Abstract
Purpose
Materials and Methods
Figures and Tables
Fig. 1
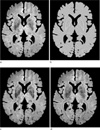
Fig. 2
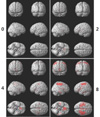
Table 1
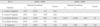
Note.- R, Right, L, Left
PSWI: paramagnetically (or positively, P) phase-masked susceptibility-weighted imaging (SWI)
NSWI: diamagnetically (or negatively, N) phase-masked SWI
After the threshold with family-wise error rate (FWE) p = 0.005 as the cluster level, only the Talairach coordinates with T-score greater than 6 are indicated on the list.
*Areas from results of voxel-based comparisons between NSWI and PSWI were selected to analyze region-of-interests (ROI).
Table 2
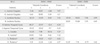
Note.- R, Right, L, Left
PSWI: paramagnetically (or positively, P) phase-masked susceptibility-weighted imaging (SWI)
NSWI: diamagnetically (or negatively, N) phase-masked SWI
After the threshold with family-wise error rate (FWE) p = 0.005 as the cluster level, only the Talairach coordinates with T-score greater than 6 are indicated on the list.
*Areas from results of voxel-based comparisons between NSWI and PSWI were selected to analyze region-of-interests (ROI).
Table 3
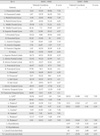
Note.- R, Right, L, Left
PSWI: paramagnetically (or positively, P) phase-masked susceptibility-weighted imaging (SWI)
NSWI: diamagnetically (or negatively, N) phase-masked SWI after the threshold with family-wise error rate (FWE) p = 0.005 as the cluster level, only the Talairach coordinates with T-score greater than 6 are indicated on the list.
*Areas from results of voxel-based comparisons between NSWI and PSWI were selected to analyze region-of-interests (ROI).
Table 4
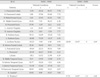
Note.- R, Right, L, Left
PSWI: paramagnetically (or positively, P) phase-masked susceptibility-weighted imaging (SWI)
NSWI: diamagnetically (or negatively, N) phase-masked SWI after the threshold with family-wise error rate (FWE) p = 0.005 as the cluster level, only the Talairach coordinates with T-score greater than 6 are indicated on the list.
*Areas from results of voxel-based comparisons between NSWI and PSWI were selected to analyze region-of-interests (ROI).