Abstract
Background and Purpose
The purpose of this study was to estimate the incidence and prevalence of epilepsy among an elderly and poor population in the United States.
Methods
Arizona Medicaid claims data from January 1, 2008 to December 31, 2010 were used for this analysis. Subjects who were aged ≥65 years and were continuously enrolled in any Arizona Medicaid health plans (eligible to patients with low income) for ≥12 months between January 1, 2008 and December 31, 2009 were considered eligible for inclusion in the study cohort. In addition to meeting the aforementioned criteria, incident and prevalent cases must have had epilepsy-related healthcare claims. Furthermore, incident cases were required to have a 1-year "clean" period immediately preceding the index date. Negative binomial and logistic regression models were used to assess the factors associated with epilepsy incidence and prevalence.
Results
The estimated epilepsy incidence and prevalence for this population in 2009 were 7.9 and 19.3 per 1,000 person-years, respectively. The incidence and prevalence rates were significantly higher for patients with comorbid conditions that were potential risk factors for epilepsy and were of younger age than for their non-comorbid and older counterparts (p<0.05). The prevalence rates were significantly higher for non-Hispanic Blacks and male beneficiaries than for non-Hispanic Whites and female beneficiaries, respectively (p<0.05).
Epilepsy involving at least two unprovoked seizure episodes1 is the third most prevalent neurological condition affecting the elderly, after stroke and dementia-related diseases.2 The age-adjusted prevalence in the general population has been estimated to range between 2.2 and 41.0 per 1,000 persons annually,3456789 while the age-adjusted incidence is between 16 and 51 per 100,000 persons.101112 However, the annual incidence rate surpasses 0.1%, and increases with age in the elderly population,13 while the cumulative incidence up to 80 years of age lies in the range 1.3-4.0%.14 The epilepsy incidence has been steadily increasing at an average annual rate of 3.5% in the elderly population over the past 2 decades,15 while the incidence rates in the pediatric and nonelderly adult population have been decreasing.
The elderly population is also prone to multiple comorbid conditions relevant to the etiology of epilepsy, such as stroke, cerebrovascular diseases, central nervous system tumors, and neurodegenerative diseases.12 It has been estimated that 60% of incident epilepsy cases in the elderly population for which the etiology was identified were associated with cerebrovascular diseases, whereas approximately 20% were related to neurodegenerative diseases.12 It is important to note that the risk of developing epilepsy is six- to eightfold higher in patients with Alzheimer's disease and other types of dementia than in their non-Alzheimer's counterparts.16
There is abundant evidence for an inverse association between socioeconomic status (SES) and epilepsy prevalence or incidence. Noronha et al.17 reported a higher epilepsy prevalence among lower-SES patients after adjusting for therapeutic gaps among socioeconomic groups. Birbeck et al.18 found that patients with epilepsy had a lower income level and poorer housing and occupation conditions compared with an age-matched cohort. After controlling for risk factors, such as whether the patients had stroke or head injury, one case-control study found that low education level and lack of home ownership were correlated with epilepsy.19 A Swedish study observed that the risk of epilepsy was higher among those with low-salary occupations (e.g., waiter or construction worker) than those with white-collar occupations.20
In the United States (US), multiple national health insurance programs are available to eligible populations, including-but not limited to-the federally funded Medicare and the joint state and federal-funded Medicaid. In general, Medicare is for people aged 65 years or older; among those who are younger than 65 years old, beneficiaries have accompanying specific disabilities or have end-stage renal disease. The primary requirement for eligibility is having paid a specified minimum in Medicare taxes while working. Medicaid is a US federal-state jointly funded public health insurance program that is administered by the individual states.
Beneficiaries receiving both Medicare and Medicaid tend to be the sickest and poorest individuals covered by either program. Most have very low incomes (≤US$ 10,000/year), and many qualify for a supplemental security income (a US federal program that provides cash assistance to very poor individuals). They are more likely to rate their health as fair or poor, to have mental health problems, and to live in nursing homes; they are also more likely to be disabled. For individuals eligible for Medicare whose incomes are just above the level allowed for Medicaid, Medicaid will pay the premiums and copayments associated with Medicare insurance.
The Arizona Medicaid program, also known as Arizona Health Care Cost Containment System (AHCCCS) was established predominantly to provide low-premium health insurance for the low-income legal residents of the state.21 Approximately 6% of Arizona Medicaid beneficiaries also have Medicare (i.e., they are dually eligible). As with Medicaid programs in other states, the AHCCCS is less restrictive with respect to the types of benefits included (e.g., Medicaid covers medication costs not covered by Medicare). It is Medicaid's policy to cover only the costs of healthcare not covered by Medicare (or other programs that an individual may be eligible for, such as veterans benefits) for persons receiving both Medicaid and Medicare. However, elderly people enrolled in the AHCCCS are unlikely to be able to pay a monthly premium to receive prescription drug benefits offered by Medicare Part D (a Medicare program that specifically provides outpatient prescription drug coverage). AHCCCS programs operate under a managed care structure, and the managed care plans offer seamless coverage to beneficiaries by providing both Medicare and Medicaid benefits. AHCCCS covers all the costs of healthcare for the elderly aged ≥65 years who are not eligible for Medicare (e.g., individuals who worked in the informal economy and did not pay federal income taxes).
As of November 1, 2012, the AHCCCS population included 1.27 million beneficiaries, approximately 6.0% of whom were aged ≥65 years, 65.3% were females, 34.1% were Caucasian/White, and 40.5% were Hispanic. Compared with the AHCCCS population, the 2012 Arizona population comprises a higher proportion of elderly (14.8%) and lower proportions of females (50.3%) and Hispanics (30.2%).22 In 2009, the AHCCCS provided healthcare coverage to approximately 1.3 million Arizonans (i.e., 16% of all Arizonans) at an annual cost of US$ 9.3 billion. AHCCCS consists of a per-member cost-per-month standard (i.e., capitation), which is used as a single financial reference point for comparing the financial performance between states and health plans. The fixed monthly capitation is based upon the age, sex, and Medicare status of each enrollee.
Previous research has assessed the epilepsy epidemiology in the general population, US Medicare patients, and younger Medicaid patients,232425 but there have been no studies focusing on a particularly vulnerable population: the low-income elderly. Thus, the aim of this study was to identify the annual incidence and prevalence of epilepsy in various patient subgroups of the Arizona Medicaid elderly population.
The AHCCCS claims database used for this study included the following file elements from January 1, 2008 to December 31, 2010: demographics, eligibility, claims header, and claims diagnosis. The demographics file included demographic information such as age, gender, race, and marital status for all beneficiaries born prior to January 1, 1944, regardless of whether death or disenrollment occurred. To avoid exposing the identity of very elderly persons, beneficiaries aged >85 years were compiled into an "85+" category. The eligibility file comprised descriptions of start and end dates of coverage for all beneficiaries who had coverage with any AHCCCS health plan. The claims diagnosis file included all diagnoses relevant to a specific claim, and the claims header file included service periods and expenditures of all medical claims (i.e., inpatient, outpatient, and healthcare professional claims; prescription claims were not included). Data from 2008 were used to determine case eligibility and patient comorbidity, but were not included in the prevalence and incidence calculations; data from 2009 and 2010 were used to apply patient eligibility criteria to identify prevalent and incident cases.
The AHCCCS claims database captures all claims relevant to Arizona Medicaid patients. In the US, Medicaid is the "last resort" of healthcare insurance payment that covers the remaining bill after payouts from all other insurance policies that a patient possesses. Relevant claims forms include both Medicaid and Medicare paid amounts, as well as payment from other insurance sources; in other words, payments and claims for patients who have both Medicare and Medicaid are captured, regardless of the amount paid from either source. Medicare-only claims for these patients are also captured in this database.
For the purpose of calculating incidence and prevalence rates, the denominator (i.e., 2009 AHCCCS cohort) required the use of patients from the overall Arizona Medicaid population who were at least 65 years of age as of January 1, 2009 and were continuously enrolled for at least 12 months between January 1, 2008 and December 31, 2009. Patients who had either no or only one gap in AHCCCS enrollment of up to 45 days during any 12-month period were considered continuously enrolled.26 Incident and prevalent cases were a subset of the 2009 cohort. In addition to the aforementioned criteria, a patient must have fulfilled all of the eligibility criteria listed below to be classified as an incident or prevalent case. The inclusion criteria were: 1) one or more healthcare claims (including claims associated with inpatient and outpatient visits) with a diagnosis code of 345.xx (indicating epilepsy), or two or more healthcare claims with a diagnosis code of 780.3x (indicating seizure) that were at least 30 days and no more than 1 year apart;2427 2) enrolled for at least 1 day in the AHCCCS during 2009; and, 3) an index event after December 31, 2008 and prior to January 1, 2010. The exclusion criterion was a recorded date of death prior to January 1, 2009. In addition, incident cases must have had a continuous enrollment of 12 full months prior to the index event (no additional gaps were allowed here so as to validly reflect comorbid conditions), could not have had their first seizure-related healthcare claim with a diagnosis code of 345.x1 (intractable epilepsy),24 and could not have a 12-month period immediately preceding the index event with any healthcare claims related to epilepsy or seizure (in order to be considered a new-onset case). Since eligible beneficiaries were not required to be continuously enrolled throughout 2009, some may have contributed less than one full year of "days at risk" to the denominator of the incidence rate in 2009.
The epilepsy incidence and prevalence estimates were compared across subgroups of gender, race/ethnicity, geographic residence location, marital status, and other epilepsy risk factors (including anoxic brain injury, stroke, atherosclerosis, brain tumor, Alzheimer's disease, Parkinson's disease, dementia, hypertension, and sleep apnea)228 using univariate Poisson regression and chi-square tests for categorical variables and a nonparametric trend test for ordinal variables. For subgroups that included more than two categories, the category with the larger number of subjects or with an extreme attribute (i.e., the lowest or highest category) was used as the reference group. A multivariate negative binomial regression and logistic regression model using the aforementioned subgroups as independent variables was subsequently performed to identify predictors of epilepsy incidence and prevalence, respectively.
While the Charlson comorbidity index can be associated with epilepsy incidence and prevalence, the association is likely to be at least partly due to certain disease components in the comorbidity index that are risk factors for epilepsy (e.g., hypertension and dementia).2930 To include the most relevant predictors, diseases associated with an increased risk of epilepsy were included in lieu of the Charlson comorbidity index to enhance model validity and reduce multicollinearity. The duration of enrollment in the AHCCCS during 2009 was used as the offset variable for the negative binomial regression. The choice between Poisson and negative binomial regression was based on whether there was evidence of overdispersion. c-Statistics were calculated to estimate the model predictability for the logistic regression model, and Monte Carlo simulations were conducted to ensure the generalizability of the c-statistics results. A c-statistic value of 0.5 indicated that the model predictability was no greater than chance, while values of >0.7 and >0.8 implied acceptable and strong predictability, respectively.31
Comorbid conditions that are potential risk factors for epilepsy were derived from the following International Classification of Diseases, Ninth Revision, Clinical Modification (ICD-9-CM) codes recorded in inpatient or outpatient claims: anoxic brain injury (ICD-9-CM: 348.1), stroke (ICD-9-CM: 362.34, 430.x-438.x), atherosclerosis (ICD-9-CM: 440.x, 443.9), primary brain tumor (ICD-9-CM: 191.x), Alzheimer's disease (ICD-9-CM: 331.0), Parkinson's disease (ICD-9-CM: 332.0), dementia (ICD-9-CM: 290.x, 294.1, 331.2); hypertension (ICD-9-CM: 401.x-405.x), and sleep apnea (ICD-9-CM: 327.2, 780.5). The comorbid conditions were calculated using the first 12 months in which a subject was continuously enrolled for the entire cohort, including incident and prevalent cases, and beneficiaries without epilepsy. For incident cases, the 12-month continuous enrollment occurs immediately prior to the index epilepsy claim date. For prevalent cases or beneficiaries without epilepsy, the 12-month continuous enrollment may or may not fall immediately prior to the index date. Missing values of patient demographics were imputed using random, hot-deck imputation techniques.32
Application of the patient eligibility criteria yielded a total of 472 incident cases and 1,220 prevalent cases (Fig. 1). The 2009 cohort consisted of 63,127 elderly beneficiaries who were continuously enrolled in the AHCCCS for at least 12 months between January 1, 2008 and December 31, 2009 (Fig. 2). The incidence and prevalence of epilepsy in this patient population were found to be 7.9 and 19.3 per 1,000 person-years, respectively.
Using the original incident case definition, bivariate analysis of the relationships between incidence rate and patient characteristics revealed that younger age and the nine comorbid conditions were associated with a significantly higher incidence (Table 1). Bivariate analysis of the relationships between prevalence rate and patient demographics and clinical attributes found that decreasing age (p<0.001), male gender (p<0.01), specific racial minorities (p<0.001), and the nine disease-based risk factors for epilepsy (all p<0.001) were associated with a significantly higher prevalence (Table 2).
After controlling for potential confounders, age and several comorbidities remained significantly associated with epilepsy incidence (p<0.05) (Table 3). The incidence of epilepsy among patients aged between 65 and 74 years of age was 1.56 [95% confidence interval (CI)=1.22-2.00] times and 2.33 (95% CI=1.67-3.23) times higher than for those aged between 75 and 84 years, and ≥85 years, respectively. Furthermore, the incidence rates among patients who had diagnostic codes for anoxic brain injury, stroke, primary brain tumor, Alzheimer's disease, dementia, and hypertension were 7.37 (95% CI=1.73-31.40), 4.65 (95% CI=3.54-6.11), 11.12 (95% CI=2.18-56.59), 2.25 (95% CI=1.56-3.27), 2.04 (95% CI=1.44-2.88), and 2.86 (95% CI=2.22-3.69) times higher than for their noncomorbid counterparts, respectively.
Age, gender, race, marital status, and several disease-based risk factors were statistically significant predictors of epilepsy prevalence. Beneficiaries aged between 65 and 74 years were associated with 1.64-fold (95% CI=1.43-1.85) and 3.03-fold (95% CI=2.50-3.70) higher odds of having epilepsy compared with those aged between 75 and 84 years and those aged ≥85 years, respectively. In addition, males were 13% (95% CI=0-28%) more likely to be a prevalent case compared with females, non-Hispanic Blacks were 33% (95% CI=3-72%) more likely to have epilepsy compared with non-Hispanic Whites, beneficiaries who were not married were 18% (95% CI=2-35%) more likely to be a prevalent case compared with married persons, and beneficiaries who had stroke, atherosclerosis, primary brain tumor, Alzheimer's disease, Parkinson's disease, dementia, and hypertension were associated with 3.81-fold (95% CI=3.34-4.33), 1.18-fold (95% CI=1.01-1.38), 4.44-fold (95% CI=2.14-9.20), 1.45-fold (95% CI=1.20-1.76), 1.69-fold (95% CI=1.30-2.20), 2.26-fold (95% CI=1.90-2.69), and 1.81-fold (95% CI=1.58-2.07) higher odds of having epilepsy compared with their noncomorbid counterparts, respectively.
The median value obtained in simulations of the c-Statistics for the logistic regression model of prevalence rate was 0.76 (SD=0.01), indicating that the model had reasonable predictability.
The incidence and prevalence of epilepsy among a low-income, elderly population in the US were calculated in this study using AHCCCS data. The overall incidence and prevalence of epilepsy in 2009 were exceptionally high in this patient population: 7.9 and 19.3 out of 1,000 patients were estimated to have developed newly diagnosed epilepsy and to have ongoing or newly diagnosed epilepsy, respectively. Several patient subgroups were at increased risk of developing incident epilepsy. Patients of younger age and those with specific disease-based risk factors (anoxic brain injury, stroke, primary brain tumor, Alzheimer's disease, dementia, and hypertension) had significantly higher incidence rates compared with their noncomorbid counterparts. Younger age, male gender, being a non-Hispanic Black, not-married status, and having existing risk factors for epilepsy (including stroke, atherosclerosis, primary brain tumor, Alzheimer's disease, Parkinson's disease, dementia, and hypertension) were associated with a higher prevalence of epilepsy.
This study did not use outpatient prescription claims to define epilepsy cases due to concerns regarding missing prescription claims data arising from the AHCCCS claims central database. Although the use of prescription drug claims is known to increase the specificity of case identification,33 this approach is likely to compromise algorithm sensitivity. In addition, while patients with epilepsy are highly likely to be prescribed outpatient antiepileptic drug, this is not guaranteed. Ideally, conducting a set of sensitivity analyses assessing the impact of using reliable prescription drug data to define cases can add clarity to the epidemiology of epilepsy in this patient population.
The current study found that the incidence and prevalence of epilepsy in the Medicaid elderly population were approximately 3.3-fold (current study vs. Faught et al.:24 7.9 vs. 2.4 per 1,000) and 1.8-fold (current study vs. Faught et al.:24 19.3 vs. 10.8 per 1,000) higher than the US Medicare population, respectively.24 Fewer stringent criteria were applied in the present study compared with that of Faught et al.,24 whereby patients were eligible as incident cases if they had a 1-year clean period without any seizure or epilepsy ICD-9 codes prior to the index date (as opposed to 2 years in Faught et al.24). In addition, patients who had one healthcare claim with a seizure ICD-9-CM code in 2009 and another in 2010 were included in the current study, as were patients who were not continuously enrolled throughout 2008 and 2009, but who were enrolled for at least 12 months; in contrast, Faught et al.24 excluded these latter patients. Both of these criteria boosted the incidence rate estimate to some extent: the former criterion increased the numerator (i.e., number of eligible cases) of the incidence rate, while the latter criterion increased both the numerator and denominator, with the degree of increase being greater for the numerator. However, these criteria were considered critical to more accurately reflect the incidence rate, since failure to account for these cases may lead to underestimation of the incidence.2434 For example, consider a patient who is diagnosed with epilepsy but who was not continuously enrolled throughout 2009. To accurately reflect the incidence, the patient should be included as a case (numerator value increased by 1), and the time of enrollment in the associated insurance program(s) should be added to the denominator [denominator value increased by <1 (year)]. Including this patient may result in an increase in the estimated incidence. A recent study performed by Kaiboriboon et al.25 estimated the epidemiology of nonelderly adult beneficiaries of the Ohio Medicaid system, and found that the incidence increased with age, from 1.5 per 1,000 person-years for those aged 18-24 years, to 5.7 per 1,000 person-years for those aged 55-64 years. Extrapolating this estimation by age would approximate an overall incidence of 7.9 per 1,000 person years in the present Medicaid elderly population. The prevalence was 46% higher for older Arizona beneficiaries (≥65 years) than for the younger (<65 years) Ohio Medicaid beneficiaries,25 but a lack of data precluded any attempt to extrapolate the Ohio data to an older age. These findings indicate that epilepsy may have both a high prevalence and incidence in the dually eligible population in the US compared with other populations, and immediate focus may be required to alleviate the burden.
In the current study, the incidence was highest among patients aged between 65 and 74 years and lowest among those aged >85 years; this trend was contrary to the patterns that are generally found in developed countries.1024 This finding may be partially explained by the population of interest predominantly comprising patients with minimal incomes, representing a vulnerable population of low SES. As such, individuals with epilepsy may have a high mortality rate. The age trend among the elderly poor can be compared with trends identified in countries with a comparatively lower SES-namely the developing countries. In developing countries, age does not appear to have a positive relationship with incidence.51035 The improvement of cerebrovascular mortality was regarded as an important factor contributing to the inverse relationship between age and the incidence rate of epilepsy among elderly patients.10 As the rate of stroke mortality appeared to be highest among low-income worldwide regions,36 the opposite age trend identified in this study may also indicate the need to focus on improving cerebrovascular mortality in this patient population.
Consistent with the existing literature, the present findings demonstrate an increased risk of epilepsy incidence and prevalence among Medicaid patients with cardiovascular (e.g., stroke and hypertension) or neurodegenerative conditions (e.g., dementia).3738 It has been shown that stroke, atherosclerosis, and neurodegenerative diseases account for 35.8%, 14.9%, and 12.0% of the etiology of epilepsy in the US elderly population.12 In contrast, the causes of epilepsy in younger adult patients are more likely to be related to congenital issues, head trauma, or brain abnormality.12 Overall, taking preventive actions against these diseases or conditions may further mitigate the risk of epilepsy.
This study was subject to several limitations that should be considered when interpreting its findings. First, the possibility of coding errors or underestimation of long-term epilepsy patients in the claims data and reported ICD-9 diagnostic codes cannot be excluded. Second, the primary analysis excluded the use of outpatient prescription drugs to define incident cases. This approach increased the sensitivity, with a trade-off against the specificity of the algorithm for defining an epilepsy case.27 Overestimation of incidence and underestimation of prevalence are possible when prescription drug data are not used, since some patients may have received antiepileptic drugs prior to the index event but did not have any epilepsy-related inpatient or outpatient visits. This approach is consistent with that used to estimate the epidemiology of epilepsy in the US Medicare population,24 and so the direction of overestimation or underestimation should be fairly consistent with the said study. Third, the number of diagnosis codes used to define epilepsy in this study was near the upper range used in other studies.2425 Increasing the number of diagnosis codes required to define a case may also increase the specificity of the identified sample (while decreasing the sensitivity). Fourth, using a 1-year "clean-period" of no seizure or epilepsy diagnosis codes may not effectively exclude all prevalent cases.39 The study conducted by Bakaki et al.39 using Ohio Medicaid claims data found that the incidence rates of epilepsy for clean periods of 1 year and 3 years were 5.50 and 4.05, respectively. Relative to data obtained using the 3-year clean period as the baseline approach, results using a 1-year clean period represent a 35.8% overestimation from an incidence rate ratio perspective, or an absolute value difference of 1.45 from an incidence rate difference perspective. Incidence estimates from the present study can be roughly adjusted to 5.8 using the incidence rate ratio approach and 6.5 using the incidence rate difference approach, both still exceeding the incidence estimates obtained from the US Medicare or the general population by a factor of at least 2.2324 Patients with stable epilepsy might be chronically receiving antiepileptic drugs without the need to receive further medical care within 1 year. It is recommended that standard "rules of thumb" that optimize the internal and external validity with respect to the time frame of the clean period used to define an incident case be developed in order to increase the validity of incident case identification and enhance the comparability of results across study. Fifth, it was not possible to identify each beneficiary enrolled in Medicare and the type of insurance coverage (e.g., fee-for-service, capitated, or both) in this study due to data restrictions. Including these data in the regression models may more accurately measure the relationships between patient subgroups and the incidence and prevalence of epilepsy. Finally, the observational nature of the study means that the associations found between epilepsy incidence/prevalence and patient characteristics in the models may not represent causal relationships.
In summary, this study found an exceptionally high incidence of epilepsy in a population of US elderly on low incomes; the incidence was approximately 3 and 16 times higher than the incidence reported for the US Medicare population24 and the worldwide incidence (50.4 per 100,000 person-years), respectively.23 The prevalence was 79% higher in this population compared with the US Medicare population.24 Younger patients, males, non-Hispanic Blacks, and patients with particular disease-based risk factors for epilepsy had an increased risk of developing or having epilepsy. The high incidence/prevalence in this patient population is more likely to be attributable to the distinguishing patient characteristics (i.e., having both a low SES and being elderly) rather than geographic residence location. The mortality rate in this population could be substantial, since the prevalence-to-incidence ratio was less than 3. More original or meta-analytic epidemiologic studies involving various subpopulations of patients with epilepsy should be conducted to reduce the bias of indirect comparisons between the findings of different studies.
Figures and Tables
Table 1
Incidence per 1,000 person years in patient subgroups

Table 2
Annual prevalence in patient subgroups

*p<0.01, †p<0.001 in chi-square test for categorical subgroups and non-parametric trend test for ordinal subgroups, ‡Number of patients in each subgroup (n) refers to study-defined cases and controls, §Number of patients with various comorbid conditions slightly varies between incidence and prevalence cohorts (in Table 1 and 2) due to the different 1-year time frame used to identify comorbid conditions.
n: number of patients with epilepsy.
Table 3
Multivariate negative binomial regression on epilepsy incidence and prevalence*
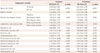
Acknowledgements
This study was funded by the Center for Disease Control and Prevention (CDC) Grant number 5U48DP001925. DHT was supported by the American Foundation for Pharmaceutical Education (AFPE) pre-doctoral fellowship.
References
1. Guidelines for epidemiologic studies on epilepsy. Commission on epidemiology and prognosis, international league against epilepsy. Epilepsia. 1993; 34:592–596.
3. Kelvin EA, Hesdorffer DC, Bagiella E, Andrews H, Pedley TA, Shih TT, et al. Prevalence of self-reported epilepsy in a multiracial and multiethnic community in New York City. Epilepsy Res. 2007; 77:141–150.


4. Koul R, Razdan S, Motta A. Prevalence and pattern of epilepsy (Lath/Mirgi/Laran) in rural Kashmir, India. Epilepsia. 1988; 29:116–122.


5. Lavados J, Germain L, Morales A, Campero M, Lavados P. A descriptive study of epilepsy in the district of El Salvador, Chile, 1984-1988. Acta Neurol Scand. 1992; 85:249–256.


6. Lee SY, Jung KY, Lee IK, Yi SD, Cho YW, Kim DW, et al. Prevalence of treated epilepsy in Korea based on national health insurance data. J Korean Med Sci. 2012; 27:285–290.


7. Melcon MO, Kochen S, Vergara RH. Prevalence and clinical features of epilepsy in Argentina. A community-based study. Neuroepidemiology. 2007; 28:8–15.


8. Picot MC, Baldy-Moulinier M, Daurès JP, Dujols P, Crespel A. The prevalence of epilepsy and pharmacoresistant epilepsy in adults: a population-based study in a Western European country. Epilepsia. 2008; 49:1230–1238.


9. Winkler AS, Kerschbaumsteiner K, Stelzhammer B, Meindl M, Kaaya J, Schmutzhard E. Prevalence, incidence, and clinical characteristics of epilepsy--a community-based door-to-door study in northern Tanzania. Epilepsia. 2009; 50:2310–2313.


10. Banerjee PN, Filippi D, Allen Hauser W. The descriptive epidemiology of epilepsy-a review. Epilepsy Res. 2009; 85:31–45.


11. Benn EK, Hauser WA, Shih T, Leary L, Bagiella E, Dayan P, et al. Estimating the incidence of first unprovoked seizure and newly diagnosed epilepsy in the low-income urban community of Northern Manhattan, New York City. Epilepsia. 2008; 49:1431–1439.


12. Hauser WA, Annegers JF, Kurland LT. Incidence of epilepsy and unprovoked seizures in Rochester, Minnesota: 1935-1984. Epilepsia. 1993; 34:453–468.


13. Cloyd J, Hauser W, Towne A, Ramsay R, Mattson R, Gilliam F, et al. Epidemiological and medical aspects of epilepsy in the elderly. Epilepsy Res. 2006; 68:Suppl 1. S39–S48.


14. Beghi E. Epilepsy. In : Feigin VL, Bennett DA, editors. Handbook of Clinical Neuroepidemiology. New York: Nova Science Publisher;2007. p. 123–165.
15. Sillanpää M, Lastunen S, Helenius H, Schmidt D. Regional differences and secular trends in the incidence of epilepsy in Finland: a nationwide 23-year registry study. Epilepsia. 2011; 52:1857–1867.


16. Hesdorffer DC, Hauser WA, Annegers JF, Kokmen E, Rocca WA. Dementia and adult-onset unprovoked seizures. Neurology. 1996; 46:727–730.


17. Noronha AL, Borges MA, Marques LH, Zanetta DM, Fernandes PT, de Boer H, et al. Prevalence and pattern of epilepsy treatment in different socioeconomic classes in Brazil. Epilepsia. 2007; 48:880–885.


18. Birbeck G, Chomba E, Atadzhanov M, Mbewe E, Haworth A. The social and economic impact of epilepsy in Zambia: a cross-sectional study. Lancet Neurol. 2007; 6:39–44.


19. Hesdorffer DC, Tian H, Anand K, Hauser WA, Ludvigsson P, Olafsson E, et al. Socioeconomic status is a risk factor for epilepsy in Icelandic adults but not in children. Epilepsia. 2005; 46:1297–1303.


20. Li X, Sundquist J, Sundquist K. Socioeconomic and occupational risk factors for epilepsy: a nationwide epidemiological study in Sweden. Seizure. 2008; 17:254–260.


21. Arizona Health Care Cost Containment System (US). Arizona Health Care Cost Containment System [Internet]. Phoenix, AZ: Arizona Health Care Cost Containment System;2012. cited 2013 Apr. Available from: http://www.azahcccs.gov/.
22. United States Census Bureau (US). United States Census Bureau [Internet]. Washington, DC: United States Census Bureau;2013. cited 2014 Mar 15. Available from: http://www.census.gov/.
23. Ngugi AK, Kariuki SM, Bottomley C, Kleinschmidt I, Sander JW, Newton CR. Incidence of epilepsy: a systematic review and meta-analysis. Neurology. 2011; 77:1005–1012.


24. Faught E, Richman J, Martin R, Funkhouser E, Foushee R, Kratt P, et al. Incidence and prevalence of epilepsy among older U.S. Medicare beneficiaries. Neurology. 2012; 78:448–453.


25. Kaiboriboon K, Bakaki PM, Lhatoo SD, Koroukian S. Incidence and prevalence of treated epilepsy among poor health and low-income Americans. Neurology. 2013; 80:1942–1949.


26. National Quality Measures Clearinghouse (US). National Quality Measures Clearinghouse [Internet]. Rockville, MD: National Quality Measures Clearinghouse;2012. cited 2013 Feb 14. Available from: http://www.qualitymeasures.ahrq.gov/index.aspx.
27. Kee VR, Gilchrist B, Granner MA, Sarrazin NR, Carnahan RM. A systematic review of validated methods for identifying seizures, convulsions, or epilepsy using administrative and claims data. Pharmacoepidemiol Drug Saf. 2012; 21:Suppl 1. 183–193.


28. Arain AM, Abou-Khalil BW. Management of new-onset epilepsy in the elderly. Nat Rev Neurol. 2009; 5:363–371.


29. Charlson ME, Pompei P, Ales KL, MacKenzie CR. A new method of classifying prognostic comorbidity in longitudinal studies: development and validation. J Chronic Dis. 1987; 40:373–383.


30. Quan H, Sundararajan V, Halfon P, Fong A, Burnand B, Luthi JC, et al. Coding algorithms for defining comorbidities in ICD-9-CM and ICD-10 administrative data. Med Care. 2005; 43:1130–1139.


31. Hosmer DW, Lemeshow S. Applied logistic regression. 2nd ed. New York: John Wiley & Sons;2000.
32. Harrell FE. Regression modeling strategies: with applications to linear models, logistic regression, and survival analysis. New York: Springer;2001.
33. Holden EW, Grossman E, Nguyen HT, Gunter MJ, Grebosky B, Von Worley A, et al. Developing a computer algorithm to identify epilepsy cases in managed care organizations. Dis Manag. 2005; 8:1–14.


34. Holden EW, Thanh Nguyen H, Grossman E, Robinson S, Nelson LS, Gunter MJ, et al. Estimating prevalence, incidence, and disease-related mortality for patients with epilepsy in managed care organizations. Epilepsia. 2005; 46:311–319.


35. Mani KS, Rangan G, Srinivas HV, Kalyanasundaram S, Narendran S, Reddy AK. The Yelandur study: a community-based approach to epilepsy in rural South India--epidemiological aspects. Seizure. 1998; 7:281–288.


36. Johnston SC, Mendis S, Mathers CD. Global variation in stroke burden and mortality: estimates from monitoring, surveillance, and modelling. Lancet Neurol. 2009; 8:345–354.


37. Amatniek JC, Hauser WA, DelCastillo-Castaneda C, Jacobs DM, Marder K, Bell K, et al. Incidence and predictors of seizures in patients with Alzheimer's disease. Epilepsia. 2006; 47:867–872.

