Abstract
Objective
To evaluate the effect of computer-aided detection (CAD) system on observer performance in the detection of malignant lung nodules on chest radiograph.
Materials and Methods
Two hundred chest radiographs (100 normal and 100 abnormal with malignant solitary lung nodules) were evaluated. With CT and histological confirmation serving as a reference, the mean nodule size was 15.4 mm (range, 7-20 mm). Five chest radiologists and five radiology residents independently interpreted both the original radiographs and CAD output images using the sequential testing method. The performances of the observers for the detection of malignant nodules with and without CAD were compared using the jackknife free-response receiver operating characteristic analysis.
Results
Fifty-nine nodules were detected by the CAD system with a false positive rate of 1.9 nodules per case. The detection of malignant lung nodules significantly increased from 0.90 to 0.92 for a group of observers, excluding one first-year resident (p = 0.04). When lowering the confidence score was not allowed, the average figure of merit also increased from 0.90 to 0.91 (p = 0.04) for all observers after a CAD review. On average, the sensitivities with and without CAD were 87% and 84%, respectively; the false positive rates per case with and without CAD were 0.19 and 0.17, respectively. The number of additional malignancies detected following true positive CAD marks ranged from zero to seven for the various observers.
Chest radiography is the most commonly used method for the evaluation of lung parenchyma in clinical practice. It is a fast, relatively inexpensive, and easily achievable imaging tool with low radiation exposure. However, it is not without its problems; malignant lung nodules are frequently overlooked and missed on chest radiograph, even by experienced radiologists (1-4), and many of them detected retrospectively (2-4).
Thus, to improve the diagnostic accuracy of chest radiography, technical advances including computer-aided detection (CAD) techniques have been developed and investigated to overcome the intrinsic limitations of human perception. With this CAD technique, similar to reports on chest CT (5-7), several studies generally indicate that CAD can indeed improve the detection rate of subtle lung nodules on chest radiograph (8-15). However, these studies evaluated the performance of a CAD system only (10, 12), reported the results of observer performance test only with sensitivities (6, 7, 11), or conventional receiver operating characteristic analysis that does not require the localization of nodules (5, 8). Most recently, de Hoop et al. (16) reported that CAD did not significantly improve observer performance in nodule detection on chest radiograph mainly because readers had difficulty in differentiating TP from FP CAD markings. With these conflicting results, the role of CAD as a second reader in clinical practice still remains uncertain and further investigation is necessary. In comparison to the study population was limited to a lung cancer screening group in the study by de Hoop et al. (16), we also included the chest radiographs with diagnostic purpose, which is more frequent in clinical practice.
In this study, we observed the effect of the CAD system on observer performance using a jackknife free-response receiver operating characteristic (JAFROC) analysis in the detection of nodules on chest radiographs with CT and histopathologic results as the reference standard. Furthermore, the sensitivity and number of false positive marks were also estimated.
Institutional review board approval was obtained, and the requirement for informed patient consent was waived in this retrospective study.
From the database of the Department of Radiology in our hospital between January 2004 and May 2008, we retrospectively reviewed and included 100 consecutive patients with solitary malignant pulmonary nodules on chest radiography and 100 normal control cases. The study population consisted of 103 men and 97 women that had a mean age of 56 years (age range, 21-86 years).
Patient inclusion criteria were the presence of a solitary malignant pulmonary nodule measuring 20 mm or less in diameter with pathologic proof visible on chest radiograph, and chest radiograph performed within 1 month of a chest CT. Nodule size was measured on chest radiograph with an electronic caliper. In the control group, the absence of lung nodules was confirmed on chest CT and chest radiography was performed within 1 month of chest CT in all cases.
All radiographs were obtained using either a flat-panel detector system (Digital Diagnost; Philips, Best, the Netherland) with a cesium iodide scintillator or a computed radiography system (FCR-5501; Fuji, Tokyo, Japan) with a 35 × 43 cm imaging plate (ST-55BD, Fuji, Tokyo, Japan). The flat-panel system had a matrix of 3001 × 3001 pixels × 12 bits, and a panel size of 43 × 43 cm. The automatic exposure control mode was adjusted to 400-speed, and exposure parameters were 125 kVp and 400 mA, with a grid ratio of 10 : 1. The computed radiography images had a matrix of 1760 × 2140 pixels × 10 bits and were obtained at 100 kV, 320 mA, and 0.012 second with a 10 : 1 grid. All chest radiographs were obtained using the default post-processing algorithms specified by the manufacturer. All digital images were transferred to a Picture Archiving and Communication System (PACS).
Ten readers including 5 chest radiologists and 5 residents participated in this observer performance study. Of the 5 residents, 3 were first year residents and 2 were 4th year residents.
Readers independently interpreted both the original radiographs and CAD output images in the sequential testing mode using the PACS workstation on a 5 megapixel LCD monitor (M511L; Totoku, Tokyo, Japan). Readers marked the detected nodule on a paper on which the corresponding chest radiograph printed and recorded the confidence ratings for the presence of a nodule ranging from 1 to 100. A 100 rating implied that a nodule was present with absolute certainty. In calculating sensitivity, confidence ratings from 0 to 49 were considered negative and confidence ratings from 50 to 100 were considered positive.
All 200 cases were randomly distributed into 4 interpretation sessions with a 1 week interval between sessions, and in each session, an observer interpreted 50 cases. For each observer, the reading order of the 50 chest radiographs in each group was randomly varied. Reading time was not limited; however, actual reading time was monitored. Before the performance study to familiarize the observers with the CAD system, all observers were instructed with 5 training cases which were not included in the observer test.
The reference standard for the presence and location of nodules was based on the review of CT performed within 1 month of the index chest radiography by one radiologist who did not participate in the observer performance study. Both true positive (TP) and false positive (FP) rates were assessed. The reference standard for the malignancy of nodules was established histopathologically in all cases.
All images were analyzed by using a commercially available chest radiographic CAD system (IQQA-Chest, EDDA Technology, Princeton Junction, NJ, USA), as previously described (14). The incorporation of this program into PACS provided a secondary evaluation of chest radiographs for occult pulmonary nodules. According to the manufacturer, the algorithm was optimized to detect nodules 5-15 mm in diameter; although in practice, it also marks larger and smaller nodules. Candidate areas are presented as semitransparent circles for readers to review. The CAD program was run by the readers following completion of the initial visual interpretation of the radiographs, and provided highlighted suspect areas with regions of interest (ROIs). The observers then had the opportunity to accept or discard any CAD marked ROIs.
To assess observer performance in the detection of malignant nodules with and without CAD, JAFROC analysis was performed using a maximum likelihood estimation program (JAFROC, version 3; downloaded from http://www.devchakraborty.com) (17). This method combines the elements of free-response receiver operating characteristics and the Dorfman-Berbaum-Metz methods. Mean diagnostic accuracy was calculated according to the mean figure of merit (FOM), defined as the probability that a lesion is rated higher than the highest rated non-lesion on normal images.
Sensitivity was calculated as the number of TP markings divided by the total number of malignancies. FP fraction was also calculated as the number of FP markings divided by the total number of chest radiographs. McNemar's test was used to compare reader sensitivity before and after CAD.
Since it is controversial whether the application of CAD as a second reader also allows for discharge of candidates seen without CAD (18), we also evaluated a situation in which the observers could only increase their confidence level after CAD markings, while preserving all lesion locations seen without CAD (16). For all analyses, a p value of less than 0.05 was considered statistically significant.
Reading time, averaged over the 10 observers was 139 minutes. Reader 2 was the fastest at 84 minutes, while Reader 7 was the slowest at 239 minutes.
Mean nodule diameter was 15.4 mm with a range of 7 mm to 20 mm. A mean nodule subtlety score of 5.2 out of 10 was determined by one chest radiologist who did not participate in the observer study. A pathology assessment revealed that of the 100 malignant nodules, 97 were primary lung cancers (79 adenocarcinomas, 11 squamous cell carcinomas, 7 others) and 3 nodules were metastatic nodules (2 from breast cancer, 1 from hepatocellular carcinoma). Figure 1 shows the sites of the 100 malignant nodules. Ninety-three nodules were located in the unobscured lung, four nodules in the overlapped area of the clavicle and the rib, two in the retrocardiac area, and one in the azygoesophageal recess.
Fifty-nine malignant nodules out of 100 were detected by the CAD system with a false positive rate of 1.9 nodules per chest radiograph (range, 0 to 5 nodules). CAD detected 4 of the 8 nodules which were detected by only three or less observers. However, among the 50 nodules which all of the observers detected without CAD, the CAD system alone could not detect 16 malignant nodules (32%). Nodule subtlety was not significantly different between the CAD-detected nodules (5.2) and CAD-missed nodules (5.2) (p = 0.98). Nodule diameter was also not significantly different between the CAD-detected nodules (15.34 mm) and CAD-missed nodules (15.22 mm) (p = 0.84).
Without CAD, the average FOM of all ten observers was 0.90 (Table 1). In a subgroup analysis, the average FOM was 0.93 for radiologists and 0.87 for residents. The radiologists had an average sensitivity of 85.2%, with 0.03 FP annotations per chest radiograph. The residents had an average sensitivity of 82.8%, with 0.28 FP annotations per chest radiograph.
All of the 100 malignant nodules were detected by at least one observer. Fifty of the 100 (50%) nodules were detected by all ten observers without the use of CAD. In addition, 19 nodules were detected by nine observers, 11 were detected by eight, 2 by seven, 3 by six, 6 by five, 1 by four, 4 by three, 2 by two observers, and 2 malignant nodules by only one radiologist without the use of CAD. The median value of nodule subtlety for 50 nodules detected by all 10 observers was 6 and that for 8 nodules detected by three or less observers was 2.
When the observers were allowed to freely adjust their confidence ratings depending on CAD markings, the average FOM of all ten observers increased from 0.90 to 0.91, but was not statistically significant. The average FOM of radiologists and residents increased from 0.93 to 0.94 and 0.87 to 0.88 respectively, although the differences were not statistically significant (Table 1).
While most observers revealed increased sensitivity and FOM with the CAD annotation, only one first-year resident, Reader 6, showed contrary results - her sensitivity and FOM decreased after the CAD annotation. Because Reader 6 showed an unusually low performance in reading chest radiographs and interpreting the CAD results, a statistical analysis was also performed on the nine of the 10 readers, excluding Reader 6. When one first-year resident (Reader 6) was excluded from the analysis, the average FOM improved from 0.90 to 0.92 with statistical significance (p = 0.04). An individual analysis was also performed showed significant improvement in the FOM value in two radiologists and two residents.
On average, the sensitivities with and without CAD were 87% and 84%; false positive rates per case with and without CAD were 0.19 and 0.17; and three more malignant nodules were detected with CAD.
For the subgroup analysis, the average sensitivity increased from 85% to 89% (range, 86-91%) for radiologists and from 83% to 85% for residents. The average number of FP detections per chest radiograph remained virtually unchanged in the radiologist subgroup (0.03 to 0.04) and in the resident subgroup (0.28 to 0.31).
Although CAD-alone sensitivity (59%) was much lower, reader sensitivity improved after application of CAD in all observers except one first year resident (Reader 6). In this first year resident had the highest sensitivity, the false positive rate was also two to twelve times higher than other observers. This resident rejected many nodules that CAD did not detect.
When readers were only allowed to increase their confidence scores after obtaining CAD results, average FOM of all 10 observers increased from 0.90 to 0.91, and was statistically significant (p = 0.04) (Table 2).
On average, the sensitivities with and without CAD were 88% and 84%, respectively; however, the false positive rates per case with and without CAD were 0.25 and 0.17, respectively. Four more malignant nodules were detected with CAD.
For the subgroup analysis, the average sensitivity increased from 85% to 89% for radiologists and from 83% to 87% (range, 81-92%) for residents. The average number of FP annotations per chest radiograph remained virtually unchanged in the chest radiologist subgroup (0.03 to 0.04) and increased from 0.28 to 0.41 in the resident subgroup.
In total, 10 observers added 39 TP nodules after reviewing the CAD marks. The number of additional malignancies detected following TP CAD marks ranged from zero to seven for the various observers. Though 6 of 10 observers did not dismiss any TP marks, 4 observers dismissed 9 TP marks (Table 3). Together, ten observers accepted 135 FP marks. On average, radiologists accepted one per 50 chest radiographs and residents accepted one per 9 chest radiographs.
The number of malignancies not initially detected by the observers but correctly detected after review of CAD marks varied between 6 and 12 per observer. 53% (44 of 83) of these TP CAD marks were rejected by the observers. Both the radiologist and resident groups rejected 53% of TP CAD marks (23 of 43 marks, 21 of 40 marks each). Two examples of nodule detection with and without CAD, as well as the interaction between CAD and radiologists are illustrated in Figures 2 and 3.
In this retrospective study, we evaluated observer performance and its interaction with the CAD system in the detection of malignant nodules on chest radiograph using the JAFROC analysis. Our results showed that 1) the CAD system can improve diagnostic performance in the detection of nodules on chest radiograph, 2) the overall sensitivity increased from 84 to 87% with CAD marks, and 3) the FP rate also increased with CAD in the radiology resident group.
Most remarkably, although the increase in average FOM was small, it was statistically significant for all observers except observer 6 who showed unusually high sensitivity, high FP rate, and dismissed many TP nodules that CAD did not detect. Without this observer, the average FOM value increased from 0.90 to 0.92 (p = 0.04). When lowering the confidence score was not allowed, the average FOM value also increased from 0.90 to 0.91 (p = 0.04) with slightly higher sensitivity and a higher false positive rate for all observers. Our results are consistent with previous reports (8, 12) that have demonstrated an improvement of observer performance in nodule detection with CAD. However, these studies did not calculate the FP rate and the lung nodules were not pathologically confirmed.
The CAD system has been shown to have the potential to detect substantial portions of lung cancers missed by radiologists on chest radiographs (15, 19). However, no significant improvement in observer performance was able to be observed as subtle TP nodules correctly marked by CAD were frequently rejected by the observers (16). In this study, patient cases not only included screening but also diagnostic imaging; moreover, 91% of nodules were detected by more than half of the observers without the help of CAD. Thus, patient cases that may have consisted of more obvious nodules in our study may explain the higher observer sensitivity and significant improvement in observer performance compared to previous studies.
On subgroup analysis, the average FOM value of both the resident group and radiologist group increased after reviewing the CAD marks, however the difference was not statistically significant. In our study, we observed that the radiologist group benefited more with the use of the CAD system - increased sensitivity with almost no trade off in FP rate. Residents group showed a similar degree increased sensitivity with the radiologist group, but the FP rate was higher. Our study results indicate that radiologist groups showed a better ability to differentiate TP from FP CAD markings, as well as demonstrate the importance of CAD-radiologist interactions.
When using CAD, a relatively high FP rate is a given. Thus, it is essential for observers to be able to correctly differentiate TP from FP CAD markings. Detecting a subtle TP nodule among FP marks is difficult and is one of the major factors determining the observer performance level. Sufficient training and experience on CAD images will help in the discrimination of TP and FP markings. Furthermore, future CAD systems with a lower false positive rate and better sensitivity are expected to solve this problem and contribute to increased observer performance.
Our study has several limitations. First, our patient cases are considered to consist of a higher proportion of conspicuous nodules than previous studies (16) because we only included nodules visible on chest radiographs. To evaluate the value of CAD, we believe that nodules should be at least visible on chest radiographs. Second, this is a retrospective study and the performance of CAD in clinical practice should be further investigated. Furthermore, increased reading time, integration into to the PACS system, and the role as a second reader, should be considered as part of the clinical setting.
In conclusion, our data suggests that although the increase in average FOM value was small, the CAD system can improve observer performance in detecting malignant lung nodules on chest radiograph. Further studies on the effect of CAD in routine practice should be performed.
Figures and Tables
Fig. 2
72-year-old woman with 16 mm adenocarcinoma in left lower lobe. There are four computer-aided detection (CAD) marks among which only mark in left lower lung is true positive. Small calcified nodule in right upper lobe was excluded from analysis. At initial reading, three radiologists and two residents detected true lesion. After review of CAD marks, one radiologist and two residents accepted true CAD mark, while one radiologist and one resident rejected true CAD mark. One resident detected one false positive lesion and added one more false positive lesion after review of CAD marks.
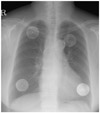
Fig. 3
61-year-old man with 11 mm adenocarcinoma in right upper lobe. There is one computer-aided detection (CAD) mark in right upper lobe which is true positive. At initial reading, two radiologists and one resident detected true lesion. After review of CAD marks, two radiologists and two residents accepted true CAD mark, while one radiologist and two residents rejected true CAD mark. Two residents detected one false positive lesion each, but rejected false positive lesion after review of CAD marks.
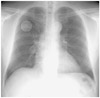
Table 1
Individual Outcome of Observer Study with and without CAD When Lowering of Confidence Score Was Allowed
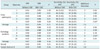
References
1. Muhm JR, Miller WE, Fontana RS, Sanderson DR, Uhlenhopp MA. Lung cancer detected during a screening program using four-month chest radiographs. Radiology. 1983. 148:609–615.
2. Austin JH, Romney BM, Goldsmith LS. Missed bronchogenic carcinoma: radiographic findings in 27 patients with a potentially resectable lesion evident in retrospect. Radiology. 1992. 182:115–122.
3. Monnier-Cholley L, Arrivé L, Porcel A, Shehata K, Dahan H, Urban T, et al. Characteristics of missed lung cancer on chest radiographs: a French experience. Eur Radiol. 2001. 11:597–605.
4. Quekel LG, Kessels AG, Goei R, van Engelshoven JM. Miss rate of lung cancer on the chest radiograph in clinical practice. Chest. 1999. 115:720–724.
5. Choi EJ, Jin GY, Han YM, Lee YS, Kweon KS. Solitary pulmonary nodule on helical dynamic CT scans: analysis of the enhancement patterns using a computer-aided diagnosis (CAD) system. Korean J Radiol. 2008. 9:401–408.
6. Song KD, Chung MJ, Kim HC, Jeong SY, Lee KS. Usefulness of the CAD system for detecting pulmonary nodule in real clinical practice. Korean J Radiol. 2011. 12:163–168.
7. Goo JM. A computer-aided diagnosis for evaluating lung nodules on chest CT: the current status and perspective. Korean J Radiol. 2011. 12:145–155.
8. Kakeda S, Moriya J, Sato H, Aoki T, Watanabe H, Nakata H, et al. Improved detection of lung nodules on chest radiographs using a commercial computer-aided diagnosis system. AJR Am J Roentgenol. 2004. 182:505–510.
9. Shiraishi J, Li F, Doi K. Computer-aided diagnosis for improved detection of lung nodules by use of posterior-anterior and lateral chest radiographs. Acad Radiol. 2007. 14:28–37.
10. Bley TA, Baumann T, Saueressig U, Pache G, Treier M, Schaefer O, et al. Comparison of radiologist and CAD performance in the detection of CT-confirmed subtle pulmonary nodules on digital chest radiographs. Invest Radiol. 2008. 43:343–348.
11. He Q, He W, Wang K, Ma D. Effect of multiscale processing in digital chest radiography on automated detection of lung nodule with a computer assistance system. J Digit Imaging. 2008. 21:Suppl 1. S164–S170.
12. Kasai S, Li F, Shiraishi J, Doi K. Usefulness of computer-aided diagnosis schemes for vertebral fractures and lung nodules on chest radiographs. AJR Am J Roentgenol. 2008. 191:260–265.
13. Li F, Engelmann R, Metz CE, Doi K, MacMahon H. Lung cancers missed on chest radiographs: results obtained with a commercial computer-aided detection program. Radiology. 2008. 246:273–280.
14. van Beek EJ, Mullan B, Thompson B. Evaluation of a real-time interactive pulmonary nodule analysis system on chest digital radiographic images: a prospective study. Acad Radiol. 2008. 15:571–575.
15. White CS, Flukinger T, Jeudy J, Chen JJ. Use of a computer-aided detection system to detect missed lung cancer at chest radiography. Radiology. 2009. 252:273–281.
16. de Hoop B, De Boo DW, Gietema HA, van Hoorn F, Mearadji B, Schijf L, et al. Computer-aided detection of lung cancer on chest radiographs: effect on observer performance. Radiology. 2010. 257:532–540.
17. Chakraborty DP. Analysis of location specific observer performance data: validated extensions of the jackknife free-response (JAFROC) method. Acad Radiol. 2006. 13:1187–1193.
18. Giger ML, Chan HP, Boone J. Anniversary paper: History and status of CAD and quantitative image analysis: the role of Medical Physics and AAPM. Med Phys. 2008. 35:5799–5820.
19. Li Q, Li F, Shiraishi J, Katsuragawa S, Sone S, Doi K. Investigation of new psychophysical measures for evaluation of similar images on thoracic computed tomography for distinction between benign and malignant nodules. Med Phys. 2003. 30:2584–2593.