Abstract
Objectives
This study evaluated the structural characteristics of a scientific network of psychiatry and the effect of social networks on the performance of scholars.
Methods
The data were extracted from 261 articles published from 1996 to 2013 in the Journal of the Korean Neuropsychiatric Association, and were transformed into a co-author and their affiliation matrix. We used measures from network analysis (i.e., degree centrality, weighted degree centrality, eigenvector centrality, betweenness centrality) for evaluating the effect of co-authorship network on the performance of scholars (h-index). Netminer 4.1 was used for the network analysis.
Results
Both co-authorship and affiliation network demonstrated power law distribution. Coauthor's centralities were correlated with research achievements. Results from poisson regression analysis showed that the eigenvector centrality has a significant positive influence on the h-index and the weighted degree centrality has a significant negative influence on the h-index.
Conclusion
This study shows that the small world phenomenon exists in the psychiatric coauthorship network, and finds collaboration patterns and effects on scientific performance. The results suggest that in order to achieve better research performance it would be helpful for scholars to work with other well-performing scholars and avoid other scholars who previously worked together.
Figures and Tables
Fig. 1
Co-authorship network in psychiatry in Korea from 2009 to 2013. Each node represents one author in the data. The size of each node is proportional to the eigenvector centrality. Color shifts from red to purple indicate h-index of each node from lower to larger, respectively. Each link between the nodes indicates their connection.
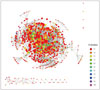
Fig. 2
Affiliation network in psychiatry in Korea from 2009 to 2013. Each node represents one affiliation. The size of each node is proportional to the eigenvector centrality. Color shifts from red to purple indicate degree centrality of each node from lower to larger, respectively. Each link between the nodes indicates their connection.
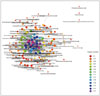
References
1. Scott J. Social Network Analysis. 3rd ed. London: Sage;2012.
2. Barabási AL, Gulbahce N, Loscalzo J. Network medicine: a network-based approach to human disease. Nat Rev Genet. 2011; 12:56–68.


3. Yan E, Ding Y. Applying centrality measures to impact analysis: a coauthorship network analysis. J Am Soc Inf Sci Technol. 2009; 60:2107–2118.


4. Freeman LC. Centrality in social networks conceptual clarification. Soc Networks. 1979; 1:215–239.


5. Hanneman RA, Mark Riddle. [homepage on Internet]. Introduction to social network methods. Riverside, CA: University of California;Riverside, 2005. cited 2015 Apr 10. Available from: http://faculty.ucr.edu/~hanneman/.
6. Jung M, Chung D. [Co-author and keyword networks and their clustering appearance in preventive medicine fields in Korea: analysis of papers in the Journal of Preventive Medicine and Public Health, 1991~2006]. J Prev Med Public Health. 2008; 41:1–9.


7. Abbasi A, Altmann J, Hossain L. Identifying the effects of co-authorship networks on the performance of scholars: a correlation and regression analysis of performance measures and social network analysis measures. J Informetr. 2011; 5:594–607.


8. Hill VA. Collaboration in an academic setting: does the network structure matter? Center for the Computational Analysis of Social and Organizational Systems. 2008. Available from: http://www.casos.cs.cmu.edu/publications/papers/CMU-ISR-08-128.pdf.
10. National Research Foundation of Korea. cited 2015 Apr 10. Available from: https://www.kci.go.kr/.
11. Hirsch JE. An index to quantify an individual's scientific research output. Proc Natl Acad Sci U S A. 2005; 102:16569–16572.


12. Moussa S, Touzani M. Ranking marketing journals using the Google Scholar-based hg-index. J Informetr. 2010; 4:107–117.


13. Cyram N. Cyram Netminer 4.1. Seoul: Cyram;2013.
14. Gansner ER, Koren Y, North S. Graph drawing by stress majorization. In : Pach J, editor. Graph Drawing. . New York: Springer Berlin Heidelberg;2005. p. 239–250.
15. Carrington PJ, Scott J, Wasserman S. Models and methods in social network analysis. Vol. 28. Cambridge: Cambridge University Press;2005.
16. Knoke D, Yang S. Social network analysis. 2nd ed. Thousand Oaks, CA: Sage;2008.
17. Kadushin C. Understanding social networks: theories, concepts and findings. . New York: Oxford University Press;2012. p. 127–128.
18. Clauset A, Shalizi CR, Newman MEJ. Power-law distributions in empirical data. SIAM Rev Soc Ind Appl Math. 2009; 51:661–703.

