Abstract
Purpose
Materials and Methods
Results
Figures and Tables
Fig. 1
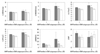
Table 1
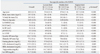
M, male; F, female; ln, natural logarithm; SDNN, standard deviation of all normal RR intervals; RMSSD, root mean square of successive differences; LF, low frequency power; HF, high frequency power; pNN50, percentage of differences between adjacent RR intervals that are greater than 50 ms; LF/HF, ratio of the low/high frequency power; BP, blood pressure; LDL, low density lipoprotein; HDL, high density lipoprotein; hs-CRP, high sensitivity C-reactive protein.
Values are presented as mean (SD). To convert to SI units, multiply by 0.0259 for LDL and HDL cholesterol (mmol/L) and by 0.0113 for triglycerides (mmol/L).
*Analyzed using linear regression test.
Table 2

ln, natural logarithm; SDNN, standard deviation of all normal RR intervals; RMSSD, root mean square of successive differences; LF, low frequency power; HF, high frequency power; pNN50, percentage of differences between adjacent RR intervals that are greater than 50 ms; LF/HF, ratio of the low/high frequency power.
*p<0.01, Spearman correlation between each adiposity measure and HRV index.
†p<0.05, comparison of two correlation coefficients (% body fat mass vs. body mass index, or waist-to-hip ratio vs. body mass index).
‡p<0.05, comparison of two correlation coefficients (% body fat mass vs. waist-to-hip ratio).
Table 3
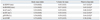
ln, natural logarithm; SDNN, standard deviation of all normal RR intervals; RMSSD, root mean square of successive differences; LF, low frequency power; HF, high frequency power; pNN50, percentage of differences between adjacent RR intervals that are greater than 50 ms; LF/HF, ratio of the low/high frequency power; ROC, receiver operating characteristics.
Values are presented as area under the curve (standard error) to predict low HRV (<15 percentile vs. ≥15 percentile of each HRV index.
*p<0.05 using pair-wise comparison of ROC curves analysis for the difference of area under the curve between waist-to-hip ratio and other adiposity measures.
Table 4
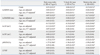
ln, natural logarithm; SDNN, standard deviation of all normal RR intervals; RMSSD, root mean square of successive differences; LF, low frequency power; HF, high frequency power; pNN50, percentage of differences between adjacent RR intervals that are greater than 50 ms; LF/HF, ratio of the low/high frequency power; CV, cardiovascular.
Values are regression coefficient (standard error) for HRV measures with adiposity measures (per 1 standard deviation change) in multiple linear regression model.
*p<0.05.
†CV included high blood pressure (<130/85 mm Hg vs. ≥130/85 mm Hg), fasting glucose, low density lipoprotein cholesterol, high density lipoprotein cholesterol, triglycerides, and high sensitivity C-reactive protein.
ACKNOWLEDGEMENTS
References



















