Abstract
Diagnosis of asthma is often challenging in primary-care physicians due to lack of tools measuring airway obstruction and variability. Symptom-based diagnosis of asthma utilizing objective diagnostic parameters and appropriate software would be useful in clinical practice. A total of 302 adult patients with respiratory symptoms responded to a questionnaire regarding asthma symptoms and provoking factors. Questions were asked and recorded by physicians into a computer program. A definite diagnosis of asthma was made based on a positive response to methacholine bronchial provocation or bronchodilator response (BDR) testing. Multivariate logistic regression analysis was used to evaluate the significance of questionnaire responses in terms of discriminating asthmatics. Asthmatic patients showed higher total symptom scores than non-asthmatics (mean 5.93 vs. 4.93; p<0.01). Multivariate logistic regression analysis identified that response to questions concerning the following significantly discriminated asthmatics; wheezing with dyspnea, which is aggravated at night, and by exercise, cold air, and upper respiratory infection. Moreover, the presence of these symptoms was found to agree significantly with definite diagnosis of asthma (by kappa statistics). Receiver-operating characteristic curve analysis revealed that the diagnostic accuracy of symptom-based diagnosis was high with an area under the curve of 0.647±0.033. Using a computer-assisted symptom-based diagnosis program, it is possible to increase the accuracy of diagnosing asthma in general practice, when the facilities required to evaluate airway hyperresponsiveness or BDR are unavailable.
Asthma is one of the most common health problems worldwide that have great influence not only on the daily lives of affected subjects but also on their communities (1, 2). In addition, the prevalence of asthma is increasing in recent decades, accompanied by the rise of the cost of asthma care as well (3-5). One of many problems in asthma managements is that a lot of patients with asthma are not diagnosed as asthma ever and misdiagnosed as other respiratory diseases such as common cold, acute bronchitis, or chronic obstructive pulmonary disease (COPD) (6, 7). Making a correct diagnosis of asthma is very important in that it reduces cost of inappropriate treatment and leads to effective treatment of asthma.
International guidelines recommend to make a diagnosis of asthma based on typical symptoms of asthma and identification of airway hyperresponsiveness (AHR) or variable airway obstruction, the key characteristics of asthma (8). Bronchial provocation test using nonspecific stimuli, such as methacholine or histamine, is useful for the determination of AHR (9). However, it is somewhat invasive in nature and is not easy to perform for primary-care physician. In addition, it is not available in primary-care clinics or even in many general hospitals. Bronchodilator response (BDR) to short-acting β2-agonists is a valuable test to evaluate variable airway obstruction, which is only useful in patients with reduced lung function at the time of visit. Without information about AHR and BDR of a patient, a physician has to make a diagnosis of asthma based on respiratory symptoms and physical examination only. Thus the decision is not up to objective evidence of asthma but up to experience of the physician.
There have been several trials to develop asthma questionnaire to evaluate the prevalence of asthma in epidemiologic studies (10-15) or to identify asthmatics in primary care (16). Although some questionnaires were proved to be related to AHR (11, 12, 15) and clinical diagnosed asthma (16), these were seldom used in clinical practice for lack of information about their diagnostic value and inconvenience of using them on spot. Scoring systems present the likelihood of a disease or a condition of a patient numerically and make it easy to estimate at a glance. While some scoring system is now in use for evaluating asthma control concerning adequacy of treatment (17, 18), no scoring system has been developed yet for the diagnosing asthma in clinical practice. There have been a few trials to develop a scoring system for identifying asthmatics among general population (19-21), but these were not intended to predict the possibility of asthma in patients with respiratory symptoms visiting hospitals.
Recently it was possible to use personal computers in nearly almost hospitals in medical practice. Thus we tried to develop a new computer program to help physicians diagnose asthma by providing objective parameters for the possibility of asthma depending on total symptom scores and the specific symptoms each patient had. This study was designed to develop computer-assisted, symptom-based diagnosis and to evaluate whether it could be a useful approach in diagnosing asthma.
We enrolled 302 adult patients, who visited the outpatient department of six hospitals for various respiratory symptoms, such as dyspnea, cough, or wheezing. At study entry, all patients were asked by physician to respond to eleven questions regarding symptoms of asthma and provoking factors of dyspnea and wheezing (Table 1). These questions were developed or selected by asthma experts meeting in Korea among various questions, which were validated to be typical characteristics of asthma and were used for the diagnosis of asthma. The answers to each question were recorded by a computer program by physician on spot, and the total symptom score was calculated by summing up the scores corresponding to each question. Using spirometry, forced expiratory volume in one second (FEV1) was measured. Patients with FEV1 of more than 70% of predictive value underwent a methacholine bronchial provocation test (MBPT), while the rest were evaluated for BDR to short-acting β2-agonist. Definite diagnosis of asthma was made based on positive response to MBPT (PC20 <16 mg/mL of methacholine) (22) or BDR (FEV1 increase by more than 12% of baseline value and 200 mL) (23). Patients were divided according to definite diagnosis of asthma into two groups, 'asthmatics' and 'non-asthmatics'. Of 302 patients who were enrolled in this study, 210 (69.5%) showed positive response to MBPT or BDR test and were grouped as asthmatics. Baseline characteristics of both asthmatics and non-asthmatics are shown in Table 2.
Comparison of means of the total symptom score between the two groups was performed by using Student's t-test. Multivariate logistic regression analysis was performed to identify significant questions for discrimination of asthmatics using eleven questions as independent variables. To evaluate agreement of symptom-based diagnosis with definite diagnosis of asthma, kappa (κ) coefficient was calculated for each question. A receiver-operating characteristic (ROC) curve analysis was done to assess the diagnostic accuracy of symptom-based diagnosis determining values of sensitivity, specificity, positive predictive value, and negative predictive value. From ROC curve, the ROC area under the curve (AUC) and the optimal cutoff value with the highest sensitivity and specificity were obtained. A p value of <0.05 was considered to be statistically significant.
The distribution of the total symptom scores of asthmatics was relatively right-shifted compared with that of non-asthmatics with a higher mean value of 5.93 (vs. 4.93 in asthma-negative patients; p<0.01) (Fig. 1). Symptoms and provoking factors with a high prevalence in asthmatics are the followings in decreasing order of rate: wheezing with dyspnea (86%), nocturnal aggravation (64%), fluctuation of exacerbation and improvement (64%), upper respiratory infection (50%), cold air (44%), exercise (40%), etc (Fig. 2 and Table 3). Multivariate logistic regression analysis identified symptoms significantly related with asthma diagnosis based on MBPT or BDR. Nocturnal aggravation was the most significant symptom discriminating asthmatics and non-asthmatics (OR=3.152, 95% CI 1.892 to 5.253, p<0.001) (Table 3). Wheezing with dyspnea (OR=2.953, 95% CI 1.479 to 4.705, p=0.002), exercise (OR=2.353, 95% CI 1.334 to 4.150, p=0.003), cold air (OR=2.209, 95% CI 1.288 to 3.788, p=0.004), and upper respiratory infection (OR=1.968, 95% CI 1.182 to 3.277, p=0.009) were also associated with the diagnosis of asthma. Paroxysmal coughing was much higher in non-asthmatics than in asthmatics (53% vs. 23%) and negatively associated with the diagnosis of asthma (OR=0.458, 95% CI 0.278 to 0.754, p=0.002).
As a diagnostic test to tell asthmatics, each question was evaluated for its agreement with definite diagnosis of asthma by kappa statistics. Nocturnal symptom, with the highest OR in multivariate logistic regression analysis, showed fair agreement with a kappa of 0.247 (p<0.001) (Table 4). Wheezing with dyspnea (κ value=0.175, p=0.001), cold air (κ value=0.138, p=0.004), exercise (κ value=0.134, p=0.003), and upper respiratory infection (κ value=0.133, p=0.009) showed also significant agreement with the definite diagnosis of asthma, while the other symptoms were not in agreement with it.
The diagnostic value of symptom-based diagnosis was evaluated by ROC analysis of total symptom scores. ROC curve, shown in Fig. 3, represents sensitivity and specificity graphically. AUC of ROC curve was 0.647±0.033 representing that the probability that the total symptom score of a subject of asthmatics was higher than that of a subject in the normal group was 64.7% (Fig. 3). Table 5 lists the different values of sensitivity and specificity for each cutoff value of total symptom scores. With an increase of cutoff value, sensitivity decreased, while specificity increased. The cutoff value of total symptom score ≥4 was associated with the highest combination of sensitivity (85.2%) and specificity (25.0%). However, even at the same total symptom scores, the diagnostic value varied according to the combination of positive symptoms. For example, at the total symptom score of 4, sensitivities, specificities, positive predictive values, and negative predictive values were all different among various combinations of positive symptoms (Table 6). Among those combinations, the positive predictive value was highest at the combination of dyspnea with wheezing, cold air and exercise, while negative predictive value was highest at that of dyspnea with wheezing and fluctuation of exacerbation and improvement.
Our study of 302 patients with respiratory symptoms showed that computer-assisted, symptom-based diagnosis is a useful tool in diagnosing asthma. We found specific questions discriminated asthmatics well, and could raise the diagnostic power by calculating total symptom scores and combining symptoms and provoking factors of each subject. This is the first trial to develop computer-assisted, symptom-based diagnosis, which provides the objective diagnostic parameters in terms of sensitivity, specificity, positive predictive value, and negative predictive value in diagnosing asthma. These parameters could give enhanced confidence in diagnosing asthma to primary-care physicians, leading to more appropriate treatment of asthma.
Among selected asthma symptoms and provoking factors, one with the most discriminating capacity to discriminat asthmatics from non-asthmatics was dyspnea with wheezing, which is aggravated at night, and by exercise, cold air, and upper respiratory infection. This was validated by multivariate logistic regression analysis using definite diagnosis of asthma based on the result of MBPT or BDR as a dependent variable. Questions regarding provoking asthma symptoms could better discriminate asthmatics compared with other asthma symptoms. Contrary to our expectation, paroxysmal coughing was less common in asthmatics than in non-asthmatics, giving negative information in diagnosing asthma.
We tried to develop a scoring system and to find an optimal cutoff value discriminating patients with asthma from those without asthma. The ROC curve analysis revealed that the diagnosis based on total symptom score is a useful tool in diagnosing asthma (Fig. 3). However, we were not fully successful in selecting an optimal cutoff value with both the highest sensitivity and the highest specificity. For example, the total symptom score of four or more as a cutoff value showed sensitivity of 86.2% and specificity of 25.0%. Whereas the sensitivity was high enough to select asthmatics, the specificity was too low to make the correct diagnosis of asthma compared with those of others (21). Even at the same total symptom score, there were many different combinations of positive symptoms. As shown in Table 6, although the total symptom score was four in any case, sensitivity, specificity, positive predictive value, and negative predictive value were all different from each other. In cases with the total symptom scores of four, the sensitivity ranged from 13.8% to 56.2%, and the specificity was between 69.6% and 93.5%. These findings mean that diagnostic values should be drawn not merely from the total symptom scores but from the combination of positive symptoms. Using a computer program, we presented diagnostic values (sensitivity, specificity, positive predictive value, and negative predictive value) at each total symptom score and combination of positive symptoms. The example is illustrated in Table 7A, B. By doing this, general physicians were provided with objective parameters regarding the possibility of asthma based on symptoms of each patient and were able to make a diagnosis of asthma with confidence. In addition, a list of differential diagnose and check points are illustrated following diagnostic values to make the physicians to consider other possible diagnosis (Table 7C).
A computer program was developed to help diagnose childhood asthma in the general practice in Australia (16). In this program, children or their parents input information about asthma symptoms by themselves. This might lead to a collection of incorrect data regarding asthmatic symptoms, because they were not provided with any further explanation about terms or meaning in questions. In addition, this program did not give any diagnostic parameters to physician while seeing a patient.
In conclusion, by using a computer-assisted, symptom-based diagnosis program, it will be possible to make more correct diagnosis of asthma in general practice, where facilities to evaluate AHR or BDR are not available.
Figures and Tables
![]() | Fig. 2Percentages of patients who responded "yes" to each question in asthmatics and non-asthmatics. At entry, the "yes" response rate to each question is shown according to definite diagnosis of asthma. |
![]() | Fig. 3ROC curve for the total symptom score. ROC curve was plotted by sensitivity and 1-specificity of total symptom scores. |
Table 3
Percentages of patients who responded "yes" to each question in asthmatics and non-asthmatics
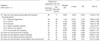
Table 5
Sensitivity and specificity of the test according to various cutoff values of total symptom scores
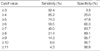
Table 6
Adequacy of test and predictability of disease according to the total symptom scores and questions with "yes" responses
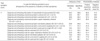
Table 7
Example of information about diagnostic values of asthma provided by a computer program
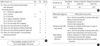
*, This banner is shown when physicians finish entering all answers to the questions. †, By clicking this hyperlinked phrase, tables such as Table 7B and 7C appear in a monitor simultaneously. ‡, TB is an abbreviation for tuberculosis. COPD, chronic obstructive pulmonary disease; EKG, electrocardiography.
References
1. The International Study of Asthma and Allergies in Childhood (ISAAC) Steering Committee. Worldwide variation in prevalence of symptoms of asthma, allergic rhinoconjunctivitis, and atopic eczema: ISAAC. Lancet. 1998. 351:1225–1232.
2. Kim YK, Kim SH, Tak YJ, Jee YK, Lee BJ, Kim SH, Park HW, Jung JW, Bahn JW, Chang YS, Choi DC, Chang SI, Min KU, Kim YY, Cho SH. High prevalence of current asthma and active smoking effect among the elderly. Clin Exp Allergy. 2002. 32:1706–1712.


3. Singh M. The burden of asthma in children: an Asian perspective. Paediatr Respir Rev. 2005. 6:14–19.


4. Sennhauser FH, Braun-Fahrlander C, Wildhaber JH. The burden of asthma in children: a european perspective. Paediatr Respir Rev. 2005. 6:2–7.


5. Beasley R. The burden of asthma with specific reference to the united states. J Allergy Clin Immunol. 2002. 109:5 suppl. S482–S489.


7. Enright PL, McClelland RL, Newman AB, Gottlieb DJ, Lebowitz MD. Underdiagnosis and undertreatment of asthma in the elderly. Cardiovascular health study research group. Chest. 1999. 116:603–613.
8. Global initiative for asthma 2002. Update from: Global strategy for asthma management and prevention nhlbi/who workshop report 1995. 2002. Bethesda, md.: National institutes of health;(dhhs publication no. (NIH) 02-3659.).
9. Van Schoor J, Joos GF, Pauwels RA. Indirect bronchial hyperresponsiveness in asthma: Mechanisms, pharmacology and implications for clinical research. Eur Respir J. 2000. 16:514–533.


10. Burney P, Chinn S. Developing a new questionnaire for measuring the prevalence and distribution of asthma. Chest. 1987. 91:6 suppl. 79S–83S.


11. Burney PG, Laitinen LA, Perdrizet S, Huckauf H, Tattersfield AE, Chinn S, Poisson N, Heeren A, Britton JR, Jones T. Validity and repeatability of the IUATLD (1984) bronchial symptoms questionnaire: An international comparison. Eur Respir J. 1989. 2:940–945.
12. Venables KM, Farrer N, Sharp L, Graneek BJ, Newman Taylor AJ. Respiratory symptoms questionnaire for asthma epidemiology: Validity and reproducibility. Thorax. 1993. 48:214–219.


13. Bai J, Peat JK, Berry G, Marks GB, Woolcock AJ. Questionnaire items that predict asthma and other respiratory conditions in adults. Chest. 1998. 114:1343–1348.


14. Toren K, Brisman J, Jarvholm B. Asthma and asthma-like symptoms in adults assessed by questionnaires. A literature review. Chest. 1993. 104:600–608.
15. Shaw RA, Crane J, Pearce N, Burgess CD, Bremner P, Woodman K, Beasley R. Comparison of a video questionnaire with the iuatld written questionnaire for measuring asthma prevalence. Clin Exp Allergy. 1992. 22:561–568.


16. Kable S, Henry R, Sanson-Fisher R, Ireland M, Corkrey R, Cockburn J. Childhood asthma: can computers aid detection in general practice? Br J Gen Pract. 2001. 51:112–116.
17. Juniper EF, O'Byrne PM, Guyatt GH, Ferrie PJ, King DR. Development and validation of a questionnaire to measure asthma control. Eur Respir J. 1999. 14:902–907.


18. Juniper EF, Norman GR, Cox FM, Roberts JN. Comparison of the standard gamble, rating scale, aqlq and sf-36 for measuring quality of life in asthma. Eur Respir J. 2001. 18:38–44.


19. Frank TL, Frank PI, McNamee R, Wright T, Hannaford P, Morrison J, Hirsch S, Pickering CA. Assessment of a simple scoring system applied to a screening questionnaire of asthma in children aged 5-15 yrs. Eur Respir J. 1999. 14:1190–1197.
20. Hirsch S, Frank TL, Shapiro JL, Hazell ML, Frank PI. Development of a questionnaire weighted scoring system to target diagnostic examinations for asthma in adults: A modelling study. BMC Fam Pract. 2004. 5:30.


21. Frank PI, Frank TL, Cropper J, Hirsch S, Niven RM, Hannaford P, McNamee R. The use of a screening questionnaire to identify children with likely asthma. Br J Gen Pract. 2001. 51:117–120.
22. Crapo RO, Casaburi R, Coates AL, Enright PL, Hankinson JL, Irvin CG, MacIntyre NR, McKay RT, Wanger JS, Anderson SD, Cockcroft DW, Fish JE, Sterk PJ. Guidelines for methacholine and exercise challenge testing-1999. This official statement of the American Thoracic Society was adopted by the ATS board of directors, July 1999. Am J Respir Crit Care Med. 2000. 161:309–329.
23. American thoracic society. Lung function testing: Selection of reference values and interpretative strategies. Am Rev Respir Dis. 1991. 144:1202–1218.