Abstract
Because autoimmune diseases (AIDs) result from a complex combination of genetic and epigenetic factors, as well as an altered immune response to endogenous or exogenous antigens, systems biology approaches have been widely applied. The use of multi-omics approaches, including blood transcriptomics, genomics, epigenetics, proteomics, and metabolomics, not only allow for the discovery of a number of biomarkers but also will provide new directions for further translational AIDs applications. Systems biology approaches rely on high-throughput techniques with data analysis platforms that leverage the assessment of genes, proteins, metabolites, and network analysis of complex biologic or pathways implicated in specific AID conditions. To facilitate the discovery of validated and qualified biomarkers, better-coordinated multi-omics approaches and standardized translational research, in combination with the skills of biologists, clinicians, engineers, and bioinformaticians, are required.
Autoimmune diseases (AIDs) are the third most common category of disease after cancer and heart disease and affect approximately 5~8% of the general population. Sex-linked differences are striking, with AIDs considered a top 10 leading cause of death in women under the age of 65 (1). More than 100 different types have been recognized, and the majority of autoimmune problems are associated with eight disorders: rheumatoid arthritis (RA), type I diabetes mellitus, multiple sclerosis, psoriasis, systemic lupus erythematosus (SLE), ulcerative colitis, Crohn's disease, and scleroderma (2). AIDs arise from interactions between environmental, epigenetic, and genetic factors that result in persistent activation of immune responses and their biological networks (3). Conventionally, the development of AID occurs in three separate stages (Fig. 1).
The development of autoimmunity is strongly influenced by inherited polymorphisms (or DNA sequence variations). However, the enormous diversity of genetic variants contributes to the susceptibility for AIDs. These genetic associations can be dissected into distinct classes. For most AIDs, the strongest genetic associations are with the HLA locus. HLA haplotype linkages are shared between AIDs, and most HLA associations seem to be disease-specific (4). For example, the HLA class II molecule, HLA-DR3, shows a strong correlation with SLE, Sjögren's syndrome (5), and subtypes of autoimmune myositis and is associated with the production of antibodies against DNA in lupus and anti-Jo1 production in myositis (6). In Sjögren's syndrome, the association between HLA-DRB1*03 and the production of auto-antibodies against Ro is even stronger than the association of HLA with the disease (7).
Many of the strong loci that have been associated with one AID are also involved in other AIDs. Typically, the allele of these shared variants that is a risk factor for one autoimmune disease is also a risk factor for other autoimmune diseases. For instance, variants in the PTPN22 gene have been associated, with varying degrees of risk, with type 1 diabetes, autoimmune thyroid diseases, and RA (8,9).
In genome-wide association studies, several inherited polymorphisms or DNA sequence variations that cause AIDs have been identified. The sequence variations (single nucleotide polymorphisms, SNPs) are highly "polygenic," predominantly determined by non-HLA genes, and have limited impact on disease onset (10).
However, most of the identified SNPs are not linked to disease-related genetic markers. A number of SNPs are estimated to share pathogenic pathways in the development of AIDs or link common genes with the risk of autoimmune and/or inflammatory diseases. About 100 disease-risk SNPs have been identified in celiac disease, Crohn's disease (11), multiple sclerosis (12), psoriasis (13), rheumatoid arthritis (14,15), SLE (16), and type 1 diabetes (17). Interestingly, Hu et al. found that 47/107 (44%) immune-mediated disease risk SNPs are associated with multiple autoimmune diseases, leading to the proposal that distinct groups of interacting molecules that share a disease risk are encoded close to SNPs that influence the same subsets of diseases (18). To help identify the precise causal alleles in specific cell types, the genomes of diseased cell types that are studied need to contain disease-associated genetic variants. This challenge is highlighted in systemic AIDs, such as RA, where any of a broad range of immunological cell types are potentially impacted by genetic variation to cause disease. Potentially pathogenic cell types in autoimmune diseases have been identified: transitional B cell genes in SLE, epithelial-associated stimulated dendritic cell genes in Crohn's disease, and CD4+ effector and memory T cell genes within RA loci (18).
The most striking evidence of environmental effects on the development of AIDs is the high discordance rate observed in monozygotic twins (19). Discordance enables the study of epigenetic changes that lead to the development of autoimmunity. In an individual with a susceptible genotype, exposure to environmental factors, including sunlight, diet, chemicals, smoking, allergens, infectious agents, or environmental toxins, not only triggers downstream signaling of the susceptible genes but also initiates epigenetic changes before and after the development of autoimmune responses (20,21).
Epigenetics is defined as the inheritable effects that influence gene activity without changes in DNA sequence. Epigenetic gene regulation has an essential role in determining individual gene function and activity in each specific cell type. The epigenetic changes include four predominant mechanisms (22): DNA methylation, histone modifications, nucleosome positioning, and microRNAs (miRNAs). These four mechanisms control gene expression and assist in the regulation of cell differentiation, proliferation, DNA repair, and replication. Epigenetic modifications are far more sensitive to environmental stimuli than the sequence of DNA. Candidate gene studies of AIDs have identified a small set of genes that undergo epigenetic changes, such as aberrant DNA demethylation, histone modification, and miRNAs, in rheumatic diseases. T cells from patients with SLE or RA, as well as synovial fibroblasts from individuals with RA (8,23), contain hypomethylated DNA sequences and/or histone modifications in particular cell types. The identification of target sequences for epigenetic deregulation will provide clinical markers for diagnosis, disease progression, and therapies for AIDs. High-throughput sequencing is necessary to screen for epigenetic alterations in specific tissue and cell types that are relevant to disease pathogenesis (24). Another area of study is miRNAs, a class of small noncoding RNAs that regulate gene expression by pairing with their target mRNAs and are often deregulated in AIDs (25). To further understand the role of epigenetic changes in AIDs, we have to keep in mind that most autoimmune disorders are not due simply to the possession of a specific gene but also to conditions that affect the gene.
Exposure to environmental triggers by a genetically susceptible host favors the activation of both the innate and adaptive immune systems. The innate immune system, consisting of physical mucosal barriers, proteins, and cells, serves as the front line against infectious agents or other environmental challenges. Innate immune activation in autoimmunity leads to the production of type 1 interferon (IFN), usually produced in response to viral infection, which in turn activates about 400 genes, denoted the interferon signatures (26). Type 1 IFN induces the activation of dendritic cells (27,28), increasing the expression of MHC class I and II molecules, costimulatory molecules, chemokines, and chemokine receptors (29) and inducing B-cell maturation and differentiation into plasma cells by secretion of IL-6, production of B cell activating factor (TNFSF13B), and a proliferation-inducing ligand (TNFSF13). Dendritic cells activated by IFN-α support and contribute to the plasticity and differentiation of T-cell subsets, and promote the expansion of T cells. IFN-α further stimulates CD4+ T cells to enhance antigen-specific B-cell responses. In the adaptive immune system, antigen presenting cells, including monocytes/macrophages and dendritic cells, can be activated by endogenous and/or exogenous antigens, resulting in the release of cytokines that promote differentiation of naive T cells into effector subsets. Th1 cell differentiation is promoted by IL-12 and IFN-γ, Th2 cells depend on IL-4, and TGF-β is crucial for the development of regulatory T cells. In addition, in the presence of IL-6, TGF-β differentiates into a highly proinflammatory subset of Th17 cells, initially characterized by IL-17 production. Stable differentiation and proliferation of Th17 cells also needs IL-21 and IL-23. The differentiated effector T cells drive inflammation, tissue injury, and autoantibody production.
Th17 cells are closely associated with many systemic AIDs. Increased amounts of IL-17 and a high proportion of IL-17-producing T cells have been reported in patients with SLE; a correlation with disease activity has also been noted. T cells that produce IL-17 are detectable in the main target organs in SLE (i.e., the skin, kidneys, and lungs), suggesting that IL-17 has a role in local inflammation and tissue damage (30). Furthermore, patients with Sjögren's syndrome show high levels of expression and increased concentrations of IL-17 and IL-23 in the plasma. Enhanced expression of IL-17, together with augmented production of IFN-γ and expression of MHC class I, has been identified in muscle specimens from autoimmune myositis patients. Several genetic risk factors in SLE and Sjögren's syndrome are related to the formation or maintenance of Th17 cells. Interferon regulatory factor 5 (IRF5) plays a role in type 1 IFN pathways and in the expression of genes important for Th17 cell responses (31) by acting as a transcription factor for IL-6 and the p40 subunit of IL-23. The potential functional effect of this genetic association is supported by increased amounts of both IL-6 and p40 in patients with SLE (32) and Sjögren's syndrome (5). Among pre-immune B cells, augmented proportions of transitional and naive B cells are detectable in patients with Sjögren's syndrome. These memory cells can be activated by a combination of toll-like receptor agonists and TNFSF13 or TNFSF13B and by cytokine combinations, such as IL-21 and TNFSF13B.
Blood transcriptome studies to determine the expression levels of mRNAs of a given cell population are essential for autoimmunity-related translational research (33,34,35,36). Such transcriptome studies measure the levels of RNA transcripts in patient samples, which have been influenced by environmental factors or pathologic conditions. Samples from cells, serum, fluid, and tissues are crucial for AIDs translational research and should be carefully stored, under standardized methods of patient sample aquisition, archiving, sequential high-throughput molecular analyses, and ongoing phenotypic data possession, allowing for the bioinformatics testing. Using polychromatic flow cytometry, intracellular or extracellular markers in each cell phenotype can be identified. In vitro cellular assays can measure the innate or antigen-specific responsiveness of cells exposed to exogeneous or endogenous antigens. Multiple proteomic assays can be used to determine the antibody specificity of serum levels of cytokines or chemokines (37).
During the past decade, new next generation sequencing technology has been used for DNA-based microarrays and studies of cellular RNA levels for different AIDs. Whole transcriptome profiling studies have been used to identify biomarkers for diagnosis, assess disease activity, predict flare-ups, and determine the response to treatment. More quantitative and sensitive high-throughput RNA sequencing methods have resulted in the precise evaluation of the blood transcriptome as a routine test in the clinical setting. Well-known examples of successful blood transcriptome analyses have been reported in the case of SLE. In 2003, two independent teams identified a strong IFN signature in pediatric and adult SLE patients (38), with a central role for dendritic cells and IFN-α in the disease (39), and this was later confirmed by additional studies using microarrays and RT-PCR. Subsequent genetic studies identified strong associations between SLE and type I IFN-related gene polymorphisms (i.e., IRF5 (31), STAT4 (40,41), TREX1 (42,43)) and proteomics studies showed that the levels of some IFN-regulated chemokines could be used to predict SLE flare-ups in patients with quiescent disease (44). Although DNA microarray studies have led to important advances in the study of autoimmunity, their use is limited because changes in transcript abundance can occur by many parameters other than disease status. Biological variability makes it difficult to control false positive results. An additional problem lies in the fact that a large proportion of mRNAs are not translated into proteins, despite being upregulated at the level of transcription. Thus, more standardized proteomic work and well-designed public datasets need to be developed. Transcriptomic research in patients with AIDs can be used to guide other biologic approaches, including proteomics or genomic studies, and can also provide the basis for early translational and clinical applications.
Biomarkers have been identified from the variety of human genes, genetic variation, measurements of RNA, proteins, and metabolites. Numerous biomarkers can be quantitatively measured in patient samples, including plasma, serum, cerebrospinal fluid, bronchoalveolar lavage, tissue biopsies, whole blood, urine, and saliva. Biomarkers are dynamic and informative biological substances (i.e., cellular, biochemical, molecular, genetic, protein, metabolite, specific post-translational modification, or physiological or physical signs) that can be evaluated as indicators of normal versus pathogenic biological processes, and disease progression or response to a therapeutic intervention. Clinically, biomarkers can be classified based on their application, such as predictor, diagnostic, prognostic, mechanistic, pharmacodynamic, safety, and surrogate endpoint biomarkers (45). Advances in high-throughput and multiplexed processes, microarray, and global-scale genomic and proteomic approaches have made it possible to characterize different clinical spectra associated with disease and the therapeutic interventions. Novel analytical technologies have led to various methods of immune cell profiling, such as multi-parameter flow cytometry, multiplex analysis of intra- and extra-cellular mediators, and genome-wide profiles of gene expression.
The use of multi-omics platforms and multiplexed approaches in the analysis of systemic AIDs will not only help to identify numerous useful biomarkers but also will expose areas for further improvement in translational research (46,47,48,49).
There is growing agreement that more than one biomarker is needed for accurate efficacy or safety decisions. In this context, using multiplexed technology and a multi-omics approach could offer a significant contribution to biomarker discovery for translational research in early stages of AIDs. Improved genomic approaches that use high-throughput molecular and cellular profiling of cells in different clinical subsets and consisting of measuring the abundance of cellular RNA and microRNAs expressed by T cells will help in biomarker discovery.
The biomarker discovery used for the CD4+ T cell differentiation process is shown in Fig. 2. During the differentiation of Th17 cells in the presence of chemical compounds or small interfering RNA (siRNA) libraries, cell-specific transcriptomics and proteomics were applied to discover biomarkers targeting Th17 cells (50,51). The function of identified candidate genes was further investigated using RNA interference (RNAi) and by defining regulatory protein-binding sites in the genome via chromatin immunoprecipitation (ChIP) (Fig. 2) (52).
Researchers have recently explored the genes expressed at different time points during Th17 cell development. They detected almost 1,300 genes involved in over 10,000 interactions, with 71 regulators (53). Researchers validated potential immune regulatory biomarkers for Th17 autoimmunity using silicon nanowires to deliver siRNA into naive T cells (54). The biomarkers they found during Th17 cell differentiation, derived from improved, advanced approaches with multi-omics, could be particularly useful in the development of targeted therapies and identification of AIDs subgroups. Most of the novel biomarkers and their correlations to disease were derived largely from two or more samples at one point in time. The methodologies employed show snapshot events of the disease state at a specific time, whereas signaling networks are dynamic in both structure and their use. Because single measurements rarely capture all dimensions of clinical outcomes, a multidimensional and continuous model is needed (34).
The human transcriptome consists of approximately 25,000 genes. The post-genomic era has provided a wealth of novel approaches for generating high-dimensional genetic and transcriptomic data sets from large cohorts of human diseases and normal individuals. Over the last 10 years, there has been a strong focus on molecular characterization of biological systems in various disease states. Blood transcriptome analysis has been useful for screening new biomarkers in various AIDs. In addition to the blood profiling approach, the study of specific cell subsets using multi-omics approaches will be useful for dissecting molecular heterogeneity, allowing for the discovery of new biomarkers and translation of those biomarkers into new therapeutic options for AIDs (Fig. 3).
Systems biology in AIDs has included expression profiling and functional analysis of DNA, RNA, protein, and metabolites of specific cell subsets in each stage of disease (55). Current efforts for discovery and development of disease-related biomarkers will assist in optimal decision-making throughout the various stages of disease. Furthermore, effective translation of biomarkers into the clinic will lead to effective implementation of personalized therapies that benefit patients with AIDs. In order to achieve this, well-designed, large-scale profiling studies should be designed for new biomarker discovery. Because extensive cellular and molecular profiling of human subjects generates vast amounts of disparate data, effective data management and integration solutions are essential for preserving this information in an interpretable form. Aside from ongoing technological improvements, data mining will play an essential role in generating large amounts of information and for providing the full potential of high throughput profiling approaches in patients with AIDs (34).
Systems biology approaches have been broadly applied in treating a variety of AIDs and have been well-suited for investigating molecular heterogeneity. Microarray technology for blood transcriptome analysis has provided new insight into the pathogenesis of complex diseases and the discovery of new biomarkers (56). To identify the most predictive biomarkers across DNA, RNA, protein, phenotype, and metabolites, more standardized multi-omics approaches, along with network analysis for large-scale data mining, are required. Along with ongoing technological improvement, carefully designed large-scale profiling studies should be adopted for better translation into clinical application.
Figures and Tables
Figure 1
Stages of autoimmune diseases. A variety of environmental factors and epigenetic changes in a genetically susceptible host trigger the initiation of autoimmune responses (trigger stage). The autoimmune response is activated by immune mediated attack against endogenous or exogenous self-antigens (preclinical stage). The duration of antigen exposure, impact of susceptible genes and/or epigenetic changes, and influence of effector function may dictate the phenotypic expression of AIDs (clinical stage).
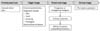
Figure 2
Biomarker discovery of a specific Th subset. T cells are differentiated into functionally distinct phenotypes, and the T cell subtypes are analyzed by complementary transcriptomics and functional proteomics using mass spectrometry or gel chromatography. When candidate genes are recognized, their role in cellular functions can be studied using RNA interference. The chromatin immunoprecipitation (ChIP) method is used to investigate the interaction between transcription factors and genomic DNA and to identify genes putatively regulated by these factors.
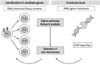
Figure 3
Systems biology approaches for AIDs in combination with multi-OMICs and network analysis. The multiple 'omics' (multi-omics) approaches (e.g., genomics, transcriptomics, proteomics, and metabolomics), in combination with bioinformatics and biostatistics, have made it possible to accelerate the discovery and development of specific biomarkers for AIDs. The combination of the biologic approaches, known as systems biology, provides a network analysis of post-transcriptional regulation and cellular regulatory circuits, includeing transcriptional networks, signal pathways, protein-protein interaction, and metabolic pathways, in each specific cell subset at different stages of AIDs.

ACKNOWLEDGEMENTS
This work was supported by Basic Science Research Program through the National Research Foundation (NRF) funded by the Ministry of Science, ICT & Future Planning (2013R1A1A2059368).
References
2. Blumberg RS, Dittel B, Hafler D, von Herrath M, Nestle FO. Unraveling the autoimmune translational research process layer by layer. Nat Med. 2012; 18:35–41.


3. Goldblatt F, O'Neill SG. Clinical aspects of autoimmune rheumatic diseases. Lancet. 2013; 382:797–808.


5. Nocturne G, Mariette X. Advances in understanding the pathogenesis of primary Sjogrens syndrome. Nat Rev Rheumatol. 2013; 9:544–556.


6. Harley JB, Sestak AL, Willis LG, Fu SM, Hansen JA, Reichlin M. A model for disease heterogeneity in systemic lupus erythematosus Relationships between histocompatibility antigens, autoantibodies, and lymphopenia or renal disease. Arthritis Rheum. 1989; 32:826–836.
7. Lessard CJ, Li H, Adrianto I, Ice JA, Rasmussen A, Grundahl KM, Kelly JA, Dozmorov MG, Miceli-Richard C, Bowman S, Lester S, Eriksson P, Eloranta ML, Brun JG, Gøransson LG, Harboe E, Guthridge JM, Kaufman KM, Kvarnström M, Jazebi H, CunninghameGraham DS, Grandits ME, Nazmul-Hossain AN, Patel K, Adler AJ, Maier-Moore JS, Farris AD, Brennan MT, Lessard JA, Chodosh J, Gopalakrishnan R, Hefner KS, Houston GD, Huang AJ, Hughes PJ, Lewis DM, Radfar L, Rohrer MD, Stone DU, Wren JD, Vyse TJ, Gaffney PM, James JA, Omdal R, Wahren-Herlenius M, Illei GG, Witte T, Jonsson R, Rischmueller M, Rönnblom L, Nordmark G, Ng WF; UK Primary Sjögren's Syndrome Registry, Mariette X, Anaya JM, Rhodus NL, Segal BM, Scofield RH, Montgomery CG, Harley JB, Sivils KL. Variants at multiple loci implicated in both innate and adaptive immune responses are associated with Sjogren's syndrome. Nat Genet. 2013; 45:1284–1292.


8. Viatte S, Plant D, Raychaudhuri S. Genetics and epigenetics of rheumatoid arthritis. Nat Rev Rheumatol. 2013; 9:141–153.


9. Zhebrun D, Kudryashova Y, Babenko A, Maslyansky A, Kunitskaya N, Popcova D, Klushina A, Grineva E, Kostareva A, Shlyakhto E. Association of PTPN22 1858T/T genotype with type 1 diabetes, Graves' disease but not with rheumatoid arthritis in Russian population. Aging. 2011; 3:368–373.


10. Hewagama A, Richardson B. The genetics and epigenetics of autoimmune diseases. J Autoimmun. 2009; 33:3–11.


11. Franke A, McGovern DP, Barrett JC, Wang K, Radford-Smith GL, Ahmad T, Lees CW, Balschun T, Lee J, Roberts R, Anderson CA, Bis JC, Bumpstead S, Ellinghaus D, Festen EM, Georges M, Green T, Haritunians T, Jostins L, Latiano A, Mathew CG, Montgomery GW, Prescott NJ, Raychaudhuri S, Rotter JI, Schumm P, Sharma Y, Simms LA, Taylor KD, Whiteman D, Wijmenga C, Baldassano RN, Barclay M, Bayless TM, Brand S, Büning C, Cohen A, Colombel JF, Cottone M, Stronati L, Denson T, De Vos M, D'Inca R, Dubinsky M, Edwards C, Florin T, Franchimont D, Gearry R, Glas J, Van Gossum A, Guthery SL, Halfvarson J, Verspaget HW, Hugot JP, Karban A, Laukens D, Lawrance I, Lemann M, Levine A, Libioulle C, Louis E, Mowat C, Newman W, Panés J, Phillips A, Proctor DD, Regueiro M, Russell R, Rutgeerts P, Sanderson J, Sans M, Seibold F, Steinhart AH, Stokkers PC, Torkvist L, Kullak-Ublick G, Wilson D, Walters T, Targan SR, Brant SR, Rioux JD, D'Amato M, Weersma RK, Kugathasan S, Griffiths AM, Mansfield JC, Vermeire S, Duerr RH, Silverberg MS, Satsangi J, Schreiber S, Cho JH, Annese V, Hakonarson H, Daly MJ, Parkes M. Genome-wide meta-analysis increases to 71 the number of confirmed Crohn's disease susceptibility loci. Nat Genet. 2010; 42:1118–1125.


12. Oksenberg JR, Baranzini SE, Sawcer S, Hauser SL. The genetics of multiple sclerosis: SNPs to pathways to pathogenesis. Nat Rev Genet. 2008; 9:516–526.


13. Duffin KC, Chandran V, Gladman DD, Krueger GG, Elder JT, Rahman P. Genetics of psoriasis and psoriatic arthritis: update and future direction. J Rheumatol. 2008; 35:1449–1453.
14. Stahl EA, Raychaudhuri S, Remmers EF, Xie G, Eyre S, Thomson BP, Li Y, Kurreeman FA, Zhernakova A, Hinks A, Guiducci C, Chen R, Alfredsson L, Amos CI, Ardlie KG; BIRAC Consortium, Barton A, Bowes J, Brouwer E, Burtt NP, Catanese JJ, Coblyn J, Coenen MJ, Costenbader KH, Criswell LA, Crusius JB, Cui J, de Bakker PI, De Jager PL, Ding B, Emery P, Flynn E, Harrison P, Hocking LJ, Huizinga TW, Kastner DL, Ke X, Lee AT, Liu X, Martin P, Morgan AW, Padyukov L, Posthumus MD, Radstake TR, Reid DM, Seielstad M, Seldin MF, Shadick NA, Steer S, Tak PP, Thomson W, van der Helm-van Mil AH, van der Horst-Bruinsma IE, van der Schoot CE, van Riel PL, Weinblatt ME, Wilson AG, Wolbink GJ, Wordsworth BP; YEAR Consortium, Wijmenga C, Karlson EW, Toes RE, de Vries N, Begovich AB, Worthington J, Siminovitch KA, Gregersen PK, Klareskog L, Plenge RM. Genome-wide association study meta-analysis identifies seven new rheumatoid arthritis risk loci. Nat Genet. 2010; 42:508–514.


15. Zhernakova A, Stahl EA, Trynka G, Raychaudhuri S, Festen EA, Franke L, Westra HJ, Fehrmann RS, Kurreeman FA, Thomson B, Gupta N, Romanos J, McManus R, Ryan AW, Turner G, Brouwer E, Posthumus MD, Remmers EF, Tucci F, Toes R, Grandone E, Mazzilli MC, Rybak A, Cukrowska B, Coenen MJ, Radstake TR, van Riel PL, Li Y, de Bakker PI, Gregersen PK, Worthington J, Siminovitch KA, Klareskog L, Huizinga TW, Wijmenga C, Plenge RM. Meta-analysis of genome-wide association studies in celiac disease and rheumatoid arthritis identifies fourteen non-HLA shared loci. PLoS Genet. 2011; 7:e1002004.


16. Han JW, Zheng HF, Cui Y, Sun LD, Ye DQ, Hu Z, Xu JH, Cai ZM, Huang W, Zhao GP, Xie HF, Fang H, Lu QJ, Xu JH, Li XP, Pan YF, Deng DQ, Zeng FQ, Ye ZZ, Zhang XY, Wang QW, Hao F, Ma L, Zuo XB, Zhou FS, Du WH, Cheng YL, Yang JQ, Shen SK, Li J, Sheng YJ, Zuo XX, Zhu WF, Gao F, Zhang PL, Guo Q, Li B, Gao M, Xiao FL, Quan C, Zhang C, Zhang Z, Zhu KJ, Li Y, Hu DY, Lu WS, Huang JL, Liu SX, Li H, Ren YQ, Wang ZX, Yang CJ, Wang PG, Zhou WM, Lv YM, Zhang AP, Zhang SQ, Lin D, Li Y, Low HQ, Shen M, Zhai ZF, Wang Y, Zhang FY, Yang S, Liu JJ, Zhang XJ. Genome-wide association study in a Chinese Han population identifies nine new susceptibility loci for systemic lupus erythematosus. Nat Genet. 2009; 41:1234–1237.


17. Barrett JC, Clayton DG, Concannon P, Akolkar B, Cooper JD, Erlich HA, Julier C, Morahan G, Nerup J, Nierras C, Plagnol V, Pociot F, Schuilenburg H, Smyth DJ, Stevens H, Todd JA, Walker NM, Rich SS. Type 1 Diabetes Genetics Consortium. Genome-wide association study and meta-analysis find that over 40 loci affect risk of type 1 diabetes. Nat Genet. 2009; 41:703–707.


18. Hu X, Kim H, Stahl E, Plenge R, Daly M, Raychaudhuri S. Integrating autoimmune risk loci with gene-expression data identifies specific pathogenic immune cell subsets. Am J Hum Genet. 2011; 89:496–506.


19. Ermann J, Fathman CG. Autoimmune diseases: genes, bugs and failed regulation. Nat Immunol. 2001; 2:759–761.


20. Jaenisch R, Bird A. Epigenetic regulation of gene expression: how the genome integrates intrinsic and environmental signals. Nat Genet. 2003; 33:Suppl. 245–254.


21. Jirtle RL, Skinner MK. Environmental epigenomics and disease susceptibility. Nat Rev Genet. 2007; 8:253–262.


22. Portela A, Esteller M. Epigenetic modifications and human disease. Nat Biotechnol. 2010; 28:1057–1068.


23. Sekigawa I, Kawasaki M, Ogasawara H, Kaneda K, Kaneko H, Takasaki Y, Ogawa H. DNA methylation: its contribution to systemic lupus erythematosus. Clin Exp Med. 2006; 6:99–106.


24. Quintero-Ronderos P, Montoya-Ortiz G. Epigenetics and autoimmune diseases. Autoimmune Dis. 2012; 2012:593720.


25. Pauley KM, Cha S, Chan EK. MicroRNA in autoimmunity and autoimmune diseases. J Autoimmun. 2009; 32:189–194.


26. Pascual V, Chaussabel D, Banchereau J. A genomic approach to human autoimmune diseases. Annu Rev Immunol. 2010; 28:535–571.


27. Blanco P, Palucka AK, Gill M, Pascual V, Banchereau J. Induction of dendritic cell differentiation by IFN-alpha in systemic lupus erythematosus. Science. 2001; 294:1540–1543.


28. Shodell M, Shah K, Siegal FP. Circulating human plasmacytoid dendritic cells are highly sensitive to corticosteroid administration. Lupus. 2003; 12:222–230.


29. Bennett L, Palucka AK, Arce E, Cantrell V, Borvak J, Banchereau J, Pascual V. Interferon and granulopoiesis signatures in systemic lupus erythematosus blood. J Exp Med. 2003; 197:711–723.


30. Vincent FB, Northcott M, Hoi A, Mackay F, Morand EF. Clinical associations of serum interleukin-17 in systemic lupus erythematosus. Arthritis Res Ther. 2013; 15:R97.


31. Krausgruber T, Blazek K, Smallie T, Alzabin S, Lockstone H, Sahgal N, Hussell T, Feldmann M, Udalova IA. IRF5 promotes inflammatory macrophage polarization and TH1-TH17 responses. Nat Iimmunol. 2011; 12:231–238.


32. Banchereau J, Pascual V. Type I interferon in systemic lupus erythematosus and other autoimmune diseases. Immunity. 2006; 25:383–392.


33. Nikula T, Mykkanen J, Simell O, Lahesmaa R. Genome-wide comparison of two RNA-stabilizing reagents for transcriptional profiling of peripheral blood. Transl Res. 2013; 161:181–188.


34. Chiche L, Jourde-Chiche N, Pascual V, Chaussabel D. Current perspectives on systems immunology approaches to rheumatic diseases. Arthritis Rheum. 2013; 65:1407–1417.


35. Croze E. Differential gene expression and translational approaches to identify biomarkers of interferon beta activity in multiple sclerosis. J Interferon Cytokine Res. 2010; 30:743–749.


36. Mohr S, Liew CC. The peripheral-blood transcriptome: new insights into disease and risk assessment. Trends Mol Med. 2007; 13:422–432.


37. Robinson WH, Steinman L, Utz PJ. Proteomics technologies for the study of autoimmune disease. Arthritis Rheum. 2002; 46:885–893.


38. Baechler EC, Batliwalla FM, Karypis G, Gaffney PM, Ortmann WA, Espe KJ, Shark KB, Grande WJ, Hughes KM, Kapur V, Gregersen PK, Behrens TW. Interferon-inducible gene expression signature in peripheral blood cells of patients with severe lupus. Proc Natl Acad Sci USA. 2003; 100:2610–2615.


39. Pascual V, Banchereau J, Palucka AK. The central role of dendritic cells and interferon-alpha in SLE. Curr Opin Rheumatol. 2003; 15:548–556.
40. Sigurdsson S, Nordmark G, Garnier S, Grundberg E, Kwan T, Nilsson O, Eloranta ML, Gunnarsson I, Svenungsson E, Sturfelt G, Bengtsson AA, Jönsen A, Truedsson L, Rantapää-Dahlqvist S, Eriksson C, Alm G, Göring HH, Pastinen T, Syvänen AC, Rönnblom L. A risk haplotype of STAT4 for systemic lupus erythematosus is over-expressed, correlates with anti-dsDNA and shows additive effects with two risk alleles of IRF5. Hum Mol Genet. 2008; 17:2868–2876.


41. Remmers EF, Plenge RM, Lee AT, Graham RR, Hom G, Behrens TW, de Bakker PI, Le JM, Lee HS, Batliwalla F, Li W, Masters SL, Booty MG, Carulli JP, Padyukov L, Alfredsson L, Klareskog L, Chen WV, Amos CI, Criswell LA, Seldin MF, Kastner DL, Gregersen PK. STAT4 and the risk of rheumatoid arthritis and systemic lupus erythematosus. N Engl J Med. 2007; 357:977–986.


42. Elkon KB, Stone VV. Type I interferon and systemic lupus erythematosus. J Interferon Cytokine Res. 2011; 31:803–812.


43. Stetson DB, Ko JS, Heidmann T, Medzhitov R. Trex1 prevents cell-intrinsic initiation of autoimmunity. Cell. 2008; 134:587–598.


44. Bauer JW, Petri M, Batliwalla FM, Koeuth T, Wilson J, Slattery C, Panoskaltsis-Mortari A, Gregersen PK, Behrens TW, Baechler EC. Interferon-regulated chemokines as biomarkers of systemic lupus erythematosus disease a validation study. Arthritis Rheum. 2009; 60:3098–3107.


45. Tektonidou MG, Ward MM. Validity of clinical associations of biomarkers in translational research studies: the case of systemic autoimmune diseases. Arthritis Res Ther. 2010; 12:R179.


46. Kussmann M, Raymond F, Affolter M. OMICS-driven biomarker discovery in nutrition and health. J Biotechnol. 2006; 124:758–787.


47. Plebani M, Pittoni M, Celadin M, Bernardi D, Mion MM. Recent advances in diagnostic technologies for autoimmune diseases. Autoimmun Rev. 2009; 8:238–243.


48. Kussmann M, Blum S. OMICS-derived targets for inflammatory gut disorders: opportunities for the development of nutrition related biomarkers. Endocr Metab Immune Disord Drug Targets. 2007; 7:271–287.


49. Delaleu N, Immervoll H, Cornelius J, Jonsson R. Biomarker profiles in serum and saliva of experimental Sjogren's syndrome: associations with specific autoimmune manifestations. Arthritis Res Ther. 2008; 10:R22.
50. Schweitzer B, Predki P, Snyder M. Microarrays to characterize protein interactions on a whole-proteome scale. Proteomics. 2003; 3:2190–2199.


51. Ciofani M, Madar A, Galan C, Sellars M, Mace K, Pauli F, Agarwal A, Huang W, Parkurst CN, Muratet M, Newberry KM, Meadows S, Greenfield A, Yang Y, Jain P, Kirigin FK, Birchmeier C, Wagner EF, Murphy KM, Myers RM, Bonneau R, Littman DR. A validated regulatory network for Th17 cell specification. Cell. 2012; 151:289–303.


52. Ivanov II, Zhou L, Littman DR. Transcriptional regulation of Th17 cell differentiation. Semin Immunol. 2007; 19:409–417.


53. Yosef N, Shalek AK, Gaublomme JT, Jin H, Lee Y, Awasthi A, Wu C, Karwacz K, Xiao S, Jorgolli M, Gennert D, Satija R, Shakya A, Lu DY, Trombetta JJ, Pillai MR, Ratcliffe PJ, Coleman ML, Bix M, Tantin D, Park H, Kuchroo VK, Regev A. Dynamic regulatory network controlling TH17 cell differentiation. Nature. 2013; 496:461–468.


54. Solt LA, Kumar N, Nuhant P, Wang Y, Lauer JL, Liu J, Istrate MA, Kamenecka TM, Roush WR, Vidović D, Schürer SC, Xu J, Wagoner G, Drew PD, Griffin PR, Burris TP. Suppression of TH17 differentiation and autoimmunity by a synthetic ROR ligand. Nature. 2011; 472:491–494.

