Abstract
Figures and Tables
Fig. 1
Data flow in the chart system of the Korean Neonatal Network. The database required for DDS are selectively extracted from the central KNN database by an automatic scheduler program and are separately stored. After member verification on the member's site, certified users can find a list of "report name" corresponding to the variables of interest in the web page of "Data Statistics". Once a variable is clicked (data field), a HTML5-based chart program in the remote server requests the data statistics of the variable that meet the specific conditions defined by users. K-NIH, Korean National Institute of Health; HTML, HyperText Markup Language; KNN, Korean Neonatal Network.
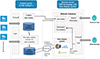
Fig. 2
A representative chart showing the rankings of a fictitious center the hospitals in terms of number of annual admissions. Logged-in users from "hospital A" can see that their hospital is ranked second. The rankings of the other KNN hospitals are concealed and are displayed in descending order as H001, H002, etc.
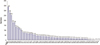
Fig. 3
Various types of charts that compare the data statistics of a fictitious hospital with those of entire centers in KNN. (A) Horizontal percentage stacked bar graphs that display the percentage of each category of respiratory support mode given at 28 days of life. (B) Vertical bar graphs that display the number of patients' registration who were born at a specific gestational week. (C) Horizontal number bar graphs that display the stacked number of annual hospitalizations of very-low-birth-weight infants. (D) Broken line plots that display the trend of mean base deficit in the blood gas analysis that is performed within the first hour of life.
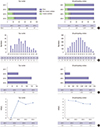
Fig. 4
Rearrangement of the plotted data by using the toggle function. (A) Five different categories of treatment policy for patent ductus arteriosus of a fictitious center are displayed as the horizontal percent bar chart with default setting. (B) Once the last two categories are inactivated by using the toggle function, a chart composed of the remaining three categories is newly displayed.
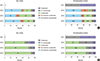
Fig. 5
Data statistics defined by specified conditions. The horizontal percent bar charts shows the patients' status at the time of hospital discharges (survival and discharge to home, survival and transfer to other hospitals, death and hospitalization >1 yr) in a fictitious hospital. This is a newly generated cohort composed of the cases that meet the following four conditions: year of birth between 2012 and -2013, gestational weeks <30, complete course of antenatal steroid administration, and the presence of maternal histological chorioamnionitis.
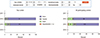
Table 1
List of variables displayed on the chart of Korean Neonatal Network
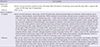
BPD, bronchopulmonary dysplasia; IVH, intraventricular hemorrhage; NEC, necrotizing enterocolitis; ROP, retinopathy of prematurity, PROM, premature rupture of membrane; PMA, postmenstrual age; PDA, patent ductus arteriosus; PVL, periventricular leukomalacia; VEGF, vascular endothelial growth factor; GMFCS, gross motor function classification system; K-DST, Korean developmental screening test.