Abstract
Clinical professionals gain new information to assist in patient care when they read the medical literature. Similarly, in clinical preventive medicine, medical science documents that have previously published can be searched and evaluated in order to confirm the scientific support for the clinical preventive medical service offered in order to prevent chronic disease. This paper introduces the medical informatics techniques for knowledge extraction that can become the basis for clinical practice. Particularly, it discusses the clinical document retrieval and knowledge discovery tools that can search for extracting the knowledge which the medical expert desires with data mining techniques. For example, Clinical medical personnel and medical researchers can locate the information from the latest literature rapidly or find and evaluate the scientific basis for the treatment and prevention of infection. This study can be used when they analyze the correlation between accumulated and different type of data and contributes to the detection of new knowledge. Recently, the concern about the visualization of massive data and information is high as the importance of big data has received greater attention. Contributions to this technique and decision support tools will increase gradually due to the way support for decision-making through scientific evidence for the pattern changing disease is evaluated or as one of the clinical practice guidelines is accepted.
Figures and Tables
Figure 1
PathwayAssist graphical interface. Pathway assist enables researchers to create their own pathways and produce publication quality pathway diagrams. Pathway-Assist uses a proprietary graph visualization engine to allow for the visual features (From Nikitin A, et al. Bioinformatics 2003;19:2155-2157, with permission from Oxford University Press) [22].
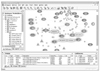
Figure 2
AliBaba Graphical Interface. (Parts of the) graph resulting from five PubMed ab-stracts for the query 'FADD'. Information on the selected protein 'caspase-8' is given in the right panel, for instance, association partners and evidence texts (From Plake C, et al. Bioinformatics 2006;22:2444-2445, with permission from Oxford University Press) [25].
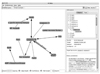
Acknowledgement
This research was supported by grant no. 10037283 from the Industrial Strategic Technology Development Program funded by the Ministry of Knowledge Economy.
References
1. Woolf SH, Grol R, Hutchinson A, Eccles M, Grimshaw J. Clinical guidelines: potential benefits, limitations, and harms of clinical guidelines. BMJ. 1999. 318:527–530.


2. Buchanan BG, Shortliffe EH. Rule based expert systems: the MYCIN experiments of the Stanford Heuristic Programming Project. 1984. Boston: Addison-Wesley Longman Publishing.
3. Vapnik VN. Statistical learning theory. 1998. New York: Wiley-Interscience.
4. Tan PN, Steinbach M, Kumar V. Introduction to data mining. 2007. Boston: Addison Wesley Longman.
5. Heckerman D. Bayesian networks for data mining. Data Min Knowl Discov. 1997. 1:79–119.
6. Lam W, Low KF, Ho CY. Pollack M, editor. Using a Bayesian network induction approach for text categorization. Proceedings of the 15th International Joint Conference on Artificial Intelligence. 1997. 1997 Aug 23-29; Nagoya, Japan. San Francisco: Morgan Kauffman;745–750.
7. Cho SB, Won HH. Chen YP, editor. Machine learning in DNA microarray analysis for cancer classification. Proceedings of First Asia-Pacific Bioinformatics Conference. 2003 Feb 4-7; Adelaide, Australia. Darlinghurst: Australian Computer Society;189–198.
8. Orr MJ. Introduction to radial basis function networks. 1996. Edinburgh: Centre for Cognitive Science.
9. Domingos P, Pazzani M. On the optimality of the simple Bayesian classifier under zero-one loss. Mach Learn. 1997. 29:103–130.
10. Cristianini N, Shawe-Taylor J. An introduction to support vector machines and other kernel-based learning methods. 2000. Cambridge: Cambridge University Press.
11. Grishman R, Sundheim B. Tsujii J, editor. Message understanding conference-6: a brief history. Proceedings of the 16th Conference on Computational Linguistics. 1996. 1996 Aug 5-7; Copenhagen, Denmark. Stroudsburg: Association for Computational Linguistics;466–471.
12. Kim SN, Martinez D, Cavedon L, Yencken L. Automatic classification of sentences to support evidence based medicine. BMC Bioinformatics. 2011. 12:Suppl 2. S5.


13. Nawaz R, Thompson P, McNaught J, Ananiadou S. Nicoletta C, Khalid C, Bente M, Joseph M, Jan O, Stelios P, Mike R, Daniel T, editors. Meta-knowledge annotation of bio-events. Proceedings of the 7th International Conference on Language Resources and Evaluation (LREC-2010). 2010. 2010 May 17-23; Valletta, Malta. [place unknown]: European Language Resources Association;2498–2505.
14. Pan F. Multi-dimensional fragment classification in biomedical text [dissertation]. 2006. Ontario: Queen's University.
15. Song MH, Kim SH, Park DK, Lee YH. A multi-classifier based guideline sentence classification system. Healthc Inform Res. 2011. 17:224–231.


16. Duda RO, Hart PE, Stork DG. Pattern classification. 2000. 2nd ed. New York: Wiley-Interscience.
17. Liu H, Yu L. Toward integrating feature selection algorithms for classification and clustering. IEEE Trans Knowl Data Eng. 2005. 17:491–502.


18. Baharudin B, Lee LH, Khan K. A review of machine learning algorithms for text-documents classification. J Adv Inf Technol. 2010. 1:4–20.


19. Yang Y, Pedersen JO. Fisher DH, editor. A comparative study on feature selection in text categorization. ICML 97: proceedings of the 14th International Conference on Machine Learning. 1997. 1997 Jul 8-12; Nashville, USA. San Francisco: Morgan Kaufmann;412–420.
20. Silla CN, Pappa GL, Freitas AA, Kaestner CA. Automatic text summarization with genetic algorithm-based attribute selection. Adv Artif Intell. 2004. 3315:305–314.


21. Anbarasi M, Anupriya E, Iyengar NC. Enhanced prediction of heart disease with feature subset selection using genetic algorithm. Int J Eng Sci Technol. 2010. 2:5370–5376.
22. Nikitin A, Egorov S, Daraselia N, Mazo I. Pathway studio: the analysis and navigation of molecular networks. Bioinformatics. 2003. 19:2155–2157.


23. Aronson AR. Effective mapping of biomedical text to the UMLS Metathesaurus: the MetaMap program. Proc AMIA Symp. 2001. 17–21.
24. Pub anatomy: integrated exploration of biomedical literature and data [Internet]. cited 2012 Jul 23. Ann Arbor: Microarray Lab;Available from: http://brainarray.mbni.med.umich.edu/Brainarray/prototype/PubAnatomy.
25. Plake C, Schiemann T, Pankalla M, Hakenberg J, Leser U. AliBaba: PubMed as a graph. Bioinformatics. 2006. 22:2444–2445.


26. Stoyanovich J, Mee W, Ross KA. Li F, Moro MM, Ghandeharizadeh S, Haritsa JR, Weikum G, Carey MJ, Casati F, Chang EY, Manolescu I, Mehrotra S, Dayal U, Tsotras VJ, editors. Semantic ranking and result visualization for life sciences publications. Proceedings of the 26th International Conference on Data Engineering, ICDE 2010. 2010. 2010 Mar 1-6; Long Beach, USA. [place unknown]: IEEE;860–871.

