Abstract
Coronary heart disease (CHD) is a significant cause of morbidity and mortality worldwide. Many risk prediction models have been developed in an effort to assist clinicians in risk assessment and the prevention of CHD. However, it is unclear whether the existing CHD prediction tools can improve clinical performance, and recently, there has been a lot of effort being made to improve the accuracy of the prediction models. A large number of novel biomarkers have been identified to be associated with cardiovascular risk, and studied with the goal of improving the accuracy and clinical utility of CHD risk prediction. Yet, controversy still remains with regard to the utility of novel biomarkers in CHD risk assessment, and in finding the best statistical methods to assess the incremental value of the biomarkers. This article discusses the statistical approaches that can be used to evaluate the predictive values of new biomarkers, and reviews the clinical utility of novel biomarkers in CHD prediction, specifically in the Korean population.
Epidemiology has contributed to the improvement of clinical practice in diverse ways. Epidemiological studies have identified most major risk factors, especially for cardiovascular disease (CVD). Clinical epidemiology also plays a critical role in the development and evaluation of disease screening, diagnosis, and treatment modalities. Disease risk prediction is a relatively new, but very active area of research where epidemiologic findings are translated into clinical applications. If we could better predict an individual's future risk for certain diseases, we would then be able to make an efficient and personalized treatment plan for the individual. Traditional epidemiologic studies measure the strength of association between a risk factor and a disease in relative terms such as relative risk and odds ratio. These measures tell us the "relative difference of disease risk" between a group of people who are exposed to the risk factor and a group who are not exposed. However, with these relative measures, we cannot estimate an individual's "absolute" disease risk, which is more relevant information when making decision in a clinical setting. Additionally, an individual can be exposed to multiple risk factors at various exposure levels, and this makes it difficult to estimate an individual's absolute disease risk. For these reasons, disease risk prediction models have been developed to assess multiple risk factors together and estimate absolute disease risk for each individual.
The predictive capacities among the known major cardiovascular risk factors including age, sex, cigarette smoking, high blood pressure, abnormal blood lipids, and diabetes mellitus have been well established. Using these major risk factors in a combined manner, risk prediction tools have been developed in an effort to assist clinicians in cardiovascular risk assessment and in treatment planning.1-3) The most widely accepted model is the Framingham Risk Score which enrolls traditional risk factors such as age, diabetes, smoking, blood pressure, total (or low density lipoprotein) cholesterol, and high density lipoprotein-cholesterol (HDL-C) with different points (coefficients) in order to assess an individual's 10-year coronary heart disease (CHD) risk.4) The third Report of the National Cholesterol Education Program Expert Panel on Detection, Evaluation, and Treatment of High Blood Cholesterol in Adults {Adult Treatment Panel III (ATP-III)} and many other guidelines for CHD risk assessment and prevention are largely based on the Framingham equations to predict an individual's 10-year risk of CHD.5-7) For example, according to the ATP-III guidelines, individuals can be categorized into one of 3 risk categories shown in Fig. 1 below.8) For individuals without established CHD or CHD risk equivalents, a primary prevention strategy is determined by using their predicted 10-year risk for CHD. Individuals with a 10-year CHD risk >20% are considered to be at high risk and recommended for immediate use of lipid-lowering medication. For individuals with moderate risk, the recommendation can be either to start drug therapy or to pursue other noninvasive testing for further risk stratification. Low risk individuals are typically not recommended for drug therapy, but for lifestyle modification as appropriate treatment. Studies have shown that the CHD risk prediction models, which are based on traditional risk factors, are considered acceptable in various populations.9) However, there is little evidence supporting the idea that existing CHD risk prediction tools can improve clinical outcomes,10) and there has been much effort to improve the accuracy of the existing CHD risk prediction tools.
A large number of newer biomarkers, which reflect inflammation, endothelial function, fibrin formation and fibrinolysis, oxidative stress, renal function, ventricular function, and even myocardial cell damage have been reported to be associated with cardiovascular risk and their predictive values have been studied.11-20) However, only a small number of studies have observed a significant improvement by adding newer biomarkers in the prediction of CHD risk. Even in studies that observed improved predictive power when including new biomarkers, the incremental predictive powers were disappointingly small. A multi-marker risk prediction approach, which includes several newer biomarkers simultaneously, has been studi-ed with the goal of improving the accuracy and clinical utility of CHD risk prediction.21-24) Some studies have suggested that adding several newer biomarkers can substantially improve risk classification, 23)24) but others have observed only minimal improvement in the ability to classify cardiovascular risk by adding multiple biomarkers.21)22) Thus, for most of the newer biomarkers, controversy remains in the predictability of CHD in the clinical setting. Debate remains about the appropriate statistical methods for assessing the incremental value of new biomarkers in the CHD prediction model.
When a new biomarker is proposed as a risk predictor, the most common approach is to compare a new prediction model, including the new biomarker, to the existing prediction models that do not include the new biomarker. A new prediction model should meet at least 2 criteria in order to replace the existing disease prediction model. First, the new model must predict disease risk more accurately than existing models. Second, the predicted risks must be sufficiently different between the existing and new models to permit changes to an individual's treatment plan.1)25)26) Diverse statistical measures should be used to check whether a new prediction model meets th-ese 2 criteria. McGeechan et al.25) proposed to assess the clinical utility of new biomarkers or prediction models in 4 summary measures; global model fitness, discrimination, calibration, and reclassification. "Global model fitness" can be assessed in many ways, among which the Akaike Information Criterion and Bayes the Information Criterion are the most commonly used measures. These methods are useful to not only find the best fit, but also a parsimonious prediction model, because a penalty is paid for increasing the number of predictor variables. A model with fewer variables would be preferred among the alternative models of equal prediction performance. "Discrimination" is a measure of how well a proposed model separates people who develop the disease of interest from people who do not. The most common measure of discrimination is the C-statistic {or area under the receiver operating characteristic curve, area under the curve (AUC)}.27) Incremental discriminative power can be assessed by comparing AUCs between the new model and the existing model (Fig. 2). The discriminatory power of disease prediction can be also assessed by other indices including, the Yates slope (the difference between predicted risk between cases and controls; larger values indicate better discrimination), the Brier score (the sum of squared difference between the observed outcome and fitted probability; smaller values indicate better fit), and the integrated discrimination improvement.28)29) "Calibration" refer to how close the predicted disease risks are to the actual observed risks. Usually, the mean predicted risk and observed actual risk are compared according to different risk groups (e.g., deciles of risk) (Fig. 3). The significance of the difference between the predicted and actual risks can be tested by the Hosmer and Lemeshow28) chi-square test. "Reclassification" methods have been suggested to provide more clinically relevant information by comparing risk classification results from a new model to those from an existing model.29) Discrimination indices do not provide information about the proportion of people who are classified into low or high risk groups, but reclassification tables can measure the changes in predicted risk categories between the existing and new models. In addition, the net reclassification improvement can be calculated for those changes in estimated prediction probabilities that imply a change from one category to another according to the method described by Pencina et al.29) (Fig. 4). In addition to the 4 measures described above, bar graphs and scatter plots may effectively show clinically important information about the predictive performance (Fig. 5).2) Lastly calculating the number needed to screen (NNS) is another useful way to assess the clinical utility of new biomarkers or prediction models. The NNS indicates the number of people needed to be tested for the additional biomarker to identify the high-risk individual.
Annually, more than 1000 studies report independent disease risk factors or predictors,30) and CHD is among the most frequently studied diseases. Numerous biomarkers have been reported to be independently associated with CHD risk. However, most clinicians do not measure these biomarkers in daily practice, because their clinical utility is limited or uncertain. Newer biomarkers may reflect or play a role in inflammation, endothelial dysfunction, fibrin formation and fibrinolysis, oxidative stress, renal dysfunction, ventricular dysfunction, and myocardial cell damage.11-20) Although some of these new biomarkers were consistently and independently associated with CHD risk, the clinical utility of new biomarkers is minimal to moderate in the prediction of CHD. A good example is C-reactive protein (CRP), an inflammatory marker. CRP level has been studied extensively as a potential biomarker that can improve CHD risk prediction, 16)22)31-36) but its clinical utility remains controversial. In the Women's Health Study, CRP was strongly associated with CHD risk and improved disease prediction.33)36)37) Nested case-control analyses in the Nurses' Health Study and in the Health Professional Follow-up Study observed that CRP level was independently associated with CHD risk in men, but not in women.34) In the British Women's Heart and Health Study, CRP was not significantly associated with either CHD or CVD, and it did not improve the identification of risk in women.35) In the Women's Health Study, CRP levels ≥3 mg/L were significantly associated with CVD risk, independent of metabolic abnormalities.33) However, those relationships were not observed in the nested case-control studies performed in the Nurses' Health Study.34) In the Women's Health Initiative datasets, CRP was not significantly associated with CHD after adjustment for traditional risk factors (p=0.767), and did not improve discriminative power of CHD prediction (p=0.296).2) According to a systematic review by Shah et al.,38) CRP level is consistently associated with CHD risk, but CRP testing provides only limited information for risk prediction than tests of association alone might suggest. In summary, there is strong evidence that CRP level is associated with CHD risk, but its clinical utility as a routine screening test is still uncertain because moderate improvement in risk stratification has been observed only in parts of the studies.39) Researchers examined other biomarkers including lipoprotein-associated phospholipase A2, N-terminal prohormone of brain natriuretic peptide (NT-proBNP), troponins, myeloperoxidase, fibrinogen, fibrin, D-dimer, macrophage chemoatractant protein-1, adiponenctin, cystatin C, and interleukin-6.26) Many of these biomarkers were consistently associated with CHD incidence or mortality but their practical value in the CHD prediction was not evident.
Even with the multiple biomarker approach, we have not yet found a reliable set of biomarkers that improves CHD risk stratification sufficiently. When compared to the risk prediction using conventional risk factors, adding multiple biomarkers did not significantly improve discrimination index in the Framingham Heart Study21) and the Cardiovascular Health Study.40) In the nested case-control study from the Women's Health Initiative, adding the 5 most significant biomarkers moderately improved discrimination between cases and controls (C-statistic increased by 0.016, p=0.027).2) On the other hand, the Uppsala Longitudinal Study reported that a set of biomarkers (CRP, NT-proBNP, troponin I, and cystatin C) markedly increased C-statistic by 0.11 (p<0.001).24) However the Uppsala study can be distinguished from others in several aspects; their study population was older (≥75 years), the primary outcome was not CHD incidence but mortality, and the biomarker panel included cystatin C, troponins, and NT-proBNP reflect existing cardiac or renal damage.
The United States Preventive Services Task Force systematically reviewed the 9 most commonly studied emerging risk factors {CRP level, coronary artery calcium, lipoprotein(a), homocysteine, leukocyte count, fasting glucose level, periodontal disease, ankle-brachial index, and carotid inima-media thickness} for the prediction of CHD, and evaluated the clinical usefulness of these 9 risk factors.41) They concluded that the current evidence does not support the routine use of any of the 9 risk factors for improved CHD risk stratification. In addition, the task force discussed CRP as being the best candidate for use in screening, but it remains uncertain as to whether a change in CRP will lead to the primary prevention of CHD.39)
Cardiovascular risk prediction tools have also been suggested for the Korean population42)43) but they are of limited clinical use for a few reasons. First, the clinical utility of newer biomarkers in CHD prediction can be population-specific. When we add a new biomarker measurement to existing risk predictors, the added biomarker should be able to improve the predicted risk enough to change an individual's treatment plan. This issue is of concern within the scope of clinically relevant risk reclassification, and is critically important in Korea. Clinically significant reclassification is not frequent in Korea. Because CHD incidence is relatively low in Korea, most individuals fall into the low-risk group when their CHD risk is assessed. Even if a new biomarker significantly improves the prediction accuracy, changes in the predicted CHD risk are mostly within the same risk category. This makes it difficult to identify high-risk individuals by measuring additional biomarkers.
Clinically useful biomarkers in a certain population can be of little use in a different population.
Moreover, clinical practice guidelines for risk assessment and the prevention of CHD, which are customized for the Korean population, are not available. Thus, it is impractical to evaluate whether a new biomarker could change an individual's treatment plan or not. In addition to the clinically relevant reclassification, the cost-effectiveness of the testing of new biomarkers needs to be determined. In order to determine the clinical utility of new biomarkers in the prediction of CHD, we need a wealth of epidemiological and clinical data that are more relevant to the Korean population. Further studies should be finely designed to answer the full range of issues and criteria relating to utilization on a clinical level.
In summary, biomarkers that are currently available provide only a minimal to moderate improvement in the prediction of CHD risk. Some newer biomarkers may have the potential to improve CHD risk assessment and may contribute to the personalized primary prevention of CHD, however newer biomarkers should be assessed more extensively for their predictive power and clinical utility, especially in the Korean population.
Figures and Tables
Fig. 1
CHD risk classification according to the ATP-III guidelins. Coronary heart disease risk equivalents include peripheral arterial disease, abdominal aortic aneurysm, and carotid artery disease (i.e., transient ischemic attacks, stroke of carotid origin, or >50% obstruction), or diabetes mellitus. Risk factors include cigarette smoking, hypertension, low HDL-C (<40 mg/dL), family history of premature CHD, and age (men ≥45 years; women ≥55 years). CHD: coronary heart disease, ATP-III: Adult Treatment Panel III, HDL-C: high density lipoprotein-cholesterol.
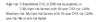
Fig. 2
Simulated area under the receiver operating characteristic curves (AUC or C-statistic). AUC: area under the curve.

Fig. 3
Simulated calibration charts for 10-year coronary heart disease prediction. A shows good calibration, while B shows poor calibration. CHD: coronary heart disease.
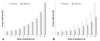
Fig. 4
Simulated reclassification tables comparing two prediction models. Net reclassification index (NRI)=Proportion of cases who were reclassified into higher category (11.7+1.7+1.0=14.4%)-Proportion of cases who were reclassified into lower category (0.7+0.3+0.7=1.7%)+Proportion of controls who were reclassified into lower category (0.8+0.3+0.5=1.6%)-Proportion of controls who were reclassified into higher category (3.3+0.8+0.5=4.6%)=9.8%.
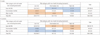
Fig. 5
Simulated scatter plot showing the performance of two prediction models. Both X and Y axes are in logarithmic scales. Red dots indicate people who developed CHD, and blacks dots indicate people who did not. Blue lines indicate cut-off points determining risk categories. CHD: coronary heart disease.
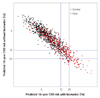
Acknowledgments
This work was supported by a grant from the Korea Healthcare technology R&D Project of the Ministry of Health and Welfare, Republic of Korea (A102065).
References
1. Lloyd-Jones DM. Cardiovascular risk prediction: basic concepts, current status, and future directions. Circulation. 2010. 121:1768–1777.
2. Kim HC, Greenland P, Rossouw JE, et al. Multimarker prediction of coronary heart disease risk: the Women's Health Initiative. J Am Coll Cardiol. 2010. 55:2080–2091.
3. Wood AM, Greenland P. Evaluating the prognostic value of new cardiovascular biomarkers. Dis Markers. 2009. 26:199–207.
4. Wilson PW, D'Agostino RB, Levy D, Belanger AM, Silbershatz H, Kannel WB. Prediction of coronary heart disease using risk factor categories. Circulation. 1998. 97:1837–1847.
5. National Cholesterol Education Program (NCEP) Expert Panel on Detection, Evaluation, and Treatment of High Blood Cholesterol in Adults (adult treatment panel III). Third report of the National Cholesterol Education Program (NCEP) expert panel on detection, evaluation, and treatment of high blood cholesterol in adults (adult treatment panel III) final report. Circulation. 2002. 106:3143–3421.
6. Wood D, De Backer G, Faergeman O, Graham I, Mancia G, Pyörälä K. Prevention of coronary heart disease in clinical practice: recommendations of the Second Joint Task Force of European and other Societies on Coronary Prevention. Atherosclerosis. 1998. 140:199–270.
7. De Backer G, Ambrosioni E, Borch-Johnsen K, et al. European guidelines on cardiovascular disease prevention in clinical practice: Third Joint Task Force of European and other Societies on Cardiovascular Disease Prevention in Clinical Practice (constituted by representatives of eight societies and by invited experts). Atherosclerosis. 2004. 173:381–391.
8. Grundy SM, Cleeman JI, Merz CN, et al. Implications of recent clinical trials for the National Cholesterol Education Program Adult Treatment Panel III guidelines. J Am Coll Cardiol. 2004. 44:720–732.
9. D'Agostino RB Sr, Grundy S, Sullivan LM, Wilson P. CHD Risk Prediction Group. Validation of the Framingham coronary heart disease prediction scores: results of a multiple ethnic groups investigation. JAMA. 2001. 286:180–187.
10. Sheridan SL, Crespo E. Does the routine use of global coronary heart disease risk scores translate into clinical benefits or harms? A systematic review of the literature. BMC Health Serv Res. 2008. 8:60.
11. Cushman M, Lemaitre RN, Kuller LH, et al. Fibrinolytic activation markers predict myocardial infarction in the elderly: the Cardiovascular Health Study. Arterioscler Thromb Vasc Biol. 1999. 19:493–498.
12. Mangoni AA, Jackson SH. Homocysteine and cardiovascular disease: current evidence and future prospects. Am J Med. 2002. 112:556–565.
13. Ridker PM, Rifai N, Rose L, Buring JE, Cook NR. Comparison of C-reactive protein and low-density lipoprotein cholesterol levels in the prediction of first cardiovascular events. N Engl J Med. 2002. 347:1557–1565.
14. Chambless LE, Folsom AR, Sharrett AR, et al. Coronary heart disease risk prediction in the Atherosclerosis Risk in Communities (ARIC) Study. J Clin Epidemiol. 2003. 56:880–890.
15. Koenig W, Löwel H, Baumert J, Meisinger C. C-reactive protein modulates risk prediction based on the Framingham score: implications for future risk assessment: results from a large cohort study in southern Germany. Circulation. 2004. 109:1349–1353.
16. Danesh J, Wheeler JG, Hirschfield GM, et al. C-reactive protein and other circulating markers of inflammation in the prediction of coronary heart disease. N Engl J Med. 2004. 350:1387–1397.
17. Wang TJ, Larson MG, Levy D, et al. Plasma natriuretic peptide levels and the risk of cardiovascular events and death. N Engl J Med. 2004. 350:655–663.
18. Danesh J, Lewington S, Thompson SG, et al. Plasma fibrinogen level and the risk of major cardiovascular diseases and nonvascular mortality: an individual participant meta-analysis. JAMA. 2005. 294:1799–1809.
19. Shlipak MG, Sarnak MJ, Katz R, et al. Cystatin C and the risk of death and cardiovascular events among elderly persons. N Engl J Med. 2005. 352:2049–2060.
20. Zethelius B, Johnston N, Venge P. Troponin I as a predictor of coronary heart disease and mortality in 70-year-old men: a community-based cohort study. Circulation. 2006. 113:1071–1078.
21. Wang TJ, Gona P, Larson MG, et al. Multiple biomarkers for the prediction of first major cardiovascular events and death. N Engl J Med. 2006. 355:2631–2639.
22. Folsom AR, Chambless LE, Ballantyne CM, et al. An assessment of incremental coronary risk prediction using C-reactive protein and other novel risk markers: the atherosclerosis risk in communities study. Arch Intern Med. 2006. 166:1368–1373.
23. Ridker PM, Buring JE, Rifai N, Cook NR. Development and validation of improved algorithms for the assessment of global cardiovascular risk in women: the Reynolds Risk Score. JAMA. 2007. 297:611–619.
24. Zethelius B, Berglund L, Sundström J, et al. Use of multiple biomarkers to improve the prediction of death from cardiovascular causes. N Engl J Med. 2008. 358:2107–2116.
25. McGeechan K, Macaskill P, Irwig L, Liew G, Wong TY. Assessing new bio-markers and predictive models for use in clinical practice: a clinician's guide. Arch Intern Med. 2008. 168:2304–2310.
26. Blumenthal RS, Foody JM, Wong ND, Braunwald E. Preventive Cardiology: a Companion to Braunwald's Heart Disease. 2011. Philadelphia, PA: Elsevier Saunders.
27. Cook NR. Use and misuse of the receiver operating characteristic curve in risk prediction. Circulation. 2007. 115:928–935.
28. Hosmer DW, Lemeshow S. Applied Logistic Regression. 2000. 2nd ed. New York: John Wiley & Sons, Inc..
29. Pencina MJ, D'Agostino RB Sr, D'Agostino RB Jr, Vasan RS. Evaluating the added predictive ability of a new marker: from area under the ROC curve to reclassification and beyond. Stat Med. 2008. 27:157–172.
30. Brotman DJ, Walker E, Lauer MS, O'Brien RG. In search of fewer independent risk factors. Arch Intern Med. 2005. 165:138–145.
31. Van der Meer IM, de Maat MP, Kiliaan AJ, van der Kuip DA, Hofman A, Witteman JC. The value of C-reactive protein in cardiovascular risk prediction: the Rotterdam Study. Arch Intern Med. 2003. 163:1323–1328.
32. Wilson PW, Nam BH, Pencina M, D'Agostino RB Sr, Benjamin EJ, O'Donnell CJ. C-reactive protein and risk of cardiovascular disease in men and women from the Framingham Heart Study. Arch Intern Med. 2005. 165:2473–2478.
33. Ridker PM, Buring JE, Cook NR, Rifai N. C-reactive protein, the metabolic syndrome, and risk of incident cardiovascular events: an 8-year follow-up of 14 719 initially healthy American women. Circulation. 2003. 107:391–397.
34. Pischon T, Hu FB, Rexrode KM, Girman CJ, Manson JE, Rimm EB. Inflammation, the metabolic syndrome, and risk of coronary heart disease in women and men. Atherosclerosis. 2008. 197:392–399.
35. May M, Lawlor DA, Brindle P, Patel R, Ebrahim S. Cardiovascular disease risk assessment in older women: can we improve on Framingham? British Women's Heart and Health prospective cohort study. Heart. 2006. 92:1396–1401.
36. Everett BM, Kurth T, Buring JE, Ridker PM. The relative strength of C-reactive protein and lipid levels as determinants of ischemic stroke compared with coronary heart disease in women. J Am Coll Cardiol. 2006. 48:2235–2242.
37. Cook NR, Buring JE, Ridker PM. The effect of including C-reactive protein in cardiovascular risk prediction models for women. Ann Intern Med. 2006. 145:21–29.
38. Shah T, Casas JP, Cooper JA, et al. Critical appraisal of CRP measurement for the prediction of coronary heart disease events: new data and systematic review of 31 prospective cohorts. Int J Epidemiol. 2009. 38:217–231.
39. Buckley DI, Fu R, Freeman M, Rogers K, Helfand M. C-reactive protein as a risk factor for coronary heart disease: a systematic review and meta-analyses for the U.S. Preventive Services Task Force. Ann Intern Med. 2009. 151:483–495.
40. Shlipak MG, Fried LF, Cushman M, et al. Cardiovascular mortality risk in chronic kidney disease: comparison of traditional and novel risk factors. JAMA. 2005. 293:1737–1745.
41. Helfand M, Buckley DI, Freeman M, et al. Emerging risk factors for coronary heart disease: a summary of systematic reviews conducted for the US Preventive Services Task Force. Ann Intern Med. 2009. 151:496–507.
42. Jee SH, Park JW, Lee SY, et al. Stroke risk prediction model: a risk profile from the Korean study. Atherosclerosis. 2008. 197:318–325.
43. Barzi F, Patel A, Gu D, et al. Cardiovascular risk prediction tools for populations in Asia. J Epidemiol Community Health. 2007. 61:115–121.