Abstract
To explore the following hypotheses: 1) Gas exchange, Organ failure, Cause, Associated disease (GOCA) score, which reflects both general health and the severity of lung injury, would be a better mortality predictor of acute respiratory distress syndrome (ARDS) than acute physiology and chronic health evaluation (APACHE II) or simplified acute physiology score (SAPS II), which are not specific to lung injury, and lung injury score (LIS) that focuses on the lung injury; 2) the performance of APACHE II and SAPS II will be improved when reinforced by LIS, we retrospectively analyzed ARDS patients (N=158) admitted to a medical intensive care unit for five years. The overall mortality of the ARDS patients was 53.2%. Calibrations for all models were good. The area under the curve of (AUC) of LIS (0.622) was significantly less than those of APACHE II (0.743) and SAPS II (0.753). The AUC of GOCA (0.703) was not better than those of APACHE II and SAPS II. The AUCs of APACHE II and SAPS II tended to further increase when reinforced by LIS. In conclusion, GOCA was not superior to APACHE II or SAPS II. The performance of the APACHE II or SAPS II tended to improve when combining a general scoring system with a scoring system that focused on the severity of lung injury.
Acute respiratory distress syndrome (ARDS) is a significant cause of admission and death in intensive care units (ICUs), despite recent development in ventilatory strategies and supportive critical care (1). Reported ARDS mortality rates vary significantly (2-10); this variability is thought to be partly due to the heterogeneity of disease severity as well as the vague definition of ARDS (4) and variable therapeutic strategies.
To compare mortality rates between different institutions, the effects of newer treatment methods, and to identify high mortality groups, a scoring system that can objectively measure the severity-of-illness of ARDS patients would be helpful. Over the past 20 yr, there have been numerous efforts to develop models that provide severity-of-illness measures to objectively describe ICU populations (11, 12), and ICU risk-prediction models were constructed for heterogeneous patients in ICUs. Severity scoring systems that can be applied to patients with ARDS can be divided into two types. One type aims to estimate general health condition of critically ill patients, and the other focuses on the severity of lung injury.
Among the severity scoring systems that have been proposed for critically ill patients, the acute physiology and chronic health evaluation (APACHE) and the simplified acute physiology score (SAPS) systems are frequently used. The performances of these systems in ICUs have been reported as satisfactory (3, 7, 13).
APACHE II and SAPS II were originally developed for predicting the prognosis of critically ill patients. Both of these scoring systems focus on evaluating the general health of the patients. By contrast, the lung injury score (LIS) (14)and the Gas exchange, Organ failure, Cause, Associated disease (GOCA) score (15) were developed specifically for patients with ARDS to define or stratify the disease (but not to predict prognosis). LIS mainly focuses on the severity of lung injury
The scoring systems for general critically ill patients may not accurately predict the prognosis in highly specialized patients such as ARDS (16, 17). Furthermore, the scoring systems that focus on mainly lung condition also can not predict the prognosis of ARDS because the degree of hypoxia was not correlated with the mortality of those patients (6-8). Theoretically, GOCA can be superior to APACHE II or SAPS II for predicting the prognosis of patients with ARDS because it considers the severity of lung injury as well as general health condition. The mortality predictive performance of the GOCA system for ARDS patients has not been adequately addressed, and there are few reports that have evaluated the overall performances of LIS, APACHE II, and SAPS II on ARDS patients.
We formed two hypotheses in this study. One is that neither the severity scoring systems that reflect general condition nor those reflect severity of lung injury can accurately predict prognosis of patients with ARDS when used alone. Therefore, GOCA, which reflects general health condition in addition to the severity of lung injury, can be a better mortality predictor than APACHE II, SAPS II and LIS. The other is that the performance of APACHE II and SAPS II will be improved when they are reinforced by a scoring system that focuses on the severity of lung injury (e.g., LIS). To address these hypotheses, we compared the performances of the above severity scoring systems - separately and combined for predicting mortality of patients with ARDS.
The study was performed in a 28-bed medical intensive care unit (MICU) at the Asan Medical Center, a university-affiliated tertiary referral center in Seoul, Korea. Data from 158 patients with ARDS admitted to the MICU from 1 January 1997 to 31 December 2001 were retrospectively evaluated.
To identify eligible patients, we reviewed the medical records of 1,787 patients treated with mechanical ventilation during the study period. The diagnosis of ARDS was made by a physician and a radiologist according to the American- European Consensus Conference criteria (4). Patients under 17 yr old and those who had a history of chronic obstructive pulmonary disease, interstitial lung disease, lung cancer or tuberculous destroyed lung were excluded from the analysis. There were 369 patients who showed PaO2/FiO2 ratios less than 200 mmHg without any exclusion criteria. Of these, 158 patients showed bilateral chest radiograph infiltrates compatible with ARDS and no evidence of left atrial hypertension (4) as determined by the Swan-Ganz catheter method or echocardiography. Survival status was evaluated at hospital discharge.
APACHE II (11), SAPS II (12), GOCA (15), and LIS (14)were calculated for all ARDS patients. Two models were made for each scoring system via logistic regression. One model used severity scores only (model 1), while the other model included patient location before MICU admission in addition to severity scores (model 2). We also evaluated the performance of combined scoring systems-APACHE II and LIS, SAPS II, and LIS.
The mean LIS was 2.80±0.47. LIS was higher than 2.5 in most of the patients (82%). We treated LIS as a continuous variable.
To compare categorical data, chi-square and Fisher's exact tests were used. Student t-tests were used for continuous data. The ability to provide risk estimations corresponding to actual mortality rates (calibration) and the ability to correctly classify survivors and non-survivors according to the estimated probability of death (discrimination) were measured for each scoring system.
Calibrations of each model were compared by Hosmer-Lemeshow's goodness-of-fit test. Hosmer-Lemeshow's C and H chi-square tests, representing two different strategies for classification of estimated probabilities of death, were used to assess the goodness of fit for each model. For the evaluation of calibration, model 2 was used. The areas under the curves of the receiver operating characteristics (AUCs) and 2×2 classification tables at decision criteria of 50% and 55%were used for the evaluation of discrimination of the scoring systems.
The difference of severity scores according to patient location before MICU admission was determined by one-way ANOVA. All statistical tests were two-sided, and we set a significance level of p<0.05.
Demographics, initial physiologic and laboratory data, and severity scores of patients are shown in Table 1. Also presented are the results from statistical comparisons between survivors and non-survivors. Eighty-four (53.2%) patients died in hospital and 74 patients survived. Five patients died on the medical floor after discharge from the ICU. The ICU mortality was 50%.
Thirty-five patients had ARDS associated with extrapulmonary diseases and 123 patients had pulmonary causes. Pulmonary causes of ARDS were pneumonia (n=84), aspiration (n=22), near drowning (n=5), miliary tuberculosis (n=6), acute interstitial pneumonitis (n=5), and inhalation injury (n=1). Extrapulmmonary causes were sepsis (n=23), trauma (n=5), pancreatitis (n=2), massive transfusion (n=1), and drug and others (n=4). The most common predisposing factors for ARDS were pneumonia and nonpulmonary sepsis.
There was no difference in mortality between pulmonary and extrapulmonary ARDS (p=0.183). There was no difference in mortality according to the year of admission (p=0.288), either. We used pressure-controlled ventilation, low tidal volume, prone position, and inhalation of nitric oxide for ARDS. There were no changes in treatment strategies for ARDS in our institution during the study period.
Non-survivors were older than survivors and also showed significantly higher positive end expiratory pressure (PEEP), lower pH, lower bicarbonate, lower hematocrit, higher blood urea nitrogen, higher glucose, lower cholesterol, higher bilirubin, and lower potassium levels than survivors. The means of all severity scores were significantly higher in non-survivors than survivors. However, gender and PaO2/FiO2 ratios were not significantly different between the two groups (Table 1).
Mortality rates were significantly different according to the patient location before MICU admission (p<0.001). Patients who had been transferred from other hospitals showed better survival than those transferred from the medical floor, emergency room, or other ICUs, with patients who had been transferred from the medical floor showing the worst prognosis. Except for LIS, all severity scores showed significant differences according to the patient location before MICU admission (SAPS II, p=0.011; GOCA, p<0.001; APACHE II, p=0.010; LIS, p=0.890). In post-hoc comparisons, SAPS II values for patients from the medical floor were higher than those from other hospitals; GOCA scores for patients from the medical floor were higher than those from other hospitals, other ICUs, and the emergency room; and APACHE II scores of patients from the medical floor were higher than those from other ICUs.
ROC curves for the models are shown in Fig. 2. The AUCs of model 1 were 0.743 for APACHE II, 0.753 for SAPS II, 0.703 for GOCA, and 0.622 for LIS (Table 3). The discrimination power of LIS was significantly inferior to APACHE II and SAPS II. When the model included age, sex, patient location before MICU admission, and cholesterol in addition to test scores, only patient location before MICU admission increased the AUC. The AUCs of APACHE II and SAPS II tended to further increase when the mortality prediction models were reinforced by LIS, with values of 0.800 and 0.810, respectively (Table 3).
Table 4 shows the 2×2 classification matrices at decision criteria of 50% and 55%. The decision criteria were chosen arbitrarily because actual mortality was 53.2%. Overall correct classifications of scoring systems were approximately 70% and were similar among systems.
To our knowledge, this is the first report that evaluates the performance of GOCA for patients with ARDS. Although this study failed to reveal the superiority of GOCA to other severity scoring systems, it suggested that a scoring system that combined general health condition and lung condition can well predict the mortality of patients with ARDS.
The general severity scoring systems, such as APACHE II and SAPS II, showed better performance in predicting mortality of patients with ARDS than LIS, which focuses only on the severity of respiratory failure. APACHE II and SAPS II scores have been previously reported to be independent predictors of hospital mortality in patients with ARDS (3, 7, 13, 18). However, these studies evaluated the significance of severity scores using only Student t-tests or logistic regression. Although these tests have shown excellent performances on overall critically ill patients, there are only a few reports that have validated the overall performance of these severity scoring systems in ARDS patients (12, 19).
The discriminative powers of APACHE II and SAPS II were considered excellent in previous reports addressing overall critically ill patients (12, 19, 20). The discriminative powers of these scores were inferior in this study compared to previous studies, possibly because these scoring systems were originally developed for overall critically ill patients, not specifically for patients with ARDS.
We found that LIS was inferior to APACHE II and SAPS II in predicting ARDS mortality. LIS is obtained by dividing the aggregate sum by the number of components that are used. There are four components; the extent of alveolar consolidation (0-4), the degree of hypoxia (0-4), the degree of respiratory system compliance (0-4), and the degree of PEEP (0-4). The PaO2/FiO2 ratio was not different between survivors and non-survivors, and this lack of relationship between the degree of hypoxia and mortality might have contributed to the shortage of discriminative power of LIS. The relationship between hypoxia and mortality of ARDS patients remains controversial, as has been reported in previous studies (6-8).
Previous studies have also reported that LIS was not suitable for predicting ARDS patient outcome (6-8) and the discriminative power of LIS for overall survival was inferior to SAPS or APACHE II scores (6, 21). There are two possible explanations for the inferiority of the LIS. First, the development of many strategies that support respiratory ability have led to general health condition, rather than lung function, being the major prognostic factor even in patients with ARDS. Second, LIS was originally developed to define ARDS, not to predict prognosis (14).
The GOCA stratification system was proposed by the American-European Consensus Conference on ARDS to assess the severity of acute lung injury and the associated clinical features (15). GOCA considers not only the severity of lung injury but also a few general conditions such as the number of organ failure and associated diseases. The GOCA score is the sum of four variables; the severity of gas exchange (0-3), the number of organ failure (0-3), the cause of lung injury (0: lung only, 1: direct lung injury, and 2: indirect lung injury) and associated diseases (0: no associated disease that will cause death within 5 yr, 1: coexisting diseases that will cause death within 5 yr but not within 6 months, and 2: coexisting diseases that will cause death within 6 months).
Although the GOCA score is not designed to predict outcome, we have hypothesized that GOCA can be better than the other scoring systems in predicting prognosis of ARDS patients because it considers the general health condition of patients in addition to the severity of lung injury. Our results did not, however, show any predictive advantage of the GOCA system. This may be because the scoring for associated diseases in GOCA is dependent on the subjective judgment of the attending physician and the differentiation of primary disease is too crude. On the other hand, GOCA may prove to be more convenient to use than APACHE II or SAPS II because it requires fewer variables but provides the same predictive power.
Monchi et al. proposed an ARDS severity scoring system showing excellent discrimination and calibration that included variables representing general patient condition and oxygenation (3). Reiter et al. also reported that risk adjustments were improved in critically ill trauma patients by combining two different scoring systems (22). In our patients, AUCs tended to increase when APACHE II and SAPS II were reinforced by LIS. The significant difference of the PEEP level between non-survivors and survivors can contribute to the AUC increase in the combined model although compliance (31.1±14.6 vs. 33.5±14.3, p=0.353) and PaO2/FiO2 ratio were not different from survivors and non-survivors. The improved discrimination implies that excellent mortality prediction models for ARDS can be developed by considering both general health condition and severity of lung injury if the variables that evaluate general health are more refined than those of GOCA.
The overall hospital mortality in this study was 53.2%, which was similar to the rates reported in several other studies (6, 8, 9, 18). Hospital mortality in this study was highly affected by patient location before MICU admission. The prognosis of patients who transferred from other hospitals was better than in the other three groups, while patients transferred from the medical floor showed the worst prognosis. Differences in mortality among these groups might be due to differences in the severity of illness. Patients who could be transferred from other hospitals probably represented less severe cases than those from the medical floor. Many patients from the medical floor were transferred after cardiopulmonary resuscitation and, therefore, must have been severely ill.
Because the severity scores of patients from the medical floor were significantly higher than those of the other groups, the use of the patient location before MICU admission as an independent variable might be unreasonable. However, multivariate logistic regression analysis for GOCA, APACHE II, SAPS II, LIS, and prior location before MICU showed that the patient location before MICU admission was an important independent mortality predictor (p=0.014). Furthermore, model 1, which did not include the patient location before MICU admission showed a similar result as in model 2; the discriminative power of LIS was inferior to those of APACHE II and SAPS II and that of GOCA was similar to those of APACHE II and SAPS II.
The calibration of a model refers to how well predicted outcomes compare to observed outcomes over the full range of risk levels (23). In general, Hosmer-Lemeshow statistics lower than 15 and p values of 0.2 to 0.8 are considered good (24). With this guideline, the severity scoring systems in this study calibrated well in ARDS patients.
There were a few limitations in this study. The first is that only patients admitted to the MICU were included, and therefore, the conclusion might have been different when patients in surgical ICUs were included. Secondly, the number of patients was relatively small compared to studies on overall critically ill patients. Because of this relatively small sample size, we could not divide our patients into developmental and validation data sets. Nevertheless, our series was still larger than several other ARDS-specific studies (6-8).
In conclusion, GOCA failed to show the superiority to APACHE II or SAPS II. The performance of APACHE II or SAPS II tended to improve when combining a general scoring system with a scoring system that focused on the severity of lung injury. Therefore, a new severity scoring system should consider all of these variables to possess excellent mortality predictive performance for ARDS patients.
Figures and Tables
Fig. 1
Calibration curves for APAHCE II, SAPS II, GOCA, and LIS. Unmarked lines represent the line of perfect correspondence between actual and predicted risk of death; marked lines represent the calibration curves. The models used for calibration curves were made using patient location before MICU admission in addition to severity scores.
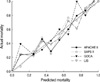
Fig. 2
ROC curves of severity scores. The discriminative power of LIS was inferior to both APACHE II and SAPS II (A). When the models incorporated patient location before MICU admission in addition to severity scores, all the areas under the curves were increased (B).
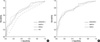
Table 2
Hosmer-Lemeshow's H and C chi-square tests for scoring systems
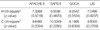
*H denotes that the tables were collapsed on fixed values of the estimated probabilities; †C denotes that the tables were collapsed on deciles of the estimated probabilities.
The models used in estimating calibrations were made using patient location before MICU admission in addition to severity scores.
References
1. Krafft P, Fridrich P, Pernerstorfer T, Fitzgerald RD, Koc D, Schneider B, Hammerle AF, Steltzer H. The acute respiratory distress syndrome: definitions, severity and clinical outcome. An analysis of 101 clinical investigations. Intensive Care Med. 1996. 22:519–529.


2. Venet C, Guyomarc'h S, Pingat J, Michard C, Laporte S, Bertrand M, Gery P, Page D, Vermesch R, Bertrand JC, Zeni F. Prognostic factors in acute respiratory distress syndrome: a retrospective multivariate analysis including prone positioning in management strategy. Intensive Care Med. 2003. 29:1435–1441.


3. Monchi M, Bellenfant F, Cariou A, Joly LM, Thebert D, Laurent I, Dhainaut JF, Brunet F. Early predictive factors of survival in the acute respiratory distress syndrome: a multivariate analysis. Am J Respir Crit Care Med. 1998. 158:1076–1081.
4. Bernard GR, Artigas A, Brigham KL, Carlet J, Falke K, Hudson L, Lamy M, Legall JR, Morris A, Spragg R. The consensus committee. The American-European Consensus Conference on ARDS. Definitions, mechanisms, relevant outcomes, and clinical trial coordination. Am J Respir Crit Care Med. 1994. 149:818–824.
5. Milberg JA, Davis DR, Steinberg KP, Hudson LD. Improved survival of patients with acute respiratory distress syndrome (ARDS): 1983-1993. JAMA. 1995. 273:306–309.


6. Zilberberg MD, Epstein SK. Acute lung injury in the medical ICU: comorbid conditions, age, etiology, and hospital outcome. Am J Respir Crit Care Med. 1998. 157:1159–1164.
7. Page B, Vieillard-Baron A, Beauchet A, Aegerter P, Prin S, Jardin F. Low stretch ventilation strategy in acute respiratory distress syndrome: eight years of clinical experience in a single center. Crit Care Med. 2003. 31:765–769.


8. Dolye RL, Szaflarski N, Modin GW, Wiener-Kronish JP, Matthay MA. Identification of patients with acute lung injury: predictors of mortality. Am J Respir Crit Care Med. 1995. 152:1818–1824.


9. Moon SH, Song SH, Jung HS, Yeun DJ, Uh ST, Kim YH, Park CS. Acute respiratory distress syndrome in respiratory intensive care unit. Tuberc Respir Dis. 1998. 45:1252–1264.


10. Seo MS, Lee PB, Lee YJ. Effect of protective strategy on outcome in patients with acute respiratory distress syndrome (ARDS). Korean J Anesthesiol. 2005. 48:153–158.


11. Knaus WA, Draper EA, Wagner DP, Zimmerman JE. APACHE II: a severity of disease classification system. Crit Care Med. 1985. 13:818–829.
12. Le Gall JR, Lemeshow S, Saulnier F. A new simplified acute physiology score (SAPS II) based on a European/North American multicenter study. JAMA. 1993. 270:2957–2963.


13. Vieillard-Baron A, Girou E, Valente E, Brun-Buisson C, Jardin F, Lemaire F, Brochard L. Predictors of mortality in acute respiratory distress syndrome. Focus on the role of right heart catheterization. Am J Respir Crit Care Med. 2000. 161:1597–1601.
14. Murray JF, Matthay MA, Luce JM, Flick MR. An expanded definition of the adult respiratory distress syndrome. Am Rev Respir Dis. 1988. 138:720–723.


15. Artigas A, Bernard GR, Carlet J, Dreyfuss D, Gattinoni L, Hudson L, Lamy M, Matthay MA, Pinsky MR, Spragg R, Suter PM. The American-European Consensus Conference on ARDS, Part 2. ventilatory pharmacologic, supportive therapy, study design strategies and issues related to recovery and remodeling. Intensive Care Med. 1998. 24:378–398.
16. Shaughnessy TE, Mickler TA. Does Acute Physiologic and Chronic Health Evaluation (APACHE II) scoring predict need for prolonged support after coronary revascularization? Anesth Analg. 1995. 81:24–29.


17. Murphy-Filkins R, Teres D, Lemeshow S, Hosmer DW. Effect of changing patient mix on the performance of an intensive care unit severity-of-illness model: how to distinguish a general from a specialty intensive care unit. Crit Care Med. 1996. 24:1968–1973.
18. Brun-Buisson C, Minelli C, Bertolini G, Brazzi L, Pimentel J, Lewandowski K, Bion J, Romand JA, Villar J, Thorsteinsson A, Damas P, Armaganidis A, Lemaire F; ALIVE Study Group. Epidemiology and outcome of acute lung injury in European intensive care units. Results from the ALIVE study. Intensive Care Med. 2004. 30:51–61.
19. Rowan KM, Kerr JH, Major E, McPherson K, Short A, Vessey MP. Intensive care society's APAHCE II study in Britain and Ireland-II: Outcome comparisons of intensive care units after adjustment for case mix by the American APACHE II method. BMJ. 1993. 307:977–981.
20. Wong D, Crofts SL, Gomez M, McGuire GP, Byrick RJ. Evaluation of predictive ability of APACHE II system and hospital outcome in Canadian intensive care unit patients. Crit Care Med. 1995. 23:1177–1183.


21. Heffner JE, Brown LK, Barbieri CA, Harpel KS, DeLeo J. Prospective validation of an acute respiratory distress syndrome predictive score. Am J Respir Crit Care Med. 1995. 152:1518–1526.


22. Reiter A, Mauritz W, Jordan B, Lang T, Polzl A, Pelinka L, Metnitz PG. Improving risk adjustment in critically ill trauma patients: the TRISS-SAPS Score. J Trauma. 2004. 57:375–380.


23. Lemeshow S, Hosmer DW Jr. A review of goodness of fit statistics for use in the development of logistic regression models. Am J Epidemiol. 1982. 115:92–106.
24. Hunt JP, Meyer AA. A predicting survival in the intensive care unit. Curr Probl Surg. 1997. 34:527–599.