Abstract
Purpose
Lymphatic invasion (LI) is regarded as a predictor of the aggressiveness of ovarian cancer (OC). However, LI is not always the major determinant of long-term patient survival. To establish proper diagnosis and treatment for OC, we analyzed differentially expressed genes (DEGs) for patients with serous epithelial OC, with or without LI, who did or did not survive for 5 years.
Materials and Methods
Gene expression data from 63 patients with OC and LI, and 35 patients with OC but without LI, were investigated using an Affymetrix Human Genome U133 Array and analyzed using The Cancer Genome Atlas (TCGA) database. Among these 98 patients, 16 survived for 5 years or more. DEGs were identified using the Bioconductor R package, and their functions were analyzed using the DAVID web tool.
Results
We found 55 significant DEGs (p<0.01) from the patients with LI and 20 highly significant DEGs (p<0.001) from those without it. Pathway analysis showed that DEGs associated with carbohydrate metabolism or with renal cell carcinoma pathways were enriched in the patients with and without LI, respectively. Using the top five prognostic marker genes, we generated survival scores that could be used to predict the 5-year survival of patients with OC without LI.
According to data released by the Centers for Disease Control and Prevention, ovarian cancer (OC) was ranked the fifth cause of cancer-associated death in women in the USA,1 and 14270 cases of deaths from OC were reported in that country in 2014.2 Many prognostic methods have been evaluated in attempts to identify the most reliable diagnostic strategies for treating women with OC.3,4 Among these, tumor stage and the degree of lymphatic invasion (LI) have been considered to be determinants for overall survival.4,5,6 However, there is a need for better prognostic indicators, because the tumor stage merely indicates how the cancer cells have spread in the body but does not predict their response to chemotherapy.
The improvement of methods for analyzing a large set of gene expression data has provided useful insights for cancer treatment.7,8 Although there have been several relevant studies for OC,9 there have been only a limited number of gene expression studies to suggest a clinical subclassification associated with survival or treatment strategies. A recent study using The Cancer Genome Atlas (TCGA) described prognostic gene expression profiles in patients with OC.10 Among 193 suggested prognostic marker genes, only four common genes were validated in three different sets (p<0.05), and none of them was validated with more strict statistical criteria (p<0.01).10 This reflects the difficulty of finding representative marker genes for overall survival among patients with OC. Subgroup analysis may show either consistent or largely different results among different categories of patients.11 We hypothesized that prognostic indicators might vary between patients with and without LI, because different sets of co-regulated genes were induced with the activation of different pathways related to invasion.12 In this study, we divided patients with OC into subgroups with or without LI and analyzed those significantly differentially expressed genes (DEGs) that were linked with 5-year survival.
We analyzed data from 98 patients with serous OC using the TCGA datasets. Gene expression profiles were produced on an Affymetrix Human Genome U133 Array (Affymetrix, Inc., Santa Clara, CA, USA). Between 1995 and 2010, 489 clinically annotated patients with high-grade serous OC at tumor stages II–IV underwent surgery before systemic treatment. All patients received a platinum-based agent. Among 489 patients, the clinical annotation of 309 patients did not contain information on LI, and no information on 5-year survival was available for 82 patients. For patients with LI phenotypes described as 'lymphatic invasion' and 'lymphovascular invasion indicator' in the TCGA database, only 98 had LI, and for those we can trace possible 5-year survival, only 63 had LI and 35 did not. All patients were classified as having grade II or IV tumors. The patients were followed until 25 August 2010.
We performed microarray gene expression analysis for these patients using the TCGA database with Bioconductor R.13 Background adjustment was carried out employing the Robust Multi-Array Analysis algorithm from the 'affy' package for preprocessing, and the 'limma' package for differential expression analysis.
We identified significant DEGs between patients with and without 5-year survival and those with or without LI. The p-values were adjusted using the Benjamini-Hochberg method. Two-sided Student's t tests were applied, and statistical criteria of p<0.01 or p<0.001 were used as thresholds to determine the significance of any differences in gene expression.
Hierarchical clustering (HC) analysis was performed based on Pearson correlation coefficients in the expression pattern of genes. For gene clustering, we used 'pvclust' in the R package to assess any uncertainty of HC analysis.14 For each HC result, p-values were calculated using multiple bootstrap resampling.
We performed Gene Ontology annotations and Pathway Mapping of DEGs using the DAVID program.15 We used the Pathway category provided by DAVID for analysis of pathways that were overrepresented by three genes (EPAS1, WNT6, and PIK3R1) as 5-year survival-related DEGs for patients with OC without LI.
Statistical analyses were performed using the R package (above). Multiple linear regression analyses were considered in modeling predictions of the 5-year survival score by the expression of five marker genes among patients with serous OC without LI. The five marker genes were selected by a combination of pathway-associated DEGs and rank correlation analysis. Pearson's correlation coefficients were also calculated to assess the associations between five marker genes (WNT6, EPAS1, SWAP70, PIK3R1, and IGFBP2) and 5-year survival outcomes. Receiver operating characteristics (ROC) curves were analyzed using IBM SPSS Statistics (version 23, IBM Corp., Armonk, NY, USA).
In total, 180 patients (116 with and 64 without LI) were enrolled in the study using the TCGA database. Among the enrolled patients, 82 (53 with and 29 without LI) were excluded from the analysis because their follow-up period was less than 5 years. Finally, 98 patients (63 with and 35 without LI) were analyzed in this study (Table 1).
We divided these 98 patients into two groups: those who survived for 5 years and those who did not. We identified 133 DEGs (Supplementary Table 1 and 2, only online) between the survival and nonsurvival groups. As shown in the heat map in Fig. 1A, clustering of these DEGs could not clearly distinguish the survival from the nonsurvival groups.
We tried to identify DEGs in subgroups of respective OCs with and without LI. As shown in the heat map (Fig. 1B), 55 DEGs (Supplementary Table 1 and 3, only online) identified from the patients with LI could fairly well divide the survival from the nonsurvival groups. However, measurements of Manhattan distance and Pvclust dendrograms indicated that the patients with LI could not be clearly divided into survival and nonsurvival groups by the expression pattern of these 55 DEGs (data not shown).
We conducted the same analysis for 35 patients with OC without LI, and 20 DEGs were identified (Table 2, Supplementary Table 1, only online). The heat map (Fig. 1C) showed an obvious difference in the expression patterns between the survival and nonsurvival groups. Results from a Manhattan distance plot (Fig. 2A) and HC dendrogram (Fig. 2B) confirmed that patients without LI could be clearly classified into 5-year survival and nonsurvival groups by these DEGs.
Pathway analysis showed that among the 20 DEGs, EPAS1, WNT6, and PIK3R1 were associated with cancer and renal cell carcinoma pathways (Table 3). To gain further insight, we selected five genes (WNT6, EPAS1, SWAP70, PIK3R1, and IGFBP2) among 20 DEGs whose expression showed strong correlation with the 5-year survival pattern. After normalization to the expression of five housekeeping genes (ACTB, GAPDH, RPLP0, GUSB, and TFRC) found in both tumor and normal tissues in equivalent amounts, we formulated one equation to predict the 5-year survival scores for five marker genes using multiple linear regression analysis as follows.
Using H as the mean expression value of the five housekeeping genes, the following factors were derived.
As shown in Fig. 3, all six patients who survived for 5 years showed positive score values, while all 27 nonsurviving patients showed negative values, based on this equation. When comparing the ROC curve estimates, we found high precision for our formula in estimating patient survival (Supplementary Fig. 1, only online)
LI has been considered an important predictive component for many cancers. However, our TCGA-based analysis showed that LI alone could not efficiently predict the 5-year survival of these patients with OC. If we could find prognostic markers for the OCs, we could then select patients who would gain an advantage from adjuvant therapy. Therefore, we used expression profiles to detect prognostic markers that could predict the 5-year survival rates, and found that information about the presence of LI alone was not sufficient, but it still affected the efficiency of prediction using gene expression profiling. The clustering of survival and nonsurvival groups from all patients with OC using DEGs was not as clear as the subgroups with or without LI, suggesting that the cancers from each subgroup might have had a different pathophysiology that influenced the survival of the patients. Most previous research on OC has focused on LI, however, none of these studies have attempted to separately analyze the factors influencing survival between patients with or without LI. To our best knowledge, the present study is the first to use OC samples to analyze survival by subgroup with or without LI.
The subgroup of patients without LI could be divided into 5-year survival and nonsurvival groups using the 20 DEGs more clearly and significantly than among all patients with OC or subgroups with LI. The survival score values using five prognostic marker genes (WNT6, EPAS1, SWAP70, PIK3R1, and IGFBP2) among 20 DEGs could efficiently predict the 5-year survival of patients with OC but no LI. Patients with low expression levels of three genes (PIK3R1, EPAS1, and SWAP70) and high expression of two genes (WNT6 and IGFBP2) showed longer survival.
PIK3R1 encodes the 85 kDa regulatory subunit of phosphatidylinositol 3-kinase, which is an important player in cancer development and progression. The PI3K/AKT/mTOR signaling pathway is frequently activated and has been considered as a possible therapeutic target for OC.16,17 A recent meta-analysis showed that high PI3K levels were associated with poor survival in patients with epithelial OC.18 Lower expression of PIK3R1 might lead to downregulation of the PI3K/AKT/mTOR pathway, thereby contributing to the increased survival of patients with OC.
EPAS1, also known as HIF2A, encodes a transcription factor and is induced by hypoxic stress. There have been many publications about the link between hypoxia and cancers. Thus, the level of expression of EPAS1 and genes of its downstream signaling pathways affect the aggressiveness of OC;19 higher expressions of those genes were significantly correlated with poor prognosis, similar to our findings. Better adaption to the hypoxic microenvironment common in solid tumors might explain those findings.
SWAP70 is expressed in various cell types, including activated B-lymphocytes and mast cells.20 Although its role in cancer has not been reported frequently, one recent study showed increased expression of SWAP70 in malignant gliomas and a strong correlation between high expression and poor patient survival.21 Our data also showed that its expression was upregulated in poorly surviving patients with OC but no LI, suggesting a similar mechanism.
WNT6 is a member of the WNT family of genes, which encode highly conserved glycoproteins secreted during embryonic development. The WNT signaling pathway is altered in many types of cancers and is regarded as a good candidate for cancer therapy.21 WNT family members have been generally regarded as poor prognostic factors for patients with cancer because of their effects on cell proliferation, migration, and survival.22 However, there is also a report that elevated levels of the Wnt-5a protein was associated with better outcomes for patients with prostate cancer, similar to our findings.23
IGFBP2 encodes one of the members of the insulin-like growth factor binding (IGFBP) proteins, and its oncogene-like action by activation of Akt signaling has been reported.24 There have been contradictory reports about the prognostic value of circulating and intratumorous IGFBP2 levels.25 A recent report showed that expression of IGFBP2 was associated with better survival in a specific group (body mass index ≤25 kg/m2) of patients with breast cancer.26 The above and our present findings suggest that the prognostic efficacy of gene expression patterns can be useful in specific subclasses of patients, however, further validation studies are needed. Our results suggest that the expression level of these five marker genes might be useful for deciding a prognosis for patients with OC and in making decisions on adjuvant hormonal therapy.
There were not enough data on patients that contained both LI and 5-year survival rates within the open serous OC dataset. Nonetheless, validation of the equation using an independent cohort can be done in the future. We believe that this topic is worthy of future studies, and that our results would be helpful for researchers as well as clinicians in the OC field.
Figures and Tables
Fig. 1
Heat map of gene expression profiles from patients with OC. The rows represent genes, and columns represent individual patients. Red indicates a high, and green indicates a low expression level. Differentially expressed genes between 5-year survival and nonsurvival groups were selected from all patients with OC (p<0.01) (A), patients with lymphatic invasion (p<0.01) (B), and patients without lymphatic invasion (p<0.001) (C). OC, ovarian cancer.
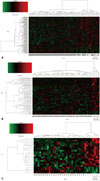
Fig. 2
Clustering of 5-year survival and nonsurvival groups of patients with OC without lymphatic invasion. (A) Manhattan distance plot of gene expression profiles in 20 survival-related genes and their association with patients without lymphatic invasion. (B) Cluster dendrogram of gene expression profiles and their association with patients without lymphatic invasion. OC, ovarian cancer.
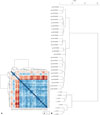
Fig. 3
Survival score values calculated by multiple linear regression analysis with the five prognostic marker genes. The Y-axis indicates the score values; the 5-year survival group showed positive values, while the nonsurvival group showed negative values.
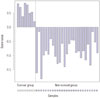
Table 1
TCGA Data for Patients with Ovarian Cancer, with or without Lymphatic Invasion, in Terms of 5-Year Survival Outcomes
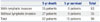
5 yr death | 5 yr survival | Total | |
---|---|---|---|
With lymphatic invasion | 55 patients | 8 patients | 63 |
Without lymphatic invasion | 27 patients | 8 patients | 35 |
Total | 82 patients | 16 patients | 98 |
Table 2
DEG List for Patients with Ovarian Cancer, without Lymphatic Invasion
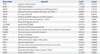
Table 3
Pathway Analysis of DEGs in Patients with Ovarian Cancer, without Lymphatic Invasion

ACKNOWLEDGEMENTS
This study was supported by the Research Grant from Seoul National University Hospital (No. 04-2009-0780). This research was supported by a grant of the Korean Health Technology R&D Project through the Korea Health Industry Development Institute (KHIDI), funded by the Ministry of Health & Welfare, Republic of Korea (grant number: HI13C2148). The study resources were provided by TCGA.
References
1. Centers for Disease Control and Prevention, National Cancer Institute. United States Cancer Statistics (USCS): 1999-2012 cancer incidence and mortality data. accessed on 2015 July 31. Available at: http://www.cdc.gov/uscs.
3. Bast RC Jr, Hennessy B, Mills GB. The biology of ovarian cancer: new opportunities for translation. Nat Rev Cancer. 2009; 9:415–428.


4. Holschneider CH, Berek JS. Ovarian cancer: epidemiology, biology, and prognostic factors. Semin Surg Oncol. 2000; 19:3–10.


5. Matsuo K, Yoshino K, Hiramatsu K, Banzai C, Hasegawa K, Yasuda M, et al. Effect of lymphovascular space invasion on survival of stage I epithelial ovarian cancer. Obstet Gynecol. 2014; 123:957–965.


6. Matsuo K, Sheridan TB, Yoshino K, Miyake T, Hew KE, Im DD, et al. Significance of lymphovascular space invasion in epithelial ovarian cancer. Cancer Med. 2012; 1:156–164.


7. van‘t Veer LJ, Dai H, van de, He YD, Hart AA, Mao M, et al. Gene expression profiling predicts clinical outcome of breast cancer. Nature. 2002; 415:530–536.


8. Setlur SR, Mertz KD, Hoshida Y, Demichelis F, Lupien M, Perner S, et al. Estrogen-dependent signaling in a molecularly distinct subclass of aggressive prostate cancer. J Natl Cancer Inst. 2008; 100:815–825.


9. Mok SC, Chao J, Skates S, Wong K, Yiu GK, Muto MG, et al. Prostasin, a potential serum marker for ovarian cancer: identification through microarray technology. J Natl Cancer Inst. 2001; 93:1458–1464.


10. Cancer Genome Atlas Research Network. Integrated genomic analyses of ovarian carcinoma. Nature. 2011; 474:609–615.
11. Dijkman B, Kooistra B, Bhandari M. Evidence-Based Surgery Working Group. How to work with a subgroup analysis. Can J Surg. 2009; 52:515–522.
12. Kamai T, Tsujii T, Arai K, Takagi K, Asami H, Ito Y, et al. Significant association of Rho/ROCK pathway with invasion and metastasis of bladder cancer. Clin Cancer Res. 2003; 9:2632–2641.
13. Reimers M, Carey VJ. Bioconductor: an open source framework for bioinformatics and computational biology. Methods Enzymol. 2006; 411:119–134.
14. Suzuki R, Shimodaira H. Pvclust: an R package for assessing the uncertainty in hierarchical clustering. Bioinformatics. 2006; 22:1540–1542.


15. Huang DW, Sherman BT, Tan Q, Collins JR, Alvord WG, Roayaei J, et al. The DAVID Gene Functional Classification Tool: a novel biological module-centric algorithm to functionally analyze large gene lists. Genome Biol. 2007; 8:R183.


16. Li H, Zeng J, Shen K. PI3K/AKT/mTOR signaling pathway as a therapeutic target for ovarian cancer. Arch Gynecol Obstet. 2014; 290:1067–1078.


17. Eskander RN, Tewari KS. Exploiting the therapeutic potential of the PI3K-AKT-mTOR pathway in enriched populations of gynecologic malignancies. Expert Rev Clin Pharmacol. 2014; 7:847–858.


18. Cai J, Xu L, Tang H, Yang Q, Yi X, Fang Y, et al. The role of the PTEN/PI3K/Akt pathway on prognosis in epithelial ovarian cancer: a meta-analysis. Oncologist. 2014; 19:528–535.


19. Raspaglio G, Petrillo M, Martinelli E, Li Puma DD, Mariani M, De Donato M, et al. Sox9 and Hif-2α regulate TUBB3 gene expression and affect ovarian cancer aggressiveness. Gene. 2014; 542:173–181.


20. Seol HJ, Smith CA, Salhia B, Rutka JT. The guanine nucleotide exchange factor SWAP-70 modulates the migration and invasiveness of human malignant glioma cells. Transl Oncol. 2009; 2:300–309.


22. Huang CL, Liu D, Nakano J, Ishikawa S, Kontani K, Yokomise H, et al. Wnt5a expression is associated with the tumor proliferation and the stromal vascular endothelial growth factor--an expression in non-small-cell lung cancer. J Clin Oncol. 2005; 23:8765–8773.


23. Syed Khaja AS, Helczynski L, Edsjö A, Ehrnström R, Lindgren A, Ulmert D, et al. Elevated level of Wnt5a protein in localized prostate cancer tissue is associated with better outcome. PLoS One. 2011; 6:e26539.


24. Dunlap SM, Celestino J, Wang H, Jiang R, Holland EC, Fuller GN, et al. Insulin-like growth factor binding protein 2 promotes glioma development and progression. Proc Natl Acad Sci U S A. 2007; 104:11736–11741.


Supplementary Material
Supplementary Fig. 1
ROC curve of survival for patients with ovarian cancer predicted by five marker genes and the result of the equation used for calculating risk. All values were log-transformed and divided by the average expression level of five housekeeping genes. ROC, receiver operating characteristics.