accuracy of administrative data pertaining to the identification of stroke diagnosis and treatment are often unsatisfactory. 310
Nationwide epidemiological data such as stroke incidence or death rate are mandatory and necessary not only for evaluating the socioeconomic cost of stroke care, but also for establishing health and medical policies for stroke treatment and prevention. Unfortunately, there are no in-depth nationwide epidemiological data for stroke in Korea, despite stroke being the second cause of death, with an estimated annual incidence of more than 100,000.11 Korea has had a National Health Insurance (NHI) system that covers the entire population as a compulsory social insurance system since 1989. The Korean NHI claims data can be useful in stroke research since they can allow estimation of the national trends regarding the incidence of stroke, as well as use of important stroke treatments such as thrombolytic therapies. Although several previous studies have estimated the nationwide prevalence, incidence, and cost of stroke using stroke-related diagnosis codes from the Korean NHI claims data, the accuracy of stroke diagnosis using those codes has not yet been validated. 1213141516 Therefore, the aim of this study was to validate the accuracy of hospital discharge data used for NHI claims in identifying acute stroke and use of thrombolytic therapy.
The sixth edition of the Korean Standard Classification of Diseases (KCD), which is based on the tenth revision of the ICD,17 was used to verify hospital discharges with acute stroke, and the validating methods and algorithms reported by Tirschwell and Longstreth1 were employed. The data source was hospital discharge data from Jeju National University Hospital (JNUH), which is the only university hospital on Jeju island, which is located off the southern coast of the Korean peninsula and in 2015 had population of 550,000. JNUH was designated as 1 of 12 regional stroke centers by Korean government in 2009, and has an annual case volume of acute stroke admissions of approximately 250-300. The accuracy of the results was tested in a different clinical setting, using the hospital discharge data from Seoul Medical Center (SMC). SMC, as a public hospital for Seoul citizens with a low socioeconomic status, is a teaching community hospital managed by Seoul metropolitan government and located in the northeast part of Seoul. Annual stroke volume of SMC is comparable with that of JNUH.
This study was approved by the Institutional Review Board of JNUH.
Between January 2012 and December 2013, hospital discharges with the following criteria were identified: 1) patient age ≥18 years, 2) stroke-related diagnosis codes in any diagnosis position [G46 (cerebrovascular disease), I60 (subarachnoid hemorrhage, SAH), I61-2 (intracranial hemorrhage, ICH), I63 (cerebral infarction), and I64 (cerebrovascular disease, not otherwise specified)], and 3) first admission. The following information were obtained from the hospital discharge data: 1) demographic characteristics (age, sex, and discharge date), 2) discharge diagnosis code up to nine positions, 3) department at the time of discharge, 4) drug code for recombinant tissue plasminogen activator (rtPA), and 5) procedure codes indicating intra-arterial thrombolytic therapy (IAT).
Discharge with ischemic stroke (IS), ICH (including non-traumatic subdural hematoma), and SAH were identified with KCD codes I63, I61-2, and I60, respectively. Use of intravenous thrombolysis (IVT) was identified with the drug code for rtPA since there is no procedure code for IVT in the Korean NHI claims data. IAT was identified using the procedure codes for percutaneous thrombolysis for the intracranial artery, percutaneous thrombolysis for other artery, and mechanical or aspiration thrombectomy (M6631, M6632, and M6633, respectively). The following European Cooperative Acute Stroke Study III criteria were used to detect symptomatic intracranial hemorrhage (SICH) following thrombolytic therapy: any apparently extravascular blood in the brain or within the cranium associated with a worsening of the National Institutes of Health Stroke Scale score of ≥4 or leading to death.18
Reviews of medical records and brain imaging or prospective stroke registry were considered the gold standards. In this study, stroke was defined as an acute neurological deficit caused by focal cerebral ischemia, brain parenchymal hemorrhage, or bleeding into the subarachnoid space.19 Admissions with acute stroke were identified by limiting admissions to within 4 weeks of symptom onset. Three algorithms for stroke diagnoses were tested: 1) all available diagnoses up to nine positions, 2) one primary diagnosis and one secondary diagnosis, and 3) only one primary diagnosis. The sensitivity, specificity, positive predictive value (PPV), and agreement were calculated for each algorithm using a 2×2 table [hospital discharge data diagnosis (yes/no) versus gold standard diagnosis (yes/no)]. Finally, overall agreement was computed using all stroke subtypes (4×4 table) together for a given algorithm. The accuracies among different hospital departments were examined by performing the same analysis by discharge department. The degree of agreement, κ, was defined as substantial (0.61≤κ≤0.80) or almost perfect (0.81≤κ≤1.00).20
Among 22,799 discharges during the period from January 2012 to December 2013, 975 unique discharges (4.3%) had a stroke-related diagnosis at JNUH. After applying the same inclusion criteria, 836 unique discharge data were obtained at SMC. The patients were aged 66±14 years (mean±standard deviation) and 56% were men. Overall, 1,233 of all patients (68.1%) were admitted via the emergency department. At the time of discharge, most of the patients had been treated at the Departments of Neurology, Neurosurgery, Rehabilitation Medicine, or Internal Medicine. Among them, 1,352 hospital discharges (74.7%) were confirmed as acute stroke by chart or registry review. The final stroke diagnosis was IS in 968 discharges (71.6%), ICH in 271 discharges (20.0%), and SAH in 113 discharges (8.4%). Among 968 discharges confirmed as IS, IVT alone was used in 84 discharges (8.7%), and either IAT or combined IVT and IAT was performed in 28 patients (2.9%) (Table 1).
Among three algorithms for stroke diagnosis, the overall κ for the JNUH data set was higher for algorithms 1 and 2 than for algorithm 3, and for IS and SAH, algorithms 1 and 2 both had a sensitivity and specificity of approximately >90%, with almost perfect agreement (κ=0.83-0.84). For ICH, only algorithm 1 had almost perfect agreement (κ=0.82). Overall, the other two algorithms still exhibited a substantial level of agreement (κ=0.69-0.76). In the SMC data set, there was no significant difference in agreement among the three algorithms overall. Regarding types of stroke, both ICH and SAH exhibited almost perfect agreement for all three algorithms (κ=0.86-0.92). In contrast, IS had a lower range of agreement for all three algorithms compared with hemorrhagic stroke (κ=0.64-0.66) (Table 2). The validities of the three algorithms for stroke diagnosis according to discharge department are given in Supplementary Table 1,2,3,4 (in the online-only Data Supplement): the overall agreement was 0.89-0.91 for the Department of Neurology, 0.87-0.89 for the Department of Neurosurgery, 0.42-0.82 for the Department of Rehabilitation Medicine, and 0.50-0.57 for all other departments including the Department of Internal Medicine. Among 1,352 patients with a final diagnosis of stroke, 73 patients (5.4%) developed stroke during their admission to the hospitals (67 patients with IS, 5 patients with ICH, and 1 patient with SAH); 71 of 114 patients (62.3%) admitted to hospital departments other than that of neurology or neurosurgery experienced an in-hospital stroke. Examination of the validity of hospital discharge codes for the identification of in-hospital IS revealed unsatisfactory overall sensitivity and agreement because the diagnosis codes were frequently absent or were not present at the primary or secondary position (Supplementary Table 5 in the online-only Data Supplement).
The final review revealed only four patients in the SMC data set who underwent IAT during the study period, and so it was not possible to calculate the accuracy of the codes indicating IAT. IVT exhibited almost perfect agreement in both data sets (κ=0.91-0.99), and IAT exhibited a very high agreement in the JNUH dataset (κ=0.91) (Table 3). Among 112 patients who received thrombolytic therapy, 9 (8.0%) had SICH following the treatment: SICH developed in 7 of these patients following IVT and in 2 following IAT or combined treatment. SAH did not occur following the thrombolytic therapy. Among the nine patients with SICH following the treatment, three patients had an I61 code in the secondary diagnosis position, and one patient had an I61 code in the first position. According to the method, patients with both IS and ICH codes were classified as ICH in algorithms 1 and 2. The remaining patients did not have ICH discharge codes in the either primary or secondary diagnosis positions.
This study has demonstrated how stroke-related KCD codes can be accurately applied to confirm discharges with acute stroke that occurred within 4 weeks of symptom onset. As expected, the algorithm using all discharge diagnosis codes (algorithm 1) had the greatest sensitivity and agreement rate, while the algorithm using only the primary diagnosis (algorithm 3) had the greatest specificity. This finding was true for all stroke types. With IS in particular, the specificity decreased to 68% when all discharge diagnosis codes were used. However, the PPV of the diagnosis for IS was 82%, which is similar to that described in a recent systematic review.21 As reported previously,122 the highest PPV was observed when only the primary diagnosis code (algorithm 3) was used to identify stroke discharges in all types of stroke.
A distinctive finding of this study was that the accuracies of the discharge codes for IS differed between the two hospitals, despite the similar numbers of stroke admissions annually. The overall agreement rates of stroke with algorithm 1 also differed between the two data sets (0.81 in JNUH and 0.65 in SMC), a finding that is largely attributable to the difference in the agreement rate for IS. Differences in coding accuracy by regional location of the hospital, teaching status,23 department at discharge,22 and demographic features of the patients24 have been reported previously. Stroke patients admitted to the SMC were older and more likely to be male, and were less likely to be discharged from department of rehabilitation medicine compared to those patients admitted to JNUH. In particular, the reimbursement for stroke service differed between the two hospitals, with a prospective payment system using diagnosis-related group (DRG) codes being applied at SMC, whereas a "fee for service" system is used for reimbursement at JNUH. The higher false-positive rate for IS in the SMC data set compared to the JNUH might be attributable to the use of the DRG-based reimbursement system, since this is reportedly associated with the assignment of discharge diagnostic codes in a way that would increase reimbursement.25
An alternative explanation for a relatively low accuracy of disease codes for IS in the SMC data set may be the inclusions of older patients, with the associated likelihood of a higher rate of comorbidities, given the higher discharge rate from the Department of Internal Medicine among SMC patients. Furthermore, it is not clear with the I63 code whether the IS is acute or chronic; thus, there were some cases in which the I63 code was used for IS that had developed more than 4 weeks after symptom onset or just with the previous history of stroke. In contrast to IS, differences in hospital characteristics, including the reimbursement system, did not seem to affect the accuracy of disease codes for either ICH or SAH. The higher accuracy of codes for hemorrhagic stroke may be attributable to a more robust association between clinical symptoms and signs with image findings in that indication than for IS. In the JNUH dataset, algorithm 3 had a lower level of agreement compared with the other two algorithms. This may be explained by stroke diseases codes such as I60-163 not being used as the primary diagnosis codes for acute stroke patients who transferred to the Department of Rehabilitation Medicine. Alternatively, that department is known to use symptom- or disability-related code such as hemiparesis (G81), hemiplegia (G82), and aphasia or dysarthria (R47).
The use of thrombolytic therapy for IS could be accurately identified using the procedure codes in this study. Both the sensitivity and specificity of the procedure code for IVT were >90% for both centers, and were higher than the sensitivity of 82% observed in a previous study that used ICD-9 codes and the same procedure codes.1 This indicates that the nationwide trend of using thrombolytic therapy can be evaluated by using claims data alone in Korea, since the entire Korean population is covered by a single NHI system.
The results presented here suggest that the claims data from hospitals in Korea could be a useful source for assessing stroke epidemiology and the use of thrombolytic therapy. However, the findings should be considered in the light of some limitations. First, since chart analysis was not possible for all of the patients included in this study, instead being limited to those with a high probability of discharge codes for stroke, and patients with a low probability were eliminated from the medical record review. This could have resulted in the sensitivity values being overestimated and the specificity values being underestimated. Second, the accuracy of the discharge codes for acute stroke varied between the two data sets from the two hospitals. Thus, the validity of the algorithms should be examined in other hospitals with different clinical settings, or using other population data. However, the accuracies of discharge codes for hemorrhagic stroke and procedure codes for thrombolytic therapies were similar in the two hospitals, and were sufficiently high to confirm the true cases. The methods that were used to identify hemorrhagic stroke and the use of thrombolytic therapy in this study may be applicable to the Korean NHI claims data from other hospitals.
This study identified discharges with hemorrhagic stroke and the use of thrombolytic therapy with high reliability from administrative discharge data. For IS, although the agreements varied with different algorithms and datasets, there was still a substantial level of agreement. These findings suggest that the Korean NHI claims data are useful for obtaining nationwide epidemiological data on stroke. In addition to efforts to educate clinicians to apply correct KCD codes, future studies should focus on refining the algorithm to identify IS using discharge data from various hospitals, since the clinical setting, socioeconomic status of stroke patients, treating department, and medical reimbursement system could influence the accuracy of the diagnosis of this disorder.
Figures and Tables
Table 1
Characteristics of hospital discharge data with stroke-related diagnoses
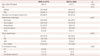
Table 2
Sensitivity, specificity, positive predictive value (PPV) and level of agreement (κ) for stroke diagnosis
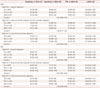
Table 3
Sensitivity, specificity, PPV, and κ for thrombolytic therapy

References
1. Tirschwell DL, Longstreth WT Jr. Validating administrative data in stroke research. Stroke. 2002; 33:2465–2470.


2. Ellekjaer H, Holmen J, Indredavik B, Terent A. Epidemiology of stroke in Innherred, Norway, 1994 to 1996. Incidence and 30-day case-fatality rate. Stroke. 1997; 28:2180–2184.
3. Leibson CL, Naessens JM, Brown RD, Whisnant JP. Accuracy of hospital discharge abstracts for identifying stroke. Stroke. 1994; 25:2348–2355.


4. Taylor TN, Davis PH, Torner JC, Holmes J, Meyer JW, Jacobson MF. Lifetime cost of stroke in the United States. Stroke. 1996; 27:1459–1466.


5. Qureshi AI, Suri MF, Nasar A, He W, Kirmani JF, Divani AA, et al. Thrombolysis for ischemic stroke in the United States: data from National Hospital Discharge Survey 1999-2001. Neurosurgery. 2005; 57:647–654. discussion 647-654


6. Qureshi AI, Chaudhry SA, Majidi S, Grigoryan M, Rodriguez GJ, Suri MF. Population-based estimates of neuroendovascular procedures: results of a state-wide study. Neuroepidemiology. 2012; 39:125–130.


7. Kleindorfer D, Lindsell CJ, Brass L, Koroshetz W, Broderick JP. National US estimates of recombinant tissue plasminogen activator use: ICD-9 codes substantially underestimate. Stroke. 2008; 39:924–928.


8. Cheng CL, Kao YH, Lin SJ, Lee CH, Lai ML. Validation of the National Health Insurance Research Database with ischemic stroke cases in Taiwan. Pharmacoepidemiol Drug Saf. 2011; 20:236–242.


9. Goldstein LB. Accuracy of ICD-9-CM coding for the identification of patients with acute ischemic stroke: effect of modifier codes. Stroke. 1998; 29:1602–1604.


10. Qureshi AI, Harris-Lane P, Siddiqi F, Kirmani JF. International classification of diseases and current procedural terminology codes underestimated thrombolytic use for ischemic stroke. J Clin Epidemiol. 2006; 59:856–858.


11. Hong KS, Bang OY, Kang DW, Yu KH, Bae HJ, Lee JS, et al. Stroke statistics in Korea: part I. Epidemiology and risk factors: a report from the korean stroke society and clinical research center for stroke. J Stroke. 2013; 15:2–20.


12. Kim RB, Kim BG, Kim YM, Seo JW, Lim YS, Kim HS, et al. Trends in the incidence of hospitalized acute myocardial infarction and stroke in Korea, 2006-2010. J Korean Med Sci. 2013; 28:16–24.


13. Lim SJ, Kim HJ, Nam CM, Chang HS, Jang YH, Kim S, et al. [Socioeconomic costs of stroke in Korea: estimated from the Korea national health insurance claims database]. J Prev Med Public Health. 2009; 42:251–260.


14. Koo BK, Lee CH, Yang BR, Hwang SS, Choi NK. The incidence and prevalence of diabetes mellitus and related atherosclerotic complications in Korea: a National Health Insurance Database Study. PLoS One. 2014; 9:e110650.


15. Kang HY, Lim SJ, Suh HS, Liew D. Estimating the lifetime economic burden of stroke according to the age of onset in South Korea: a cost of illness study. BMC Public Health. 2011; 11:646.


16. Ebrahim S, Sung J, Song YM, Ferrer RL, Lawlor DA, Davey Smith G. Serum cholesterol, haemorrhagic stroke, ischaemic stroke, and myocardial infarction: Korean national health system prospective cohort study. BMJ. 2006; 333:22.


17. Statistics Korea. Korean standard classification of diseases and causes of death [Internet]. Daejeon: Statistics Korea;2014. [cited 2014 Dec 28]. Available from: kssc.kostat.go.kr/ksscNew_web/index.jsp#.
18. Hacke W, Kaste M, Bluhmki E, Brozman M, Dávalos A, Guidetti D, et al. Thrombolysis with alteplase 3 to 4.5 hours after acute ischemic stroke. N Engl J Med. 2008; 359:1317–1329.


19. Sacco RL, Kasner SE, Broderick JP, Caplan LR, Connors JJ, Culebras A, et al. An updated definition of stroke for the 21st century: a statement for healthcare professionals from the American Heart Association/ American Stroke Association. Stroke. 2013; 44:2064–2089.


20. Lyden PD, Lau GT. A critical appraisal of stroke evaluation and rating scales. Stroke. 1991; 22:1345–1352.


21. Andrade SE, Harrold LR, Tjia J, Cutrona SL, Saczynski JS, Dodd KS, et al. A systematic review of validated methods for identifying cerebrovascular accident or transient ischemic attack using administrative data. Pharmacoepidemiol Drug Saf. 2012; 21:Suppl 1. 100–128.


22. Spolaore P, Brocco S, Fedeli U, Visentin C, Schievano E, Avossa F, et al. Measuring accuracy of discharge diagnoses for a region-wide surveillance of hospitalized strokes. Stroke. 2005; 36:1031–1034.


23. Jones SA, Gottesman RF, Shahar E, Wruck L, Rosamond WD. Validity of hospital discharge diagnosis codes for stroke: the Atherosclerosis Risk in Communities Study. Stroke. 2014; 45:3219–3225.


Supplementary Materials
The online-only Data Supplement is available with this article at http://dx.doi.org/10.3988/jcn.2016.12.1.42.
Supplementary Table 1
Validity of stroke codes using all diagnoses (algorithm 1) according to discharge department
Supplementary Table 2
Validity of stroke codes using one principal and one secondary diagnosis (algorithm 2) according to discharge department