Abstract
Objective
We wanted to evaluate the usefulness of the computer-aided detection (CAD) system for detecting pulmonary nodules in real clinical practice by using the CT images.
Materials and Methods
Our Institutional Review Board approved our retrospective study with a waiver of informed consent. This study included 166 CT examinations that were performed for the evaluation of pulmonary metastasis in 166 patients with colorectal cancer. All the CT examinations were interpreted by radiologists and they were also evaluated by the CAD system. All the nodules detected by the CAD system were evaluated with regard to whether or not they were true nodules, and they were classified into micronodules (MN, diameter < 4 mm) and significant nodules (SN, 4 ≤ diameter ≤ 10 mm). The radiologic reports and CAD results were compared.
Results
The CAD system helped detect 426 nodules; 115 (27%) of the 426 nodules were classified as true nodules and 35 (30%) of the 115 nodules were SNs, and 83 (72%) of the 115 were not mentioned in the radiologists' reports and three (4%) of the 83 nodules were non-calcified SNs. One of three non-calcified SNs was confirmed as a metastatic nodule. According to the radiologists' reports, 60 true nodules were detected, and 28 of the 60 were not detected by the CAD system.
The lung is the most common extra abdominal site of metastases from colorectal carcinoma. After resection of isolated pulmonary metastases, the 5-year survival rates range from 22-42% (1-4). Therefore, early detection of pulmonary metastases is very important for treating patients with colorectal cancer. However, detecting pulmonary nodules is time-consuming work and this requires the complete attention of a radiologist.
For this reason, computer aided methods have been developed for detecting pulmonary nodules, and their roles in assisting radiologists have been demonstrated (5-13). However, in most studies associated with use of the computer-aided detection (14) system for detecting lung nodules, the radiologists who participated in the studies knew the purpose of the study beforehand. These partly unblinded study designs could have cause an observer bias.
This study was performed in a cohort of patients with colorectal cancer. The incidence of pulmonary nodules, which are clinically significant, is higher in the population with colorectal cancer than in the general population. Furthermore, pulmonary metastases generally show a rapid clinical course. Thus, the existence and detection of pulmonary nodules in patients with colorectal cancer has tremendous clinical significance.
In this study, we compared the official radiologic reports and the CAD results in order to evaluate the usefulness of the CAD system for the detection of pulmonary nodules in a cohort of patients with colorectal cancer under real clinical circumstances and without an observer bias.
Our Institutional Review Board approved our retrospective study with a waiver of informed consent.
This study included 170 consecutive patients with proven colorectal cancer and who had attended our institution and who had undergone chest computed tomographic (CT) examination for the evaluation of possible pulmonary metastasis between March 2009 and May 2009. Among a total of 170 chest CT examinations, four examinations were excluded. As the sequela of previous pulmonary tuberculosis, the lungs of these four patients were destroyed, and many fibrotic bands and calcified nodules were observed. The CAD system marked more than 20 suspicious nodules; however, evaluation of these nodules detected by the CAD system was impossible. As a result, 166 patients were finally included in our study: 105 patients were men and 61 were women and the patients ranged in age from 38 to 76 years (mean age: 59.7).
CT scans were obtained using 64-section MDCT (LightSpeed VCT, GE Medical Systems, Milwaukee, WI). The acquisition parameters were 120 kVp, a pitch of 1.375, a 0.5 second gantry rotation time, a 40 mm beam width and 120-200 mAs/slice with size-based adjustment of the automatic exposure control. The axial images were reconstructed with a 1.25 mm slice thickness using a high spatial frequency reconstruction kernel.
Network transfer of the CT data from a picture archiving and communication system (Centricity 3.0, General Electric Medical Systems, Milwaukee, WI) to a CAD workstation was achieved using the DICOM (digital imaging and communications in medicine) protocol. The images were displayed and processed using a commercially available software program (Extended Brilliance Workspace v3.0, Philips Medical Systems, Cleveland, OH).
All the CT images were interpreted within 24 hours of the CT examination by one of five radiologists who specialized in thoracic radiology in routine daily practice. They each had one to fifteen years of experience in thoracic radiology. Their final official reports were recorded in the EMR (Electric Medical Records). They did not refer to the CAD system during the interpretation of the CT examinations.
All the CT examinations were retrospectively processed by the CAD system. The CAD system marked the suspicious pulmonary nodules with squares (Fig. 1).
Another two radiologists (a resident, and a thoracic radiologist with 10 years experience) who did not take part in the interpretation of the CT examinations carefully reviewed the results that were processed by the CAD system and the 166 official reports.
First, all the suspicious nodules marked by the CAD system were evaluated by the two radiologists as to whether or not they were true nodules. If there was a discrepancy between two radiologists, a final decision was made by consensus of the two radiologists. The criteria for true nodules included the shape, margin, size, density, location and the relationship with the surrounding structures. All of these factors were considered in determining whether or not the nodules marked by the CAD system were true nodules. All the nodules were classified according to size as either micronodules (MN, diameter < 4 mm) or significant nodules (SN, 4 ≤ diameter ≤ 10 mm), and they were also evaluated as to whether or not they contained calcification.
Next, the two radiologists, who reached a decision by consensus, reviewed the 166 official reports. They assessed the presence of nodules that had been remarked on in the official reports.
Finally, they correlated nodules that had been remarked on in the official reports with the nodules found by the CAD system. The nodules that were detected by the CAD system, but that were not remarked on in the official reports and the nodules that were mentioned in the official reports, but that were not marked by the CAD system were evaluated. The size, location, presence of calcification and opacity of all the nodules were also assessed. The location was categorized into the subpleural area, the fissural area, the costophrenic angle area and the lung parenchyma. Opacity was categorized into nodular ground-glass opacity and solid nodule.
The CAD system marked 426 candidate lesions for a rate of 2.57 (426 of 166) per patient. The number of nodules detected by the CAD system varied from zero to fifteen; 115 (27%) of the 426 candidate lesions were classified as true nodules and the remaining 311 (73%) were false nodules (Fig. 1). Of the 115 true nodules, 35 (30%) were classified as SNs; 52 (45%) had calcification and 18 (16%) were non-calcified SNs.
According to the 166 official radiologic reports, 60 nodules had been remarked on and all these nodules were true nodules; 33 (55%) of the 60 nodules were SNs.
Comparison of the two results showed that 83 (72%) of the 115 true nodules detected by the CAD system were missed or neglected in the radiologic reports; 28 (47%) of the 60 nodules that were remarked on in the official reports were not detected by the CAD system; 32 nodules were detected by both the CAD system and by the radiologists (Fig. 2). Therefore, a total of 143 true nodules were detected by both the CAD system and the radiologists (Table 1).
Most of the nodules detected by the CAD system, but neglected by the radiologist, were MNs (69 of 83, 83%) or calcified SNs (11 of 83, 13%); however, three (4%) nodules detected only by the CAD system were non-calcified SNs (Fig. 3). One of the three nodules was 9.5 mm in diameter, solid and located in the left hilar area. Another one was 6 mm in diameter, solid and located adjacent to the left cardiac border. The other was 4 mm in diameter, solid and located in the right lower lobe. In contrast, 11 (40%) of the 28 nodules that were detected by the radiologist, but missed by the CAD system were non-calcified SNs; eight of the 28 nodules were ground-glass opacity nodules, 20 of the 28 nodules were solid nodules and eight of these 20 solid nodules were located in lung parenchyma, seven were in subpleural areas, two were in fissural areas and three were in costophrenic angle areas. All these nodules were non-calcified.
The number of additional non-calcified SNs detected by the CAD system (n = 3) accounted for 0.7% of the candidate lesions marked by the CAD system (n = 426), and 5% of the nodules were remarked on in the official radiologic reports (n = 60). One of the three nodules demonstrated an interval increase in size on the follow-up CT imaging, and metastasis was surgically confirmed.
Several studies have evaluated the performance of CAD systems for the detection of lung nodules. They have demonstrated a role for CAD systems for not replacing radiologists, but for assisting radiologists. However, in the previous studies, the radiologists who participated in detecting lung nodules already knew the purpose of the studies, which means that the results of the previous studies could be skewed by an observer bias. The included population of our study was restricted to patients who were diagnosed as having colorectal cancer, and we evaluated the performance of the CAD system under real clinical circumstances without an observer bias. As a result, the CAD system additionally detected three non-calcified SNs, which was 5% of the 60 nodules remarked on in 166 radiologic reports. Considering that the patients were diagnosed as having colorectal cancer, non-calcified SNs surely needed to be remarked on. In fact, one of the three non-calcified SNs demonstrated an interval increase in size on the follow-up CT imaging, and then metastasis was surgically confirmed. Although the CAD system additionally detected three non-calcified SNs, it missed 28 nodules among the 60 nodules that were remarked on in the radiologic reports. The results of our study are consistent with the results of previous studies that demonstrated a role for CAD systems not to replace radiologists, but for assisting them.
The CAD system detected 426 suspicious lesions at a rate of 2.5 nodules per one CT examination. However, only 115 nodules were classified as true nodules. Therefore, the positive predictive value was 27%. If we consider only 18 non-calcified SNs to be positive, then the predictive value is decreased to 4%. The low positive predictive value is the reason that many radiologists hesitate to use the CAD system.
The CAD system recognizes opacity lesions surrounded by lung parenchymal attenuation as nodules. Considering this principle of the CAD system, we can expect that nodules in the subpleural, fissural and costophrenic angle areas might be missed. In our study, the CAD system missed 28 nodules among the 60 nodules that were remarked on in the radiologic reports. Eight of these 28 missed nodules were ground-glass opacity nodules and 20 were solid nodules. According to the size criteria, 11 nodules were SNs and all of them were solid nodules. With regard to location, 8, 7, 3 and 2 nodules of the 20 solid nodules were located in lung parenchyma, subpleural areas, fissural areas and costophrenic angle areas, respectively. Against our expectations, lung parenchymal nodules were also missed. This result showed the limitation of the algorithm of the CAD system.
In the previous studies, the nodule detection sensitivity of CAD systems has varied from 38% to 95% (14-18). These results might stem from the variety of CAD systems, the patient groups, the studies' methodologies and the characteristics of the nodules used in the studies. In our study, we did not know the number of nodules that were missed by both the CAD system and the radiologists. Therefore, we were not able to calculate the exact sensitivity. However, we were able to estimate that the number of nodules missed by both the CAD system and the radiologist was quite low. Therefore, the approximate sensitivity was 80% (115 of 143), which was similar to the sensitivity reported in the previous studies.
There are limitations in our study. First, we evaluated the performance of the radiologists by reviewing the official radiologic reports. During this process, we regarded non-calcified SNs that were not remarked on in the official reports as nodules that were missed by radiologists based on the assumption that non-calcified SNs needed to be remarked on, with giving consideration to the fact that the patients had colorectal cancer. However, characterization of lung nodules does not depend only on size and calcification. In fact, a number of factors influence a radiologist's decision. Therefore, based on their experience, radiologists might think that these nodules were benign and that these nodules did not need to be remarked on in the official reports. In this case, we could not differentiate nodules that were detected but not remarked on by radiologists from the nodules that were missed by radiologists. Second, a true nodule was not clearly defined. The definite criteria for true nodules have not been established. Therefore, whether or not nodules that were detected by the CAD system were true nodules was determined by a consensus between two radiologists, as was done in the previous studies (9, 10, 19). Third, we did not compare the performance between the radiologists and the CAD system for the detection of MNs. Forty one non-calcified MNs, which were not remarked on in the official reports, were additionally detected by the CAD system (Table 1). The number was almost double that of the non-calcified MNs, which were remarked on in the official reports. However, it has been suggested that small lesions are more likely to be benign (20) and the MNs were more likely not be remarked on in official reports even though they were detected by radiologists. In fact, none of the MNs detected by the CAD system were confirmed as metastases on the follow up CT scans. Furthermore, the CAD system itself still has a limitation for not being able to detect nodules less than 4 mm in diameter. These factors made comparing the performance the between radiologists and the CAD system for detecting MNs meaningless.
To conclude, the CAD system missed many of the significant nodules that were detected by radiologists, so it cannot yet replace the role of the radiologist and it probably never will. Yet in our study, which was performed under real clinical circumstances, the CAD system detected additional nodules that were missed by the radiologists. Thus, the CAD system can be useful to support the radiologist's performance for detecting nodules.
Figures and Tables
Fig. 1
57-year-old man who underwent transanal endoscopic microsurgery for rectal cancer four years before chest CT scan.
Candidate lesion is marked (arrow) on captured image from computer-aided detection. This was actually branch of right superior pulmonary vein.
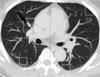
Fig. 2
55-year-old man who underwent low anterior resection for rectal cancer six months before chest CT scan.
A. On axial CT image, small non-calcified lung nodule (diameter = 5.4 mm) is noted in right minor fissure (arrow). B. On captured image from computer-aided detection, nodule is marked as C1 candidate lesion (arrow). This nodule was detected by both computer-aided detection system and radiologist. C. On axial CT image performed one year later, nodule shows no interval change of size and shape (arrow). Note newly appeared malignant pleural effusion in left hemithorax.
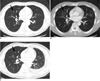
Fig. 3
61-year-old woman who underwent right hemicolectomy for colon cancer one year before chest CT scan.
A. On axial CT image, small lung nodule (diameter = 4.0 mm) is noted in right lower lobe (arrow). This non-calcified significant nodule was missed in official report. B. On captured image from computer-aided detection, same nodule is marked as C1, candidate lesion. C. On axial CT image performed four months later, nodule demonstrated interval increase in size (arrow). Metastatic adenocarcinoma was confirmed by video-assisted thoracoscopic surgery metastasectomy.
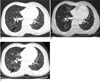
References
1. Ambiru S, Miyazaki M, Ito H, Nakagawa K, Shimizu H, Kato A, et al. Resection of hepatic and pulmonary metastases in patients with colorectal carcinoma. Cancer. 1998. 82:274–278.
2. Girard P, Ducreux M, Baldeyrou P, Rougier P, Le Chevalier T, Bougaran J, et al. Surgery for lung metastases from colorectal cancer: analysis of prognostic factors. J Clin Oncol. 1996. 14:2047–2053.
3. McAfee MK, Allen MS, Trastek VF, Ilstrup DM, Deschamps C, Pairolero PC. Colorectal lung metastases: results of surgical excision. Ann Thorac Surg. 1992. 53:780–785. discussion 785-786.
4. Yano T, Hara N, Ichinose Y, Yokoyama H, Miura T, Ohta M. Results of pulmonary resection of metastatic colorectal cancer and its application. J Thorac Cardiovasc Surg. 1993. 106:875–879.
5. Armato SG 3rd, Li F, Giger ML, MacMahon H, Sone S, Doi K. Lung cancer: performance of automated lung nodule detection applied to cancers missed in a CT screening program. Radiology. 2002. 225:685–692.
6. Awai K, Murao K, Ozawa A, Komi M, Hayakawa H, Hori S, et al. Pulmonary nodules at chest CT: effect of computer-aided diagnosis on radiologists' detection performance. Radiology. 2004. 230:347–352.
7. Doi K. Current status and future potential of computer-aided diagnosis in medical imaging. Br J Radiol. 2005. 78:S3–S19.
8. Ko JP, Naidich DP. Computer-aided diagnosis and the evaluation of lung disease. J Thorac Imaging. 2004. 19:136–155.
9. Rubin GD, Lyo JK, Paik DS, Sherbondy AJ, Chow LC, Leung AN, et al. Pulmonary nodules on multi-detector row CT scans: performance comparison of radiologists and computer-aided detection. Radiology. 2005. 234:274–283.
10. Yuan R, Vos PM, Cooperberg PL. Computer-aided detection in screening CT for pulmonary nodules. AJR Am J Roentgenol. 2006. 186:1280–1287.
11. Choi EJ, Jin GY, Han YM, Lee YS, Kweon KS. Solitary pulmonary nodule on helical dynamic CT scans: analysis of the enhancement patterns using a computer-aided diagnosis (CAD) system. Korean J Radiol. 2008. 9:401–408.
12. Lee JY, Chung MJ, Yi CA, Lee KS. Ultra-low-dose MDCT of the chest: influence on automated lung nodule detection. Korean J Radiol. 2008. 9:95–101.
13. Ozekes S, Osman O, Ucan ON. Nodule detection in a lung region that's segmented with using genetic cellular neural networks and 3D template matching with fuzzy rule based thresholding. Korean J Radiol. 2008. 9:1–9.
14. Armato SG 3rd, Giger ML, MacMahon H. Automated detection of lung nodules in CT scans: preliminary results. Med Phys. 2001. 28:1552–1561.
15. Giger ML, Bae KT, MacMahon H. Computerized detection of pulmonary nodules in computed tomography images. Invest Radiol. 1994. 29:459–465.
16. Gurcan MN, Sahiner B, Petrick N, Chan HP, Kazerooni EA, Cascade PN, et al. Lung nodule detection on thoracic computed tomography images: preliminary evaluation of a computer-aided diagnosis system. Med Phys. 2002. 29:2552–2558.
17. Ko JP, Betke M. Chest CT: automated nodule detection and assessment of change over time--preliminary experience. Radiology. 2001. 218:267–273.
18. Wormanns D, Fiebich M, Saidi M, Diederich S, Heindel W. Automatic detection of pulmonary nodules at spiral CT: clinical application of a computer-aided diagnosis system. Eur Radiol. 2002. 12:1052–1057.
19. Lee IJ, Gamsu G, Czum J, Wu N, Johnson R, Chakrapani S. Lung nodule detection on chest CT: evaluation of a computer-aided detection (CAD) system. Korean J Radiol. 2005. 6:89–93.
20. Davis SD. CT evaluation for pulmonary metastases in patients with extrathoracic malignancy. Radiology. 1991. 180:1–12.