Abstract
Objective
To investigate whether the diffusion tensor imaging-derived metrics are capable of differentiating the ischemic penumbra (IP) from the infarct core (IC), and determining stroke onset within the first 4.5 hours.
Materials and Methods
All procedures were approved by the local animal care committee. Eight of the eleven rats having permanent middle cerebral artery occlusion were included for analyses. Using a 7 tesla magnetic resonance system, the relative cerebral blood flow and apparent diffusion coefficient maps were generated to define IP and IC, half hour after surgery and then every hour, up to 6.5 hours. Relative fractional anisotropy, pure anisotropy (rq) and diffusion magnitude (rL) maps were obtained. One-way analysis of variance, receiver operating characteristic curve and nonlinear regression analyses were performed.
Results
The evolutions of tensor metrics were different in ischemic regions (IC and IP) and topographic subtypes (cortical, subcortical gray matter, and white matter). The rL had a significant drop of 40% at 0.5 hour, and remained stagnant up to 6.5 hours. Significant differences (p < 0.05) in rL values were found between IP, IC, and normal tissue for all topographic subtypes. Optimal rL threshold in discriminating IP from IC was about -29%. The evolution of rq showed an exponential decrease in cortical IC, from -26.9% to -47.6%; an rq reduction smaller than 44.6% can be used to predict an acute stroke onset in less than 4.5 hours.
In acute ischemic stroke trial, the concept of “time is brain” is based on the non-specific computed tomography findings of possible salvageable ischemic penumbra (IP) (1). In contrast, “physiology is brain” is another emerging concept with which the salvageable IP and infarct core (IC) can be specifically defined by perfusion/diffusion mismatch magnetic resonance imaging (MRI). However, in the acute setting, perfusion/diffusion mismatch can be both technically and computationally demanding. Moreover, in 28% of patients who had wake-up stroke, the onset time was not known (2).
Diffusion tensor imaging (DTI) can be applied to measure cerebral micro-structural changes after ischemia by characterizing their tensor magnitude, orientation, and anisotropy. The derived DTI metrics, such as fractional anisotropy (FA), can be used as surrogate markers in monitoring the cell membrane integrity over time after injuries (3). Recent reports have indicated the advantages of DTI in determining the onset time of ischemic stroke, either by days or hours (45). However, these human study results were mostly extrapolated from acute data of different patients. The evolution of DTI metric changes in ischemic brain within the first 6 hours of stroke onset remains unknown.
Recent advance of high Tesla preclinical MRI has significantly improved the spatial resolution of the injured brain in ischemic stroke researches. In one murine model of permanent middle cerebral artery occlusion (MCAo), a rapid reduction of axial and radial diffusivity, along with later FA reduction at 24 hours, was consistent with axonal breakdown and myelin damage (6). However, interpretation of ischemic injury of the microstructure solely based on FA changes can be misleading, since the FA is a relative scalar value defined by the ratio of pure anisotropic diffusion (q) and diffusion magnitude (L). Hence, inclusion of the parameters q and L may provide a more complete picture of diffusion environment in ischemic stroke.
In the experimental rat MCAo model, we hypothesize that the evolution of DTI metric changes may differ in IP and IC regions due to different stages of axonal and myelin damages as a result of different extents of perfusion deficit during the hyperacute stroke stage. We further hypothesize that the DTI metric alternations can be used to predict the first 6.5-hour onset time of stroke. Therefore, the purpose of our study is to investigate whether the DTI metrics are orgcapable of discriminating IP from IC, and determining the stroke onset within the first 4.5 hours.
The experiment procedure was approved by the local Institute of Animal Care and Utilization Committee. Eleven male Sprague-Dawley rats (250–300 g; National Laboratory Animal Center, Taiwan) were prepared by permanent occlusions of unilateral MCA using the intra-luminal suture, as proposed by Chiang et al. (7). Three of the eleven rats died within 6.5 hours after MCAo and were therefore excluded from the subsequent analysis. One of the eight included rats died after the 24-hour imaging.
All MRI animal experiments were performed in a 7T scanner (PharmaScan 70/16; Bruker, Ettlingen, Germany). The rats were maintained under anesthesia using 1.5–2% isoflurane with an oxygen flow of 1 L/min. Rectal temperatures were maintained at 37℃ by infusing warm air through the magnet bore. In accordance with methods published by Bråtane et al. (8), T2-weighted imaging (T2WI), diffusion-weighted imaging (DWI), DTI, and dynamic susceptibility contrast (DSC) perfusion imaging were performed at eight time points, starting from 0.5 hour after MCAo, then repeated every hour until 6.5 hours, with a final 24-hour imaging performed for the assessment of the final infarct volume. Post MCAo, 100% normobaric hyperoxia (NBO) was applied using a face mask and continued for 6.5 hours, to prolong the perfusion/diffusion mismatch evolution (9). T2WI was acquired using TR/TE of 6000/80 ms and slice thickness of 1 mm with in-plane resolution of 0.2 × 0.2 mm2. Multi-shot echo-planar imaging technique was used for DWI with b factors of 0 and 1100 s/mm2. DSC imaging was estimated by a bolus injection of Gd-DTPA (0.3 mmol/kg, Magnevist; Bayer Schering Pharma, Berlin, Germany) with a serial gradient-echo echo-planar imaging using TR/TE of 1000/10 ms, flip angle of 90°, and 40 repetitions. DTI were acquired using multi-shot echo-planar imaging, including 6 volumes with diffusion gradients applied along 6 non-collinear orientations (b = 1100 s/mm2), and one volume without diffusion weighting (10). The scanning parameters were TR/TE of 10000/23 ms, Δ/δ of 12/4 ms, and 6 averages. All data were zero-filled to generate images with a resolution of 128 × 128 pixels.
The apparent diffusion coefficient (ADC), relative cerebral blood flow (rCBF), and FA maps at each time point were calculated using in-house MATLAB (MathWorks, Natick, MA, USA) scripts. The ADC map was calculated using DWIs based on Stejskal-Tanner equation. For rCBF, the concentration-time curves were obtained from the signal-time curves of DSC MRI, followed by a gamma-variate fitting for the recirculation removal (1112). FA maps were calculated from the DTI as follows: where λi were the eigenvalues of the diffusion tenor matrix, and λ̄ was the mean diffusion. The scalar measures q and L represented pure anisotropy and diffusion magnitude, respectively (13).
Perfusion deficit was first defined, based on the criteria that the CBF-defined lesion volume at 3 hours was equal to the infarct volume at 24 hours (14). Because NBO can cause a reduction of CBF in normal brain and improvement of CBF in ischemic regions (9), a lower CBF threshold of 46% reduction was used in this study to identify CBF deficits (13). Abnormal ADC was defined using a reduction of 30% of the contralateral hemisphere with the exclusion of the ventricles (915). To delineate the areas of IC and IP, the rCBF map was first co-registered to the ADC map. IP was then defined as regions showing rCBF values < 54% and ADC > 70%, and IC was identified as regions showing rCBF values < 54% and ADC < 70% of the contralateral homologous brain (Supplementary Fig. 1 in the online-only Data Supplement). The rCBF and ADC of normal tissue (NT) were defined as the averages of the homologous areas of IP and IC values in the contralateral normal hemisphere.
Previous studies have shown variable tissue responses to the ischemic injury in cortical, subcortical gray matter (GM) and white matter (WM) (1617). In this study, we applied an atlas-based tissue classification method during image processing to identify the tissue types within each image voxel for each rat model. Specifically, a FA template of Sprague-Dawley rat brain (18) was co-registered and resampled to the FA map of the rats using a 12-parameter affine transformation implemented by the FLIRT toolbox (19). The tissue atlas originally proposed by Papp et al. (18) differentiates the rat brain into 32 WM regions and 40 GM regions. Further, we categorized 37 cerebral GM regions into cortical and subcortical regions, using the corpus callosum and external capsule as the border landmarks (Supplementary Fig. 2 in the online-only Data Supplement). Finally, the relative DTI metrics, as compared to the contralateral homologous tissue, were calculated as follows: rX = (Xipsilateral - Xcontralateral) / Xcontralateral, where X indicates the value of indices (FA, L or q value).
Statistical analyses were performed to determine whether the post-MCAo DTI metrics can be used to discriminate IP from IC and NT, regardless of the time effect. One-way analysis of variance model with post hoc analysis was applied to evaluate whether the means of DTI metrics within the IP, IC, and NT regions were significantly different at each imaging time point. Receiver operating characteristic (ROC) curve analysis was performed to determine the optimal threshold of rL to differentiate regions among IC, IP, and NT at 1.5 hours. The sensitivity, specificity, and accuracy were then calculated for the selected optimal thresholds. Nonlinear regression analysis using an exponential function to minimize the least squares, was employed to reveal the relationship between the rq values and the time after stroke (20).
Figure 1D illustrates the slight progressive increase of ischemic regions over time, on T2WI after MCAo. The L and q maps demonstrate a consistent hypointensity change in the ischemic areas over all time points (Fig. 1A, B). The FA maps exhibit an initial elevation of FA value at 0.5 hour in the ischemic region, and a later reduction at 6.5 hours after MCAo (Fig. 1C). The FA evolution depends on the changes of rL and rq values. For instance, the evolutions of rL value in IC were stagnant (with an average reduction of 41.28% and 43.25% in cortical and subcortical GM, respectively) (Fig. 2A). However, the rq value in IC exhibited a monotonic decrease, from a reduction of 28% at 0.5 hour to 50% at 6.5 hours, in both cortical and subcortical GM (Fig. 2B). Accordingly, the relatively small reduction of rq as compared to a larger reduction of rL resulted in a 20% increase of FA in the cortical and subcortical GM IC regions during the first 3.5 hours (Fig. 2C). At 6.5 hours, the reduction of rq value became greater than the reduction of rL, leading to a marked decrease in FA (negative rFA values) in the same region. On the other hand, the evolution of rFA in WM IC was relatively stable with a 6.41 ± 1.34% increment due to the similar decay profiles of rL and rq (right column in Fig. 2).
Significant differences (p < 0.05) in rL values were found between IP and IC, and between IP and NT for all tissue subtypes (Fig. 2A). The ROC curves and the optimal rL thresholds for discriminating IP from IC and NT are shown in Figure 3 and Table 1, respectively. The threshold for discriminating IP from IC is relatively constant (about -29%). However, the optimal rL threshold for separating IP from NT shows a significant difference between cortical and subcortical regions (p = 0.022). The results indicate that the tissue-specific rL threshold is an important factor in discriminating between IP and NT. The overall performance of tissue-specific rL thresholds is listed in Table 2, which shows excellent discrimination of IP from IC by rL in all tissue subtypes (average accuracy > 0.95). The discrimination performance between IP and NT is good in the subcortical GM and cortical regions, with an average accuracy of 0.83 and 0.79, respectively, and moderate in the WM region with accuracy at 0.68.
The representative maps of the rL-defined IC and IP (Fig. 4B) as compared with the corresponding perfusion/diffusion-defined IC and IP (Fig. 4A) of a rat suggested that the rL-defined maps can successfully segment the ischemic regions into IP and IC. The T2WIs at 24 hours showed complete infarct of the ipsilateral MCA territory.
A reduction of the rq value in cortical IC from -26.9% to -47.6% within the 6.5 hours after stroke onset was observed (Fig. 2B). A nonlinear regression analysis was further applied to estimate the relationship between the rq values and the time after stroke. The passed time after stroke onset can be assessed by the equation, passed time (in minutes) = 1.636 × exp (-0.115 × rq). This exponential decay of rq value over time suggests that rq value in cortical IC can be a potential surrogate marker to determine the stroke onset time at the hyeracute stage; for instance, an rq value reduction smaller than 44.6% predicts an acute stroke onset less than 4.5 hours (red dashed lines in Fig. 5).
Three important conclusions relating to clinical stroke management were derived from our study results: 1) the brain tissue subtypes (cortical, subcortical GM, and WM) exhibited different responses to ischemic injury, which can be characterized by the temporal evolutions of q and L; 2) discrimination of IP from IC and NT by rL values showed comparable results to the conventional perfusion/diffusion mismatch; 3) a reduction of 44.6% of rq value can be used as a biomarker to determine hyperacute stroke onset at less than 4.5 hours.
Previous studies reported that NBO treatment can delay the perfusion/diffusion mismatch and improve outcome after cerebral ischemia (921). Although the salvageable brain tissue in IP benefits from the NBO treatment, previous studies showed a limited therapeutic effect of NBO on IC (222324). Accordingly, monotonic decreases of rq in IC, indicative of continuous degradations of cell membrane integrity, were observed in all cortical, subcortical GM, and WM regions under NBO. In contrast, our study showed neuroprotective effects of NBO on IP, which led to a relatively stable evolution of rq in IP as opposed to that in IC.
The discrepancy of anisotropy diffusion between GM and WM can be attributed to the differences in the pathophysiologic response to ischemic injury, the biophysical diffusion mechanisms, and the blood supply from collateral circulations. The pathophysiologic responses of myelinated WM to ischemia involve hydropic swelling of oligodendroglial cell bodies and the astrocytic swelling secondary to cytotoxic edema, resulting in axonal changes (2526). The vulnerability of WM can lead to earlier and greater anisotropy changes than GM in acute stroke.
In biophysical diffusion mechanisms, the intra- and extracellular accumulation of fluid, and resultant changes in water diffusion, also differs between GM and WM (2728). Furthermore, mechanisms of cytoskeletal breakdown and disruption of axonal transport were only observed in WM, and therefore can cause further diffusion reduction (2526). Finally, the pial collateral circulation is a critical source of perfusion for the cortical GM after ischemia (29). Previous rodent study has reported that the integrity of native pial collateral circulation is a major contributor to the tissue survival after MCAo (30). Therefore, the existence of collateral circulation may interpret the lesser decrease of diffusion anisotropy in cortical IC (-26.89% of rq) as compared to the larger reduction of anisotropy in WM IC (-38.20% of rq) at 0.5 hour after MCAo (Fig. 2B).
Perfusion/diffusion mismatch has been extensively used to individually identify the salvageable IP and IC regions in acute stroke. However, this advanced imaging technique is limited by several technical and computational demands at the acute setting. First, the injection of gadolinium-based contrast agent requires normal renal function and may potentially cause nephrogenic systemic fibrosis (31). Second, although DSC-derived perfusion parameters correlated well with the quantitative PET findings in acute stroke (32), the DSC technique may overestimate the CBF and underestimate the mean transit time (33). Third, several computational issues need to be carefully addressed when deriving rCBF from DSC data. Gamma-variate fitting is a necessary process to extract the first pass of the contrast agent while reducing noise contamination (11). The rCBF estimation may e affected by the employed deconvolution model, especially in the clinical DSC data (34). Finally, perfusion- and diffusion-weighted images are acquired by using two separate imaging sequences, and therefore post-processing to co-register two image sets are required to improve the accuracy in estimating the mismatch between ADC- and CBF-defined deficits. Our findings suggested that the L-defined maps, calculated from a single DTI sequence, can help discriminate IP from IC (with accuracy > 0.95) which is comparable to the perfusion/diffusion mismatch during hyperacute stroke (Figs. 2A, 4). For the discrimination between IP and NT, the tissue-specific rL thresholds can ensure sufficient accuracies (> 0.79) for tissues in the subcortical GM and cortical region; however, only moderate accuracy can be achieved in the WM areas (0.68) (Table 2). This can be explained by the fact that the WM is a relatively scanty area in the rat brain with limited image pixels, leading to increased vulnerability to inaccuracy.
The time course of diffusion anisotropy in acute ischemic brain has been reported by several human studies (34516) and animal models (1724). These studies described an elevation of FA during the hyperacute stroke phase followed by a decrease of FA in the subacute/chronic phase (34516). Our results also showed a 20% increase of rFA in the cortical and subcortical GM IC regions during the first 3.5 hours, and a subsequent reduction to -10% at 6.5 hours after MCAo (Fig. 2C). The acute increase of FA in ischemic brain can be a combined effect from the increased membrane permeability (35), myelin fiber swelling (35), and the effect of signal to noise ratio on the calculation of the anisotropy indices (36). Furthermore, in terms of mathematics, FA value is determined by the interplay between q and L. Therefore, investigating the individual evolutions of q and L rather than their ratio, may avoid the misinterpretations of diffusion anisotropy. Our result confirmed that the acute increase of FA in the cortical and subcortical GM IC regions was due to the relatively small reduction of rq as compared to a larger reduction of rL. Hence, the value of rq may provide a more accurate measure of changes in diffusion anisotropy caused by ischemic brain injury (13).
Several human studies suggested that diffusion anisotropy measured by FA might help to determine the time of onset in cerebral ischemia (45). Changes of FA were observed by extrapolating patients with different stroke ages irrespective of topography of stroke regions, and therefore the true temporal evolution of diffusion anisotropy during the hyperacute stroke could not be revealed. In our results, the evolutions of rq value in the cortical, subcortical GM, and WM IC regions all exhibited monotonic decreases within the first 6.5 hours of stroke onset (Fig. 2B). By regression analysis, the stroke age of 4.5 hours can be estimated by an rq value of -44.6% (a 44.6% reduction) in the cortical IC regions (Fig. 5). These findings suggest that rq value could be useful in evaluating stroke onset time, particularly in patients with wake-up stroke.
Several methodological concerns must be addressed in our study. First, 100% NBO was given after MCAo and continued for 6.5 hours. Although NBO treatment is helpful to evaluate the IP regions (perfusion/diffusion mismatch) by prolonging the time window, further studies are warranted to clarify if the rq and rL changes during hyperacute stroke under normal atmospheric conditions would produce similar results. Second, the assessment of the DTI changes for rat WM can be limited by the imaging resolution (37). Because the area of a rat WM is much smaller than GM areas, insufficient spatial resolution may result in additional bias caused by the partial volume effect.
In conclusions, our results suggested that DTI could provide a quick and reliable measure to distinguish IP from IC based on rL values, and predict stroke age using the rq in cortical IC during the hyperacute phase. To our knowledge, this is the first study to demonstrate the potential utility of a single DTI sequence to substitute the conventional approach of perfusion/diffusion mismatch, and help the evaluation of stroke age with unknown onset time.
Figures and Tables
Fig. 1
Serial L (A), q (B), FA (C), and T2WI (D) maps of rat for demonstrating spatiotemporal evolutions.
L and q maps showed significant hypointensities on ischemic lesion, while FA maps displayed initial elevation (red arrows) of ischemia with later reduction (white arrows) by 6.5 hours. T2WI showed progressively minor increased intensity in ischemia areas, over time. FA = fractional anisotropy, T2WI = T2-weighted imaging
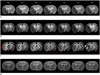
Fig. 2
Temporal evolutions of rL (A), rq (B), and rFA (C) in cortical (left column), subcortical GM (middle column), and WM regions (right column), respectively.
*Indicates significant difference between IP and NT (p < 0.05), †Represents significant difference between IP and IC (p < 0.05). Error bars are ± SEM. GM = gray matter, IC = infarct core, IP = ischemic penumbra, NT = normal tissue, WM = white matter
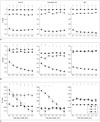
Fig. 3
ROC curves in discriminating IP from IC (blue curves) and NT (red curves) for cortical (A), subcortical GM (B), and WM (C) using rL values. Gray areas indicate range for eight rats.
GM = gray matter, IC = infarct core, IP = ischemic penumbra, NT = normal tissue, ROC = receiver operating characteristic, WM = white matter
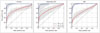
Fig. 4
IC (red) and IP (green) regions defined by perfusion-diffusion mismatch (A) and proposed L-defined method (B) rat at 90 min after MCAo. 24 hr-T2WIs are also displayed to show final infarct regions (C).
Severe edema extending to normal hemisphere is observed in 24 hr-T2WIs. IC = infarct core, IP = ischemic penumbra, MCAo = middle cerebral artery occlusion, T2WI = T2-weighted imaging
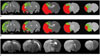
Fig. 5
Relationship between rq value and time after stroke for cortical IC.
Solid curve represents empirical relationship, i.e., elapsed time = 1.636 x exp (-0.115 × rq value), estimated by nonlinear regression analysis. Red dashed lines indicate that 4.5-hr stroke onset can be identified by 44.6% reduction of rq value. Error bars are ± SEM. IC = infarct core
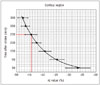
Table 1
Optimal rL Threshold (%) in Discriminating IC, IP, and NT for Each Rat
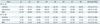
Table 2
Discrimination Performance Based on rL Values
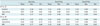
Sensitivity is calculated as proportion of positives that perfusion/diffusion-defined IP voxels are correctly identified as IP by using rL values; specificity is calculated as proportion of negatives that perfusion/diffusion-defined IC or NT voxels are correctly identified as IC or NT based on rL values; accuracy is defined as proportion of true positives and true negatives to overall testing voxels. IC = infarct core, IP = ischemic penumbra, Max = maximum, Min = minimum, NT = normal tissue, WM = white matter
References
1. Hacke W, Kaste M, Bluhmki E, Brozman M, Dávalos A, Guidetti D, et al. Thrombolysis with alteplase 3 to 4.5 hours after acute ischemic stroke. N Engl J Med. 2008; 359:1317–1329.
2. Kang DW, Kwon JY, Kwon SU, Kim JS. Wake-up or unclear-onset strokes: are they waking up to the world of thrombolysis therapy? Int J Stroke. 2012; 7:311–320.
3. Bhagat YA, Hussain MS, Stobbe RW, Butcher KS, Emery DJ, Shuaib A, et al. Elevations of diffusion anisotropy are associated with hyper-acute stroke: a serial imaging study. Magn Reson Imaging. 2008; 26:683–693.
4. Sakai K, Yamada K, Nagakane Y, Mori S, Nakagawa M, Nishimura T. Diffusion tensor imaging may help the determination of time at onset in cerebral ischaemia. J Neurol Neurosurg Psychiatry. 2009; 80:986–990.
5. Puig J, Blasco G, Daunis-I-Estadella J, Thomalla G, Castellanos M, Soria G, et al. Increased corticospinal tract fractional anisotropy can discriminate stroke onset within the first 4.5 hours. Stroke. 2013; 44:1162–1165.
6. Shereen A, Nemkul N, Yang D, Adhami F, Dunn RS, Hazen ML, et al. Ex vivo diffusion tensor imaging and neuropathological correlation in a murine model of hypoxia-ischemia-induced thrombotic stroke. J Cereb Blood Flow Metab. 2011; 31:1155–1169.
7. Chiang T, Messing RO, Chou WH. Mouse model of middle cerebral artery occlusion. J Vis Exp. 2011; (48):2761.
8. Bråtane BT, Walvick RP, Corot C, Lancelot E, Fisher M. Characterization of gadolinium-based dynamic susceptibility contrast perfusion measurements in permanent and transient MCAO models with volumetric based validation by CASL. J Cereb Blood Flow Metab. 2010; 30:336–342.
9. Henninger N, Bouley J, Nelligan JM, Sicard KM, Fisher M. Normobaric hyperoxia delays perfusion/diffusion mismatch evolution, reduces infarct volume, and differentially affects neuronal cell death pathways after suture middle cerebral artery occlusion in rats. J Cereb Blood Flow Metab. 2007; 27:1632–1642.
10. Yao X, Yu T, Liang B, Xia T, Huang Q, Zhuang S. Effect of increasing diffusion gradient direction number on diffusion tensor imaging fiber tracking in the human brain. Korean J Radiol. 2015; 16:410–418.
11. Ostergaard L, Weisskoff RM, Chesler DA, Gyldensted C, Rosen BR. High resolution measurement of cerebral blood flow using intravascular tracer bolus passages. Part I: mathematical approach and statistical analysis. Magn Reson Med. 1996; 36:715–725.
12. Lee EK, Choi SH, Yun TJ, Kang KM, Kim TM, Lee SH, et al. Prediction of response to concurrent chemoradiotherapy with temozolomide in glioblastoma: application of immediate post-operative dynamic susceptibility contrast and diffusion-weighted MR imaging. Korean J Radiol. 2015; 16:1341–1348.
13. Wang W, Steward CE, Desmond PM. Diffusion tensor imaging in glioblastoma multiforme and brain metastases: the role of p, q, L, and fractional anisotropy. AJNR Am J Neuroradiol. 2009; 30:203–208.
14. Meng X, Fisher M, Shen Q, Sotak CH, Duong TQ. Characterizing the diffusion/perfusion mismatch in experimental focal cerebral ischemia. Ann Neurol. 2004; 55:207–212.
15. Shen Q, Meng X, Fisher M, Sotak CH, Duong TQ. Pixel-by-pixel spatiotemporal progression of focal ischemia derived using quantitative perfusion and diffusion imaging. J Cereb Blood Flow Metab. 2003; 23:1479–1488.
16. Bhagat YA, Emery DJ, Shuaib A, Sher F, Rizvi NH, Akhtar N, et al. The relationship between diffusion anisotropy and time of onset after stroke. J Cereb Blood Flow Metab. 2006; 26:1442–1450.
17. Carano RA, Li F, Irie K, Helmer KG, Silva MD, Fisher M, et al. Multispectral analysis of the temporal evolution of cerebral ischemia in the rat brain. J Magn Reson Imaging. 2000; 12:842–858.
18. Papp EA, Leergaard TB, Calabrese E, Johnson GA, Bjaalie JG. Waxholm space atlas of the Sprague Dawley rat brain. Neuroimage. 2014; 97:374–386.
19. Jenkinson M, Bannister P, Brady M, Smith S. Improved optimization for the robust and accurate linear registration and motion correction of brain images. Neuroimage. 2002; 17:825–841.
20. Marquardt DW. An algorithm for least-squares estimation of nonlinear parameters. J Soc Indust Appl Math. 1963; 11:431–441.
21. Henninger N, Bratane BT, Bastan B, Bouley J, Fisher M. Normobaric hyperoxia and delayed tPA treatment in a rat embolic stroke model. J Cereb Blood Flow Metab. 2009; 29:119–129.
22. Singhal AB, Benner T, Roccatagliata L, Koroshetz WJ, Schaefer PW, Lo EH, et al. A pilot study of normobaric oxygen therapy in acute ischemic stroke. Stroke. 2005; 36:797–802.
23. Kim HY, Singhal AB, Lo EH. Normobaric hyperoxia extends the reperfusion window in focal cerebral ischemia. Ann Neurol. 2005; 57:571–575.
24. Flynn EP, Auer RN. Eubaric hyperoxemia and experimental cerebral infarction. Ann Neurol. 2002; 52:566–572.
25. Yam PS, Dewar D, McCulloch J. Axonal injury caused by focal cerebral ischemia in the rat. J Neurotrauma. 1998; 15:441–450.
26. Dewar D, Dawson DA. Changes of cytoskeletal protein immunostaining in myelinated fibre tracts after focal cerebral ischaemia in the rat. Acta Neuropathol. 1997; 93:71–77.
27. Kuroiwa T, Nagaoka T, Ueki M, Yamada I, Miyasaka N, Akimoto H. Different apparent diffusion coefficient: water content correlations of gray and white matter during early ischemia. Stroke. 1998; 29:859–865.
28. Pantoni L, Garcia JH, Gutierrez JA. Cerebral white matter is highly vulnerable to ischemia. Stroke. 1996; 27:1641–1646. discussion 1647.
29. Armitage GA, Todd KG, Shuaib A, Winship IR. Laser speckle contrast imaging of collateral blood flow during acute ischemic stroke. J Cereb Blood Flow Metab. 2010; 30:1432–1436.
30. Zhang H, Prabhakar P, Sealock R, Faber JE. Wide genetic variation in the native pial collateral circulation is a major determinant of variation in severity of stroke. J Cereb Blood Flow Metab. 2010; 30:923–934.
31. Cowper SE, Robin HS, Steinberg SM, Su LD, Gupta S, LeBoit PE. Scleromyxoedema-like cutaneous diseases in renal-dialysis patients. Lancet. 2000; 356:1000–1001.
32. Takasawa M, Jones PS, Guadagno JV, Christensen S, Fryer TD, Harding S, et al. How reliable is perfusion MR in acute stroke? Validation and determination of the penumbra threshold against quantitative PET. Stroke. 2008; 39:870–877.
33. Calamante F, Gadian DG, Connelly A. Quantification of perfusion using bolus tracking magnetic resonance imaging in stroke: assumptions, limitations, and potential implications for clinical use. Stroke. 2002; 33:1146–1151.
34. Wu O, Østergaard L, Weisskoff RM, Benner T, Rosen BR, Sorensen AG. Tracer arrival timing-insensitive technique for estimating flow in MR perfusion-weighted imaging using singular value decomposition with a block-circulant deconvolution matrix. Magn Reson Med. 2003; 50:164–174.
35. Sakai K, Yamada K, Oouchi H, Nishimura T. Numerical simulation model of hyperacute/acute stage white matter infarction. Magn Reson Med Sci. 2008; 7:187–194.
36. Pierpaoli C, Basser PJ. Toward a quantitative assessment of diffusion anisotropy. Magn Reson Med. 1996; 36:893–906.
37. Kim SJ, Choi CG, Kim JK, Yun SC, Jahng GH, Jeong HK, et al. Effects of MR parameter changes on the quantification of diffusion anisotropy and apparent diffusion coefficient in diffusion tensor imaging: evaluation using a diffusional anisotropic phantom. Korean J Radiol. 2015; 16:297–303.
Supplementary Material
The online-only Data Supplement is available with this article at https://doi.org/10.3348/kjr.2017.18.2.269.
Supplementary Fig. 1
Representative perfusion/diffusion mismatch (A) by coregistration of rCBF map (B) and ADC map (C). Perfusion deficit was determined using reduction of 46% of rCBF compared to contralateral hemisphere. Abnormal ADC was defined using reduction of 30% of contralateral hemisphere with exclusion of ventricles. ADC = apparent diffusion coefficient, rCBF = relative cerebral blood flow