Abstract
Purpose
This study aimed to evaluate the diagnostic value of SonoLiver software for parametric imaging in breast tumors.
Methods
Contrast-enhanced ultrasound (CEUS) was performed in 216 breast lesions (113 malignant, 103 benign). The CEUS parameters were compared between benign and malignant lesions. The rise time, the time to peak, the mean transit time and dynamic vessel pattern (DVP) were analyzed using SonoLiver software.
Results
Quantitative analysis showed that the rise time was 16.52±4.15 seconds in the benign group vs. 13.86±3.36 seconds in the malignant group (p=0.007), and the time to peak was 19.86±4.87 seconds in the benign group vs. 16.52±4.85 seconds in the malignant group (p=0.009). The mean transit time was 80.55±18.65 seconds in the benign group vs. 65.16±20.28 seconds in the malignant group (p=0.006). The difference between the distribution of DVP in benign and malignant tumors was statistically significant. One hundred one malignant tumors (89.4%) performed an irregular red/yellow fill in the region of interest (ROI) and 85 benign tumors (82.5%) performed a single blue/green fill in the ROI. The sensitivity, specificity, and accuracy of parametric imaging in breast tumors were 84.1%, 85.4%, 84.7%, respectively.
Several prior studies have found that contrast-enhanced ultrasound (CEUS) can differentiate between malignant and benign breast tumors [1-4]. However, the specificity was not optimal for clinical diagnostic use. Although the use of microbubbles increased the ability to distinguish between the two tumor types, the findings were only semi-quantitative and there was still a need to improve the specificity [5-8]. In order to improve the quantitative aspect of this technique, we used the SonoLiver software (the latest 1.0 version; TomTec Imaging Systems, Munich, Germany) to analyze the findings.
SonoLiver software facilitates the analysis of contrast-enhanced, dynamic vascular perfusion ultrasonography. Briefly, it provides automatic motion compensation by finding the optimal geometrical transformation for aligning a floating image with respect to a reference image [9]. Through this software, the time-intensity curve related to the perfusion can be obtained and used to analyze the quantitative perfusion characteristics of blood within a tumor, which is difficult by solely using an ultrasonography. The software was originally designed for diagnosing focal liver masses. Here, we investigated as to whether the SonoLiver software could be used to improve the analysis of breast tumors and possibly help distinguish the difference between malignant and benign lesions.
Parametric images of the relative dynamic vascular pattern (DVP) were generated by the SonoLiver software. SonoLiver implements video data linearization (i.e., creating signal amplitude of the time-intensity curve proportional to the echo power and thus proportional to the local concentration of the contrast agent). The final results are presented as parametric maps, summarizing the dynamic vascular signatures into single images, referred to as DVP parametric images; DVP parametric images reflect the different vascular signatures of lesions in comparison to the neighboring parenchyma [10]. In parametric imaging, the red area represents a shorter time whereas the blue area represents a longer time. In this study, ultrasound imaging examination was performed in 216 breast lesions. The CEUS parameters were analyzed by the latest SonoLiver software in order to discuss the diagnostic value in breast tumors.
The study was approved by the Ethics Committee of Shanghai Pudong New Area People Hospital, and patients' informed consent was obtained. From January 2010 to March 2012, 216 women (age range, 21-75 years; mean±SD, 46±12 years) were included in this study. All of the patients were prospectively selected on the basis of suspicion of breast lesions on conventional ultrasonography. Of these 216 patients, 201 had solitary lesions and 15 had multiple lesions. When a patient had multiple lesions, only the largest and most suspicious lesion on the baseline was evaluated; thus, 216 breast lesions in 216 patients constituted the study group. The maximum diameters of the lesions ranged from 6 to 31 mm (mean±SD, 18±7 mm). The diagnoses for all 216 lesions were confirmed by histopathologic examination with specimens obtained by a surgical resection. The pathologic analysis revealed 113 malignant breast lesions (93 ductal carcinoma, 4 invasive lobular carcinoma, 6 intraductal papillary carcinoma, 3 medullary carcinoma, 7 ductal carcinoma) and 103 benign breast lesions (68 fibroadenoma, 29 adenosis, 6 intraductal papilloma).
The study was performed with digital US scanners equipped with a dedicated program for low-mechanical-index studies (Sequoia; Siemens Medical Solutions, Mountain View, USA) and a 10 MHz transducer. The contrast medium employed was SonoVue (Bracco Imaging, Milan, Italy). The US study was carried out at a baseline and after an intravenous injection of a 2.5-mL bolus of contrast medium followed by about 5 mL of saline solution. CEUS was performed using continuous imaging with low mechanical index and panoramic scans targeted on the main lesion.
We used TomTec's SonoLiver software (latest 1.0 version). The quantitative analysis and parametric imaging process of SonoLiver was described in detail in the previous publications [9,10]. The SonoLiver software not only can quantitatively analyze the time-intensity of the lesion after contrast pulse sequence, but can also combine the DVP parameters. Further, SonoLiver can paint the region of interest (ROI): 1) border ROI, draw the imaging acoustic window to be analyzed, including the lesion and the surrounding tissue; 2) lesion ROI, draw the outline of the entire lesion to be analyzed; 3) reference ROI, the area neighboring the lesion ROI. SonoLiver outputs data 1) the peak intensity (maximum intensity, Imax) and the rise time (RT), defined as the time contrast that the agent reaches the lesion, associated with the blood supply, and the time to peak (TTP), defined as the time the lesions go up to the maximum contrast intensity, which is related to the lesion's enhanced speed. Mean transit time (mTT) is defined as the time the lesion began to increase to the half peak intensity, which relates to the contrast agent clearance speed in the lesion; 2) Dynamic Video, distribution of DVP (distribution of DVP parameter). DVP parametric images reflect the different vascular signatures of lesions in comparison to the neighboring parenchyma.
Quantitative analysis and parametric imaging were analyzed in all images of benign and malignant breast lesion. This study focused on the analysis of breast tumors in three time-related parameters (i.e., RT, TTP, and mTT) and on the distribution of DVP.
If the time-related parametric imaging (RT, TTP, and mTT parametric imaging) showed a clutter-color, colorful, massive or patchy distribution, it was given 1 point. If the parametric imaging showed clarity and a single blue/green color, it was given 0 points. RT, TTP, and mTT parameter of each case were scored and summed to yield a total score. For example, for the case in Figure 1, the RT parametric imaging was given 1 point (Figure 1A), the TTP parametric imaging was given 1 point (Figure 1B), and the mTT parametric imaging was given 1 point (Figure 1C). Finally, the total score of this case was 3 (1+1+1=3). In the case of Figure 2, the RT parametric imaging was given 0 points (Figure 2A), the TTP parametric imaging was given 0 points (Figure 2B), and the mTT parametric imaging was given 0 points (Figure 2C). Finally, the total score of this case was 0 (0+0+0=0). The range of the final total score of each case can only be 0 to 3. The lesion with a total score of 0 or 1 was classified as benign and the lesion with a total score of 2 or 3 was classified as malignant. Next, there were two sets of diagnostic results of this parametric method: benign (-) and malignant (+) identical to the pathological diagnosis. Finally, there were four kinds of cases: pathological (+) parameter (+); pathological (-) parameter (+); pathology (+) parameter (-); pathologic (-) parameter (-). The purpose of the pathological diagnosis, as the gold standard, was to calculate the sensitivity, specificity, and accuracy of the parameter diagnosis method. All parameter image scoring were independently analyzed by two senior physicians with extensive experience in ultrasound contrast.
SAS 8.0 statistics software (SAS Inc., Cary, USA) was used for data analysis. The w-test was applied to determine whether the data had normal or nonnormal distribution. The t-test was applied when the data followed a normal distribution. The Mann-Whitney U-nonparametric test was used when the data had a nonnormal distribution. The p <0.05 was considered as statistically significant.
Different regions in the lesions had different time values. SonoLiver software automatically codes the length of time for those that are color-coded. Purple, red, yellow, light blue, dark blue transition represents time from short to long. The red area represents a shorter time. The blue area represents a longer time. The time-dependent parametric imaging of 98 malignant lesions (98/113, 86.7%) performed a clutter-color, colorful, massive or patchy distribution (Figure 1). The time-dependent parametric imaging of 85 benign lesions (85/103, 82.5%) performed a single blue/green color distribution (Figure 2).
In this study, the rise time, time to peak and mean transit time was collectively referred to as time-related indicators. According to Table 1, the rise time and the time to peak were longer in the benign group than those in the malignant group. The mean transit time in the benign group was also longer than that in the malignant group. The difference was statistically significant (p=0.006).
The results depicted four kinds of cases: 95 cases were pathological (+) parameter (+), 15 cases were pathological (-) parameter (+), 18 cases were pathology (+) parameter (-), and 88 cases were pathologic (-) parameter (-). The sensitivity, specificity and accuracy of parametric imaging in breast tumors were 84.1%, 85.4%, 84.7%, respectively.
The distribution of DVP only appeared in the ROI of the lesion. In the distribution of DVP, yellow/red shows an enhanced level higher than the surrounding tissue, blue/green shows an enhanced level lower than the surrounding tissue, and black shows an enhanced level equal to the surrounding tissue.
The distributions of DVP in the benign and malignant tumors were significantly different. One hundred one malignant tumors (89.4%) performed an irregular red/yellow fill in ROI (Figure 3) and 85 benign tumors (82.5%) performed a single blue/green fill in ROI (Figure 4). Eighteen benign tumors (17.5%) performed a few points of linear red/yellow fill in ROI, and most of the tumors were a blue/green fill in ROI (Figure 5).
Ultrasound imaging has played a key role in the evaluation of breast lesions in clinical settings. It is very important to assess the blood flow changes in breast lesions. In this study, we investigated CEUS for the evaluation of breast lesions. To improve the quantitative aspect of this technique, we used the SonoLiver software. SonoLiver software differs from the traditional software because it uses the parameters to create DVP parametric imaging. The results show that the distribution of DVP for malignant tumors performed an irregular disorder or a red/yellow fill in ROI, whereas the distribution of DVP for benign tumors performed a single blue/green fill in ROI. Studies have confirmed that breast cancer has higher microvessel density (MVD) than benign tumors. Malignant tumors usually have a rich blood supply and a larger number of feeding arteries as compared to benign tumors [3]. The pathological basis of benign and malignant tumors results in its own characteristic distribution of DVP parametric imaging. DVP parametric imaging can reduce the pressure for ultrasound physicians in reading the images and contributing to the differential diagnosis of benign and malignant tumors.
Our study also found that the time-dependent parametric imaging (RT, TTP, and mTT parametric imaging) had significant differences in benign and malignant tumors. The 82.5% of benign lesions performed a single blue/green distribution and 86.7% of malignant lesions performed a clutter-color, colorful, massive or patchy red/yellow distribution. Parameters in each region of the lesion were different; the reasons for such difference could be due to the angiogenesis of benign breast tumor, which is the proliferation of normal breast blood vessels, thickening, such that this may result in parameter images relative to a single color. The angiogenesis of breast cancer is the revascularization and spatial distribution of clutter, vascular uneven thickness, traveling tortuous, arteriovenous fistula formation or blind-side blood vessels. These pathological changes may lead to the variation in the time points of the rise time, time to peak and mean transit time in breast cancer imaging. Therefore, it results in a strong correlation between time and imaging of breast cancer.
The rise time and time to peak of breast cancer were significantly shorter than that of benign tumors (p=0.007 and p=0.009, respectively), consistent with the study of Li et al. [11]. Breast cancer belongs to a rich blood supply of tumors, vascular thickening, distorting, and often there is dynamic arteriovenous fistula [12-14]. The vascular proliferation of benign tumors is from normal breast blood vessels. Their different vascular pathological features are the main reasons as to why RT and TTP of malignant tumors are shorter than that of benign lesions.
The mTT in the benign group is longer than that in the malignant group (p=0.006). Several studies have also obtained similar results [15-17], but only one of these studies [17] showed a statistically significant difference in mTT between benign and malignancy. The shorter mTT likely reflects these morphological differences, resulting in a more rapid flow of microbubbles through malignant tumors as compared to the more normal vasculature of a benign mass. It may also result from arteriovenous shunting due to incomplete or inappropriate blood vessel formation. In addition, the sample size of our study is very large; therefore, our findings are more credible.
Our study used a total score to quantify the rise time, time to peak and mean transit time parameters. This method is parametric imaging diagnostic method and relatively easy to use in the differential diagnosis of benign and malignant breast tumors. This method takes full advantage of the characteristics of the three time-related indicators. It is more comprehensive and objective to reflect on the characteristics of parametric imaging. The parametric imaging of the diagnostic method combines high sensitivity and specificity (84.1% and 85.4%, respectively). Moreover, this method has high clinical value. It would be interesting to determine whether CEUS improves the diagnostic accuracy of malignant versus benign characterization over gray-scale and color Doppler findings. It may not substantially improve the gray-scale diagnosis but could yield an increase in diagnostic confidence, which is also critical to the diagnosis.
However, there were limitations to this study. First, it is difficult to scan an entire breast within a few minutes before the contrast agent washes out. Therefore, breast CEUS can only image a particular lesion at any one time. Second, in this study, we selected the reference ROI, the area neighboring the lesion ROI, it included both breast parenchyma and fat tissue. It can affect the value and the analysis of the lesion ROI. Our project group will conduct further research on this issue.
In conclusion, there have been limited studies on the use of CEUS in distinguishing between benign and malignant breast tumors; yet, the results have not been consistent, indicating a need for further development [12]. Our findings indicate that CEUS parametric imaging appears to have a clinical value in improving the differential diagnosis of benign and malignant breast tumors.
Notes
This research was supported by Shanghai Health Bureau research projects (No. 20114Y165); Pudong New Area, the health system of outstanding young medical personnel training program (No. PWRq2010-09); Pudong New Area, Shanghai Health Bureau of Health Science and Technology Development (No. PW2011A-15); Pudong New Area leading talents training plan (No. PWR12012-02).
References
1. Zhao H, Xu R, Ouyang Q, Chen L, Dong B, Huihua Y. Contrast-enhanced ultrasound is helpful in the differentiation of malignant and benign breast lesions. Eur J Radiol. 2010; 73:288–293. PMID: 19559551.


2. Ricci P, Cantisani V, Ballesio L, Pagliara E, Sallusti E, Drudi FM, et al. Benign and malignant breast lesions: efficacy of real time contrast-enhanced ultrasound vs. magnetic resonance imaging. Ultraschall Med. 2007; 28:57–62. PMID: 17304413.


3. Du J, Li FH, Fang H, Xia JG, Zhu CX. Microvascular architecture of breast lesions: evaluation with contrast-enhanced ultrasonographic micro flow imaging. J Ultrasound Med. 2008; 27:833–842. PMID: 18499843.
4. Jiang YX, Liu H, Liu JB, Zhu QL, Sun Q, Chang XY. Breast tumor size assessment: comparison of conventional ultrasound and contrast-enhanced ultrasound. Ultrasound Med Biol. 2007; 33:1873–1881. PMID: 17686569.


5. Saracco A, Aspelin P, Leifland K, Themudo R, Wilczek B, Axelsson R. Bolus compared with continuous infusion of microbubble contrast agent using real-time contrast harmonic imaging ultrasound in breast tumors. Acta Radiol. 2009; 50:854–859. PMID: 19634024.


6. Sorelli PG, Cosgrove DO, Svensson WE, Zaman N, Satchithananda K, Barrett NK, et al. Can contrast-enhanced sonography distinguish benign from malignant breast masses? J Clin Ultrasound. 2010; 38:177–181. PMID: 20146214.


7. Barnard S, Leen E, Cooke T, Angerson W. A contrast-enhanced ultrasound study of benign and malignant breast tissue. S Afr Med J. 2008; 98:386–391. PMID: 18637311.
8. Kettenbach J, Helbich TH, Huber S, Zuna I, Dock W. Computer-assisted quantitative assessment of power Doppler US: effects of microbubble contrast agent in the differentiation of breast tumors. Eur J Radiol. 2005; 53:238–244. PMID: 15664287.


9. Guo L, Liu ZG, Han PH, Yuan Q, He Y, Li J. Perfusion curve f (t) analysis of breast cancer by contrast-enhanced ultrasonography. Acta Radiol. 2012; 53:981–986. PMID: 22969089.


10. Anaye A, Perrenoud G, Rognin N, Arditi M, Mercier L, Frinking P, et al. Differentiation of focal liver lesions: usefulness of parametric imaging with contrast-enhanced US. Radiology. 2011; 261:300–310. PMID: 21746815.


11. Li YJ, Wen G, Wu FL. Study on the regional hemodynamic perfusion characteristics in benign and malignant breast tumors by contrast-enhanced ultrasound quantitative analysis. Biomed Eng Clin Med. 2009; 13:431–435.
12. Balleyguier C, Opolon P, Mathieu MC, Athanasiou A, Garbay JR, Delaloge S, et al. New potential and applications of contrast-enhanced ultrasound of the breast: own investigations and review of the literature. Eur J Radiol. 2009; 69:14–23. PMID: 18977102.


13. Liu H, Jiang YX, Liu JB, Zhu QL, Sun Q. Evaluation of breast lesions with contrast-enhanced ultrasound using the microvascular imaging technique: initial observations. Breast. 2008; 17:532–539. PMID: 18534851.


14. van Esser S, Veldhuis WB, van Hillegersberg R, van Diest PJ, Stapper G, ElOuamari M, et al. Accuracy of contrast-enhanced breast ultrasound for pre-operative tumor size assessment in patients diagnosed with invasive ductal carcinoma of the breast. Cancer Imaging. 2007; 7:63–68. PMID: 17513187.


15. Caproni N, Marchisio F, Pecchi A, Canossi B, Battista R, D'Alimonte P, et al. Contrast-enhanced ultrasound in the characterisation of breast masses: utility of quantitative analysis in comparison with MRI. Eur Radiol. 2010; 20:1384–1395. PMID: 20033178.


16. Li SP, Padhani AR, Taylor NJ, Beresford MJ, Ah-See ML, Stirling JJ, et al. Vascular characterisation of triple negative breast carcinomas using dynamic MRI. Eur Radiol. 2011; 21:1364–1373. PMID: 21258931.


17. Makkat S, Luypaert R, Sourbron S, Stadnik T, De Mey J. Quantification of perfusion and permeability in breast tumors with a deconvolution-based analysis of second-bolus T1-DCE data. J Magn Reson Imaging. 2007; 25:1159–1167. PMID: 17520720.


Figure 1
In a 59-year-old woman with a palpable mass in the inner upper quadrant of her left breast, surgical pathology proved an invasive ductal carcinoma. Rise time (RT) parametric imaging was given 1 point (A), time to peak (TTP) parametric imaging was given 1 point (B), and mean transit time (mTT) parametric imaging was given 1 point (C). Finally, the total score of this case was 3 (1+1+1=3).

Figure 2
In a 35-year-old woman with a palpable mass in the outer upper quadrant of her right breast, surgical pathology proved a fibroadenoma. Rise time (RT) parametric imaging was given 0 points (A), time to peak (TTP) parametric imaging was given 0 points (B), and mean transit time (mTT) parametric imaging was given 0 points (C). Finally, the total score of this case was 0 (0+0+0=0).

Figure 3
In a 68-year-old woman with a palpable mass in the outer upper quadrant of her left breast, surgical pathology proved an invasive ductal carcinoma. Distribution of dynamic vessel pattern performed a red/yellow fill.
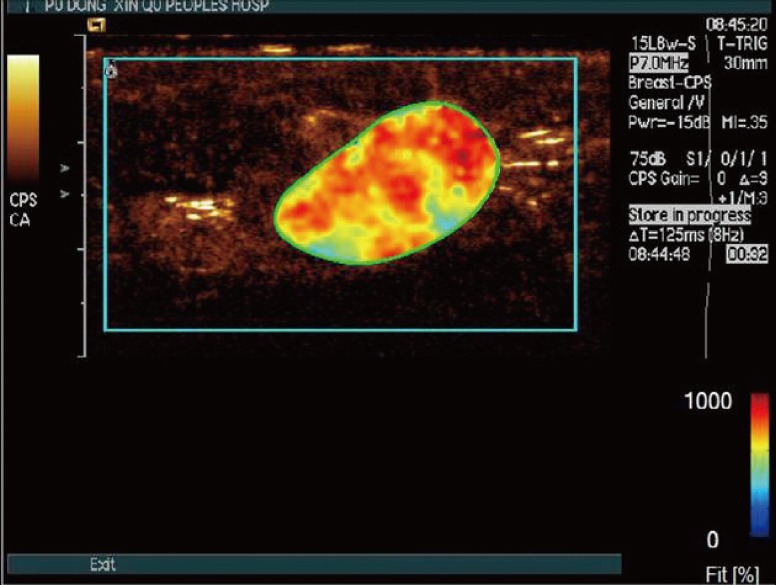
Figure 4
In a 25-year-old woman with a palpable mass in the inner upper quadrant of her left breast, surgical pathology proved a fibroadenoma. Distribution of dynamic vessel pattern performed a single blue/green fill.
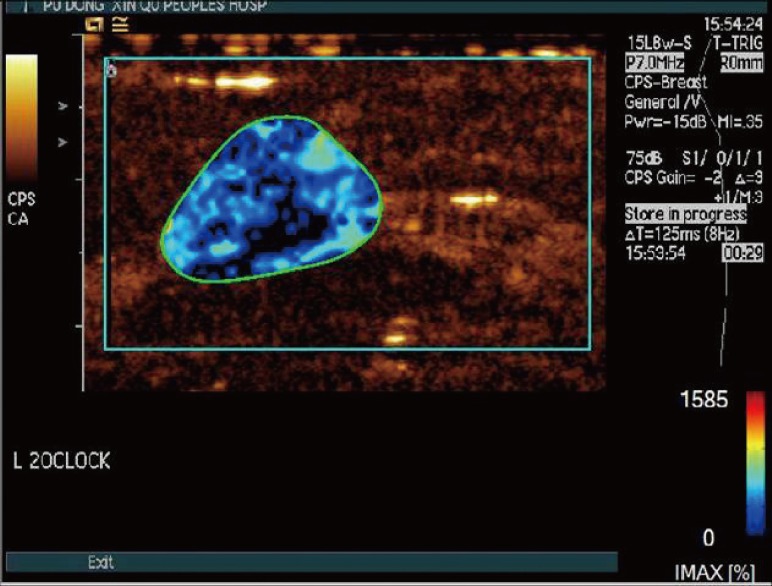