Abstract
The recent advent of "-omics" technologies have heralded a new era of personalized medicine. Personalized medicine is referred to as the ability to segment heterogeneous subsets of patients whose response to a therapeutic intervention within each subset is homogeneous. This new paradigm in healthcare is beginning to affect both research and clinical practice. The key to success in personalized medicine is to uncover molecular biomarkers that drive individual variability in clinical outcomes or drug responses. In this review, we begin with an overview of personalized medicine in breast cancer and illustrate the most encountered statistical approaches in the recent literature tailored for uncovering gene signatures.
Not all patients respond equally to cancer therapeutic compounds. Recent advances in high-throughput genomic, transcriptomic, and proteomic technologies with the ever-increasing understanding of the molecular mechanisms of cancers permit uncovering genes that harbor personal variations in clinical outcomes or drug responses. Personalized medicine has revolutionized the healthcare paradigm by integrating personal genetic information, improving the drug treatment efficacy, shifting the practice of medicine, and creating opportunities to introduce new business and healthcare economic models.
The traditional standard "one-dose-fits-all" approach to drug development and clinical therapy has been ineffective, as it incurs all risks of subsequent drug toxicities and treatment failures [1]. The percentage of patients for whom a major drug is effective is presented in Figure 1 [1]. With the great variability across diseases, 38% to 75% of patients fail to respond to a treatment. The average response rate of a cancer drug is the lowest at 25%.
Adverse drug reactions as a consequence of treatment are more of a problem. Among drugs approved in the U.S., 16% have shown adverse drug reactions [1]. A frequently cited meta-analysis revealed that 6.7% of all hospitalized patients are associated with adverse drug reactions in the U.S. and that the number of deaths exceeds 100,000 cases annually [2]. A study conducted in a major hospital identified 2,227 cases of adverse drug effects among hospitalized patients and reported that 50% of these cases are likely to be related to genetic factors [3].
Personalized medicine is the ability to segment heterogeneous subsets of patients whose response to a therapeutic intervention within each subset is homogeneous [4]. Under this new healthcare paradigm, physicians can make optimal choices to maximize the likelihood of effective treatment and simultaneously avoid the risks of adverse drug reactions; scientists can improve the drug discovery process, and pharmaceutical companies can manufacture medical devices to forecast patient prognosis, facilitating early disease detection.
The ultimate goal of personalized medicine is to furnish the proper treatment to the right person at the right time [5]. The potential impact of personalized medicine is contingent upon a systematic discovery of a novel biomarker from genome-wide candidates that account for variations across individuals. This review begins with an overview of personalized medicine and illustrates the most encountered statistical approaches for uncovering biomarkers utilized in the recent literature.
Personalized medicine has been defined in many ways. According to the U.S. National Institutes of Health (NIH), personalized medicine is "an emerging practice of medicine that uses an individual's genetic profile to guide decisions made in regard to the prevention, diagnosis, and treatment of disease" [6]. The U.S. Food and Drug Administration defined personalized medicine as "the best medical outcomes by choosing treatments that work well with a person's genomic profile or with certain characteristics in the person's blood proteins or cell surface proteins" [7]. The President's Council of Advisors on Science and Technology (PCAST) described personalized medicine as "tailoring of medical treatment to the individual characteristics of each patient" [4].
It is important to recognize that personalized medicine does not literally mean individuality. The idea of personalized medicine has often been exaggerated, as suggested in a headline in Newsweek (June 10, 2005) "Medicine Tailored Just for You." In fact, a new treatment regimen is assessed on a group of carefully selected patients but not individuals [5]. As such, PCAST reports that personalized medicine is "the ability to classify individuals into subpopulations that differ in their susceptibility to a particular disease or their response to a specific treatment" [4]. If a new treatment works effectively on a sub-patient group, a preventive intervention can then be furnished to those who will benefit, avoiding adverse drug effects and sparing expense for those who will not.
A biomarker is a reliable and accurate measurement that indicates a normal biological process, a pathogenic process, or a pharmacological response to a therapeutic intervention [8]. With this broad and general definition, biomarkers include physiological measurements such as lung function, blood pressure or electroencephalography, molecular (DNA, protein, metabolite) or cellular measures from biofluids (blood, plasma, serum, and urine), molecular, cellular or histopathological measures from solid tissue samples, and measurements from magnetic resonance imaging or computed tomography images [9].
In this review, we will concentrate on "prognostic" and "predictive" biomarkers that forecast patient outcomes. A prognostic biomarker is related with a patient's clinical outcome and can be used to select patients for an adjuvant systemic treatment irrespective of the patient response to treatment, whereas a predictive biomarker is related to the patient's response to a particular intervention.
According to a U.S. NIH Consensus Conference, "a clinical useful prognostic biomarker must be a proven independent, significant factor that is easy to determine and interpret and that has therapeutic consequences" [10]. A prognostic biomarker provides information about the patients overall cancer outcome irrespective of the therapeutic response [11]. Therefore, a prognostic biomarker can be exploited to select patients for an adjuvant systemic treatment but does not forecast the treatment response [6].
Decision making about adjuvant systemic treatment for breast cancer is usually based on nodal status [12-14], tumor size [15,16], tumor type/grade [17-20], lymphatic and vascular invasion [21,22], tumor hormone receptor and human epidermal growth factor receptor 2 (HER2)/neu status [23-26], age [27,28], and ethnicity [29-31]. Prognostic biomarkers that provide better information on relapse risk could prevent many patients from chemotherapy toxicity without compromising survival [32]. Significant prognostication of a biomarker needs to be demonstrated in prospective randomized clinical trials.
In contrast, a predictive biomarker provides information about the effect of a therapeutic intervention [32]. In other words, a predictive biomarker enables screening of a subset of patients that are responsive to a specific therapy where response is defined by any of the clinical endpoints commonly measured in clinical trials [33]. As a predictive biomarker indicates heterogeneous benefits contingent upon sub-patient risk groups classified by the status of the biomarker, a significant interaction between treatment effects and patient categories needs to be statistically validated, ideally in a randomized clinical trial [34].
Predictive biomarkers can help physicians to forecast the effects of a particular treatment. Numerous proteins and genes exist that are specifically associated with breast cancer growth, proliferation, and metastasis. The deeper understanding of their roles regarding the responses of various therapies may empower physicians to determine optimal treatments for patients with breast cancer [35].
Some biomarkers are both prognostic and predictive (Table 1) [36,37]. For example, patients with estrogen receptor (ER) and/or progesterone receptor (PR)-positive tumors have longer survival than those with hormone receptor-negative tumors [15,38]. Additionally, a recent randomized trial reported that high cellular ER and PR expression predicts the benefit from adjuvant tamoxifen [39].
As another example, HER2/neu gene amplification, which leads to overexpression of its receptor on the cell membrane in approximately 30% of human breast tumors, is related with a worse prognosis in patients with node-positive breast cancer due to increased proliferation and angiogenesis and inhibition of apoptosis [23-26]. HER2/neu is also the target for the monoclonal antibody trastuzumab from which patients with HER2/neu overexpressing tumors benefit in a metastatic and adjuvant setting [40-42].
The wide-ranging impacts and myriad opportunities provided by personalized medicine can be summarized in reference to its four major attributes [5].
Personalized medicine integrates personal genetic or protein profiles to strengthen healthcare at a more personalized level, particularly with the aid of recently emerging "-omic" technologies such as nutritional genomics, pharmacogenomics, proteomics, and metabolomics [43]. Personalized medicine targets what has a positive effect on a patient's disease and then develops safe and effective treatments for that specific disease [5]. In fact, genetic biomarkers that may be specifically associated with a disease state are the foundation of personalized medicine. Knowledge of a patient's genetic profile leads to the proper medication or therapy so that physicians can manage a patient's disease or predisposition towards it using the proper dose or treatment regimen [6].
Personalized medicine pursues not reaction but reaction. With the ability to forecast disease risk or presence before clinical symptoms appear, personalized medicine offers the opportunity to act on the disease through early intervention. In lieu of reacting to advanced stages of a disease, preventive intervention can be life-saving in many cases. For example, females with genetic mutations in the BRCA1 or BRCA2 genes have a higher chance of developing breast cancer compared to those in the general female population [44,45]. An accurate test of these breast cancer susceptibility genes can guide surveillance and preventive treatment based on objective risk measurements such as increased frequency of mammography, prophylactic surgery, and chemoprevention (Table 2) [46].
Personalized medicine enables physicians to select optimal therapies and avoid adverse drug reactions. Molecular diagnostic devices using predictive biomarkers provide valuable information regarding genetically defined subgroups of patients who would benefit from a specific therapy. For example, Oncotype DX® (Genomic Health, Redwood City, USA) uses a 16-gene signature to determine whether women with certain types of breast cancer are likely to benefit from chemotherapy [47-49]. MammaPrint® (Agendia, Amsterdam, the Netherlands) uses a 70-gene expression profile to assess the risk of distant metastasis in patients with early-stage breast cancer [50]. These complex diagnostic tests can be used to classify patients into subgroups to inform physicians whether patients would be treated successfully with hormone therapy alone or may require more aggressive chemotherapy treatment.
Personalized medicine would lead to an increase in patient adherence to treatment [51]. When personalized healthcare assures its effectiveness and can minimize adverse treatment effects sparing the expenses, patients will be more likely and willing to comply with their treatments.
The critical component to success in personalized medicine is to uncover gene signatures that drive individual variability in clinical outcomes or drug responses. A number of systematic approaches have been proposed to identify molecular fingerprints that are predictive of patient prognosis and response to cancer treatments. In this review, we focused on the most encountered methods for biomarker discovery: data-driven and knowledge-driven approaches.
In the data-driven approach, biomarkers associated with tumor traits are objectively searched in genome-wide analysis using data-mining tools. Unbiased biomarker discovery is the merit of this approach. A downside is that gene signatures identified by the data-driven approach are often difficult to interpret due to limited knowledge about their biological functions. In contrast, the knowledge-driven approach attempts to select candidate genes using prior knowledge or surveying the literature for evidence of linkage to either cancer pathological processes or pathways important in drug responses. As such, genes that are unknown to be involved in a process cannot be included.
The combination of the data-driven and knowledge-driven approach has been used to develop gene signatures [48]. Biomarker discovery in genome-wide analysis is subject to the curse of dimensionality, i.e., the situation in which there are far more genomic variables than the number of samples [52]. One way to deal with this issue is to use the knowledge-driven approach to reduce the number of candidate genes detected by an objective genome-wide search.
As an illustration of the data-driven approach, recently proposed systematic data-driven approaches based on in vitro-generated predictive profiles using cell-line models entail five key technical steps: 1) data collection, 2) quality control, 3) identification of candidate gene biomarkers, 4) construction of a multivariate prediction model, and 5) independent validation of the prediction model (Figure 2) [53-57].
Biomarker discovery begins by collecting molecular data in a drug response experiment. A large amount of genomic or genetic characteristics on cell-lines are experimentally determined using high-throughput technologies. The drug's patterns of activity in cells are measured on a continuous (percent of cell survival or death) or discrete scale (responsive or resistant).
The immediate procedure following acquisition of a large amount of molecular data is quality control or pre-processing. Due to the nature of high-throughput technologies that introduce inevitable non-biologic noises and biases during data collection, appropriate normalization according to specific array technologies is performed before further analysis. It is important to note that quality control can affect downstream data analysis.
The subsequent step after assuring an adequate level of normalization is to identify the subset of genes that are candidate predictors highly associated with drug activities. This step reduces the parameter space of gene variables in a very high dimension [41]. In the previous studies, various practical approaches have been used, including classical two-sample t-tests, variant t-tests [58-61], empirical Bayes methods [62-64], a linear mixed-effect model [65], the generalized likelihood ratio test [66] and the local-pooled-error test [67]. Note that these statistical approaches rely on underlying assumptions such as distributional specifications, exchangeability for a random-effect distribution, constant coefficients of variation, a mean-variance relationship, and others.
Upon narrowing down candidate genes to a few hundred, a statistical classification modeling technique is then used to construct a multivariate prediction model. Single biomarkers are less likely to furnish sufficient sensitivity and specificity for most applications [35]. Several classification methods have been utilized, including a variant of linear discriminant analysis [68], support vector machines [69-71], Bayesian regression [72], partial least squares [73], principal component regression [74], and between-group analysis [75]. The performance of a statistical prediction model should be tested and assessed by various statistical measures such as classification error rate and area under the receiver operating characteristic curve, the product of posterior classification probabilities [76-78], and an index so-called the misclassification-penalized posterior [79]. The leave-one-out approach, random splitting, and bootstrapping are often employed for an internal cross validation. Additionally, multicenter validation is also performed for an external cross validation. It has been implied from previous studies that no one dominating classifier outperforms all other methods.
Finally, the ultimate evidence of the usefulness of a prediction model in a clinical setting is randomized, prospective validation in a clinical trial [80]. After refinement and validation in independent cohorts, the covariates in the prediction model can be used to develop assays that accurately predict prognosis and responses to chemotherapeutic agents, contributing to the development of "personalized medicine" for patients with cancer.
Personalized medicine is receiving a large amount of growing attention for its tremendous potential with myriad new opportunities. The ultimate promise of personalized medicine depends on the discovery of the personal genetic causes of disease. The remarkable advent of current high-throughput technologies in combination with improved knowledge of the molecular basis of malignancy provides a solid base for identifying novel molecular targets. This revolutionized paradigm in healthcare is already beginning to affect both research and clinical practice.
The use of high-throughput technologies is expected to greatly increase in the next few years as the cost of technologies will continue to drop (Figure 3) [81]. Genomic sequencing and its interpretation will have to be further developed and standardized for routine clinical practice to develop efficient and effective methods for discovering and verifying new biomarkers and enabling personalized medicine technologies. In particular, efforts to standardize existing technologies will lead to more reproducible and robust identification of biomarkers.
Several challenges must be overcome before this flood of profile data is successfully translated into clinical utilities for patients with breast cancer. Improved knowledge obtained using advanced profile technologies will not be sufficient for this purpose, but all stakeholders involved in personalized medicine should work together to take responsibility. Regulatory authorities should provide clear guidelines for evaluating and approving newly developed personalized drugs and should validate the capabilities of the diagnostic devices that predict patient prognoses or drug responses. Medical educational institutions should prepare the next generation of physicians to use and interpret personal genetic information appropriately and responsibly. Finally, public and private insurers need to evaluate the clinical and economic utility of personalized drugs and devices to facilitate reimbursement.
Figures and Tables
Figure 1
Inefficacy of the one-dose-fits-all approach. This figure depicts the percentage of patients for whom a major drug is effective on average. With the high variability across diseases, 38% to 75% of patients fail to respond to a treatment. The average response rate of a cancer drug is the lowest at 25%, suggesting that 75% of patients with cancer are over-dosed and will potentially suffer from an adverse drug reaction. From Spear BB, et al. Trends Mol Med 2001;7:201-4 [1].
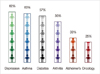
Figure 3
Cost of sequencing a human-sized genome. Note that a logarithmic scale is used on the Y axis. The cost of sequencing rapidly decreased at an exponential rate from 2001 to 2007. The sudden drop in cost around January 2008 was due to sequencing technology geared up from the first generation ("Sanger-based" or dideoxy chain termination sequencing) to the second generation (or "next-generation"). The cost of sequencing has dramatically decreased since 2008. From Wetterstrand KA. DNA sequencing costs: data from the NHGRI large-scale genome sequencing program. http://www.genome.gov/sequencingcosts/ [81].
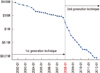
Table 1
Personalized medicine drugs for breast cancer as of July 2012
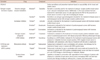
Data from National Cancer Institute. Drug information: drugs approved for different types of cancer. http://www.cancer.gov/cancertopics/druginfo/drug-page-index [36], National Cancer Institute. Drug information: drugs approved for breast cancer. http://www.cancer.gov/cancertopics/druginfo/breastcancer [37].
Table 2
In vitro diagnostic devices for breast cancer as of July 2012
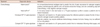
Data from U.S. Food and Drug Administration. Drugs@FDA: FDA approved drug products. http://www.accessdata.fda.gov/scripts/cder/drugsatfda [46].
References
1. Spear BB, Heath-Chiozzi M, Huff J. Clinical application of pharmacogenetics. Trends Mol Med. 2001. 7:201–204.


2. Lazarou J, Pomeranz BH, Corey PN. Incidence of adverse drug reactions in hospitalized patients: a meta-analysis of prospective studies. JAMA. 1998. 279:1200–1205.


3. Classen DC, Pestotnik SL, Evans RS, Lloyd JF, Burke JP. Adverse drug events in hospitalized patients. Excess length of stay, extra costs, and attributable mortality. JAMA. 1997. 277:301–306.


4. President's Council of Advisors on Science and Technolgy. Priorities for Personalized Medicine. 2008. Washington, DC: President's Council of Advisors on Science and Technolgy.
5. Pfizer. Think Science Now Perspective. Approaches to Cancer Care: the Promise of Personalized Medicine. 2010. New York: Pfizer.
6. U.S. National Institutes of Health, U.S. National Library of Medicine. Genetics home reference: Glossary. Accessed July 1st, 2012. http://ghr.nlm.nih.gov/glossary=personalizedmedicine.
8. Biomarkers Definitions Working Group. Biomarkers and surrogate endpoints: preferred definitions and conceptual framework. Clin Pharmacol Ther. 2001. 69:89–95.
9. Micheel C, Ball J. Institute of Medicine (U.S.). Committee on Qualification of Biomarkers and Surrogate Endpoints in Chronic Disease. Evaluation of Biomarkers and Surrogate Endpoints in Chronic Disease. 2010. Washington, DC: National Academies Press.
10. NIH consensus conference. Treatment of early-stage breast cancer. JAMA. 1991. 265:391–395.
11. Clark GM, Zborowski DM, Culbertson JL, Whitehead M, Savoie M, Seymour L, et al. Clinical utility of epidermal growth factor receptor expression for selecting patients with advanced non-small cell lung cancer for treatment with erlotinib. J Thorac Oncol. 2006. 1:837–846.


12. Saez RA, McGuire WL, Clark GM. Prognostic factors in breast cancer. Semin Surg Oncol. 1989. 5:102–110.


13. Nemoto T, Natarajan N, Bedwani R, Vana J, Murphy GP. Breast cancer in the medial half. Results of 1978 National Survey of the American College of Surgeons. Cancer. 1983. 51:1333–1338.


14. Fisher B, Bauer M, Wickerham DL, Redmond CK, Fisher ER, Cruz AB, et al. Relation of number of positive axillary nodes to the prognosis of patients with primary breast cancer. An NSABP update. Cancer. 1983. 52:1551–1557.


15. Carter CL, Allen C, Henson DE. Relation of tumor size, lymph node status, and survival in 24,740 breast cancer cases. Cancer. 1989. 63:181–187.


16. Rosen PP, Groshen S, Kinne DW, Norton L. Factors influencing prognosis in node-negative breast carcinoma: analysis of 767 T1N0M0/T2N0M0 patients with long-term follow-up. J Clin Oncol. 1993. 11:2090–2100.


17. Carstens PH, Greenberg RA, Francis D, Lyon H. Tubular carcinoma of the breast. A long term follow-up. Histopathology. 1985. 9:271–280.


18. Clayton F. Pure mucinous carcinomas of breast: morphologic features and prognostic correlates. Hum Pathol. 1986. 17:34–38.


19. Ridolfi RL, Rosen PP, Port A, Kinne D, Miké V. Medullary carcinoma of the breast: a clinicopathologic study with 10 year follow-up. Cancer. 1977. 40:1365–1385.


20. Le Doussal V, Tubiana-Hulin M, Friedman S, Hacene K, Spyratos F, Brunet M. Prognostic value of histologic grade nuclear components of Scarff-Bloom-Richardson (SBR). An improved score modification based on a multivariate analysis of 1262 invasive ductal breast carcinomas. Cancer. 1989. 64:1914–1921.


21. Rosen PP, Groshen S, Saigo PE, Kinne DW, Hellman S. Pathological prognostic factors in stage I (T1N0M0) and stage II (T1N1M0) breast carcinoma: a study of 644 patients with median follow-up of 18 years. J Clin Oncol. 1989. 7:1239–1251.


22. Neville AM, Bettelheim R, Gelber RD, Säve-Söderbergh J, Davis BW, Reed R, et al. The International (Ludwig) Breast Cancer Study Group. Factors predicting treatment responsiveness and prognosis in node-negative breast cancer. J Clin Oncol. 1992. 10:696–705.


23. Winstanley J, Cooke T, Murray GD, Platt-Higgins A, George WD, Holt S, et al. The long term prognostic significance of c-erbB-2 in primary breast cancer. Br J Cancer. 1991. 63:447–450.


24. Borg A, Tandon AK, Sigurdsson H, Clark GM, Fernö M, Fuqua SA, et al. HER-2/neu amplification predicts poor survival in node-positive breast cancer. Cancer Res. 1990. 50:4332–4337.
25. Paterson MC, Dietrich KD, Danyluk J, Paterson AH, Lees AW, Jamil N, et al. Correlation between c-erbB-2 amplification and risk of recurrent disease in node-negative breast cancer. Cancer Res. 1991. 51:556–567.
26. Clark GM, McGuire WL. Follow-up study of HER-2/neu amplification in primary breast cancer. Cancer Res. 1991. 51:944–948.
27. Nixon AJ, Neuberg D, Hayes DF, Gelman R, Connolly JL, Schnitt S, et al. Relationship of patient age to pathologic features of the tumor and prognosis for patients with stage I or II breast cancer. J Clin Oncol. 1994. 12:888–894.


28. Albain KS, Allred DC, Clark GM. Breast cancer outcome and predictors of outcome: are there age differentials? J Natl Cancer Inst Monogr. 1994. 35–42.
29. Daly MB, Clark GM, McGuire WL. Breast cancer prognosis in a mixed Caucasian-Hispanic population. J Natl Cancer Inst. 1985. 74:753–757.
30. Elledge RM, Clark GM, Chamness GC, Osborne CK. Tumor biologic factors and breast cancer prognosis among white, Hispanic, and black women in the United States. J Natl Cancer Inst. 1994. 86:705–712.


31. Pierce L, Fowble B, Solin LJ, Schultz DJ, Rosser C, Goodman RL. Conservative surgery and radiation therapy in black women with early stage breast cancer. Patterns of failure and analysis of outcome. Cancer. 1992. 69:2831–2841.


32. Oldenhuis CN, Oosting SF, Gietema JA, de Vries EG. Prognostic versus predictive value of biomarkers in oncology. Eur J Cancer. 2008. 44:946–953.


33. Bentzen SM, Buffa FM, Wilson GD. Multiple biomarker tissue microarrays: bioinformatics and practical approaches. Cancer Metastasis Rev. 2008. 27:481–494.


34. Clark GM. Prognostic factors versus predictive factors: examples from a clinical trial of erlotinib. Mol Oncol. 2008. 1:406–412.


35. Phan JH, Moffitt RA, Stokes TH, Liu J, Young AN, Nie S, et al. Convergence of biomarkers, bioinformatics and nanotechnology for individualized cancer treatment. Trends Biotechnol. 2009. 27:350–358.


36. National Cancer Institute. Drug information: drugs approved for different types of cancer. Accessed July 1st, 2012. http://www.cancer.gov/cancertopics/druginfo/drug-page-index.
37. National Cancer Institute. Drug information: drugs approved for breast cancer. Accessed July 1st, 2012. http://www.cancer.gov/cancertopics/druginfo/breastcancer.
38. Grann VR, Troxel AB, Zojwalla NJ, Jacobson JS, Hershman D, Neugut AI. Hormone receptor status and survival in a population-based cohort of patients with breast carcinoma. Cancer. 2005. 103:2241–2251.


39. Early Breast Cancer Trialists' Collaborative Group (EBCTCG). Effects of chemotherapy and hormonal therapy for early breast cancer on recurrence and 15-year survival: an overview of the randomised trials. Lancet. 2005. 365:1687–1717.
40. Romond EH, Perez EA, Bryant J, Suman VJ, Geyer CE Jr, Davidson NE, et al. Trastuzumab plus adjuvant chemotherapy for operable HER2-positive breast cancer. N Engl J Med. 2005. 353:1673–1684.


41. Smith I, Procter M, Gelber RD, Guillaume S, Feyereislova A, Dowsett M, et al. 2-year follow-up of trastuzumab after adjuvant chemotherapy in HER2-positive breast cancer: a randomised controlled trial. Lancet. 2007. 369:29–36.


42. Vogel CL, Cobleigh MA, Tripathy D, Gutheil JC, Harris LN, Fehrenbacher L, et al. Efficacy and safety of trastuzumab as a single agent in first-line treatment of HER2-overexpressing metastatic breast cancer. J Clin Oncol. 2002. 20:719–726.


43. Jones DS, Hofmann L, Quinn S. 21st Century Medicine: A New Model for Medical Education and Practice. 2010. Gig Harbor: The Institute for Functional Medicine;23–87.
44. Struewing JP, Hartge P, Wacholder S, Baker SM, Berlin M, McAdams M, et al. The risk of cancer associated with specific mutations of BRCA1 and BRCA2 among Ashkenazi Jews. N Engl J Med. 1997. 336:1401–1408.


45. National Cancer Institute. BRCA1 and BRCA2: cancer risk and genetic testing. Accessed July 1st, 2012. http://www.cancer.gov/cancertopics/factsheet/Risk/BRCA.
46. U.S. Food and Drug Administration. Drugs@FDA: FDA approved drug products. Accessed July 1st, 2012. http://www.accessdata.fda.gov/scripts/cder/drugsatfda/.
47. Hornberger J, Cosler LE, Lyman GH. Economic analysis of targeting chemotherapy using a 21-gene RT-PCR assay in lymph-node-negative, estrogen-receptor-positive, early-stage breast cancer. Am J Manag Care. 2005. 11:313–324.
48. Paik S, Tang G, Shak S, Kim C, Baker J, Kim W, et al. Gene expression and benefit of chemotherapy in women with node-negative, estrogen receptor-positive breast cancer. J Clin Oncol. 2006. 24:3726–3734.


49. Cronin M, Pho M, Dutta D, Stephans JC, Shak S, Kiefer MC, et al. Measurement of gene expression in archival paraffin-embedded tissues: development and performance of a 92-gene reverse transcriptase-polymerase chain reaction assay. Am J Pathol. 2004. 164:35–42.
50. MammaPrint Agendia. Accessed July 1st, 2012. http://www.agendia.com/pages/mammaprint/21.php.
51. Personalized Medicine Coalition. The Case for Personalized Medicine. 2011. 3rd ed. Washington, DC: Personalized Medicine Coalition.
52. Catchpoole DR, Kennedy P, Skillicorn DB, Simoff S. The curse of dimensionality: a blessing to personalized medicine. J Clin Oncol. 2010. 28:e723–e724.


53. Cheng F, Cho SH, Lee JK. Multi-gene expression-based statistical approaches to predicting patients' clinical outcomes and responses. Methods Mol Biol. 2010. 620:471–484.


54. Lee JK, Havaleshko DM, Cho H, Weinstein JN, Kaldjian EP, Karpovich J, et al. A strategy for predicting the chemosensitivity of human cancers and its application to drug discovery. Proc Natl Acad Sci U S A. 2007. 104:13086–13091.


55. Smith SC, Baras AS, Dancik G, Ru Y, Ding KF, Moskaluk CA, et al. A 20-gene model for molecular nodal staging of bladder cancer: development and prospective assessment. Lancet Oncol. 2011. 12:137–143.


56. Staunton JE, Slonim DK, Coller HA, Tamayo P, Angelo MJ, Park J, et al. Chemosensitivity prediction by transcriptional profiling. Proc Natl Acad Sci U S A. 2001. 98:10787–10792.


57. Neve RM, Chin K, Fridlyand J, Yeh J, Baehner FL, Fevr T, et al. A collection of breast cancer cell lines for the study of functionally distinct cancer subtypes. Cancer Cell. 2006. 10:515–527.


58. Efron B, Tibshirani R, Storey JD, Tusher V. Empirical bayes analysis of a microarray experiment. J Am Stat Assoc. 2001. 96:1151–1160.


59. Dudoit R, Yang YH, Callow MJ, Speed TP. Statistical methods for identifying differentially expressed genes in replicated cDNA microarray experiments. Stat Sin. 2002. 12:111–139.
60. Smyth GK. Linear models and empirical bayes methods for assessing differential expression in microarray experiments. Stat Appl Genet Mol Biol. 2004. 3:Article 3.


61. Hu J, Wright FA. Assessing differential gene expression with small sample sizes in oligonucleotide arrays using a mean-variance model. Biometrics. 2007. 63:41–49.


62. Lönnstedt I, Speed T. Replicated microarray data. Stat Sin. 2002. 12:31–46.
63. Newton MA, Kendziorski CM, Richmond CS, Blattner FR, Tsui KW. On differential variability of expression ratios: improving statistical inference about gene expression changes from microarray data. J Comput Biol. 2001. 8:37–52.


64. Newton MA, Noueiry A, Sarkar D, Ahlquist P. Detecting differential gene expression with a semiparametric hierarchical mixture method. Biostatistics. 2004. 5:155–176.


65. Wolfinger RD, Gibson G, Wolfinger ED, Bennett L, Hamadeh H, Bushel P, et al. Assessing gene significance from cDNA microarray expression data via mixed models. J Comput Biol. 2001. 8:625–637.


66. Wang S, Ethier S. A generalized likelihood ratio test to identify differentially expressed genes from microarray data. Bioinformatics. 2004. 20:100–104.


67. Jain N, Thatte J, Braciale T, Ley K, O'Connell M, Lee JK. Local-pooled-error test for identifying differentially expressed genes with a small number of replicated microarrays. Bioinformatics. 2003. 19:1945–1951.


68. Golub TR, Slonim DK, Tamayo P, Huard C, Gaasenbeek M, Mesirov JP, et al. Molecular classification of cancer: class discovery and class prediction by gene expression monitoring. Science. 1999. 286:531–537.


69. Brown MP, Grundy WN, Lin D, Cristianini N, Sugnet CW, Furey TS, et al. Knowledge-based analysis of microarray gene expression data by using support vector machines. Proc Natl Acad Sci U S A. 2000. 97:262–267.


70. Furey TS, Cristianini N, Duffy N, Bednarski DW, Schummer M, Haussler D. Support vector machine classification and validation of cancer tissue samples using microarray expression data. Bioinformatics. 2000. 16:906–914.


71. Mukherjee S, Tamayo P, Slonim D, Verri A, Golub T, Mesirov JP, et al. Support Vector Machine Classication of Microarray Data. 1998. Cambridge: Massachusetts Institute of Technology.
72. West M, Blanchette C, Dressman H, Huang E, Ishida S, Spang R, et al. Predicting the clinical status of human breast cancer by using gene expression profiles. Proc Natl Acad Sci U S A. 2001. 98:11462–11467.


73. Nguyen DV, Rocke DM. Tumor classification by partial least squares using microarray gene expression data. Bioinformatics. 2002. 18:39–50.


74. Nagji AS, Cho SH, Liu Y, Lee JK, Jones DR. Multigene expression-based predictors for sensitivity to Vorinostat and Velcade in non-small cell lung cancer. Mol Cancer Ther. 2010. 9:2834–2843.


75. Culhane AC, Perrière G, Considine EC, Cotter TG, Higgins DG. Between-group analysis of microarray data. Bioinformatics. 2002. 18:1600–1608.


76. Bradley AP. The use of the area under the ROC curve in the evaluation of machine learning algorithms. Pattern Recognit. 1997. 30:1145–1159.


77. Hand DJ. Construction and Assessment of Classification Rules. 1997. Chichester: Wiley.
78. Soukup M, Lee JK. Developing optimal prediction models for cancer classification using gene expression data. J Bioinform Comput Biol. 2004. 1:681–694.


79. Soukup M, Cho H, Lee JK. Robust classification modeling on microarray data using misclassification penalized posterior. Bioinformatics. 2005. 21:Suppl 1. i423–i430.


80. Buyse M, Sargent DJ, Grothey A, Matheson A, de Gramont A. Biomarkers and surrogate end points: the challenge of statistical validation. Nat Rev Clin Oncol. 2010. 7:309–317.


81. Wetterstrand KA. DNA sequencing costs: data from the NHGRI large scale genome sequencing program. Accessed July 1st, 2012. http://www.genome.gov/sequencingcosts/.