Abstract
Objective
To evaluate the technical feasibility, performance, and interobserver agreement of a computer-aided classification (CAC) system for regional ventilation at two-phase xenon-enhanced CT in patients with chronic obstructive pulmonary disease (COPD).
Materials and Methods
Thirty-eight patients with COPD underwent two-phase xenon ventilation CT with resulting wash-in (WI) and wash-out (WO) xenon images. The regional ventilation in structural abnormalities was visually categorized into four patterns by consensus of two experienced radiologists who compared the xenon attenuation of structural abnormalities with that of adjacent normal parenchyma in the WI and WO images, and it served as the reference. Two series of image datasets of structural abnormalities were randomly extracted for optimization and validation. The proportion of agreement on a per-lesion basis and receiver operating characteristics on a per-pixel basis between CAC and reference were analyzed for optimization. Thereafter, six readers independently categorized the regional ventilation in structural abnormalities in the validation set without and with a CAC map. Interobserver agreement was also compared between assessments without and with CAC maps using multirater κ statistics.
Results
Computer-aided classification maps were successfully generated in 31 patients (81.5%). The proportion of agreement and the average area under the curve of optimized CAC maps were 94% (75/80) and 0.994, respectively. Multirater κ value was improved from moderate (κ = 0.59; 95% confidence interval [CI], 0.56-0.62) at the initial assessment to excellent (κ = 0.82; 95% CI, 0.79-0.85) with the CAC map.
Chronic obstructive pulmonary disease (COPD) is a slowly progressing, irreversible airway disease caused by a mixture of airway inflammation and parenchymal destruction (1). COPD is the fourth leading cause of chronic morbidity and mortality in the United States and will become the third leading cause of death worldwide by 2020 (2). The diagnosis and severity assessment of COPD are typically based on the patient's symptoms and the results of spirometry (1); however, spirometry does not display the regional distribution of COPD (3).
In order to assess the regional distribution as well as changes in COPD, various techniques with the use of CT (3, 4) and MR (5, 6) have been introduced. Among them, two-phase xenon ventilation CT was recently found to be feasible for visual classification of regional ventilation abnormalities by comparing the xenon attenuation of structural abnormalities with that of adjacent normal-looking parenchyma in wash-in (WI)/wash-out (WO) images (7, 8). These regional ventilation patterns are well correlated with the various structural abnormalities in COPD (7) and may be further used to evaluate collateral ventilation (7, 8, 9, 10). Visual classification, however, is potentially affected by observer variability given that interobserver agreements in interpreting variable chest CT findings were modest to poor among radiologists (11, 12, 13, 14).
Computer-aided classification (CAC) systems have been previously shown to have the potential to classify regional lung disease patterns on CT scans and to decrease interobserver variability (15, 16, 17, 18). The purpose of our study, therefore, was to evaluate a CAC system for regional ventilation at two-phase xenon-enhanced CT in patients with COPD in terms of technical feasibility, performance, and interobserver agreement.
This single-institution, retrospective study was approved by the institutional review board of our hospital, and informed consent was obtained from all of the patients.
From April 2008 through February 2009, a total of 38 consecutive patients (36 men, 2 women; mean age, 65.9 years; age range, 46-78 years) who met the diagnostic criteria for COPD (1) underwent two-phase xenon ventilation CT, and they were included in this study. These patients were identical to the initially enrolled patients in a previous two-phase xenon ventilation study (7). Predominant lung diseases included emphysema in 32 patients, tuberculosis-destroyed lung in three patients, bronchiectasis in two patients, and postinfectious bronchiolitis obliterans in one patient.
Patients underwent xenon ventilation using tightly-fitting face masks (King Systems Co., Noblesville, IN, USA) designed to deliver positive pressure ventilation. The xenon gas was a mixture of 30% nonradioactive xenon and 70% oxygen. The patients inhaled the xenon gas for approximately 1 minute during the WI period and 100% oxygen for 2 minutes during the WO period with the use of a xenon gas inhalation system (Zetron V; Anzai Medical, Tokyo, Japan). Patients were instructed to breathe normally during the WI and WO periods. The respiratory rate, oxygen saturation, blood pressure, as well as tidal carbon dioxide and xenon concentrations were closely monitored under the supervision of a chest radiologist. After the CT examination, all of the patients were observed for 30 minutes.
All of the patients were imaged on a CT scanner (Somatom Definition; Siemens Medical Solutions, Forchheim, Germany) during breath holding at full inspiration. Pre-xenon CT was performed using a single tube with a tube voltage of 120 kV, tube current-time product of 150 mAs (reference effective milliampere seconds), and collimation of 64 × 0.6 mm that covered the entire thorax in a caudocranial acquisition. After xenon inhalation, two-phase CT scans were performed using the same coverage. The CT scan during the WI period was taken at approximately 1 minute after the initiation of xenon inhalation when the xenon concentration reached 30%. The CT scan during the WO period was usually obtained at 80-90 seconds when xenon reached zero on the tidal xenon concentration curve after stopping xenon inhalation. Scanning parameters for xenon ventilation CT were as follows: a 512 × 512-pixel matrix, 14 × 1.2-mm collimation, 51 mAs (reference effective milliampere seconds) at 140 kV and 213 mAs (reference effective milliampere seconds) at 80 kV, a pitch of 0.45, and a 0.33-second rotation time. Images were reconstructed with 1.5-mm thickness, 1.2-mm increment, and using soft-tissue reconstruction kernels (B30f for pre-xenon images and D30f for xenon images).
Wash-in and WO images were obtained with Syngo Dual Energy (Siemens Medical Solutions, Forchheim, Germany), which was based on the material decomposition theory (19). To assess data of the whole lung, the minimum value was set at -1024 Hounsfield units (HU) and the maximum value was set at -500 HU. Color-coded maps displaying a progression from blue, sky blue, green, and yellow to red in relation to HU ranging from 1 to 50 were applied to the WI and WO images.
Our proposed CAC system for regional ventilation at two-phase xenon CT consists of lung segmentation, deformable registration, determination of xenon attenuation types, pattern classification of regional ventilation, and generation of a color-coded map (Fig. 1).
To restrict the registration to the lung parenchyma, the left and right lungs were separately segmented using the three-dimensional (3D) region growing method with a threshold of -400 HU for the lung and airway, and then -950 HU for the airway with subsequent subtraction technique (20). To correct positional differences and local deformation of the lungs, the lungs on WO images were globally aligned to the WI image using affine registration based on narrow-band distance propagation, and affine-transformed lungs were nonlinearly deformed by a demon algorithm using a combined gradient force and active cells (21). Then, each pixel in the WI and WO images was assigned to one of the three attenuation types through Equation 1 (below). Lower and upper threshold values were estimated by calculating the mean and standard deviation of xenon attenuation histograms through Equations 2 and 3. Weight w was automatically estimated by calculating the ratio of the normal area to the whole lung. The normal-attenuating lung was defined as the HU of pixels -950 HU or greater in the original CT images (Fig. 2). where, t(x) is the attenuation type of pixel x, A(x) is the xenon attenuation of a pixel x, µ is the mean value of the xenon attenuation histogram, σ is the standard deviation of the xenon attenuation histogram, w is weight, and α, β are coefficients for weight.
The assigned attenuation types were used to classify the corresponding pixel of WI and WO images into the following four ventilation patterns (Fig. 3): pattern A (iso-attenuation or high attenuation in the WI image and iso-attenuation in the WO image), pattern B (iso-attenuation or high attenuation in the WI image and high attenuation in the WO image), pattern C (low attenuation in both the WI and WO images), and pattern D (low attenuation in the WI image and iso-attenuation or high attenuation in the WO image). For the visual pattern recognition of CAC maps; blue, sky blue, red, and yellow were each designated to patterns A, B, C, and D in color-coded maps.
After reviewing all of the structural abnormalities in the pre-xenon dual source CT images, a predominant xenon ventilation pattern for each structural abnormality on WI/WO images was assessed in consensus by two radiologists with experience in two-phase xenon ventilation CT (Fig. 4). For assessment of the predominant xenon ventilation pattern for each structural abnormality, a color overlay of xenon attenuation in the corresponding area on WI/WO images was compared with that in the adjacent normal-attenuating lung showing normal xenon enhancement. The assessed ventilation pattern in consensus served as the reference standard.
Two series of image datasets of structural abnormalities were randomly extracted for optimization and validation. Both the optimization and validation datasets consisted of a total of eighty image datasets including twenty structural abnormalities per pattern. Each image dataset contained axial CT, WI, and WO images, and a CAC map. A single freehand region of interest (ROI) covering the structural abnormality on CT was drawn and pasted into the WI and WO images, and the CAC map, so that the location of the structural abnormality could be easily identified by one of the authors. The average area of ROI was maintained at approximately 0.5 cm2 (range, 0.26-0.69 cm2).
The proposed CAC system was optimized by qualitative and quantitative comparisons of CAC candidate models with the reference. A series of CAC candidate models were generated by applying a series of candidate coefficients (α, β) of weight used in an equation for two thresholds (Tlow, Thigh) with one digit after the decimal point. To narrow down the number of candidate coefficients to less than five, CAC maps for eight image datasets were preliminarily generated using coefficients ranging from 0.1 to 2.0. The predominant ventilation pattern of the ROI on CAC maps was visually compared to the reference by a radiologist. The top five candidate coefficients, for which the CAC map was in good agreement with the reference, were chosen. If CAC maps generated by candidate coefficients did not definitively work out when compared to the reference, those candidate coefficients were discarded even if the number of remnant candidate coefficients was less than five. After narrowing down the number of candidate coefficients, the same review processes were performed for the full optimization set with a series of CAC maps based on those coefficients. The agreement between the CAC map and the reference was calculated on a per-lesion basis for qualitative comparison. Quantitative comparison was performed on a per-pixel basis. The number of pixels on the CAC map within the ROI in agreement with the reference was calculated.
Six radiologists with 20, 9, 8, 7, 6, and 6 years of experience in interpreting CT scans, respectively reviewed the validation image datasets. The validation image datasets were presented in a predetermined randomized order, which was the same for each reader. Each reader was blinded to the clinical information and independently classified the ventilation pattern of ROIs twice sequentially without and with a CAC map. Prior to reviewing the validation image dataset, each reader was provided with instructions including the definition and representative images of each ventilation pattern in WI and WO images, and the CAC map. The interpretation time was not limited.
The CAC model with the highest proportion of agreement for all patterns was considered to have been qualitatively optimized. For quantitative comparison, an area under the receiver operating characteristic curve was assessed with regard to the number of pixels on the CAC map within the ROI in agreement with the reference for each pattern. The CAC system having the largest average area under the curve was considered to have been quantitatively optimized.
The average percentage of agreement between reader pairs on pattern classification per image set was evaluated for validating the optimized CAC system. The number of image sets with complete agreement across all readers was calculated. Interobserver agreement for the pattern classification was determined using multirater Cohen's kappa value (22). A κ value of less than 0.20 indicated poor agreement; a κ value of 0.21-0.40, fair agreement; a κ value of 0.41-0.60, moderate agreement; a κ value of 0.61-0.80, good agreement; and a κ value of more than 0.81, excellent agreement. Interobserver agreement between reader pairs was also calculated. All analyses were compared between assessments without a CAC map and with a CAC map. Statistical analyses were performed using the SPSS package (SPSS 21.0, SPSS Inc., Chicago, IL, USA). A two-sided significance level of 5% was considered to indicate statistical significance.
Computer-aided classification maps were successfully generated in 31 of 38 patients (81.5%). Segmentation of the right and left lungs failed in seven patients. Optimized coefficient of weight for Thigh was directly judged to be 1.0 as CAC maps with other candidate coefficients clearly misclassified the ventilation pattern of ROIs. Candidate coefficients of weight for Tlow were narrowed from 0.3 to 0.7. Qualitative and quantitative analyses revealed that the optimized coefficient for Tlow was 0.5, with the proportion of agreement and average area under the curve of optimized CAC maps being 94% (75/80) and 0.994, respectively (Tables 1, 2) (Figs. 5, 6).
The number of cases in agreement between reader pairs regarding the classification of ventilation patterns ranged from 47 to 68 cases (mean, 55.5; standard deviation, 5.7) without a CAC map, and from 63 to 75 cases (mean, 70.2; standard deviation, 3.3) with a CAC map. The mean percentage of agreement between reader pair results increased from 55.4% without a CAC map to 69.2% with a CAC map. The number of cases reaching complete agreement among all readers doubled from 26 cases (32.5%) to 54 cases (67.5%). Multirater kappa value showed a significant increase from 0.59 (95% confidence interval [CI]: 0.56, 0.62) to 0.82 (95% CI: 0.79, 0.85). Among the patterns, interobserver agreement of pattern B was the highest regardless of the CAC map (without CAC; kappa, 0.72, 95% CI, 0.57-0.87; with CAC; kappa, 0.91, 95% CI, 0.75-1.00) (Table 3). Kappa values among all reader pairs ranged from 0.45 to 0.80 at the initial assessment and from 0.72 to 0.92 with a CAC map (Table 4).
In this study, we developed and optimized the CAC system for visual ventilation pattern analysis at two-phase xenon ventilation CT. The optimized CAC system was qualitatively and quantitatively proven to properly classify the pattern of regional xenon ventilation and to decrease interobserver variability regarding visual classification of regional ventilation patterns. In developing the CAC system, establishing thresholds (Tlow, Thigh) was a critical issue, and simple automatic application of mean and standard deviation of weight was proven to show less optimal performance. Therefore, we devised coefficients (α, β) for weight in determining the thresholds and optimized the CAC by selecting coefficients that showed the best performance.
The pattern classification of regional xenon ventilation was proposed to reflect the presence of inflow and outflow limitations, and collateral ventilation (7). Iso- or high attenuation in the WI image and iso-attenuation in the WO image (pattern A) means no inflow and outflow limitations, which is the typical ventilation of a normal lung and the most predominant pattern even in cases of emphysema. Iso- or high attenuation in the WI image and high attenuation in the WO image (pattern B) means delayed WO of xenon suggesting an outflow limitation. Low attenuation in the WI image in patterns C and D means delayed WI of xenon suggesting an inflow limitation. The difference between patterns C and D is the presence of collateral ventilation causing high attenuation in the WO image in pattern D. Although this proposed classification at two-phase xenon CT did not fundamentally validate whether the classified patterns of regional ventilation properly reflected the actual regional ventilation, it may have a clinical significance in understanding the heterogeneous phenotypes of COPD (23), and in identifying collateral ventilation in bronchoscopic lung volume reduction (24, 25).
Despite the potential of xenon CT in classifying ventilation patterns in COPD patients, interobserver agreements on pattern classification of regional ventilation among reviewers were limited to fair agreement as mentioned in our earlier concerns on observer variability. This may be related to the use of a relatively small size of ROIs in our study as a predominant pattern of regional ventilation may be more easily recognized in larger-sized ROIs. However, it was difficult to choose a large-sized ROI as regional ventilation is at times inhomogeneous within a single structural abnormality. In addition, pattern A of regional ventilation was much more predominant than the other patterns and this makes a larger-sized ROI inapplicable.
Despite the potential of xenon CT in classifying ventilation patterns in COPD patients, interobserver agreements on pattern classification of regional ventilation among reviewers were limited to fair agreement as mentioned in our earlier concerns on observer variability. This may be related to the use of a relatively small size of ROIs in our study as a predominant pattern of regional ventilation may be more easily recognized in larger-sized ROIs. However, it was difficult to choose a large-sized ROI as regional ventilation is at times inhomogeneous within a single structural abnormality. In addition, pattern A of regional ventilation was much more predominant than the other patterns and this makes a larger-sized ROI inapplicable.
Interobserver agreements among the reviewers improved irrespective of the type of ventilation pattern with review of CAC maps. Among the four patterns, the highest interobserver agreement was achieved in pattern B. When we consider that high xenon attenuation is only needed to designate pattern B, readers might have had more difficulties in differentiating between iso- and low-attenuation than in differentiating between iso- and high-attenuation in the WI and WO images. This may be associated with the asymmetric distribution of xenon in the normal-attenuating lung in the WI and WO images. Although the normal-attenuating lung showed a wide spectrum of xenon attenuation, the most frequent attenuation of the normal-attenuating lung was around 25 HU or less in the range of 0 to 50 in the WI image. The most frequent attenuation in the WO image was around 15 HU or less. Accordingly, readers had a narrower color range for differentiating between iso- and low-attenuation than for differentiating between iso- and high-attenuation, especially in the WO image. These difficulties in differentiating between relative xenon attenuation can increase in patients with a smaller portion of the normal-attenuating lung.
In this study, the right and left lungs could not be automatically segmented in seven patients during the registration of lung parenchyma. This failure of lung segmentation occurred in areas that were in close contact with the pleura of both lungs, also known as anterior and posterior junctional lines. These junctional lines were too thin to be perceived by the 3D region growing method, and therefore, both lungs were fused around the junctional lines after the automatic segmentation. Even though the possible failure of lung segmentation might be a potential limitation of our CAC system, we believe that this limitation can be overcome by manual segmentation in such cases. Indeed, we succeeded in generating CAC maps in the patients with failure of lung segmentation by performing manual segmentation.
There are several limitations to our study. First, the number of cases was relatively small. However, we validated the proposed CAC system using image datasets that were totally different from those used during optimization. Second, neither this proposed CAC system nor the readers involved in the review of validation set considered the effect of artifacts, which can lead to misclassification of the actual regional xenon ventilation. Therefore, when this approach is applied in clinical practice, readers should check for the presence of artifacts within the ROIs by reviewing WI and WO images prior to accepting the results of the CAC map. Third, the regional ventilation pattern at xenon-enhanced CT could not fundamentally validate whether the classified pattern of regional ventilation properly reflected the actual regional ventilation. A further investigation is needed to compare the results of CAC system with functional information obtained from other functional imaging techniques or with lung pathology.
In conclusion, our proposed CAC system demonstrated the potential to improve interobserver variability in classifying regional ventilation patterns at xenon ventilation CT using a dual-energy technique.
Figures and Tables
Fig. 1
Overall strategy of CAC system with multi-step post-processing procedures. CAC = computer-aided classification, WI = wash-in, WO = wash-out

Fig. 2
Determination of xenon attenuation in CAC system.
A. Estimation of thresholds in xenon attenuation histogram. Two thresholds (Tlow, Thigh) are estimated by calculating mean and standard deviation of xenon attenuation histogram. t(x) is attenuation type of pixel x, A(x) is xenon attenuation of pixel x, µ is mean value of xenon attenuation histogram, σ is standard deviation of xenon attenuation histogram, w is weight, and, α, β are coefficients for weight. B. Representative image of automatic estimation of weight for thresholds using ratio of normal area to whole area using sigma function. Yellow represents abnormal low-attenuating parenchyma with attenuation values less than -950 HU. Weight w is automatically estimated by calculating ratio of normal area to whole lung. CAC = computer-aided classification, HU = Hounsfield units

Fig. 3
Decision rule of CAC system in classifying regional ventilation patterns. CAC = computer-aided classification, WI = wash-in, WO = wash-out

Fig. 5
Representative images of patterns A and C for structural abnormalities in 64-year-old male with Gold stage I emphysema.
Large subpleural bulla in right basal lung on CT (A; arrows) shows high attenuation on wash-in xenon-enhanced image (B) and iso-attenuation on wash-out xenon-enhanced image (C). This ventilation pattern is compatible with pattern A shown on CAC map in blue (D). Small subpleural bulla anterior to large bulla on CT (A; arrowhead) shows low attenuation on wash-in (B) and wash-out xenon-enhanced images (C). This ventilation pattern is in agreement with pattern C and is correctly visualized on CAC map in red (D). CAC = computer-aided classification
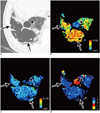
Fig. 6
Representative image of patterns B and D for structural abnormalities in 72-year-old male with Gold stage II emphysema.
Small subpleural bulla in right upper lobe on CT (A; arrowheads) shows high attenuation on wash-in (B) and wash-out xenon-enhanced images (C), which is compatible with pattern B shown in CAC map in sky blue (D). Large subpleural bulla in right lower lobe on CT (A; arrows) shows low attenuation on wash-in (B) and wash-out xenon-enhanced images (C). This ventilation pattern is in agreement with pattern D and is correctly visualized on CAC map in yellow (D). CAC = computer-aided classification

Table 4
Interobserver Agreement for All Patterns among All Reader Pairs without and with CAC Maps Using Kappa Statistics

Note.- Kappa values among all reader pairs ranged from 0.45 to 0.80 without CAC maps and ranged from 0.72 to 0.92 after reviewing CAC maps. Readers 1 through 6 have twenty years, nine years, eight years, seven years, and six years (readers 5 and 6) of experience in interpreting CT scans, respectively. CAC = computer-aided classification
References
1. Rabe KF, Hurd S, Anzueto A, Barnes PJ, Buist SA, Calverley P, et al. Global strategy for the diagnosis, management, and prevention of chronic obstructive pulmonary disease: GOLD executive summary. Am J Respir Crit Care Med. 2007; 176:532–555.
2. Murray CJ, Lopez AD. Alternative projections of mortality and disability by cause 1990-2020: Global Burden of Disease Study. Lancet. 1997; 349:1498–1504.
3. Matsuoka S, Yamashiro T, Washko GR, Kurihara Y, Nakajima Y, Hatabu H. Quantitative CT assessment of chronic obstructive pulmonary disease. Radiographics. 2010; 30:55–66.
4. Yoon SH, Goo JM, Goo HW. Quantitative thoracic CT techniques in adults: can they be applied in the pediatric population? Pediatr Radiol. 2013; 43:308–314.
5. Driehuys B, Martinez-Jimenez S, Cleveland ZI, Metz GM, Beaver DM, Nouls JC, et al. Chronic obstructive pulmonary disease: safety and tolerability of hyperpolarized 129Xe MR imaging in healthy volunteers and patients. Radiology. 2012; 262:279–289.
6. Kirby M, Mathew L, Heydarian M, Etemad-Rezai R, McCormack DG, Parraga G. Chronic obstructive pulmonary disease: quantification of bronchodilator effects by using hyperpolarized 3He MR imaging. Radiology. 2011; 261:283–292.
7. Park EA, Goo JM, Park SJ, Lee HJ, Lee CH, Park CM, et al. Chronic obstructive pulmonary disease: quantitative and visual ventilation pattern analysis at xenon ventilation CT performed by using a dual-energy technique. Radiology. 2010; 256:985–997.
8. Kim WW, Lee CH, Goo JM, Park SJ, Kim JH, Park EA, et al. Xenon-enhanced dual-energy CT of patients with asthma: dynamic ventilation changes after methacholine and salbutamol inhalation. AJR Am J Roentgenol. 2012; 199:975–981.
9. Chae EJ, Seo JB, Kim N, Song KS, Shin JH, Kim TH, et al. Collateral ventilation in a canine model with bronchial obstruction: assessment with xenon-enhanced dual-energy CT. Radiology. 2010; 255:790–798.
10. Goo HW, Yang DH, Kim N, Park SI, Kim DK, Kim EA. Collateral ventilation to congenital hyperlucent lung lesions assessed on xenon-enhanced dynamic dual-energy CT: an initial experience. Korean J Radiol. 2011; 12:25–33.
11. Hersh CP, Washko GR, Jacobson FL, Gill R, Estepar RS, Reilly JJ, et al. Interobserver variability in the determination of upper lobe-predominant emphysema. Chest. 2007; 131:424–431.
12. Mets OM, Smit EJ, Mohamed Hoesein FA, Gietema HA, Bokkers RP, Attrach M, et al. Visual versus automated evaluation of chest computed tomography for the presence of chronic obstructive pulmonary disease. PLoS One. 2012; 7:e42227.
13. Gierada DS, Pilgram TK, Ford M, Fagerstrom RM, Church TR, Nath H, et al. Lung cancer: interobserver agreement on interpretation of pulmonary findings at low-dose CT screening. Radiology. 2008; 246:265–272.
14. Watadani T, Sakai F, Johkoh T, Noma S, Akira M, Fujimoto K, et al. Interobserver variability in the CT assessment of honeycombing in the lungs. Radiology. 2013; 266:936–944.
15. Park SO, Seo JB, Kim N, Park SH, Lee YK, Park BW, et al. Feasibility of automated quantification of regional disease patterns depicted on high-resolution computed tomography in patients with various diffuse lung diseases. Korean J Radiol. 2009; 10:455–463.
16. Sluimer IC, van Waes PF, Viergever MA, van Ginneken B. Computer-aided diagnosis in high resolution CT of the lungs. Med Phys. 2003; 30:3081–3090.
17. Uppaluri R, Hoffman EA, Sonka M, Hartley PG, Hunninghake GW, McLennan G. Computer recognition of regional lung disease patterns. Am J Respir Crit Care Med. 1999; 160:648–654.
18. Jeon KN, Goo JM, Lee CH, Lee Y, Choo JY, Lee NK, et al. Computer-aided nodule detection and volumetry to reduce variability between radiologists in the interpretation of lung nodules at low-dose screening computed tomography. Invest Radiol. 2012; 47:457–461.
19. Chae EJ, Seo JB, Goo HW, Kim N, Song KS, Lee SD, et al. Xenon ventilation CT with a dual-energy technique of dual-source CT: initial experience. Radiology. 2008; 248:615–624.
20. Yim Y, Hong H. Correction of segmented lung boundary for inclusion of pleural nodules and pulmonary vessels in chest CT images. Comput Biol Med. 2008; 38:845–857.
21. Yim Y, Hong H, Shin YG. Deformable lung registration between exhale and inhale CT scans using active cells in a combined gradient force approach. Med Phys. 2010; 37:4307–4317.
22. Crewson PE. Reader agreement studies. AJR Am J Roentgenol. 2005; 184:1391–1397.
23. Han MK, Agusti A, Calverley PM, Celli BR, Criner G, Curtis JL, et al. Chronic obstructive pulmonary disease phenotypes: the future of COPD. Am J Respir Crit Care Med. 2010; 182:598–604.
24. Shah PL, Hopkinson NS. Bronchoscopic lung volume reduction for emphysema: where next? Eur Respir J. 2012; 39:1287–1289.
25. Shah PL, Geddes DM. Collateral ventilation and selection of techniques for bronchoscopic lung volume reduction. Thorax. 2012; 67:285–286.