Abstract
Objective
To compare the diagnostic performance of readout-segmented echo-planar imaging (RS-EPI)-based diffusion kurtosis imaging (DKI) and that of diffusion-weighted imaging (DWI) for differentiating malignant from benign masses in head and neck region.
Materials and Methods
Between December 2014 and April 2016, we retrospectively enrolled 72 consecutive patients with head and neck masses who had undergone RS-EPI-based DKI scan (b value of 0, 500, 1000, and 1500 s/mm2) for pretreatment evaluation. Imaging data were post-processed by using monoexponential and diffusion kurtosis (DK) model for quantitation of apparent diffusion coefficient (ADC), apparent diffusion for Gaussian distribution (Dapp), and apparent kurtosis coefficient (Kapp). Unpaired t test and Mann-Whitney U test were used to compare differences of quantitative parameters between malignant and benign groups. Receiver operating characteristic curve analyses were performed to determine and compare the diagnostic ability of quantitative parameters in predicting malignancy.
Results
Malignant group demonstrated significantly lower ADC (0.754 ± 0.167 vs. 1.222 ± 0.420, p < 0.001) and Dapp (1.029 ± 0.226 vs. 1.640 ± 0.445, p < 0.001) while higher Kapp (1.344 ± 0.309 vs. 0.715 ± 0.249, p < 0.001) than benign group. Using a combination of Dapp and Kapp as diagnostic index, significantly better differentiating performance was achieved than using ADC alone (area under curve: 0.956 vs. 0.876, p = 0.042).
Head and neck masses represent a wide spectrum of malignant and benign lesions. Accurate pre-treatment differentiation is crucial for determination of individual treatment plan (1). Differentiation work based on clinical information alone is insufficient due to remarkable overlap between malignant and benign lesions (12). Conventional computed tomography and magnetic resonance imaging (MRI) are extensively used to delineate head and neck masses. Several imaging features such as adjacent structure invasion, perineural spread, and presence of necrosis have been proven to be helpful in predicting the biological behavior of head and neck masses (34). However, interpretation of imaging features is a subjective process that can result in remarkable inter-reader variability. Therefore, an objective and useful method that is helpful in predicting head and neck malignancy is needed (5).
Diffusion-weighted imaging (DWI) and apparent diffusion coefficient (ADC) value derived from DWI have been commonly used to distinguish malignancy from benignity in head and neck region (5678). Due to high cellularity and limited extracellular space, malignant tumors usually demonstrate hyperintensity on DWI with low ADC values (678). The traditional theory of DWI assumes that the diffusion pattern of water molecules has Gaussian distribution. However, it has been reported that the diffusion pattern of water molecules tends to deviate from a Gaussian distribution (referred to as non-Gaussian distribution) due to the existence of barriers and compartments (e.g., cell membranes, intracellular and extracellular spaces) (9).
As an emerging imaging technique, diffusion kurtosis imaging (DKI) and quantitative metrics derived from DKI have been proven to be useful for quantifying the degree of non-Gaussian distribution. These derived metrics have been proven to be useful for providing more accurate characterization of water diffusion than that of ADC in many tissues (10111213), including head and neck region (141516,17). However, in previous studies, DWI and DKI were usually performed using single-shot echo-planar imaging (SS-EPI) which is prone to image artifacts (18). By contrast, readout-segmented echo-planar imaging (RS-EPI) technique has been proven to be superior in reducing ghosting artifacts, image blurring, and geometric distortions of diffusion associated imaging scan (1819). However, no study has reported the use of RS-EPI-based DKI for differentiating malignant from benign head and neck masses. Therefore, the purpose of this study was to evaluate the diagnostic performance of RS-EPI-based DKI in differentiating malignant from benign masses in head and neck region compared to that of DWI.
This retrospective study was approved by the Institutional Review Board of our hospital. The requirement of informed consent was waived. Retrospective review of our hospital database identified 111 consecutive patients with histologically confirmed head and neck masses between December 2014 and April 2016. Inclusion criteria were: 1) RS-EPI-based DKI scan was performed before biopsy or any other treatment; 2) the short-axis diameter of the mass was (Table 1) more than 1-cm; and 3) the image quality was adequate. Thirty-nine patients were excluded due to following reasons: 1) lack of DKI (n = 21); 2) biopsy or radiotherapy was administered before magnetic resonance (MR) scan (n = 10); 3) the short-axis diameter of the mass was less than 1-cm (n = 6); and 4) inadequate imaging quality with obvious susceptibility or motion artifacts (n = 2).
Finally, 72 consecutive patients (46 males and 26 females; mean age, 54.1 ± 15.0 years; range, 12–88 years) were enrolled in this study. Among these 72 patients, 16 had benign masses (4 males and 12 females; mean age, 49.1 ± 12.3 years; range, 14–67 years) and 56 had malignant masses (42 males and 14 females; mean age, 55.6 ± 15.5 years; range, 12–88 years). Detailed clinical characteristics and histological information of these included patients are summarized in Table 1.
All MRI examinations were performed with a 3T MR scanner (MAGNETOM Verio, Siemens Healthineers, Erlangen, Germany) using a 12-channel head and neck coil. Conventional MRI scan ranged from the skull base to the suprasternal notch with the following sequence: 1) axial fat-suppressed T2-weighted image (repetition time [TR]/echo time [TE] = 5930/95 ms; slice number = 40; slice thickness = 4 mm without gap; field of view [FOV] = 230 mm; matrix = 384 × 384); 2) axial T1-weighted image (TR/TE, 1460/10 ms; slice number = 40; slice thickness = 4 mm without gap; FOV = 230 mm; matrix = 384 × 384); 3) coronal fat-suppressed T2-weighted image (TR/TE = 4500/79 ms; slice number = 20; slice thickness = 4 mm without gap; FOV = 240 mm; matrix = 384 × 384); 4) coronal T1-weighted image (TR/TE = 712/9.5 ms; slice number = 20; slice thickness = 4 mm without gap; FOV = 240 mm; matrix = 384 × 384); 5) sagittal fat-suppressed T2-weighted image (TR/TE = 4200/79 ms; slice number = 20; slice thickness = 4 mm without gap; FOV = 240 mm; matrix = 384 × 384); and 6) sagittal T1-weighted image (TR/TE = 350/2.5 ms; slice number = 20; slice thickness = 4 mm without gap; FOV = 240 mm; matrix = 384 × 384). For post-contrast MR scan, gadolinium-diethylene triamine pentaacetic acid (Magnevist, Bayer AG, Berlin, Germany) as contrast agent was intravenously bolus injected at a dose of 0.1 mmol/kg of body weight followed by a 20 mL bolus injection of saline. After injecting the contrast, axial, sagittal, and coronal T1-weighted images were acquired. Total scan time of conventional MRI was about 18.5 minutes.
Readout-segmented echo-planar imaging sequence was used for DKI. Imaging parameters were consistent as follows: b values = 0, 500, 1000, and 1500 s/mm2; orthogonal directions = 3; diffusion schema = Stejskal-Tanner; Fat suppression = frequency selective; TR/TE = 4300/74 ms; slice number = 13; number of excitations = 1; FOV = 230 mm; slice thickness = 4 mm without gap; matrix = 224 × 224; phase-encoding direction = anteroposterior; echo-spacing = 0.4 ms; and number of readout segments = 5. Total acquisition time of DKI was 4 minutes 32 seconds.
Imaging data were post-processed offline with an inhouse software using monoexponential and diffusion kurtosis (DK) model. Imaging source data of 2 b values (0 and 1000 s/m2) were processed using monoexponential model to measure ADC while data of 4 b values (0, 500, 1000, and 1500 s/mm2) were processed using DK model to measure DKI-derived parameters.
For the monoexponential model, the relationship between signal intensity of DWI and b factors can be expressed by:
For the DK model, the relationship between signal intensity of DKI and b factors can be expressed by:
where S is signal intensity, b is b value (s/mm2), ADC is apparent diffusion coefficient, Dapp is apparent diffusion for Gaussian distribution, and Kapp is apparent kurtosis coefficient (a dimension-less parameter). Pixel-by-pixel maps of diffusion features (ADC, Dapp, and Kapp) were then automatically constructed.
Based on DK images (b = 1000 s/mm2), regions of interest (ROIs) were manually drawn on each slice to encompass the whole mass with reference to T2-weighted and contrast enhanced T1-weighted images. Surrounding blood vessels and large areas with cystic, necrotic, and hemorrhagic components were excluded. ROIs were slightly smaller in size than actual tumor sizes to reduce the influence of partial volume effect.
Regions of interest were placed individually by two radiologists (5 and 4 years of clinical experience in head and neck radiology for reader 1 and reader 2, respectively). Both radiologists were blinded to the study design and final diagnosis. Measurement results of these two radiologists were used to assess inter-reader reproducibility. The average value of measurement results from the two was used in further statistical analyses.
All numeric data are reported as mean ± standard deviation. Normality of quantitative parameters was evaluated with Kolmogorov-Smirnov's test. If data were normally distributed (patient age and Kapp), they were compared using unpaired t test. If they were not normally distributed (ADC and Dapp), they were compared using Mann-Whitney U test. Chi-square test was used to compare the difference in gender proportion between the two groups. Receiver operating characteristic (ROC) curve analyses were performed to determine the diagnostic value of significant parameters in predicting head and neck malignancy (20). Areas under ROC curves (AUCs) were compared using published method (21). Intra-class correlation coefficient (ICC) with 95% confidence interval and Bland-Altman method were used to evaluate the inter-reader agreement of quantitative measurements. ICC was interpreted as poor when it was < 0.40, moderate if it was 0.41– –0.60, good if it was 0.61– –0.80, and excellent if it was ≥ 0.81. A two-sided p value of less than 0.05 was considered statistically significant. Statistical analysis was carried out using statistical packages SPSS version 23.0 (IBM Corp., Armonk, NY, USA) and MedCalc (MedCalc, Mariakerke, Belgium).
Significant (p < 0.001) difference in sex distribution was found between benign and malignant groups. However, there was no significant (p = 0.129) difference in age distribution between the two groups. Results of comparison for DWI and DKI derived parameters between malignant and benign groups are summarized in Table 2. ADC and Dapp of malignant masses were all significantly lower than those of benign mimics, although Kapp of malignancy was significantly higher than that of benignity (all p < 0.001) (Fig. 1). Representative cases of adenocarcinoma and pleomorphic adenoma in the parotid gland are shown in Figures 2 and 3, respectively.
Table 3 summarizes detailed diagnostic performance of significant imaging parameters in differentiating malignant from benign head and neck masses. ROC analyses indicated that, when a combination of Dapp and Kapp was used as diagnostic index, optimal diagnostic performance in predicting malignant head and neck masses was obtained (AUC, 0.959; sensitivity, 82.1%; specificity, 100.0%). This was significantly (p = 0.042) better than using ADC alone (Fig. 4).
Using a combination of Dapp and Kapp as differentiating criterion, there were 11 false negative cases (one patient with adenocarcinoma in the parotid gland, ten patients with squamous cell carcinomas in the tongue, nasopharynx, or paranasal sinus). However, there were no false positive cases.
Excellent inter-reader agreements were achieved for quantitative measurements of ADC, Dapp, and Kapp (ICC: 0.942 vs. 0.989 vs. 0.984). Results of Bland-Altman analysis for repeated measurements are shown in Figure 5.
Our study revealed several important findings. First, malignant masses showed lower ADC and Dapp values than benign mimics. This might be related to hypercellularity and limited extracellular space of malignant masses (678). Second, the Kapp of malignancy was higher than that of benignity. Third, compared to ADC alone, a combination of Dapp and Kapp demonstrated better diagnostic performance in differentiating malignant from benign masses in head and neck region.
In agreement with previous studies (5678101122), malignant masses showed significantly lower ADC and Dapp values than benign mimics. This could be explained by the hyper-cellularity, enlarged nuclei, and reduced extracellular space in malignant tumors. We also found that Kapp values of malignant masses were significantly higher than those of benign mimics. Similar findings have also been reported in previously studies on cerebral glioma, prostate cancer, and so on (23242526). Roethke et al. (23) have found that the Kapp value is significantly lower in peripheral zone prostate cancer compared with that in control normal region. Jiang et al. (24) have found that the kurtosis value is increased as the grade of glioma is increased. They indicated that it might be associated with increased heterogeneity in high grade gliomas. Previous studies have also indicated that Kapp is proportional to the heterogeneity and complexity of microstructure of tumors (232425). Therefore, malignant masses would show higher Kapp than benign mimics. However, the most common histologic type of malignant tumors in our study was squamous cell carcinoma with features of scarce amounts of necrotic tissue. This might lead to substantially increased Kapp. This potential bias might overestimate the diagnostic accuracy for malignancy. Further study with larger cohort and more histological compositions is needed to clarify the value of DKI in such differentiation work.
Compared to conventional ADC value, a combination of Dapp and Kapp demonstrated better diagnostic performance for differentiating malignant from benign masses in this study. Compared to the assumption of Gaussian distribution in monoexponential diffusion weighted (DW) model, the non-Gaussian distribution assumed in DK model might be more accurate considering complex and heterogeneous cellular microstructure in both benign and malignant tissues. DKI could provide additional information related to tumor heterogeneity for equivocal cases. Therefore, DKI could improve the diagnostic performance.
Currently, DWI is commonly performed based on SS-EPI. However, SS-EPI is prone to image artifacts and distortion. As an alternative approach, RS-EPI technique has been applied more and more in DWI related studies (18192728). RS-EPI has been proven to be an effective technique that can significantly improve image quality by reducing susceptibility artifacts, distortion, and blurring compared to SS-EPI (2728). Therefore, we used RS-EPI technique for DKI scan in our study. We believe that prominent improvement in image quality is beneficial for ensuring the accuracy and credibility of quantitative measurements in the present study.
This study has some limitations. First, it was a retrospective study in a single center with a relatively small sample size. However, our results could be used as a base for future larger-scale prospective studies. Second, pathological conditions of subjects in our study varied widely, especially for those in the benign group while almost all cases in the malignant group were squamous cell carcinomas. Case series bias might influence statistical power and application of the derived threshold value of Dapp and Kapp. Third, due to above-mentioned limited sample size and cases series bias, some crucial subgroup differentiation among different malignant masses could not be performed, such as differential diagnosis between lymphoma and squamous cell carcinoma. Further study with a larger cohort is needed to clarity the ability of DKI in such subgroup differentiation work.
In conclusion, RS-EPI-based DKI was feasible for differentiating malignant from benign masses in head and neck region. Besides, DKI could provide additional information associated with tumor heterogeneity. Compared to ADC alone, a combination of Dapp and Kapp demonstrated better diagnostic performance. Thus, DKI could be used as a promising imaging biomarker for discriminating malignant from benign masses in head and neck region.
References
1. Lewis-Jones H, Colley S, Gibson D. Imaging in head and neck cancer: United Kingdom national multidisciplinary guidelines. J Laryngol Otol. 2016; 130:S28–S31. PMID: 27841111.


2. Wippold FJ 2nd. Head and neck imaging: the role of CT and MRI. J Magn Reson Imaging. 2007; 25:453–465. PMID: 17279529.


3. Serifoğlu Ì, Oz ÌÌ, Damar M, Tokgöz Ö, Yazgan Ö, Erdem Z. Diffusion-weighted imaging in the head and neck region: usefulness of apparent diffusion coefficient values for characterization of lesions. Diagn Interv Radiol. 2015; 21:208–214. PMID: 25910284.


4. Schafer J, Srinivasan A, Mukherji S. Diffusion magnetic resonance imaging in the head and neck. Magn Reson Imaging Clin N Am. 2011; 19:55–67. PMID: 21129635.


5. Srinivasan A, Dvorak R, Perni K, Rohrer S, Mukherji SK. Differentiation of benign and malignant pathology in the head and neck using 3T apparent diffusion coefficient values: early experience. AJNR Am J Neuroradiol. 2008; 29:40–44. PMID: 17921228.


6. Ginat DT, Mangla R, Yeaney G, Johnson M, Ekholm S. Diffusion-weighted imaging for differentiating benign from malignant skull lesions and correlation with cell density. AJR Am J Roentgenol. 2012; 198:W597–W601. PMID: 22623576.


7. Thoeny HC, De Keyzer F, King AD. Diffusion-weighted MR imaging in the head and neck. Radiology. 2012; 263:19–32. PMID: 22438440.


8. Xu XQ, Hu H, Su GY, Liu H, Hong XN, Shi HB, et al. Utility of histogram analysis of ADC maps for differentiating orbital tumors. Diagn Interv Radiol. 2016; 22:161–167. PMID: 26829400.


9. Jensen JH, Helpern JA. MRI quantification of non-Gaussian water diffusion by kurtosis analysis. NMR Biomed. 2010; 23:698–710. PMID: 20632416.


10. Sun K, Chen X, Chai W, Fei X, Fu C, Yan X, et al. Breast cancer: diffusion kurtosis MR imaging-diagnostic accuracy and correlation with clinical-pathologic factors. Radiology. 2015; 277:46–55. PMID: 25938679.


11. Nogueira L, Brandão S, Matos E, Nunes RG, Loureiro J, Ramos I, et al. Application of the diffusion kurtosis model for the study of breast lesions. Eur Radiol. 2014; 24:1197–1203. PMID: 24658871.


12. Rosenkrantz AB, Sigmund EE, Winnick A, Niver BE, Spieler B, Morgan GR, et al. Assessment of hepatocellular carcinoma using apparent diffusion coefficient and diffusion kurtosis indices: preliminary experience in fresh liver explants. Magn Reson Imaging. 2012; 30:1534–1540. PMID: 22819175.


13. Rosenkrantz AB, Sigmund EE, Johnson G, Babb JS, Mussi TC, Melamed J, et al. Prostate cancer: feasibility and preliminary experience of a diffusional kurtosis model for detection and assessment of aggressiveness of peripheral zone cancer. Radiology. 2012; 264:126–135. PMID: 22550312.


14. Yuan J, Yeung DK, Mok GS, Bhatia KS, Wang YX, Ahuja AT, et al. Non-gaussian analysis of diffusion weighted imaging in head and neck at 3T: a pilot study in patients with nasopharyngeal carcinoma. PLoS One. 2014; 9:e87024. PMID: 24466318.


15. Jansen JF, Stambuk HE, Koutcher JA, Shukla-Dave A. Non-gaussian analysis of diffusion-weighted MR imaging in head and neck squamous cell carcinoma: a feasibility study. AJNR Am J Neuroradiol. 2010; 31:741–748. PMID: 20037133.


16. Chen Y, Ren W, Zheng D, Zhong J, Liu X, Yue Q, et al. Diffusion kurtosis imaging predicts neoadjuvant chemotherapy responses within 4 days in advanced nasopharyngeal carcinoma patients. J Magn Reson Imaging. 2015; 42:1354–1361. PMID: 25873208.


17. Sakamoto J, Kuribayashi A, Kotaki S, Fujikura M, Nakamura S, Kurabayashi T. Application of diffusion kurtosis imaging to odontogenic lesions: analysis of the cystic component. J Magn Reson Imaging. 2016; 44:1565–1571. PMID: 27185307.


18. Xu XQ, Liu J, Hu H, Su GY, Zhang YD, Shi HB, et al. Improve the image quality of orbital 3 T diffusion-weighted magnetic resonance imaging with readout-segmented echo-planar imaging. Clin Imaging. 2016; 40:793–796. PMID: 27317226.


19. Koyasu S, Iima M, Umeoka S, Morisawa N, Porter DA, Ito J, et al. The clinical utility of reduced-distortion readout-segmented echo-planar imaging in the head and neck region: initial experience. Eur Radiol. 2014; 24:3088–3096. PMID: 25117744.


20. Yuan M, Zhang YD, Zhu C, Yu TF, Shi HB, Shi ZF, et al. Comparison of intravoxel incoherent motion diffusion-weighted MR imaging with dynamic contrast-enhanced MRI for differentiating lung cancer from benign solitary pulmonary lesions. J Magn Reson Imaging. 2016; 43:669–679. PMID: 26340144.


21. DeLong ER, DeLong DM, Clarke-Pearson DL. Comparing the areas under two or more correlated receiver operating characteristic curves: a nonparametric approach. Biometrics. 1988; 44:837–845. PMID: 3203132.


22. Jiang JX, Tang ZH, Zhong YF, Qiang JW. Diffusion kurtosis imaging for differentiating between the benign and malignant sinonasal lesions. J Magn Reson Imaging. 2017; 45:1446–1454. PMID: 27758016.


23. Roethke MC, Kuder TA, Kuru TH, Fenchel M, Hadaschik BA, Laun FB, et al. Evaluation of diffusion kurtosis imaging versus standard diffusion imaging for detection and grading of peripheral zone prostate cancer. Invest Radiol. 2015; 50:483–489. PMID: 25867657.


24. Jiang R, Jiang J, Zhao L, Zhang J, Zhang S, Yao Y, et al. Diffusion kurtosis imaging can efficiently assess the glioma grade and cellular proliferation. Oncotarget. 2015; 6:42380–42393. PMID: 26544514.


25. Iima M, Yano K, Kataoka M, Umehana M, Murata K, Kanao S, et al. Quantitative non-gaussian diffusion and intravoxel incoherent motion magnetic resonance imaging: differentiation of malignant and benign breast lesions. Invest Radiol. 2015; 50:205–211. PMID: 25260092.
26. Yu J, Huang DY, Li Y, Dai X, Shi HB. Correlation of standard diffusion-weighted imaging and diffusion kurtosis imaging with distant metastases of rectal carcinoma. J Magn Reson Imaging. 2016; 44:221–229. PMID: 26715111.


27. Zhao M, Liu Z, Sha Y, Wang S, Ye X, Pan Y, et al. Readout-segmented echo-planar imaging in the evaluation of sinonasal lesions: a comprehensive comparison of image quality in single-shot echo-planar imaging. Magn Reson Imaging. 2016; 34:166–172. PMID: 26541548.


28. Bogner W, Pinker-Domenig K, Bickel H, Chmelik M, Weber M, Helbich TH, et al. Readout-segmented echo-planar imaging improves the diagnostic performance of diffusion-weighted MR breast examinations at 3.0 T. Radiology. 2012; 263:64–76. PMID: 22438442.


Fig. 1
Box plots showing comparison of ADC (A), Dapp (B), and Kapp (C) for benign and malignant masses in head and neck region.
Line in box represents median. Height of box represents interquartile range. Wiskers are lowest and highest data points within 1.5 interquartile range. Circles indicate outliers. ADC = apparent diffusion coefficient, Dapp = apparent diffusion for Gaussian distribution, Kapp = apparent kurtosis coefficient
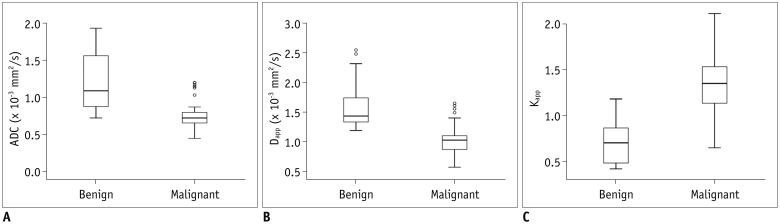
Fig. 2
68-year-old man with adenocarcinoma of parotid gland.
A. Axial T2-weighted image showing infiltrative mass located in right parotid gland. After region of interest (red line) was dawn around mass (B), color maps for ADC (C), Dapp
(D), and Kapp
(E) were obtained and superimposed on DK image (b1000 map). ADC, Dapp, and Kapp values of mass were 1.037 × 10−3 mm2/s, 1.325 × 10−3 mm2/s, and 0.766, respectively. DK = diffusion kurtosis
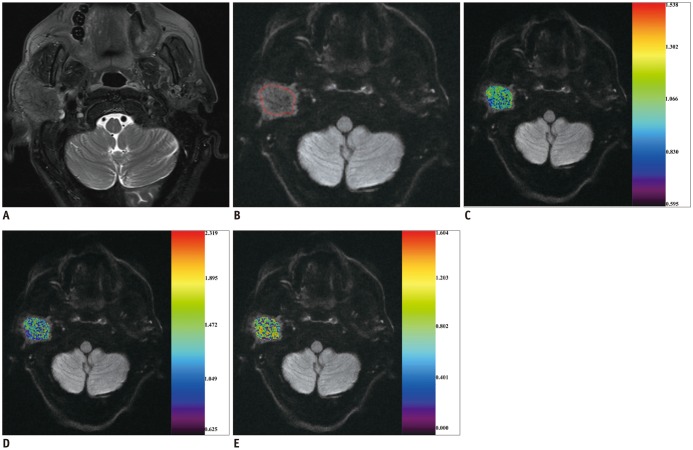
Fig. 3
53-year-old man with pleomorphic adenoma of parotid gland.
A. Axial T2-weighted image showing local mass located in right parotid gland. After region of interest (red line) was dawn around mass (B), color maps for ADC (C), Dapp
(D), and Kapp
(E) were obtained and superimposed on DK image (b1000 map). ADC, Dapp, and Kapp values of mass were 2.010 × 10−3 mm2/s, 2.308 × 10−3 mm2/s, and 0.420, respectively.
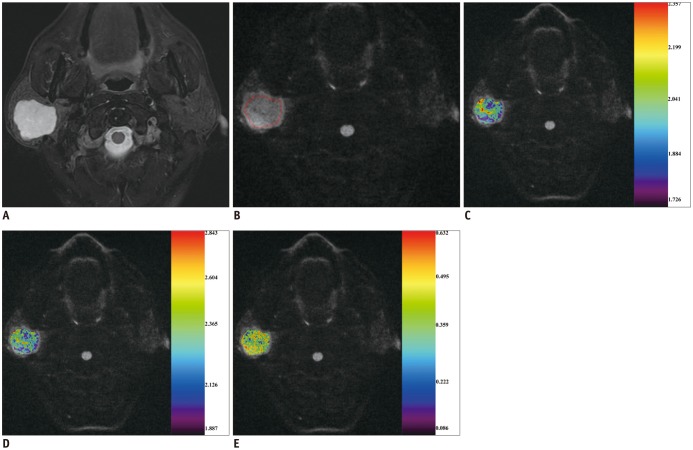
Fig. 4
Comparison of diagnostic ability for discriminating malignant from benign masses in head and neck region among different parameters.
Combination of Dapp and Kapp showed significantly (p = 0.042) higher AUC than ADC alone. AUC = area under ROC curve, ROC = receiver operating characteristic
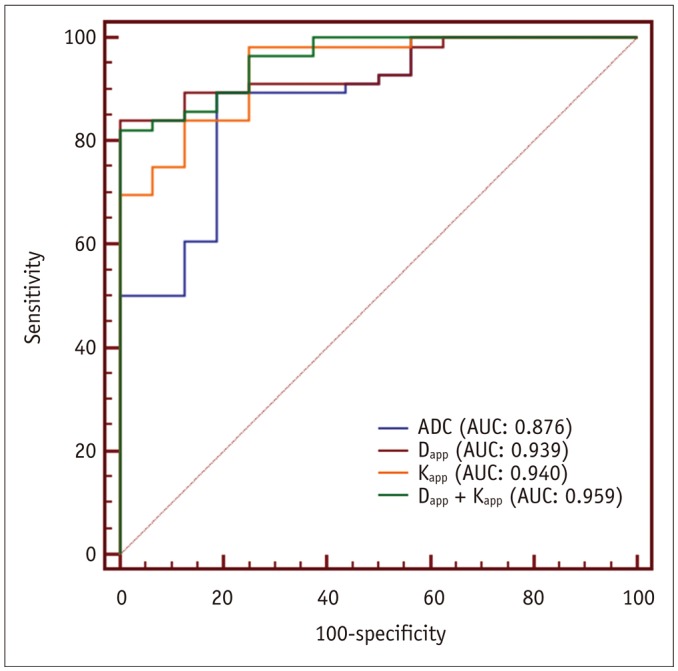
Fig. 5
Bland-Altman plots showing reproducibility of measurement for ADC (A), Dapp (B), and Kapp (C).
Blue line = mean absolute difference. Green lines = confidence interval of mean difference. Red lines = 95% confidence interval of mean difference

Table 1
Demographic and Pathological Characteristics of Our Study
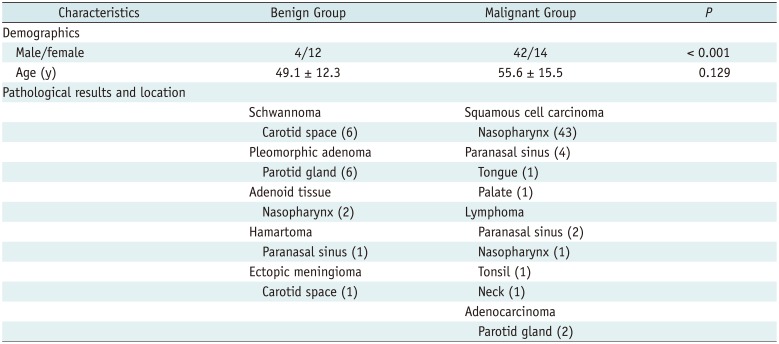
Table 2
Differences of Diffusion-Weighted and Diffusion Kurtosis Imaging Derived Parameters between Benign and Malignant Group

Parameters | Benign Group (n = 16) | Malignant Group (n = 56) | P |
---|---|---|---|
ADC | 1.222 ± 0.420 | 0.754 ± 0.167 | < 0.001 |
Dapp | 1.640 ± 0.445 | 1.029 ± 0.226 | < 0.001 |
Kapp | 0.715 ± 0.249 | 1.344 ± 0.309 | < 0.001 |
Table 3
Diagnostic Ability of Significant Imaging Parameters for Predicting Malignancy
