Abstract
The pathogenesis of antiepileptic drug (AED) resistance is multifactorial. However, most candidate gene association studies typically assess the effects of candidate genes independently of each other, which is partly because of the limitations of the parametric-statistical methods for detecting the gene-to-gene interactions. A total of 200 patients with drug-resistant epilepsy and 200 patients with drug-responsive epilepsy were genotyped for 3 representative the single nucleotide polymorphisms (SNPs) of the voltage-gated sodium channel genes (SCN1A, SCN1B, and SCN2A) by polymerase chain reaction and direct sequencing analysis. Besides the typical parametric statistical method, a new statistical method (multifactor dimensionality reduction [MDR]) was used to determine whether gene-to-gene interactions increase the risk of AED resistance. None of the individual genotypes or alleles tested in the present study showed a significant association with AED resistance, regardless of their theoretical functional value. With the MDR method, of three possible 2-locus genotype combinations, the combination of SCN2A-PM with SCN1B-PM was the best model for predicting susceptibility to AED resistance, with a p value of 0.0547. MDR, as an analysis paradigm for investigating multi-locus effects in complex disorders, may be a useful statistical method for determining the role of gene-to-gene interactions in the pathogenesis of AED resistance.
Despite numerous development in antiepileptic drug (AED) therapy, epilepsy remains uncontrolled in a significant proportion of patients, even with appropriate polytherapy at maximal tolerated doses (1). The continued occurrence of seizures is a major health problem, and it increases the risk of death from epilepsy. Although the biologic basis of medically intractability, or pharmacoresistance, is poorly understood, it is likely that, just as epilepsy itself is a heterogeneous condition with multiple etiologies, the pathogenesis of "refractoriness" is multifactorial and may include both genetic and environmental factors (2, 3).
Ion channel impairment has recently emerged as a potential factor for AED resistance. Since neuronal excitability is critically governed by the interaction of voltage- and ligandgated ion channels (4), it is not surprising that ion channel alterations may be pathogenic causes of AED resistance. The clues come from different sources: 1) most currently available AEDs exert strong effects on ionic currents (5); 2) in animal epilepsy models, the density, distribution, molecular structure, and function of ion channels are altered after seizure (6); and 3) some specific epilepsy syndromes have been shown to be associated with distinct ion channel mutations (7). These clues indicate that the voltage-gated sodium channel is crucially involved in AED resistance.
Gene-to-gene interaction has been thought to be a primary mechanism underlying the pathogenesis of many multifactorial disorders or conditions (8-10). In spite of this, most candidate gene association studies typically assess the effects of candidate genes independently of each other. This is partially due to the limitations of parametric-statistical methods for detecting gene effects that are solely or partially dependent on interactions with other genes (11). For example, logistic regression is less practical for dealing with high-dimensional data, because when high-order gene-to-gene interactions are modeled, there are many contingency-table cells that contain no observations, which can lead to very large coefficient estimates and standard errors. One solution to this problem is to collect very large numbers of samples to allow for robust estimation of interaction effects; however, the magnitudes of the samples that are often required incur prohibited expense. To address this issue, a new statistical and computational method, the multifactor-dimensionality reduction (MDR) method, has been developed for detecting and characterizing high-order gene-to-gene interactions in case-control studies with relatively small samples (10).
In the present study, single nucleotide polymorphisms (SNPs) of three voltage-gated sodium channel genes and their estimated allele frequencies were identified using a DNA pool. The independent effects of a representative SNP from each gene on AED resistance were assessed, and the MDR method was used to detect possible gene-to-gene interactions among the voltage-gated sodium channel genes in the pathogenesis of pharmacoresistance to AEDs.
Consecutive patients with an established clinical diagnosis of epilepsy were recruited from the epilepsy clinics at 2 third-referral hospitals. All study participants were eligible if they had either drug-resistant (DR group, n=200) or drug-responsive epilepsy (DS group, n=200) according to the following definitions and criteria. Drug resistance was defined as the occurrence of at least four unprovoked seizures over the year before recruitment with trials of more than two appropriate AEDs at maximal tolerated doses, which were established on the basis of the occurrence of clinical side effects at supramaximal doses. Patients who had undergone surgical treatment for drug-resistant epilepsy were classified as having drug-resistant epilepsy, regardless of the surgical outcome. However, patients who were frequently in poor compliance with AED therapy and those who had reported seizures with a questionable semiology were excluded from the present study. In patients treated with single AED, drug responsiveness was defined as complete freedom from seizures for at least one year, up to the date of the last follow-up visit, Demographic and clinical characteristics by case (drug refractory)-control (drug responsive) status are described in Table 1. Each AED listed in Table 1 has been known that the mechanism of action is closely associated with sodium-channel blocking (12). The study was approved by the Institutional Review Board of the hospital, and informed consent was obtained from all study participants.
Genomic DNA was extracted from peripheral blood lymphocytes using a standard protocol. For the time and cost-effective screening of SNPs with a relatively high minor allele frequency (MAF) in the candidate sodium channel-related genes (SCN1A, SCN1B, and SCN2A), a pool of DNA was established from each of healthy control, DR or DS groups using the protocol recommended by the manufacturer (PicoGreen dsDNA Quantitation Reagent, Molecular Probes, OR, U.S.A.). In brief, double-stranded DNAs were stained with an ultrasensitive fluorescent dye (PicoGreen dsDNA Quantitation Reagent, Molecular Probes) and the total amount of fluorescent-stained DNA in each individual was measured by a fluorometer (Picofluor™, Handheld Fluorometer, Molecular Probes). An equal amount of DNA from 200 subjects per group was mixed into a pool.
About 1.5 kbp of the 5'-directed region as well as all coding regions, including exon-intron boundary sequences of each candidate gene (SCN1A, SCN1B or SCN2A), were amplified by polymerase chain reaction (PCR). A total of 87 appropriate forward and reverse primer sets for each gene (37 for SCN1A, 11 for SCN1B, and 39 for SCN2A; data not shown in detail) were prepared based on GenBank sequences (accession number: NM_006920 for SCN1A, NM_001037 for SCN1B and NM_006920 for SCN2A). PCR assays were carried out using 1.25 U of AmpliTaq Polymerase Gold (Applied Biosystems, CA, U.S.A.), 100 ng of pooled DNA, 2.0-2.5 mM of MgCl2, and 10 µM of primer. The amplification conditions were as follows: an initial denaturation cycle at 95℃ for 5 min, followed by 35 amplification cycles (denaturation at 95℃ for 30 sec, annealing at 58℃ for SCN1A-PM, 62℃ for SCN1B-PM, and 57℃ for SCN2A-PM for 30 sec, and extension at 72℃ for 1 min), and a final extension at 72℃ for 7 min. The PCR products were electrophoresed in a 1.2% agarose gel, and the amplified genomic DNA fragments were extracted from the gel and purified using a QIAquick® gel extraction kit (Quiagen, Hilden, Germany) according to the manufacturer's instructions. Direct sequencing of both strands was performed using BigDye terminator kits (PE Biosystems, CA, U.S.A.) and each electropherogram was analyzed visually using Chromas 2.13 (Technelysium Pty Ltd, Queensland, Australia).
The relative allele frequencies for all SNPs determined in this study were estimated using the comparative method proposed by Kwok et al. (Fig. 1). In order to identify individual heterozygotes for the representative SNPs, 10 random individual DNAs consisting of the pooled DNA were genotyped using exact same PCR conditions as those used for the pooled DNA.
Based on the estimated allele frequencies of the SNPs and their theoretical functional value, one SNP per gene was selected as a representative marker for the case-control study in which an association of each marker with AED resistance would be elucidated. The representative markers were as follows: SCN1A-PM located in exon 16 of SCN1A, SCN1B-PM located in exon 3 of a splice variant of SCN1B and SCN2A-PM located in intervening intronic sequences between exon 7 and 8 of SCN2A. Both SCN1A-PM and SCN1B-PM were selected as they are nonsynonymous mutations that may have functional value. Because there was no detectable SNP in the exonic sequences of SCN2A in this study, an intronic SNP (SCN2A-PM) showing the maximum difference of estimated MAF between DR and DS groups was selected as a representative marker for SCN2A. However, the substantial value of each SNP still remains to be clear.
A total of 200 patients with drug-resistant epilepsy (DR group) and 200 patients with drug-responsive epilepsy (DS group) were genotyped for 3 representative SNPs by PCR and direct sequencing analysis. The distribution of each genotype of the SNPs between the DR and DS groups were compared statistically by chi-square analysis with Fisher's exact test. The relative risk of AED resistance in patients with the mutant allele was estimated by comparison with the wild-type allele by logistic regression analysis. Data were analyzed with SPSS (release 14 OK, Chicago, IL, U.S.A.) and differences were considered significant for p values of ≤0.05. Conformance with the Hardy-Weinberg equilibrium was tested by comparing the observed and expected genotype frequencies of the controls using the chi-square test.
A new statistical method, MDR, was introduced to identify whether gene-to-gene interactions among SCN1A, SCN1B, and SCN2A increase the risk of AED resistance.
Briefly, most parametric-statistical methods, such as logistic regression analysis, are less practical for dealing with high dimensional data. However, with MDR, multilocus genotypes are pooled into high-risk and low-risk groups, effectively reducing the genotype predictors from n dimensions to one dimension. The new, one-dimensional multilocus-genotype variable was evaluated for its ability to classify and predict disease status through cross-validation and permutation testing (Fig. 2). The null hypothesis of no association was rejected when the p value derived from the permutation test was ≤0.05.
A total of 18 biallelic SNPs in 3 sodium channel-related genes were identified using a pooled DNA from 200 control subjects: 10 from SCN1A, 2 from SCN1B and 6 from SCN2A (data not shown in detail). The SNPs found in exon 16 of SCN1A and in exon 3 in a splice variant of SCN1B were nonsynonymous mutations that resulted in amino acid changes from alanine to threonine and leucine to proline, respectively.
The MAF of each tested SNP estimated in the pool of DNA from 200 control subjects using a comparative method was compared with that observed in the individual genotyping of the pool in Table 2. A biallelic SNP with an MAF of about 0.01 (SCN1A-PM) could be identified with an observational error of 0.005. The maximum amount of observational error was 0.019, which is consistent with the result of a previous study (13).
None of the individual genotypes tested in the present study showed a significant association with AED resistance, regardless of their theoretical functional value (Table 3). The risk for susceptibility to AED resistance in patients with the mutant allele in each SNP was not significant when compared with that of the wild-type allele (Table 4).
In the absence of any statistically significant independent primary effect, the association of multi-locus genotype combinations with AED resistance was analyzed by MDR (Table 5). Of the three possible 2-locus genotype combinations, the combination of SCN2A-PM with SCN1B-PM was the best model for predicting susceptibility to AED resistance with a p value of 0.0547 (Fig. 3). In the model, the cross-validation consistency was 10/10, meaning that this model was selected as the best model in all 10 cross-validation tests. The three-locus genotype combination did not reach statistical significance in predicting susceptibility to AED resistance (p=0.1719). Both SCN2A-PM and SCN1B-PM were synergistic in predicting susceptibility to AED resistance. However, every combination of the interaction that included SCN1A-PM provided redundant information (Fig. 4).
Mechanisms of AED resistance are related to drug metabolism, drug transporters, drug targets, and ion channels (14-16). Over the past decade, much attention has been focused on both cytochrome P450 enzymes, which have the potential to affect AED metabolism, and membrane efflux transporters, such as ATP-binding cassette subfamily B member 1 (ABCB1) (15, 16). One of the most significant advances in our understanding of the role of genetic factors in the pathogenesis of AED resistance came from Siddiqui et al., who reported an association between multidrug resistance in epilepsy and a polymorphism in the drug transporter gene ABCB1, which appears to confer a susceptibility to AED refractoriness (17). However, recently published studies provide apparently conflicting evidence on the association between the ABCB1 gene and AED resistance (16, 18).
Voltage-gated sodium channels are essential for the initiation and propagation of action potentials in neurons, which is the basic requirement for the neuronal excitability underlying epileptogenesis, and they are the targets of most of the currently available AEDs (16). In addition, with the remarkable advances in molecular genetics, it has been known that at least 3 voltage-gated sodium channel genes (SCN1A, SCN1B or SCN2A gene) are directly associated with a number of epilepsy syndromes (19, 20). In this context, impairments in the functions of voltage-gated sodium channels may be potential mechanisms of the pathogenesis underlying the general resistance to AED therapy. However, to our knowledge, there has been no report on this topic thus far.
In the present study, representatives SNPs from the SCN1A, SCN1B or SCN2A genes were analyzed to determine whether each SNP was independently with AED resistance.
Even though two of the SNPs tested were nonsynonymous mutations that resulted in amino acid changes, no significant association was found between each SNP and AED resistance (Table 3, 4). However, it is not clear whether these results truly mean that the voltage-dependent sodium channel genes do not contribute to the pathogenesis of AED resistance under any circumstance. An alternative explanation for the results is either that the independent contribution of each gene to AED resistance may have been too weak to be detected with number of samples or that the SNP selected from each gene may not have been a real representative of the gene in terms of its function. As a first step to answering these questions, the MDR method was introduced in the present study to determine whether one of the weak contributions was implicated in AED resistance. It is our working hypothesis that for some genes these gene-to-gene interactions may be more important than the independent effects of the single genes.
With the MDR method used in the present study (Table 5), the best single locus model selected was a SCN2A-PM marker with a p value of 0.8281, suggesting that the power of the marker in predicting susceptibility to AED resistance is very weak. This result is consistent with that of the parametric statistical analysis. The best multi-locus MDR model selected SCN2A-PM and SCN1B-PM with a p value of 0.0547 in two-gene interaction settings. Although, as in the single locus MDR models, the interaction between SCN2A-PM and SCN1B-PM did not agree with the working hypothesis at a level of statistical significance in this study, it is clear that gene-to-gene interaction is more important than any independent effect of single genes in predicting susceptibility to AED resistance. The three-gene-interaction model did not increase the power of predicting AED resistance when compared with the two-gene-interaction model. It also contributes to the increasing amount of evidence that gene-to-gene interactions are potential causes of common multifactorial disorders, such as AED resistance, by demonstrating that some genes are synergistic in their ability to produce refractoriness to AED therapy while others are redundant. In our cases, SCN2A-PM and SCN1B-PM were synergistic in increasing the predictive power, while SCN1A-PM acted as a redundant factor.
It has been known that pooling an equal amount of DNA from individual samples and measuring the relative abundance of alleles in the pool is an efficient strategy for estimating allele frequencies in many samples because it drastically reduces the cost of the analysis and the amount of DNA consumed when compared to genotyping individuals and counting alleles (13, 21-23). Obviously, the method used to genotype SNPs in pooled DNA must provide an accurate estimation of allele frequencies and must be time and cost effective. In this study, the method proposed by Kwok et al. (13) was used to genotype SNPs and estimate their allele frequencies in a pool of DNA. The accuracy of the method in estimating allele frequency was verified, along with its time and cost effectiveness (Table 2). This provides proof that the selection of the representative SNPs based on the results from the analysis using pooled DNA was appropriate for association studies.
A limitation of the present study was that the number and characteristics of the variables (SNPs tested) were not sufficient to support an intrinsic value of the MDR method in dissecting the gene-to-gene interactions that underlie AED resistance. In reality, there is no evidence that AED resistance is determined by the neuronal excitability associated with sodium channel genes alone (14). Another limitation of this study is that the SNPs tested in this study were selected by their theoretical functional value and not by their intrinsic value. This means that, in certain circumstances, a SNP with a stronger effect than the SNP used can be obtained in the corresponding gene. Therefore, further studies that include various genes with various mechanisms underlying AED resistance and that cover all SNPs developed in a gene are needed.
Taken together, the results of this study show that the recent controversies in the previous studies concerning AED resistance (16, 18) might have been partly caused by the investigators' blind belief in a single gene effect on the complex phenotype as well as ethnic differences in the distribution of the SNPs tested. MDR, as an analysis paradigm for investigating multi-locus effects in complex disorders, may be a useful statistical method for determining the role of gene-to-gene interactions in the pathogenesis of AED resistance. To our knowledge, this is the first report of a possibility that gene-to-gene interactions associated with AED resistance may be more important than the independent effects of the single genes.
Figures and Tables
Fig. 1
A comparative analysis for estimating relative allele frequencies in a pool of DNA. Allele frequency in pooled DNA={[Reference Peak Height (Individual)/Reference Peak Height (Pool)]/[Heterozygote Peak Height (Individual)/Heterozygote Peak Height (Pool)]}×0.5. Black arrows indicate heterozygote peaks and red arrows indicate reference peaks.
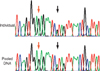
Fig. 2
The four general steps involved in using the MDR method for case-control studies (adapted from Ritchie et al., 2001). In step 1, a set of n genetic factors is selected from the pool of all factors. In step 2, the n factors and their possible multifactor classes or cells are represented in n dimensional space. For example, for three loci with three genotypes each, there are 27 three-locus-genotype combinations. The ratio of the number of cases is then estimated within each multifactor class. In step 3, each multifactor cell in n-dimensional space is labeled either as "high-risk," if the cases:control ratio meets or exceeds the given threshold (e.g., ≥1.0), or as "low-risk," if that threshold is not exceeded. This reduces the n-dimensional model to a one-dimensional model. Finally, in step 4, the prediction error of each model is estimated by 10-fold cross-validation. Here, the data (i.e., subjects) are randomly divided into 10 equal parts. Each possible 9/10 of the subjects is used to make predictions regarding the disease status of each possible 1/10 of the subjects excluded. To reduce the possibility of poor prediction error estimates due to the chance division of the data set, the 10-fold cross-validation is repeated 10 times, and the prediction errors are averaged.
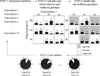
Fig. 3
Best multi-locus model for susceptibility to AED resistance. High-risk genotypes as revealed by MDR are in dark shading and the low-risk genotypes are in light shading. The numbers of individuals with refractory epilepsy are represented within each cell as the left-hand bar of the histogram and the number of individuals with responsive epilepsy are in the right-hand bar.
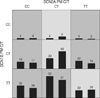
Fig. 4
The dendrogram demonstrates the nature of the interactions between SNPs. The colors used in the dendrogram comprise a spectrum of colors representing a continuum from synergy to redundancy.
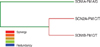
References
2. Kwan P, Brodie MJ. Refractory epilepsy: a progressive, intractable but preventable condition? Seizure. 2002. 11:77–84.


3. Regesta G, Tanganelli P. Clinical aspects and biological bases of drug-resistant epilepsies. Epilepsy Res. 1999. 34:109–122.


4. Traub RD, Borck C, Colling SB, Jefferys JG. On the structure of ictal events in vitro. Epilepsia. 1996. 37:879–891.


5. Armijo JA, Shushtarian M, Valdizan EM, Cuadrado A, de las Cuevas I, Adin J. Ion channels and epilepsy. Curr Pharm Des. 2005. 11:1975–2003.


6. Meldrum BS, Rogawski MA. Molecular targets for antiepileptic drug development. Neurotherapeutics. 2007. 4:18–61.


7. Hirose S, Mitsudome A, Okada M, Kaneko S. Epilepsy Genetic Study Group, Japan. Genetics of idiopathic epilepsies. Epilepsia. 2005. 46:Suppl 1. 38–43.
8. Gibson G. Epistasis and pleiotropy as natural properties of transcriptional regulation. Theor Popul Biol. 1996. 49:58–89.


9. Williams SM, Ritchie MD, Phillips JA 3rd, Dawson E, Prince M, Dzhura E, Willis A, Semenya A, Summar M, White BC, Addy JH, Kpodonu J, Wong LJ, Felder RA, Jose PA, Moore JH. Multilocus analysis of hypertension: a hierarchical approach. Hum Hered. 2004. 57:28–38.


10. Ritchie MD, Hahn LW, Roodi N, Bailey LR, Dupont WD, Parl FF, Moore JH. Multifactor-dimensionality reduction reveals high-order interactions among estrogen-metabolism genes in sporadic breast cancer. Am J Hum Genet. 2001. 69:138–147.


11. Templeton AR. Wade M, Brodie B, Wolf J, editors. Epistasis and complex trait. Epistasis and evolutionary process. Oxford: Oxford University Press;213–231.
12. McNamara JO. Hardman JG, Limbird LE, Gilman AG, editors. Drugs effective in the therapy of the epilepsies. Goodmann & Gilman's the pharmacological basis of therapeutics. 2001. 10th edition. New York: McGraw-Hill;521–547.
13. Kwok PY, Carlson C, Yager TD, Ankener W, Nickerson DA. Comparative analysis of human DNA variations by fluorescence-based sequencing of PCR products. Genomics. 1994. 23:138–144.


14. Evans WE, McLeod HL. Pharmacogenomics-drug disposition, drug targets, and side effects. N Engl J Med. 2003. 348:538–549.
15. Mann MW, Pons G. Various pharmacogenetic aspects of antiepileptic drug therapy: a review. CNS Drugs. 2007. 21:143–164.
16. Depondt C. The potential of pharmacogenetics in the treatment of epilepsy. Eur J Paediatr Neurol. 2006. 10:57–65.


17. Siddiqui A, Kerb R, Weale ME, Brinkmann U, Smith A, Goldstein DB, Wood NW, Sisodiya SM. Association of multidrug resistance in epilepsy with a polymorphism in the drug-transporter gene ABCB1. N Engl J Med. 2003. 348:1442–1448.
18. Kim YO, Kim MK, Woo YJ, Lee MC, Kim JH, Park KW, Kim EY, Roh YI, Kim CJ. Single nucleotide polymorphisms in the multidrug resistance 1 gene in Korean epileptics. Seizure. 2006. 15:67–72.


19. Meisler MH, Kearney JA. Sodium channel mutations in epilepsy and other neurological disorders. J Clin Invest. 2005. 115:2010–2017.


20. Kullmann DM, Hanna MG. Neurological disorders caused by inherited ion-channel mutations. Lancet Neurol. 2002. 1:157–166.


21. Bang-Ce Y, Peng Z, Bincheng Y, Songyang L. Estimation of relative allele frequencies of single-nucleotide polymorphisms in different populations by microarray hybridization of pooled DNA. Anal Biochem. 2004. 333:72–78.

