Abstract
Objective
The purpose of this study was to evaluate the reliability and quality of radiomic features in glioblastoma multiforme (GBM) derived from tumor volumes obtained with semi-automated tumor segmentation software.
Materials and Methods
MR images of 45 GBM patients (29 males, 16 females) were downloaded from The Cancer Imaging Archive, in which post-contrast T1-weighted imaging and fluid-attenuated inversion recovery MR sequences were used. Two raters independently segmented the tumors using two semi-automated segmentation tools (TumorPrism3D and 3D Slicer). Regions of interest corresponding to contrast-enhancing lesion, necrotic portions, and non-enhancing T2 high signal intensity component were segmented for each tumor. A total of 180 imaging features were extracted, and their quality was evaluated in terms of stability, normalized dynamic range (NDR), and redundancy, using intra-class correlation coefficients, cluster consensus, and Rand Statistic.
Results
Our study results showed that most of the radiomic features in GBM were highly stable. Over 90% of 180 features showed good stability (intra-class correlation coefficient [ICC] ≥ 0.8), whereas only 7 features were of poor stability (ICC < 0.5). Most first order statistics and morphometric features showed moderate-to-high NDR (4 > NDR ≥1), while above 35% of the texture features showed poor NDR (< 1). Features were shown to cluster into only 5 groups, indicating that they were highly redundant.
Conclusion
The use of semi-automated software tools provided sufficiently reliable tumor segmentation and feature stability; thus helping to overcome the inherent inter-rater and intra-rater variability of user intervention. However, certain aspects of feature quality, including NDR and redundancy, need to be assessed for determination of representative signature features before further development of radiomics.
Glioblastoma multiforme (GBM), the most frequent and malignant brain tumor in adults (1), continues to show poor prognoses and low survival rates despite decades of multimodality treatment research. Along with the increasing availability of genomic data for the brain tumors, active studies are underway to enable genomic characterization and improved clinical outcome of GBM patients. Recent studies have revealed the genomic characteristics of GBM including the distinct patterns in gene expression profiles, underlying genomic abnormalities, and epigenetic modifications (2). Improved understanding of the behavior of GBM at the molecular and genomic levels could lead to development of new drugs as well as patient-specific treatment regimens, thus facilitating precision medicine in the clinical field.
As knowledge of GBM increases from the genomic and clinical perspective, there is a growing need for reliable and efficient extraction of quantitative features from multimodality imaging data for associating imaging tumor phenotypes with genomic characteristics as well as clinical prognosis.
Radiomics, which refers to the high-throughput extraction of a large amount of quantitative features from radiologic images, has emerged as a significant research interest across a variety of specialties (345).
Several studies have shown the positive potential of radiomic features for treatment monitoring and outcome prediction as well as associating imaging phenotypes with genomic profiles in various tumors (3467). For example, Aerts et al. (3) have shown that proper analysis of radiomic features could lead to identification of signature features, which was effective in decoding of tumor phenotypes and predictive of patient prognosis in lung and head-and-neck cancer.
Glioblastoma multiforme has frequently been the study subject of radiomic and radiogenomic research. Diehn et al. (8) have identified a set of MR imaging features highly associated with gene expression patterns of several well-known gene programs and predictive of overall survival in GBM patients. Zinn et al. (9) have used a semi-automated segmentation technique to derive a set of volumetric features from MR images, and were able to produce radiogenomic mapping of edema or cellular invasion phenotypes in GBM. The association of imaging phenotypes with clinical outcomes as well as molecular subtypes, and related biological pathways in GBM (1011121314) has been studied using MRI features derived from visual grading method, manually-drawn region of interest (ROI), or various types of computer-assisted techniques.
These studies have stressed the positive potential of the radiomic approach; however, evaluating the reliability of radiomic features in GBM is also important. Previously, lung cancer-related radiomic studies have evaluated the reliability as an integral part of study (34), whereas the reliability of features in GBM remains unclear.
Tumor segmentation is regarded as the major source of variability in radiomics, since radiomic features are routinely derived from the segmented tumors using a computer algorithm (13). In studying radiomics of GBM, tumor segmentation involves more complex tasks since differing tumor imaging phenotypes appear differently such as contrast enhancement, necrosis, and edema depending on MR sequences; in addition, image registration is required prior to tumor segmentation. Thus, tumor segmentation in GBM is prone to additional sources of variability and increases the uncertainty of feature reliability, which may lead to false positives if highly variable features were employed unknowingly. Therefore, evaluating the quality of radiomic features in GBM is an important and necessary step before translating into clinical application.
In this study, we evaluated the reliability of radiomic features in GBM derived via a computer-assisted tumor segmentation procedure. In particular, we assessed the feature stability against perturbations in tumor segmentation caused by varying raters and semi-automated segmentation techniques. In addition, we evaluated the normalized dynamic range (NDR) and redundancy of feature values thus qualifying the radiomic features in GBM in multiple aspects.
MR images of 45 GBM patients were downloaded from the National Cancer Institute's “The Cancer Imaging Archive” (http://cancerimagingarchive.net/) (15). Study patients consisted of 29 males and 16 females. The average age of the male patients was 56 years (range, 32–78 years), and 57.5 years (range, 26–73 years) for the female patients. The molecular subtypes were 5 proneural, 4 classical, 4 neural, and 17 mesenchymal GBMs. Molecular subtype information was not available for 15 of the 45 patients.
Images of two MRI sequences were used for segmenting different tumor tissue components: post-contrast T1-weighted imaging for segmentation of enhancing and necrotic (NC) tissues, and fluid-attenuated inversion recovery (FLAIR) for segmentation of the non-enhancing T2 high signal intensity (NH) (Fig. 1).
The overall procedure for quality evaluation of the radiomic features in GBM is depicted in Figure 2. T1 post-contrast and T2 FLAIR images were first registered, followed by tumor segmentation using two different semi-automatic methods by two different raters. Subsequently, image features were extracted from segmented tumor ROIs using the computer algorithm. Finally, we evaluated the quality of the features in terms of robustness, NDR, and redundancy.
We considered three different tumor tissue components including contrast-enhancing (CE), NC, and NH tissues for segmenting tumor ROIs. Tumor segmentation was carried out by two experienced raters (10 and 3 years, respectively) for each tumor tissue component using semi-automated segmentation software tools. We used two different software tools: 3D Slicer (ver. 4.3.1) (16), which is a free software download (http://www.slicer.org); and TumorPrism3D (17), which is an in-house software tool developed in our laboratory. Both software tools allowed the raters to conduct semi-automated tumor segmentation. Tumor segmentation procedures for the two software tools are illustrated in Figure. 3. With 3D Slicer, the user defined the background and foreground objects by drawing sketches on them, followed by automated separation of tumor from background tissue using the grow-cut algorithm. With TumorPrism3D, the user drew an initial ROI within the tumor, followed by automated tumor segmentation using the deformable model-based algorithm. Thus, four computer-assisted tumor segmentation datasets (2 software tools × 2 raters) were produced for 3 different tumor tissue components in each patient.
In addition, for evaluation of the accuracy of the computer-assisted semi-automated tumor segmentation, we created a reference tumor segmentation dataset for the same cases from in-house software data acquired by two radiologists in consensus.
Accuracy of computer-assisted tumor segmentation was evaluated by calculating the similarity between the reference and the computer-assisted tumor segmentation. We used the dice similarity coefficient (DSC) measure for calculating the similarity between the two segmented tumor volumes. The similarity measures were obtained from the binary tumor masks generated from the segmented tumor volumes.
The DSC measure represents the relative overlap between two binary volume data, and is expressed in Eq. (1).
where V denotes the volume of binary data, C and R represent the computer-assisted and reference tumor segmentation data, respectively. The DSC score is 1.0 when the two volumes match perfectly.
We considered three groups of radiomic features such as the first order statistic, texture, and morphometric features, which have been used in previous studies (18192021).
First order statistics described the pattern of pixel intensity distribution within the segmented tumor ROI. Thirteen first order statistic features were extracted from the three tumor component ROIs, thus generating a total of 42 first order statistic features.
Texture features represented the changing pattern of pixel intensity between neighboring pixels. We extracted 22 gray level co-occurrence matrix-based and 11 gray level run-length matrix-based texture features from the three tumor component ROIs, thus generating a total of 99 texture features.
Morphometric features were descriptors of the size and shape of the tumor. We extracted 11 morphometric features from the three tumor component ROIs, thus generating a total of 33 morphometric features. All feature extraction was performed with a software program written in MATLAB (The Mathworks, Natick, MA, USA). The three groups of extracted features are listed in Table 1. Details of extraction methods are described in Supplementary (in the online-only Data Supplement).
We evaluated the quality of features in terms of stability, NDR, and redundancy.
Stability represented the agreement of features derived by two raters using the computer-assisted tumor segmentation procedure, and was tested with the intra-class correlation coefficient (ICC). Features with ICC ≥ 0.8 were considered as good stability, 0.8 > ICC ≥ 0.5 as moderate stability, and ICC < 0.5 as poor stability.
Normalized dynamic range described the relative range of feature values as compared to the average value, and was expressed in Eq. (2).
Max10: average of top 10% in feature value
Min10: average of bottom 10% in feature value
Normalized dynamic range calculates the difference between feature values of top 10% and bottom 10% samples from study population, which was then normalized by the absolute mean feature value. Average feature values from two raters were used in NDR calculation. Features with NDR ≥ 4 were considered as good dynamic range, 4 > NDR ≥ 1 as moderate dynamic range, and NDR < 1 as poor dynamic range.
Redundancy denoted the similarity shared among different features. Different features reflect unique aspects of tumor phenotype and do not resemble each other. In practice, however, computer extracted tumor features often exhibit similarity among one another resulting in redundancy in the radiomic feature pool.
We examined the redundancy of radiomic features in GBM by assessing their cluster properties; features belonging to the same cluster were regarded as redundant. We applied the consensus clustering technique using an R package ConsensusClusterPlus (22) to assess the cluster properties of the radiomic features. With this package, we obtained the cluster consensus (CC) for each feature cluster, which was defined as the average consensus between all pairs of features belonging to the same cluster. The CC (range [0–1]) indicates the robustness of a cluster over multiple runs of experiments with resampled parameters during cluster generation procedure. We chose 5 clusters that gave the most robust CC. We regarded those clusters with CC ≥ 0.8, 0.8 > CC ≥ 0.5, and CC < 0.5 as good, moderate, and poor robustness, respectively.
In addition, we assessed the cluster agreement for each feature cluster, which was defined as the average agreement of feature pairs belonging to the same cluster derived from two different raters. The Rand Statistic (RS) was used to measure the cluster agreement as shown in Eq. (3).
where |YY| is the number of feature pairs that cluster together from both rater 1 and rater 2, |YN| is the number of feature pairs that cluster together from rater 1 but not from rater 2, |NY| is the number of feature pairs that cluster together from rater 2, but not from rater 1 and |NN| is the number of feature pairs that do not cluster together from both rater 1 and rater 2. We regarded those clusters with RS ≥ 0.8, 0.8 > RS ≥ 0.5, and RS < 0.5 as good, moderate, and poor agreement, respectively.
Statistical analyses were performed with software (SPSS, R, and MATLAB). ICC, CC, and RS were calculated using SPSS Statistics for Windows (Version 22.0, IBM Corp., Armonk, NY, USA), R version 3.1.2 (http://www.R-project.org), and MATLAB version R2016a (The Mathworks), respectively.
Accuracy of the segmented tumors obtained with the two semi-automated tumor segmentation tools as assessed with DSC is shown in Table 2. With both semi-automated tumor segmentation tools, two raters produced tumor masks with similar accuracy. With TumorPrism3D, the DSC of tumor segmentation ranged from 0.79 to 0.84 for the first rater, and 0.76 to 0.80 for the second rater. With 3D Slicer, the DSC ranged from 0.74 to 0.77 for the first rater and 0.71 to 0.74 for the second rater.
Figure 4 shows example segmentation results of the three tumor tissue components (i.e., CE, NC, and NH) with the two semi-automated segmentation tools. Case A represents a case with similar results, while Case B represents a case with slightly different results.
Stabilities of the 180 radiomic features as assessed with ICC are summarized in Table 3. Overall, the two semi-automated software tools produced similarly stable features. With TumorPrism3D, 40 of 42 first order statistic features, 88 of 99 texture features, and 36 of 39 morphometric features showed high stability. With 3D Slicer, 41 of 42 first order statistic features, 86 of 99 texture features, and 35 of 39 morphometric features showed excellent stability. However, a few (n = 7) of the texture and the morphometric features showed poor ICC, which suggests that those features might be more susceptible to rater-dependent differences in tumor segmentation.
Features showed different NDR depending on feature group (Fig. 5). Of the total 180 features, only 37 (20.5%) and 40 (22.2%) features showed good NDR, while 44 (24.4%) and 40 (22.2%) features showed poor NDR, with TumorPrism3D and 3D Slicer, respectively. Among the three feature groups, first order statistic group showed relatively higher NDR: 39 out of 42 (92.8%) features showed good or moderate NDR with both software tools. In contrast, a majority of features that showed poor NDR were in the texture feature group. In morphometric feature group, a majority of features (37 with TumorPrism3D, 32 with 3D Slicer) showed moderate NDR. Summary of NDRs for the GBM radiomic features is shown in Table 4.
Application of consensus clustering to the 180 radiomic features revealed 5 distinct feature clusters. Properties of the five clusters are compared in Table 5. Robustness of 5 clusters as assessed with CC ranged from 0.73 to 0.98, and their agreements as assessed with RS ranged from 0.62 to 0.97. Notably, the CC and RS except the smallest cluster were all higher than 0.8. Figure 6 shows the CC maps for the two segmentation tools. In both maps, size of cluster 1 was considerably small with moderate robustness and agreement, and the other 4 clusters were of similar size with good robustness and agreement.
Figure 7 illustrates the decomposition of each feature cluster into three feature groups. Cluster 1 was composed solely of morphometric features; clusters 2 and 5 were composed mostly of 1st order statistic and texture features; and clusters 3 and 4 of all three feature groups. Figure 8 illustrates the decomposition of each feature cluster into three tumor tissue components. Clusters 1 and 4 were composed mostly of CE and NH tissues; clusters 2 and 3 were of CE and NC; and cluster 5 mostly of NH. Notably, the same tendency was observed in both cluster maps derived with TumorPrism3D and 3D Slicer.
More details of clustered features are described in Supplementary Table 1 (in the online-only Data Supplement).
In this study, we investigated the quality of radiomic features in GBM. We categorized radiomic features into first order statistic, texture, and morphometric feature groups and assessed their quality in terms of stability, NDR, and redundancy.
Image segmentation is the first step in radiomic feature analysis, and thus can be considered as a major source of variability in radiomics. Use of a semi-automated software for tumor segmentation is a preferred option in radiomics studies since it offers markedly improved efficiency and may reduce inter-observer variability in tumor delineation (2324). The 3D Slicer has often been used in previous studies for segmenting 3D tumor volume due to its free availability in public domain. However, study settings may include different software tools employing differently evolved algorithms. Therefore, comparing the performance of different semi-automated software tools in terms of quality assessment of radiomic features could form the basis for study design and experimental tools.
In our experiment, using two semi-automated software tools, the tumor segmentation accuracy as assessed with DSC ranged from 0.71 to 0.84 depending on differing raters and software tools. Compared to reported values of segmentation accuracy of brain tumors that ranged considerably (0.48 to 0.97) (25), the two software tools employed in our study appear to provide consistently good accuracy in segmenting GBM tumors. Thus, we regarded the two software tools as adequate for use in the subsequent assessment of the quality of radiomic features in GBM.
Stability has been often used for quality assurance and selection of robust features at the first step in radiomic feature analysis (4). Our study results showed that most of the radiomic features in GBM were highly stable. Over 90% of 180 features showed good stability (ICC ≥ 0.8), whereas only 7 features had poor stability (ICC < 0.5) with both software tools. In general, first order statistic group showed relatively higher stability, followed by morphometric group and texture group, in order. These results agree with the data reported by Parmar et al. (4). They examined the stability of radiomic features in CT lung cancer scans against three independent raters with the 3D Slicer as a semi-automated segmentation tool; the results indicated overall high ICC (0.85 ± 0.15) with 74% of 3D radiomic features showing good stability (ICC ≥ 0.8) and only 3 of 56 features showing poor stability (ICC < 0.5). This congruence suggests that these semi-automated software tools are sufficiently reliable in extracting radiomic features in different study settings, including CT for the diagnosis of lung cancer and MRI for the diagnosis of GBM.
Dynamic range has often been used as a measure of informativeness of radiomic features. As a certain degree of perturbation is unavoidable in extracted radiomic features due to inherent variability from different sources, features with higher dynamic range are regarded as more robust to feature perturbation and thus regarded to possess relatively good information compared to those with narrow dynamic range.
In this study, we defined the NDR as the dynamic range of a feature over the study population divided by its mean; NDR was used for comparison of the relative dynamic range of radiomic features regardless of their feature value range. Our study results indicated differences in NDR among differing feature groups. Most first order statistics and morphometric features (93 and 95%, respectively) showed good or moderate NDR. In contrast, texture features showed relatively lower NDR, with > 35% of texture features of poor NDR. In a previous study on reproducibility of radiomic features in CT lung cancer, Balagurunathan et al. (26) examined the dynamic range of different feature groups, and also found that significant differences existed in dynamic range of different features.
Majority of the morphometric and histogram features were among the higher rank of dynamic range; whereas, significant portion of texture features were among the lower rank. These findings suggest the need for an understanding of differences in feature dynamic range in determining truly reliable and informative features in radiomic feature analysis.
Typically, several features are extracted at an early stage in the radiomic feature-analysis process, which may reach up to several hundreds and exceed the number of samples. This can cause data overfitting and increase the risk of false positives leading to decreased reliability of the study results. Therefore, reducing the redundant features and choosing a small subset of representative signature features is an essential step in radiomics study. In this process, assessment of feature cluster property reflects the degree of similarity as well as distinctness among feature groups and thus facilitates the determination of the characteristics of a representative feature subset.
The CC map produced in our study revealed that the 180 features were highly redundant and could be compressed into 5 distinct clusters. In addition, both CC and RS derived using two different segmentation software tools showed very similar trends across the five clusters. These findings suggest that those feature cluster properties shown in our study were of fundamental nature in radiomic features of GBM regardless of segmentation software tools and rater's experience.
We expected that diverse delineation pattern of CE, NC, and NH tumor tissues appearing on multi-parametric MR images would form more complex cluster pattern. However, we identified 5 clusters, which was less than the previously reported 11 and 13 clusters found from 440 CT radiomic features of lung cancer and head and neck cancer, respectively (27).
Significant differences existed in the proportion of features based on the concurrent tumor components. A substantial proportion of features (in clusters 2 and 3) related to CE and NC tissues, which might indicate a strong interaction between the CE and NC components. In contrast, less proportion of features were of CE and NH tissues (in cluster 4), which might indicate a weaker interaction between the CE and NH components. Further study is required for a pathophysiological interpretation of this finding.
Our study has several limitations. First, only two types of software tools were used for evaluation. Semi-automated segmentation tools employ sophisticated algorithms to reduce user's manual intervention as much as possible and provide reliable segmentation results at the same time. Computer vision community has developed different kinds of segmentation algorithms specialized for use in medical imagery. To our best knowledge, grow-cut algorithm and deformable model-based algorithm were two representative semi-automated algorithms for segmenting tumors on medical images, and were implemented in the 3D Slicer and the TumorPrism3D, respectively. As image segmentation algorithms continue to evolve requiring less intervention from users and making use of more learned knowledge from a large image database, radiomics applications of additional software tools with novel algorithms should be investigated in future.
Second, stability of radiomic features was evaluated with a single scan dataset. Though variation of segmented tumor volumes due to inconsistent user intervention is regarded a major source of instability of radiomic features, varying physio-physical state of patient and scanner might cause additional perturbations to radiomic features. Accordingly, it would be desirable to use a same-day repeat scan data set to evaluate the stability of features against overall sources of variability. However, such a data set was not available to our study. Therefore, our stability data should be interpreted with caution in that they are applicable only to limited sources of variability.
In addition, our study used an MRI data set acquired with a relatively simple protocol. As a variety of pulse sequences are used in MR imaging, it is obvious that images acquired with different pulse sequence would bring about different feature quality in GBM study. For example, diffusion imaging is increasingly used in GBM, which produces much noisier images and accordingly would give rise to radiomic features of much different quality. Thus, our study results cannot be generalized to all MR GBM studies.
In conclusion, the use of two different semi-automated software tools by different raters showed similar high stability in radiomic features in GBM regardless of difference in raters' experience, indicating that semi-automated software tools provide sufficiently reliable segmentation output and help overcome the inherent inter-and intra-rater variability from user intervention. However, significant differences existed in NDR among features, which suggests that features convey information with differing strength. Among the feature groups, texture features showed the weakest NDR. A total of 180 radiomic features in our study were highly redundant, and compressible to 5 distinct clusters. However, significant differences existed in the proportion of features according to the tumor tissue components appearing together.
A well-established quality assurance procedure has an important role in the future advancement of radiomics and translation into patient care. The findings in our study may be useful in guiding the development of quality assurance procedure of the radiomics pipeline, particularly for GBM.
Notes
This work was supported by the National Research Foundation of Korea (NRF) grant funded by the Korea government (MSIP) (NRF-2016R1A2B4010401), in part by grants (grant number: HI16C1127, HI14C1234) of the Korea Health Technology R&D Project through the Korea Health Industry Development Institute (KHIDI), funded by the Ministry of Health & Welfare, Republic of Korea.
References
1. Ohgaki H, Kleihues P. Epidemiology and etiology of gliomas. Acta Neuropathol. 2005; 109:93–108. PMID: 15685439.


2. Verhaak RG, Hoadley KA, Purdom E, Wang V, Qi Y, Wilkerson MD, et al. Integrated genomic analysis identifies clinically relevant subtypes of glioblastoma characterized by abnormalities in PDGFRA, IDH1, EGFR, and NF1. Cancer Cell. 2010; 17:98–110. PMID: 20129251.


3. Aerts HJ, Velazquez ER, Leijenaar RT, Parmar C, Grossmann P, Carvalho S, et al. Decoding tumour phenotype by noninvasive imaging using a quantitative radiomics approach. Nat Commun. 2014; 5:4006. PMID: 24892406.


4. Parmar C, Rios Velazquez E, Leijenaar R, Jermoumi M, Carvalho S, Mak RH, et al. Robust radiomics feature quantification using semiautomatic volumetric segmentation. PLoS One. 2014; 9:e102107. PMID: 25025374.


5. Kim M, Kim HS. Emerging techniques in brain tumor imaging: what radiologists need to know. Korean J Radiol. 2016; 17:598–619. PMID: 27587949.


6. Tixier F, Le Rest CC, Hatt M, Albarghach N, Pradier O, Metges JP, et al. Intratumor heterogeneity characterized by textural features on baseline 18F-FDG PET images predicts response to concomitant radiochemotherapy in esophageal cancer. J Nucl Med. 2011; 52:369–378. PMID: 21321270.


7. El Naqa I, Grigsby P, Apte A, Kidd E, Donnelly E, Khullar D, et al. Exploring feature-based approaches in PET images for predicting cancer treatment outcomes. Pattern Recognit. 2009; 42:1162–1171. PMID: 20161266.


8. Diehn M, Nardini C, Wang DS, McGovern S, Jayaraman M, Liang Y, et al. Identification of noninvasive imaging surrogates for brain tumor gene-expression modules. Proc Natl Acad Sci U S A. 2008; 105:5213–5218. PMID: 18362333.


9. Zinn PO, Mahajan B, Sathyan P, Singh SK, Majumder S, Jolesz FA, et al. Radiogenomic mapping of edema/cellular invasion MRI-phenotypes in glioblastoma multiforme. PLoS One. 2011; 6:e25451. PMID: 21998659.


10. Cancer Genome Atlas Research Network. Comprehensive genomic characterization defines human glioblastoma genes and core pathways. Nature. 2008; 455:1061–1068. PMID: 18772890.
11. Gevaert O, Mitchell LA, Achrol AS, Xu J, Echegaray S, Steinberg GK, et al. Glioblastoma multiforme: exploratory radiogenomic analysis by using quantitative image features. Radiology. 2014; 273:168–174. PMID: 24827998.


12. Gutman DA, Cooper LA, Hwang SN, Holder CA, Gao J, Aurora TD, et al. MR imaging predictors of molecular profile and survival: multi-institutional study of the TCGA glioblastoma data set. Radiology. 2013; 267:560–569. PMID: 23392431.


13. Yang D, Rao G, Martinez J, Veeraraghavan A, Rao A. Evaluation of tumor-derived MRI-texture features for discrimination of molecular subtypes and prediction of 12-month survival status in glioblastoma. Med Phys. 2015; 42:6725–6735. PMID: 26520762.


14. Itakura H, Achrol AS, Mitchell LA, Loya JJ, Liu T, Westbroek EM, et al. Magnetic resonance image features identify glioblastoma phenotypic subtypes with distinct molecular pathway activities. Sci Transl Med. 2015; 7:303ra138.


15. The Cancer Imaging Archive (TCIA). Web site. March 5, 2016. http://www.cancerimagingarchive.net/.
16. 3D Slicer. Web site. March 15, 2016. http://www.slicer.org/.
17. Lee M, Cho W, Kim S, Park S, Kim JH. Segmentation of interest region in medical volume images using geometric deformable model. Comput Biol Med. 2012; 42:523–537. PMID: 22402196.


18. Balagurunathan Y, Gu Y, Wang H, Kumar V, Grove O, Hawkins S, et al. Reproducibility and prognosis of quantitative features extracted from CT images. Transl Oncol. 2014; 7:72–87. PMID: 24772210.


19. Kim H, Park CM, Lee SM, Lee HJ, Goo JM. A comparison of two commercial volumetry software programs in the analysis of pulmonary ground-glass nodules: segmentation capability and measurement accuracy. Korean J Radiol. 2013; 14:683–691. PMID: 23901328.


20. Egger J, Kapur T, Fedorov A, Pieper S, Miller JV, Veeraraghavan H, et al. GBM volumetry using the 3D Slicer medical image computing platform. Sci Rep. 2013; 3:1364. PMID: 23455483.


21. Zhu Y, Young GS, Xue Z, Huang RY, You H, Setayesh K, et al. Semi-automatic segmentation software for quantitative clinical brain glioblastoma evaluation. Acad Radiol. 2012; 19:977–985. PMID: 22591720.


22. Wilkerson MD. ConsensusClusterPlus (Tutorial). 2016. July 1, 2016. Available at: https://www.bioconductor.org/packages/devel/bioc/vignettes/ConsensusClusterPlus/inst/doc/ConsensusClusterPlus.pdf.
23. de Hoop B, Gietema H, van Ginneken B, Zanen P, Groenewegen G, Prokop M. A comparison of six software packages for evaluation of solid lung nodules using semiautomated volumetry: what is the minimum increase in size to detect growth in repeated CT examinations. Eur Radiol. 2009; 19:800–808. PMID: 19018537.


24. Jung SC, Choi SH, Yeom JA, Kim JH, Ryoo I, Kim SC, et al. Cerebral blood volume analysis in glioblastomas using dynamic susceptibility contrast-enhanced perfusion MRI: a comparison of manual and semiautomatic segmentation methods. PLoS One. 2013; 8:e69323. PMID: 23950891.


25. Zou KH, Warfield SK, Bharatha A, Tempany CM, Kaus MR, Haker SJ, et al. Statistical validation of image segmentation quality based on a spatial overlap index. Acad Radiol. 2004; 11:178–189. PMID: 14974593.
26. Balagurunathan Y, Kumar V, Gu Y, Kim J, Wang H, Liu Y, et al. Test-retest reproducibility analysis of lung CT image features. J Digit Imaging. 2014; 27:805–823. PMID: 24990346.


27. Parmar C, Leijenaar RT, Grossmann P, Rios Velazquez E, Bussink J, Rietveld D, et al. Radiomic feature clusters and prognostic signatures specific for Lung and Head & Neck cancer. Sci Rep. 2015; 5:11044. PMID: 26251068.


Supplementary Materials
The online-only Data Supplement is available with this article at https://doi.org/10.3348/kjr.2017.18.3.498.
Fig. 1
MR images of example TCGA GBM case (TCGA-06-0213, 55-year-old female patient).
Tumor segmentation was performed semi-automatically with TumorPrism3D.
A. T1W post-contrast image. B. Segmented ROIs for enhancement (red) and necrosis (green) components. C. FLAIR image. D. Segmented ROI for non-enhancing T2 high signal intensity component (blue). FLAIR = fluid-attenuated inversion recovery, GBM = glioblastoma multiforme, ROI = region of interest, TCGA = The Cancer Genome Atlas, T1W = T1-weighted
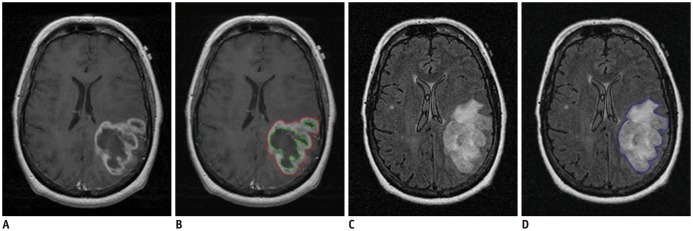
Fig. 2
Overall procedure of this study.
Contrast enhanced-T1W and T2 FLAIR images were registered, followed by tumor segmentation by two different raters using two different semi-automated software tools. Subsequently, total of 180 imaging features were extracted from segmented ROIs and used for evaluating feature quality. FLAIR = fluid-attenuated inversion recovery, GBM = glioblastoma multiforme, ROIs = regions of interest, TCIA = The Cancer Imaging Archive, T1W = T1-weighted
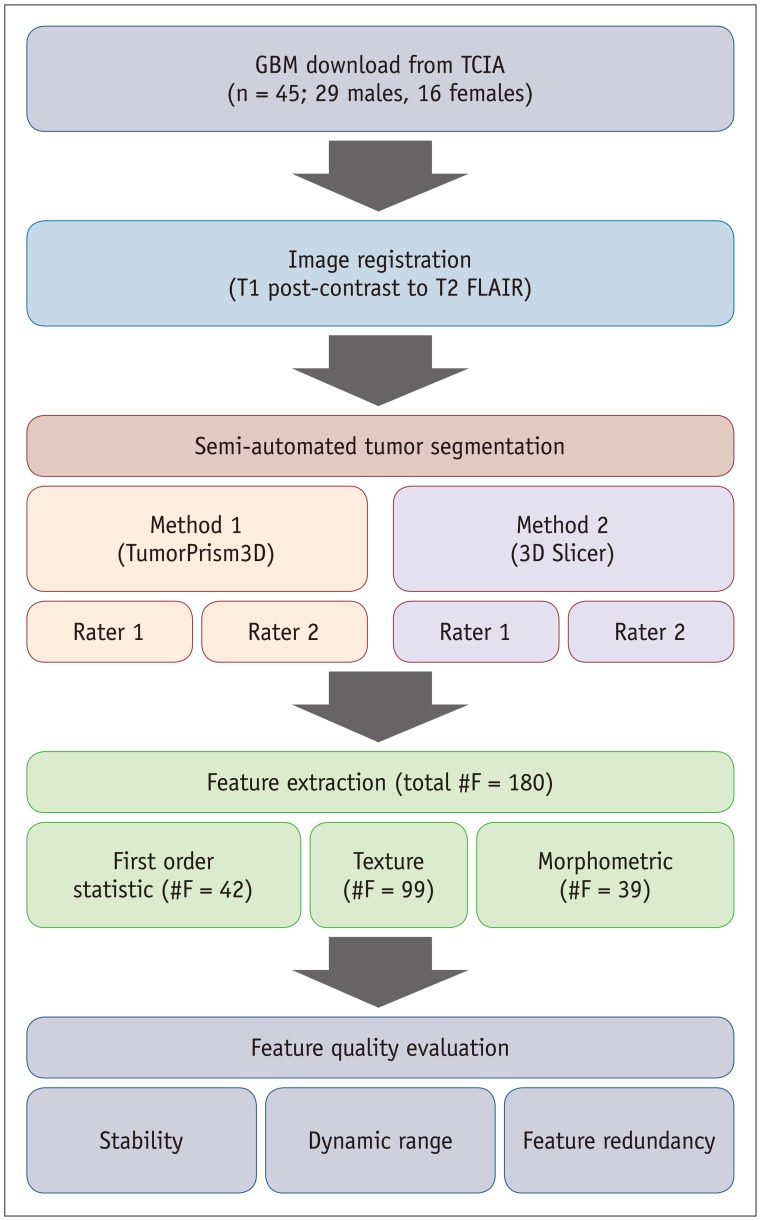
Fig. 4
Example of segmentation results with two semi-automated software tools.
Contrast-enhanced, necrotic, and non-enhancing T2 high signal intensity components are indicated by red, green, and blue color, respectively.
A. Represents case in which similar segmentation results were produced. B. Represents case in which difference was observed in segmentation results. FLAIR = fluid-attenuated inversion recovery
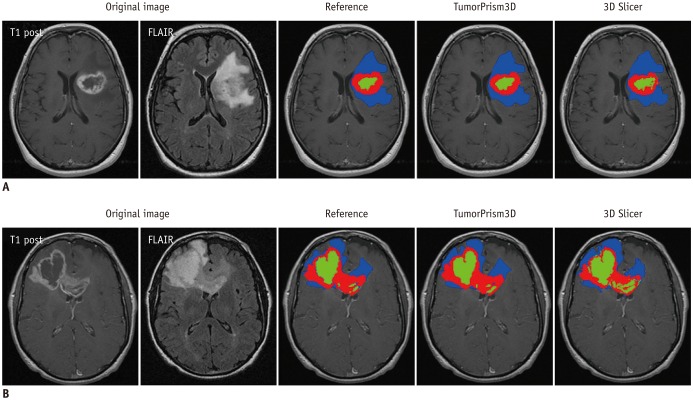
Fig. 5
Waterfall diagram of normalized dynamic range for three feature groups extracted from segmented tumor volumes with TumorPrism3D.
A. 1st order statistic feature. B. Morphometric feature. C. Texture features. CE = contrast-enhancing, NC = necrotic, NH = non-enhancing T2 high signal intensity
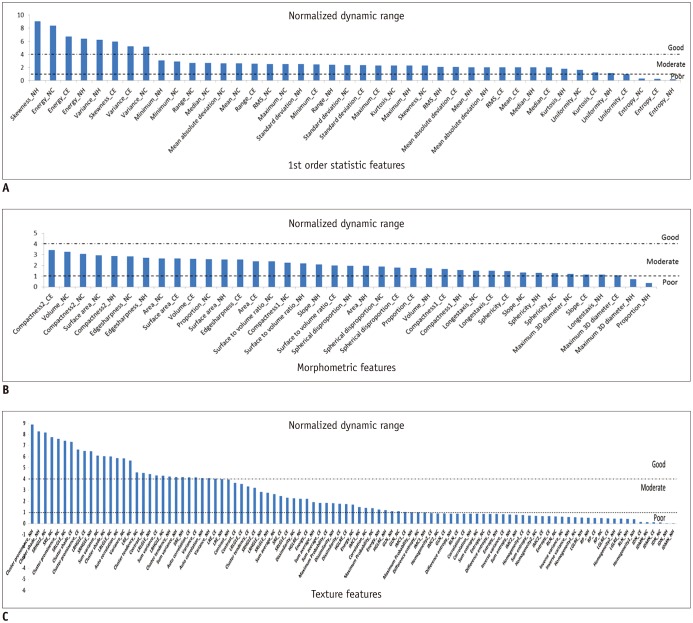
Table 1
List of Extracted Features for Each Feature Group

Table 2
Accuracy of Segmented Tumors Obtained with Two Semi-Automated Tumor Segmentation Tools

Table 3
Stability of 180 Radiomic Features as Assessed with ICC

Table 4
Proportion of Grades of NDR for Radiomic Features According to Feature Group

Table 5
Comparison of Cluster Properties for Two Semi-Automated Segmentation Tools
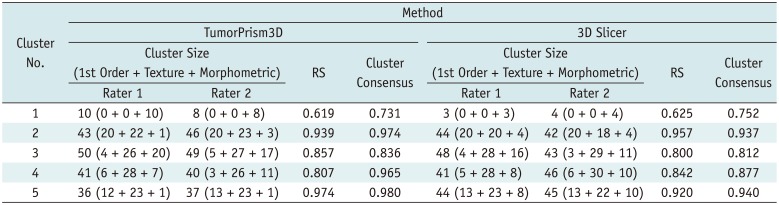