Abstract
Better biomarkers are urgently needed to cancer detection, diagnosis, and prognosis. While the genomics community is making significant advances in understanding the molecular basis of disease, proteomics will delineate the functional units of a cell, proteins and their intricate interaction network and signaling pathways for the underlying disease. Great progress has been made to characterize thousands of proteins qualitatively and quantitatively in complex biological systems by utilizing multi-dimensional sample fractionation strategies, mass spectrometry and protein microarrays. Comparative/quantitative analysis of high-quality clinical biospecimen (e.g., tissue and biofluids) of human cancer proteome landscape has the potential to reveal protein/peptide biomarkers responsible for this disease by means of their altered levels of expression, post-translational modifications as well as different forms of protein variants. Despite technological advances in proteomics, major hurdles still exist in every step of the biomarker development pipeline. The National Cancer Institute's Clinical Proteomic Technologies for Cancer initiative (NCI-CPTC) has taken a critical step to close the gap between biomarker discovery and qualification by introducing a pre-clinical "verification" stage in the pipeline, partnering with clinical laboratory organizations to develop and implement common standards, and developing regulatory science documents with the US Food and Drug Administration to educate the proteomics community on analytical evaluation requirements for multiplex assays in order to ensure the safety and effectiveness of these tests for their intended use.
In the post-genome era, the field of proteomics sparked great interest in the pursuit of protein/peptide biomarkers especially after mass spectrometry (MS) demonstrated the capability of characterizing a large number of proteins and their post-translational modifications (PTMs) in complex biological systems [1-12]. Technological advances in protein science such as protein/antibody chips, depletion of multiple high abundance proteins by affinity columns, and affinity enrichment of targeted protein analytes as well as multidimensional chromatographic fractionation, all of which expanded the dynamic range of detection for low abundance proteins by several orders of magnitude in serum or plasma, have made it possible for the detection of disease-relevant proteins in these complex biological matrices [13-21]. In fact, proteomics has been widely applied in various areas of science, ranging from the deciphering of molecular pathogenesis of diseases, the characterization of novel drug targets, to the discovery of potential diagnostic and prognostic biomarkers, where the technology is able to identify and quantify proteins associated with a particular disease by means of their altered levels of expression [22-24] and/or PTMs [25-27] between the control and disease states (i.e., biomarker candidates). This type of comparative (semi-quantitative) analysis enables correlations to be drawn between the range of proteins, their variations and modifications produced by a cell, tissue and biofluids and the initiation, progression, therapeutic monitoring or remission of a disease state. Post-translational modifications including phosphorylation, glycosylation, acetylation and oxidation, in particular, have been of great interest in this field as it has been demonstrated to be linked to disease pathology and useful targets for therapeutics. In addition to MS-based large-scale protein and peptide sequencing, other innovative approaches including self-assembling protein microarrays [28] and bead-based flow cytometry [29] to identify and quantify proteins and protein-protein interaction in a high throughput manner have furthered our understanding in the molecular mechanisms involved in diseases. In summary, clinical proteomics has come a long way in the past decade in terms of technology/platform development, protein chemistry, and bioinformatics to identify molecular signatures of diseases based on protein pathways and signaling cascades. Hence, it undoubtedly holds great promise for disease diagnosis, prognosis, and prediction of therapeutic outcome on an individualized basis. However, without proper study design and implementation of robust analytical techniques, the efforts and expectations to make biomarkers a useful reality in the near future can easily be hampered. This is clearly manifested by the stagnant rate of clearance or approval of protein biomarkers for all diseases by the Food and Drug Administration (FDA) in the US (i.e., averaging ~1.5 protein/yr in the past 15 yr [30]), in contrast to over 1,200 biomarker candidates reported in the scientific literature for cancer alone. The question arises as to what has caused such a huge disconnect between biomarker discovery using modern proteomic technologies and biomarker qualification requiring much more stringent analytical and clinical criteria. Several major barriers have been postulated to be responsible for this discrepancy, including: (1) technological variability within/across proteomic platforms; (2) improper biospecimen collection, handling, storage and processing; (3) incapability of credentialing biomarker candidates prior to costly and time-consuming clinical qualification studies using well-established methodologies; (4) a lack of knowledge in the evaluation criteria required for these distinct processes in the pipeline and in regulatory science by the research community; (5) insufficient publicly available high-quality reagents and data sets to the cancer research community; (6) need for improved data analysis tools for the analysis, characterization, and comparison of large datasets and multi-dimensional data; and (6) a lack of proper experimental study design when performing studies involving clinical samples in biomarker studies (Fig. 1). If proteomics is to successfully make its way into clinical diagnostics, universally accepted metrics will be needed at many steps along the way to ensure that observed changes are attributable to biological states, not workflow variability.
On the discovery front, semi-quantitative proteomic methodologies routinely used for biomarker research between normal and diseased states are differential two-dimensional gel electrophoresis (2DGE), comparative label-free and labeling approaches (e.g., Isotope Coded Affinity Tags, iTRAQ, Stable Isotope Labeling with Amino Acids in Cell Culture) followed by liquid chromatography mass spectrometry (LC-MS). Although such comparative analysis yields insightful information on possible changes as a result of disease, these current methods in clinical proteomics based, for the most part, on MS and its combination with 2DGE, chromatography or biobead technology have a concentration sensitivity level (CSL) not lower than 10 nM. This coupled to the use of blood as a biospecimen in discovery research (a commonly used biospecimen which is highly complex and has a wide dynamic range of protein concentrations), makes it is very difficult to discover (measure) low abundance proteins (potential biomarkers). One remedy to this problem is to develop and apply nanotechnology in clinical proteomics which can substantially enhance the CSL, as well as the throughput of analytical measurement systems while lowering their cost. Not only does nanotechnology have the potential of satisfying many criteria required for the advancement of clinical proteomics, essential changes in the physicochemical properties of substances on their conversion to the nanostructured state have also made it possible to create efficient systems for drug delivery to targets. Currently, one of the most promising nanotechnological proteomics being developed for medical research is biosensor-based nanodiagnostics. An example of this is the development of a magneto-nano sensor protein chip and a multiplex magnetic sorter based on magnetic nanoparticles that allow rapid conversion of discrete biomolecules binding events into electrical signals, which can detect target molecules down to the single molecule level in less than an hour [31].
In reality, proteomics has not lived up to the hopes for identifying effective biomarkers in the past decade, due in part to the lack of coherent pipeline connecting biomarker discovery efforts with well-established methods for clinical qualification studies (commonly known as biomarker validation). As a result, among the critical challenges facing the proteomics community is the lack of an ability to accurately and reproducibly measure a meaningful number of proteins in biospecimens across institutions. Better understanding of the challenges and strategies inherent in each phase of the proteomics pipeline is required to both accelerate the pace and quality of biomarker development and facilitate the delivery and deployment of novel clinical tests. Indeed, challenges in proteomics encompass several stages of the biomarker development pipeline, including a lack of technology standardization/optimization; quality affinity reagents; analytical validation review documents for developers of multiplex proteomics assays; and proper experimental design when performing studies involving clinical samples (e.g., statistical power calculation on the number of biospecimens needed to ensure meaningful results, and biospecimen quality with proper representation of patient population).
The conventional biomarker development pipeline involves a discovery stage followed by a qualification stage (commonly known as biomarker validation) on large cohorts (Fig. 2A), prior to clinical implementation. Traditionally, the discovery stage is performed on a MS-based platform for global unbiased sampling of the proteome, while biomarker qualification and clinical implementation generally involve the development of an antibody-based protocol, such as the widely used enzyme linked immunosorbent assays (ELISAs). Although this process has the potential to deliver clinically important biomarkers, it is not the most efficient as the latter is low-throughput, very costly and time-consuming. In many cases, affinity reagents for novel protein candidates do not even exist and it is difficult to multiplex targets without creating significant interferences and cross-reactivity. These limitations of immunoassays have incentivized the development of alternative approaches. The recent explosion in the advancement of proteomic technologies centering on targeted MS and protein microarrays has provided great opportunities for researchers to use them as "bridging technologies" for clinical proteomic investigation of disease-relevant changes in tissues and biofluids.
To address many of the critical challenges facing the protein biomarker community, the NCI launched the Clinical Proteomic Technologies for Cancer initiative (CPTC) in 2006 (http://proteomics.cancer.gov). The overall goals of CPTC during the first 5 yr were to focus on removing several of the major barriers in proteomics research to enable the accurate, efficient and reproducible identification, and quantification of meaningful numbers of proteins that could drive high value clinical biomarker qualification studies. Achieving this goal would provide a firm foundation for the field of discovery proteomics and enable the rational development of clinical biomarkers to address various needs in cancer drug development, diagnostics and clinical management.
Since its launch, CPTC has made significant progress in developing an accurate and quantitative biomarker assay workflow for proteomics, incorporating common technology standards, standard operating procedures (SOPs), data analysis standards, critically needed reagents (affinity and reference materials), and an open access proteomics database [32-37]. The new protein biomarker workflows developed by CPTC that incorporate go/no-go decision points, address the variability of methods and technologies-which enable researchers to accurately, reliably and quantitatively identify large numbers of proteins (see below). Consequently, CPTC has quickly evolved into a national (and international) community resource that links technologists with cancer biologists and clinicians to accelerate the development, improvement and standardization of proteomic technologies for the detection of cancer-relevant proteins/peptides in clinical biospecimens.
NCI-CPTC incorporated an intermediate "bridging" step called "biomarker verification" in its pipeline to efficiently translate proteomic discoveries into clinical qualification studies (Fig. 2B). In this context, biomarker verification is defined as the process of credentialing prioritized "biomarker candidates" using analytically robust, reproducible and quantitative multiplex assays on statistically powered number of samples with clinical relevance. Credentialed proteins successfully passing this stage of the pipeline are considered verified biomarkers of high value for translating into large-scale clinical qualification studies.
One of the main verification technologies currently being tested by CPTC is based on an existing technology, Multiple Reaction Monitoring Mass Spectrometry (MRM-MS), which has been used for decades in clinical reference laboratories to accurately measure small molecules in plasma, such as drug metabolites [38, 39]. MRM-MS has only recently proven suitable for use in pre-clinical studies to rapidly screen and measure large numbers of candidate proteins in complex patient samples necessary for biomarker verification [40-43]. MRM-MS provides a rapid way to measure the abundance of a particular candidate(s) and determine whether changes in abundance correspond to the presence or stage of a disease. Prior to applying MRM-MS based assays on clinical biospecimen, precursor peptide ions are selected from previous experiments combining empirical MS data, database searches of peptide libraries, and software predictions to develop the best peptide candidates to monitor. As illustrated in Fig. 3, the general components of MRM-MS based approaches for targeted protein quantitation encompass three quadrupoles (QQQ) for enhanced sensitivity and selectivity on a triple quadrupole mass spectrometer. The first quadrupole (Q1) is designed to transmit only the selected precursor peptide ion (m/z) into the second quadrupole (Q2) where collisionally-induced dissociation (CID) occurs to generate a signature fragment ion of particular m/z value or several fragment ions allowed to be admitted into the third quadrupole (Q3) for monitoring. Consequently, peptide quantitation is based on measuring the intensity of the product ion(s) selected from Q3. MRM-MS can precisely detect a wide variety of peptides and proteins via CID or MS/MS. When coupled with chemically identical heavy isotope labeled internal standard peptides of known amounts (stable isotope dilution mass spectrometry), this approach has the potential benefits for accurate quantitation of target peptides that make it a desirable alternative to immunoassays including, but not limited to, shorter assay development timeline, lower cost, high multiplexing capability, potential for high specificity, inclusion of internal standards, and particular advantages for PTMs, mutations and other variants of a protein. On the other hand, there are potential drawbacks of MRM-MS assays which may include: the complex selection process for determining target precursor peptide ions to monitor, relatively low resolution of QQQ-MS (usually unit resolution) in resolving components in complex biofluids, and the possibility of interference from in-source fragmentation of abundant peptides that prevent analysis of desired precursor peptides. In terms of sensitivity, MRM-MS assays alone are at best in the range of µg per milliliter of biofluids. However, when coupled with immunoaffinity enrichment [i.e., Stable Isotope Standards and Capture by Anti-Peptide Antibodies (SISCAPA)] [44-46], it elevates the sensitivity of detection several orders of magnitude. With improvements in generating monoclonal antibodies against target signature peptides, instrument designs and workflow automation, MRM-MS may potentially provide a very reliable go/no-go decision point in the new CPTC biomarker development pipeline. The complement of a LC-MRM-MS assay is iMALDI (immuno matrix-assisted laser desorption ionization), where the beads with the affinity-bound peptides attached are placed directly on a MALDI mass spectrometer, and the MALDI matrix solvent elutes the peptides from the beads [47, 48]. The presence of the peptide, and its peak height or peak area, are then determined from an MS spectrum for quantitation in the MS mode, while peptide identities are confirmed with CID or MS/MS. In principle, iMALDI can be performed with only a MALDI-MS instrument, but it can also be used in the "MRM mode" on an MALDI-MS/MS mode.
To determine the reliability and transferability of this technology across instrument platforms and laboratories, the CPTC network conducted a first-of-its-kind seminal study to assess the reproducibility of this approach in 2009. CPTC data [34] shows that MRM-MS based platforms can consistently measure a number of candidate proteins across multiple laboratories sensitively and quantitatively in a high throughput fashion on instruments already deployed in clinical diagnostic laboratories. The collective results demonstrated the generation of reproducible MRM-MS data using 11 target peptides from 7 proteins (22 in total including their heavy isotope labeled internal standards) to construct linear concentration curves without affinity enrichment. The study has shown that even in the most complex scenario (study III) where multi-step sample preparation was individually performed at 8 sites, the highest CV was <23% using only a single transition of MRM (one fragment ion), except for one peptide (LEP-IND) whose CVs were consistently higher than others in all three studies. It is conceivable that analytical variability will further decrease with reduced or more streamlined sample preparation. Furthermore, automation with robotics in conjunction with software development should eventually reduce labor-intensive workflow, variability between different instrumentation platforms, and the need for high level of expertise currently required to perform this type of assays, while assay specificity will be improved by monitoring multiple transitions for a single peptide and multiple peptides (an average of 3-5) from the same protein and highly specific antibodies. Nevertheless, this inter-laboratory study represents the first critical step toward potential widespread implementation of assays for the pre-clinical verification of candidate biomarkers. Follow-up studies to this are ongoing which include targeting cancer-specific proteins, enhancing sensitivity, lowering the coefficients of variation (CV), increasing multiplex level, and assessing reproducibility across a larger network of institutions on more instrument platforms.
In addition to restructuring the biomarker development pipeline, it is critical to introduce regulatory science to the proteomics research and clinical chemistry community in order for this technology to be translated from the laboratory to the clinic. Navigating the regulatory process is daunting by itself, and this intensifies when new technologies are brought under review as the kind of proteomic technologies being used for biomarkers are relatively new to the US FDA, which creates uncertainty both on the part of researchers in how their findings should be presented in a submission to the agency, and on the part of the FDA in evaluating the data.
In the USA, device clearance or approval rests on the ability of the sponsor to provide analytical and clinical data that demonstrate that the device performance is adequate to meet its claimed intended use [49]. In case of novel markers, simple analytical detection or quantification of an analyte is inadequate. Significance of the measurement of novel markers for clinical management of the patient must also be demonstrated, either through clinical data, or in some cases, through sufficient credible published information that supports clinical use. A premarket submission document includes a device intended use/indication for use, a description of the device covering both the instrument and reagents, and analytical and clinical performance studies evaluating performance of the device for its intended use. The analytical performance of the device (i.e., test or assay) is described in terms of precision, accuracy and performance around the cut-off point, along with other performance measurements such as specificity, sensitivity, linearity, limit of detection and limit of quantitation, as required for any assays. A detailed description of appropriate internal and external controls and calibrators used in the assay should also be included in the submission. To complicate these even more, laboratory-developed tests (LDTs or "homebrew tests") currently exist for at least 96 protein analytes for which there is no FDA-approved test. LDTs have historically been a general subject of FDA enforcement discretion. However, the FDA's longstanding policy of enforcement discretion may undergo some major changes as witnessed by the FDA's public meeting on the oversight of LDTs [50] as proper clinical validation of multiplex proteomics-based tests used in a clinical setting might be beyond the scope of a single laboratory. Multiplex protein-based tests will likely require further simplification and analytical robustness in order to be used extensively in clinical applications.
Multiplex protein-based assays discussed here, defined as device/test systems where one or more protein/peptide targets are simultaneously detected via a common process of sample preparation, measurement, and interpretation, are intrinsically very complex. If multiple results from multiple measurements are subsequently interpreted via an in vitro Diagnostic Multivariate Index Assay (IVDMIA) software, which combines the values of multiple variables using an interpretation function to yield a single, patient-specific result (e.g., a classification, score, etc.) whose derivation is non-transparent and cannot be independently derived or verified by the end user, it becomes even more complicated for individual users to validate. A recent community-wide research effort extensively evaluated current practices on the development and validation of classifiers and composite scores using DNA microarray-based predictive models [51]. Furthermore, the FDA drafted a guidance document on IVDMIA devices [52]. An example of an IVDMIA using individually measured values of multiple proteins to derive a patient-specific score to help a physician evaluate the likelihood that an ovarian adnexal mass is malignant or benign prior to a planned surgery is the FDA-cleared OVA1 test developed by Vermillion, Inc. [53]. As the field continues to evolve, establishing a standardized evaluation paradigm for these types of complex tests involving measurements of multiple analytes and interpretive software should help ensure the highest level of performance within and across laboratories in order to provide the most accurate test results for patients. For a multiplex protein-based assay, analytical validation becomes much more challenging as the FDA requires that all analytes in the panel meet analytical performance criteria rather than extrapolating the performance of one analyte to all others. In particular, this type of assays should address cross-reactivity or interference of analytes within and outside the panel. Having realized the importance of this aspect for the greater research community, the NCI-CPTC collaborates with the FDA to understand likely analytical evaluation requirements for diagnostic assays based on these multiplex protein-based technologies to ensure the safety and effectiveness of these tests for their intended use. As a result, mock 510(k) premarket submissions based on targeted proteomic platforms of MRM-MS and multiplex immunological arrays were developed to educate the community on the regulatory processes in assay clearance/approval by the FDA [54, 55] (Fig. 4).
In practice, there are currently multiplex MRM-MS-based assays being used or under development in clinical laboratories. Thyroglobulin, for example, a serum biomarker already used (immunoassays) in conjunction with imaging to determine the clinical management of patients following treatment of thyroid carcinoma, represents one of the best-validated serum tumor markers in clinical chemistry [56, 57]. However, it is also an example of a biomarker whose clinical utility is limited in analytical methods due to interferences from nonspecific heterophilic interfering antibodies [58, 59] and thyroglobulin autoantibodies [60], etc. In order to circumvent these problems, an MRM-MS based assay in combination with immunoaffinity enrichment of 3 tryptic peptides of thyroglobulin has been developed as a LDT [61]. As a result, serum thyroglobulin was quantified based on the 3 endogenous peptide using LC-MRM-MS with external calibrators after extracting them from tryptic digests of human serum using polyclonal antibodies raised against them. It has been demonstrated that this approach has the ability to detect tryptic peptides of thyroglobulin at picomolar concentrations, while digesting the endogenous immunoglobulins that can potentially interfere with traditional immunoassays.
To meet FDA's requirements for analytical validation using multiplex MRM-MS technologies, three critical components of the assay configured for targeted analytes in the context of their intended use should be considered: (1) sample preparation leading to final analysis on a MS platform; (2) the instrument platform itself (i.e., QQQ-MS); (3) software (instrument control and result analysis). Firstly, sample preparation should address the need to include controls for assessing the efficiency and variability of proteolytic digestion of proteins in different samples because evidence for trypsin digestion variability in proteomic analysis exists and needs to be addressed [62]; and/or gauging analytical recovery of immunoaffinity-enriched proteins and PTMs during sample preparation [63] prior to being considered for use in the clinical setting. It is the reproducibility of the "overall measured protein concentration" calculated from target proteolytic peptides derived from the same protein that would need to be shown consistent from run to run. If one of the peptide measurements appears consistently as an outlier, assay developers need to understand why this occurred, what effect it would have on the assay, and whether the outlier and its effect would be recognized by the assay quality control (QC) system since an outlier could signal the presence of PTMs, or interferences from other proteins and single nucleotide polymorphisms (SNPs), etc.
Secondly, although MS for clinical use, as previously described under 21 CFR 862.2860, are considered Class I device (low risk usually requiring only general controls) and exempt from 510(k) process [64], FDA regulations differentiate between those MS instrumentation and more complex instrumentation such as those instruments that include interpretive software and complex measurement functions, which may be regulated similarly to the multiplex instrumentation for nucleic acid assays (Class II). Moreover, instrumentation used to run a specific assay takes on the classification of that assay when submitted for that particular intended use. For instance, a Class II assay based on MS analysis would involve evaluation of that MS as a part of a Class II assay for that intended use. Although basic operating principles are the same, modern instrument design from different manufacturers and interfaced HPLC systems (nano-scale vs. micro-scale flow rates, chip vs. non-chip-based) could be different, potentially causing non-equivalent performance of assays on different instruments, and thus requiring different specifications for each instrument. In light of this, the NCI-CPTC network conducted an interlaboratory study, as previously described, which showed promise in obtaining reproducible MRM-MS data across instrument platforms and laboratories [32]. Once analytical performance would be demonstrated as equivalent on different MS platforms, separate regulatory evaluations of the same analyte(s) on each instrument may become simplified or unnecessary. For FDA submission, if performance on a specific MS instrument/platform as a part of a diagnostic assay is adequate, it generally leads to an approval of an assay only on that specific instrument used in evaluating performance. This can be followed by subsequent submissions addressing any modifications to the assay, such as addition of another instrument platform. For example, the most commonly used QQQ-MS could be cleared as a part of an MRM-MS assay by measuring a protein analyte with relatively low-risk intended use, which would make this instrument manufactured under quality system (Good Manufacturing Practice [GMP]) available for clinical laboratory testing, and can be followed by addition of other QQQ-MS manufacturers on the same analyte. The evaluation of instrument platform, however, should include all necessary components and accessories (e.g., HPLC columns and tubings, electrospray source, software). Any changes to this cleared platform would be re-evaluated by the assay manufacturer, and may or may not need subsequent regulatory submissions to address the safety and effectiveness of the changed platform. Additional analytes or kits can subsequently be cleared for use on the same platform without additional platform-specific information, other than assay-specific components of the instrumentation.
Finally, pre-defining certain parameters in software packages on instruments (e.g., MS) and manufacturing instruments under GMP guidelines should reduce operator-specific bias, requirements for extensive specialized training and analytical variability at the platform level. If interpretive IVDMIA software to reach a patient-specific result from multiple measurements is used, the FDA generally requires that software algorithms included in the assay for data and results interpretation be pre-specified before analyzing study data. Alteration of the algorithm to better fit the data after the study is performed is generally unacceptable [54].
Clinical chemists undoubtedly play a huge role in the analytical validation of diagnostic tests and are thus required to routinely verify (confirm) previously cleared/approved tests by the regulatory agency in their facilities. Post-market analytical validation is routinely performed by clinical chemists as the QC process to evaluate whether or not a previously cleared test (instrument, controls, reagents, etc.) complies with regulations, specifications, or conditions. These QC studies typically involve precision, accuracy, linearity and lower limit of detection and quantitation. When clinical chemists set up a method for an approved multiplex protein assay using a patient-specific "score", they should consider how to perform studies to validate the score. One approach may involve running adequate number of positive and negative patients to assess the performance of such a "score" in their diagnosis when compared to their medical charts and final clinical diagnosis. In the USA, even though clinical labs are not necessarily responsible for clinical validation of tests, the lab director should consider clinical validity when selecting a test for patients.
Additionally, international collaborative efforts provide an effective means to educate key clinical laboratory audiences on the need for and use of common technologies and standards in proteomic workflows and to share knowledge and experience on commonly interesting targets, assays and new technologies.
Clinical Laboratory Standards Institutes (CLSI) documents are useful to assay sponsors and the regulatory agency in the process of preparing and reviewing premarket submissions, and are highly regarded by other organizations of clinical professionals. CLSI aims to develop global consensus standards and guidelines for healthcare testing (industry, government, and professional) [65]. A CLSI document goes through rounds of rigorous review prior to publication. These documents are developed and approved by consensus of stakeholders in particular areas, which may include FDA representatives, and go through a public comment phase. The FDA can either fully or partially recognize CLSI documents as standards, and compliance with the recommendations of CLSI documents may be accepted as evidence of fulfillment of certain FDA analytical requirements. EP-17A, Vol. 24, No. 34, Protocols for Determination of Limits of Detection and Limits of Quantitation; Approved Guideline, for example, is a commonly referenced document for analytical performance of a test. While CLSI documents on multiplex proteomics assays currently do not exist, general guidance could be drawn from the nucleic acid-based multiplex world. An example of that is MM-17A (Verification and Validation of Multiplex Nucleic Acid Assays; Approved Guideline).
The mapping of the human genome represents a true milestone in medicine and has led to an explosion in discoveries and translative research in life sciences. Indeed, this important knowledge base has enabled rapid development in the areas of diagnostics, gene therapy, new drug targets discovery, and personalized therapies [66, 67]. The expansion of biological knowledge through the Human Genome Project (HGP) has also been accompanied by the development of new high throughput techniques, providing extensive capabilities for the analysis of a large number of genes or the whole genome, for example, the development of multiplex cytogenetic arrays for the detection of copy number variations (CNVs) and SNPs. The completion of the human genome, however, has presented a new and even more challenging task for scientists: the characterization of the human proteome. Unlike the genome project, there are major challenges in defining a comprehensive Human Proteome Project (HPP) due to (1) potentially very large number of proteins with PTMs, mutations, splice variants, etc.; (2) the diversity of technology platforms involved; (3) the variety of overlapping biological "units" into which the proteome might be divided for organized conquest; and (4) sensitivity limitations in detecting proteins present in low abundances.
The ultimate goal for translational medicine is to be able to perform assays in various clinical samples at multiple levels: DNA (genome), RNA (transcriptome) and protein (proteome) using the knowledge and technologies coming out of these large-scale projects correlative to a specific phenotype. Currently, large-scale multidisciplinary team science based initiatives, such as The Cancer Genome Atlas (TCGA) and The International Cancer Genome Consortium (ICGC), are characterizing diseased cancer genomes of tumor tissues to understand different cancers at a genetic level. As a result, genetic alterations associated with cancers including copy number aberration, mutation, microdeletion and others have been generated by multidimensional data sets and high level integrative analysis [68, 69]. This information now provides a genetic basis and a great opportunity for the community to characterize and quantify proteins (reflecting genetic alterations if detectable) and their alterations and PTMs in the cell. The resulting proteomic evidence will corroborate or complement the genetic aberrations detected in these tumors, providing deeper understanding of cancer and other diseases in the context of biology and clinical utility. For instance, the integrative analysis of DNA copy number, gene expression and DNA methylation aberrations in 206 glioblastomas (GBM), the most common form of adult brain cancer, as characterized by the TCGA, revealed the roles of ERBB2, NF1 and TP53, and frequent mutations of the phosphatidylinositol-3-OH kinase regulatory subunit gene PIK3R1, as well as providing a network view of the pathways altered in the development of glioblastoma [70]. In addition, integration of mutation, DNA methylation and clinical treatment data showed a link between O6-methylguanine-DNA methyltransferase (MGMT) promoter methylation and a hypermutator phenotype consequent to mismatch repair deficiency in treated glioblastomas, an observation with potential clinical implications. Another study performed by the TCGA network described a robust gene expression-based molecular classification of GBM into Proneural, Neural, Classical, and Mesenchymal subtypes as defined by aberrations and gene expression of EGFR (Classical), NF1 (Mesenchymal), and PDGFRA/IDH1 (Proneural) [71]. Interestingly, response to aggressive therapy differs by subtype, with the greatest benefit in the Classical subtype and no benefit in the Proneural subtype. This finding potentially provides a framework that unifies transcriptomic and genomic dimensions for GBM molecular stratification, and if corroborated at the protein level, it would vastly expand our knowledge on GBM and ultimately improve the clinical practice of medicine for GBM patients. By leveraging the advances in reproducibility and transferability of clinical proteomic platforms from the first 4.5 yr of the NCI-CPTC initiative to genomic evidence provided by TCGA and ICGC, for example, will provide a rational pathway for analytically verified biomarker candidates (Fig. 5). In this envisioned route, high throughput proteomics will analyze statistically-powered unbiased biospecimens (tissues, proximal fluids, and blood) to identify and quantify proteins as the power of statistics in biomarker research has long been ignored, contributing to the failure of qualifying new protein biomarkers by the FDA at a reasonable rate. The integration and interrogation of the proteomic and genomic data will provide potential biomarker candidates which will be prioritized for downstream targeted proteomic analysis. These biomarker targets will be used to create multiplex, quantitative assays for verification and prescreening to test the relevance of the targets in clinically relevant and unbiased samples. The outcomes from this approach will provide the community with verified biomarkers which could be used for clinical qualification studies; high quality and publicly accessible datasets; and analytically validated, multiplex, quantitative protein/peptide assays and their associated high quality reagents for the research and clinical community.
Beyond the integration of "-omics" data and information, future proteomic endeavors should continue to support technology development, optimization and standardization. Incorporation of the most up-to-date and efficient technologies is critical in successfully propelling the translation of proteomic findings into clinically relevant biomarkers. Meanwhile, rigorous assessment of biospecimen and data quality through quality assessment (QA)/QC criteria at each step of the biomarker development pipeline should continue to be supported to make "go" or "no-go" decisions. These efforts, combined with continued collaborations with regulatory agencies and clinical chemists, will expedite the development of individualized patient care through clinical proteomics.
Acknowledgements
Certain commercial equipment, instruments, or materials are identified in this paper to specify adequately the experimental detail and procedure. Such identification does not imply recommendation or endorsement by the National Cancer Institute, National Institutes of Health, nor does it imply that the materials or equipment identified are necessarily the best available for the purpose.
References
1. Etzioni R, Urban N, Ramsey S, McIntosh M, Schwartz S, Reid B, et al. The case for early detection. Nat Rev Cancer. 2003; 3:243–252. PMID: 12671663.


2. Anderson L. Candidate-based proteomics in the search for biomarkers of cardiovascular disease. J Physiol. 2005; 563:23–60. PMID: 15611012.


3. Rifai N, Gillette MA, Carr SA. Protein biomarker discovery and validation: the long and uncertain path to clinical utility. Nat Biotechnol. 2006; 24:971–983. PMID: 16900146.


4. García-Foncillas J, Bandrés E, Zárate R, Remírez N. Proteomic analysis in cancer research: potential application in clinical use. Clin Transl Oncol. 2006; 8:250–261. PMID: 16648100.


5. Bouchal P, Roumeliotis T, Hrstka R, Nenutil R, Vojtesek B, Garbis SD. Biomarker discovery in low-grade breast cancer using isobaric stable isotope tags and two-dimensional liquid chromatography-tandem mass spectrometry (iTRAQ-2DLC-MS/MS) based quantitative proteomic analysis. J Proteome Res. 2009; 8:362–373. PMID: 19053527.


6. Wiener MC, Sachs JR, Deyanova EG, Yates NA. Differential mass spectrometry: a label-free LC-MS method for finding significant differences in complex peptide and protein mixtures. Anal Chem. 2004; 76:6085–6096. PMID: 15481957.


7. Geiger T, Cox J, Ostasiewicz P, Wisniewski JR, Mann M. Super-SILAC mix for quantitative proteomics of human tumor tissue. Nat Methods. 2010; 7:383–385. PMID: 20364148.


8. Anderson L, Hunter CL. Quantitative mass spectrometric multiple reaction monitoring assays for major plasma proteins. Mol Cell Proteomics. 2006; 5:573–588. PMID: 16332733.


9. Wang H, Wong CH, Chin A, Kennedy J, Zhang Q, Hanash S. Quantitative serum proteomics using dual stable isotope coding and nano LC-MS/MSMS. J Proteome Res. 2009; 8:5412–5422. PMID: 19817497.


10. Lee J, Soper SA, Murray KK. Microfluidic chips for mass spectrometry-based proteomics. J Mass Spectrom. 2009; 44:579–593. PMID: 19373851.


11. Pierobon M, Calvert V, Belluco C, Garaci E, Deng J, Lise M, et al. Multiplexed cell signaling analysis of metastatic and nonmetastatic colorectal cancer reveals COX2-EGFR signaling activation as a potential prognostic pathway biomarker. Clin Colorectal Cancer. 2009; 8:110–117.


12. Ramachandran N, Raphael JV, Hainsworth E, Demirkan G, Fuentes MG, Rolfs A, et al. Next-generation high-density self-assembling functional protein arrays. Nat Methods. 2008; 5:535–538. PMID: 18469824.


13. Beirne P, Pantelidis P, Charles P, Wells AU, Abraham DJ, Denton CP, et al. Multiplex immune serum biomarker profiling in sarcoidosis and systemic sclerosis. Eur Respir J. 2009; 34:1376–1382. PMID: 19541722.


14. Kelleher MT, Fruhwirth G, Patel G, Ofo E, Festy F, Barber PR, et al. The potential of optical proteomic technologies to individualize prognosis and guide rational treatment for cancer patients. Target Oncol. 2009; 4:235–252. PMID: 19756916.


15. Wang P, Whiteaker JR, Paulovich AG. The evolving role of mass spectrometry in cancer biomarker discovery. Cancer Biol Ther. 2009; 8:1083–1094. PMID: 19502776.


16. Whiteaker JR, Zhang H, Eng JK, Fang R, Piening BD, Feng LC, et al. Head-to-head comparison of serum fractionation techniques. J Proteome Res. 2007; 6:828–836. PMID: 17269739.


17. Ernoult E, Bourreau A, Gamelin E, Guette C. A proteomic approach for plasma biomarker discovery with iTRAQ labeling and OFFGEL fractionation. J Biomed Biotechnol. 2010; 2010:927917. PMID: 19888438.
18. Nirmalan NJ, Hughes C, Peng J, McKenna T, Langridge J, Cairns DA, et al. Initial development and validation of a novel extraction method for quantitative mining of the formalin-fixed, paraffin-embedded tissue proteome for biomarker investigations. J Proteome Res. 2010; 10:896–906. PMID: 21117664.


19. Krishhan VV, Khan IH, Luciw PA. Multiplexed microbead immunoassays by flow cytometry for molecular profiling: basic concepts and proteomics applications. Crit Rev Biotechnol. 2009; 29:29–43. PMID: 19514901.


20. Cha S, Imielinski MB, Rejtar T, Richardson EA, Thakur D, Sgroi DC, et al. In situ proteomic analysis of human breast cancer epithelial cells using laser capture microdissection: annotation by protein set enrichment analysis and gene ontology. Mol Cell Proteomics. 2010; 9:2529–2544. PMID: 20739354.


21. Anderson KS, Sibani S, Wallstrom G, Qiu J, Mendoza EA, Raphael J, et al. Protein microarray signature of autoantibody biomarkers for the early detection of breast cancer. J Proteome Res. 2011; 10:85–96. PMID: 20977275.


22. Bateman NW, Sun M, Hood BL, Flint MS, Conrads TP. Defining central themes in breast cancer biology by differential proteomics: conserved regulation of cell spreading and focal adhesion kinase. J Proteome Res. 2010; 9:5311–5324. PMID: 20681588.


23. Kristiansen TZ, Harsha HC, Grønborg M, Maitra A, Pandey A. Differential membrane proteomics using 18O-labeling to identify biomarkers for cholangiocarcinoma. J Proteome Res. 2008; 7:4670–4677. PMID: 18839982.
24. An HJ, Lebrilla CB. A glycomics approach to the discovery of potential cancer biomarkers. Methods Mol Biol. 2010; 600:199–213. PMID: 19882130.


25. Choudhary C, Mann M. Decoding signalling networks by mass spectrometry-based proteomics. Nat Rev Mol Cell Biol. 2010; 11:427–439. PMID: 20461098.


26. Madian AG, Regnier FE. Profiling carbonylated proteins in human plasma. J Proteome Res. 2010; 9:1330–1343. PMID: 20121119.


27. Iwabata H, Yoshida M, Komatsu Y. Proteomic analysis of organ-specific post-translational lysine-acetylation and -methylation in mice by use of anti-acetyllysine and -methyllysine mouse monoclonal antibodies. Proteomics. 2005; 5:4653–4664. PMID: 16247734.


28. Ceroni A, Sibani S, Baiker A, Pothineni VR, Bailer SM, LaBaer J, et al. Systematic analysis of the IgG antibody immune response against varicella zoster virus (VZV) using a self-assembled protein microarray. Mol Biosyst. 2010; 6:1604–1610. PMID: 20514382.


29. Wong J, Sibani S, Lokko NN, LaBaer J, Anderson KS. Rapid detection of antibodies in sera using multiplexed self-assembling bead arrays. J Immunol Methods. 2009; 350:171–182. PMID: 19732778.


30. Anderson NL. The clinical plasma proteome: a survey of clinical assays for proteins in plasma and serum. Clin Chem. 2010; 56:177–185. PMID: 19884488.


31. Osterfeld SJ, Yu H, Gaster RS, Caramuta S, Xu L, Han SJ, et al. Multiplex protein assays based on real-time magnetic nanotag sensing. Proc Natl Acad Sci USA. 2008; 105:20637–20640. PMID: 19074273.


32. Paulovich AG, Billheimer D, Ham AJ, Vega-Montoto L, Rudnick PA, Tabb DL, et al. Interlaboratory study characterizing a yeast performance standard for benchmarking LC-MS platform performance. Mol Cell Proteomics. 2010; 9:242–254. PMID: 19858499.


33. Rudnick PA, Clauser KR, Kilpatrick LE, Tchekhovskoi DV, Neta P, Blonder N, et al. Performance metrics for liquid chromatography-tandem mass spectrometry systems in proteomics analyses. Mol Cell Proteomics. 2010; 9:225–241. PMID: 19837981.


34. Addona TA, Abbatiello SE, Schilling B, Skates SJ, Mani DR, Bunk DM, et al. Multi-site assessment of the precision and reproducibility of multiple reaction monitoring-based measurements of proteins in plasma. Nat Biotechnol. 2009; 27:633–641. PMID: 19561596.


35. Tabb DL, Vega-Montoto L, Rudnick PA, Variyath AM, Ham AJ, Bunk DM, et al. Repeatability and reproducibility in proteomic identifications by liquid chromatography-tandem mass spectrometry. J Proteome Res. 2010; 9:761–776. PMID: 19921851.


36. Rodriguez H, Snyder M, Uhlén M, Andrews P, Beavis R, Borchers C, et al. Recommendations from the 2008 international summit on proteomics data release and sharing policy: the amsterdam principles. J Proteome Res. 2009; 8:3689–3692. PMID: 19344107.


37. Gloriam DE, Orchard S, Bertinetti D, Björling E, Bongcam-Rudloff E, Borrebaeck CA, et al. A community standard format for the representation of protein affinity reagents. Mol Cell Proteomics. 2010; 9:1–10. PMID: 19674966.


38. Xu X, Zhang J, Zhang L, Liu W, Weisel CP. Selective detection of monohydroxy metabolites of polycyclic aromatic hydrocarbons in urine using liquid chromatography/triple quadrupole tandem mass spectrometry. Rapid Commun Mass Spectrom. 2004; 18:2299–2308. PMID: 15384151.


39. Xu X, Roman JM, Issaq HJ, Keefer LK, Veenstra TD, Ziegler RG. Quantitative measurement of endogenous estrogens and estrogen metabolites in human serum by liquid chromatography-tandem mass spectrometry. Anal Chem. 2007; 79:7813–7821. PMID: 17848096.


40. James A, Jorgensen C. Basic design of MRM assays for peptide quantification. Methods Mol Biol. 2010; 658:167–185. PMID: 20839104.


41. Kiyonami R, Schoen A, Prakash A, Peterman S, Zabrouskov V, Picotti P, et al. Increased selectivity, analytical precision, and throughput in targeted proteomics. Mol Cell Proteomics. 2011; 10:M110.002931. PMID: 20664071.


42. Gerszten RE, Carr SA, Sabatine M. Integration of proteomic-based tools for improved biomarkers of myocardial injury. Clin Chem. 2010; 56:194–201. PMID: 20022985.


43. Kuhn E, Wu J, Karl J, Liao H, Zolg W, Guild B. Quantification of C-reactive protein in the serum of patients with rheumatoid arthritis using multiple reaction monitoring mass spectrometry and 13C-labeled peptide standards. Proteomics. 2004; 4:1175–1186. PMID: 15048997.
44. Kuhn E, Addona T, Keshishian H, Burgess M, Mani DR, Lee RT, et al. Developing multiplexed assays for troponin I and interleukin-33 in plasma by peptide immunoaffinity enrichment and targeted mass spectrometry. Clin Chem. 2009; 55:1108–1117. PMID: 19372185.


45. Anderson NL, Anderson NG, Haines LR, Hardie DB, Olafson RW, Pearson TW. Mass spectrometric quantitation of peptides and proteins using stable isotope standards and capture by anti-peptide antibodies (SISCAPA). J Proteome Res. 2004; 3:235–244. PMID: 15113099.


46. Ahn YH, Lee JY, Lee JY, Kim YS, Ko JH, Yoo JS. Quantitative analysis of an aberrant glycoform of TIMP1 from colon cancer serum by L-PHA-enrichment and SISCAPA with MRM mass spectrometry. J Proteome Res. 2009; 8:4216–4224. PMID: 19645485.


47. Reid JD, Holmes DT, Mason DR, Shah B, Borchers CH. Towards the development of an immuno MALDI (iMALDI) mass spectrometry assay for the diagnosis of hypertension. J Am Soc Mass Spectrom. 2010; 21:1680–1686. PMID: 20199871.


48. Jiang J, Parker CE, Fuller JR, Kawula TH, Borchers CH. An immunoaffinity tandem mass spectrometry (iMALDI) assay for detection of Francisella tularensis. Anal Chim Acta. 2007; 605:70–79. PMID: 18022413.


49. Mansfield E, O'Leary TJ, Gutman SI. Food and drug administration regulation of in vitro diagnostic devices. J Mol Diagn. 2005; 7:2–7. PMID: 15681468.


50. FDA/CDRH Public Meeting: oversight of Laboratory Developed Tests (LDTs), July 19-20, 2010. U.S. Food and Drug Administration (FDA). Updated on Sep 2010. http://www.fda.gov/MedicalDevices/NewsEvents/WorkshopsConferences/ucm212830.htm.
51. Shi L, Campbell G, Jones WD, Campagne F, Wen Z, Walker SJ, et al. The MicroArray Quality Control (MAQC)-II study of common practices for the development and validation of microarray-based predictive models. Nat Biotechnol. 2010; 28:827–838. PMID: 20676074.


52. Draft guidance for industry, clinical laboratories, and FDA staff: in vitro diagnostic multivariate index assays. Center for Devices and Radiological Health (CDRH). Updated on Jul 2007. http://www.fda.gov/downloads/MedicalDevices/DeviceRegulationandGuidance/GuidanceDocuments/ucm071455.pdf.
53. 510(k) Substantial equivalence determination decision summary. U.S. Food and drug administration. Updated on Mar 2011. http://www.accessdata.fda.gov/cdrh_docs/reviews/K081754.pdf.
54. Rodriguez H, Tezak Z, Mesri M, Carr SA, Liebler DC, Fisher SJ, et al. Analytical validation of protein-based multiplex assays: a workshop report by the NCI-FDA interagency oncology task force on molecular diagnostics. Clin Chem. 2010; 56:237–243. PMID: 20007859.


55. Regnier FE, Skates SJ, Mesri M, Rodriguez H, Tezak Z, Kondratovich MV, et al. Protein-based multiplex assays: mock presubmissions to the US food and drug administration. Clin Chem. 2010; 56:165–171. PMID: 20007858.


56. Pacini F. Follow-up of differentiated thyroid cancer. Eur J Nucl Med Mol Imaging. 2002; 29(S2):S492–S496. PMID: 12192551.


57. Saghari M, Gholamrezanezhad A, Mirpour S, Eftekhari M, Takavar A, Fard-Esfahani A, et al. Efficacy of radioiodine therapy in the treatment of elevated serum thyroglobulin in patients with differentiated thyroid carcinoma and negative whole-body iodine scan. Nucl Med Commun. 2006; 27:567–572. PMID: 16794517.


58. Spencer CA. Recoveries cannot be used to authenticate thyroglobulin (Tg) measurements when sera contain Tg autoantibodies. Clin Chem. 1996; 42:661–663. PMID: 8653888.


59. Spencer CA. Challenges of serum thyroglobulin (Tg) measurement in the presence of Tg autoantibodies. J Clin Endocrinol Metab. 2004; 89:3702–3704. PMID: 15292292.


60. Preissner CM, O'Kane DJ, Singh RJ, Morris JC, Grebe SK. Phantoms in the assay tube: heterophile antibody interferences in serum thyroglobulin assays. J Clin Endocrinol Metab. 2003; 88:3069–3074. PMID: 12843145.


61. Hoofnagle AN, Becker JO, Wener MH, Heinecke JW. Quantification of thyroglobulin, a low-abundance serum protein, by immunoaffinity peptide enrichment and tandem mass spectrometry. Clin Chem. 2008; 54:1796–1804. PMID: 18801935.


62. Hoofnagle AN. Peptide lost and found: internal standards and the mass spectrometric quantification of peptides. Clin Chem. 2010; 56:1515–1517. PMID: 20739635.


63. Ciccimaro E, Hanks SK, Yu KH, Blair IA. Absolute quantification of phosphorylation on the kinase activation loop of cellular focal adhesion kinase by stable isotope dilution liquid chromatography/mass spectrometry. Anal Chem. 2009; 81:3304–3313. PMID: 19354260.


64. CFR-Code federal regulations title 21. U.S. Food and Drug Administration (FDA). Updated on Apr 2010. http://www.accessdata.fda.gov/scripts/cdrh/cfdocs/cfcfr/CFRSearch.cfm?fr=862.2860.
65. Clinical and Laboratory Standards Institute (CLSI). Updated on Feb 2011. http://www.clsi.org.
66. Spitz MR, Bondy ML. The evolving discipline of molecular epidemiology of cancer. Carcinogenesis. 2010; 31:127–134. PMID: 20022891.


67. Rosa DD, Ismael G, Lago LD, Awada A. Molecular-targeted therapies: lessons from years of clinical development. Cancer Treat Rev. 2008; 34:61–80. PMID: 17826917.


68. Bredel M, Scholtens DM, Harsh GR, Bredel C, Chandler JP, Renfrow JJ, et al. A network model of a cooperative genetic landscape in brain tumors. JAMA. 2009; 302:261–275. PMID: 19602686.


69. International Cancer Genome Consortium. International network of cancer genome projects. Nature. 2010; 464:993–998. PMID: 20393554.
70. Cancer Genome Atlas Research Network. Comprehensive genomic characterization defines human glioblastoma genes and core pathways. Nature. 2008; 455:1061–1068. PMID: 18772890.
71. Verhaak RG, Hoadley KA, Purdom E, Wang V, Qi Y, Wilkerson MD, et al. Integrated genomic analysis identifies clinically relevant subtypes of glioblastoma characterized by abnormalities in PDGFRA, IDH1, EGFR, and NF1. Cancer Cell. 2010; 17:98–110. PMID: 20129251.


Fig. 2
The envisioned National Cancer Institute-Clinical Proteomic Technologies for Cancer initiative (NCI-CPTC) development pipeline from discovery to qualification. (A) The gap in the current proteomics research pipeline. (B) The incorporation of verification into the NCI-CPTC pipeline between discovery and qualification.
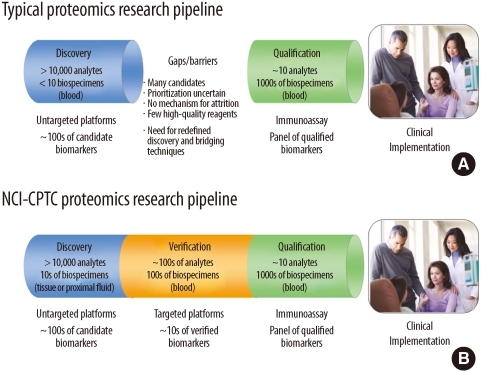
Fig. 3
Multiple Reaction Monitoring Mass Spectrometry (MRM-MS). A schematic of a triple quadrupole mass spectrometer (QQQ-MS) commonly used in MRM-MS analysis: Q1 and Q3 represent two mass filters for precursor and fragment ion selection while Q2 (collision cell) creates fragment ions via collisionally-induced dissociation (CID). In this case, one of the three peptide precursor ions (colored in blue) is selected in Q1, fragmented in Q2 and quantitated using one of its fragment ions (transition) selected in Q3 by the relative intensity of its peak area. An MRM-MS assay offers multiplexing capability of many target analytes in a single HPLC run.
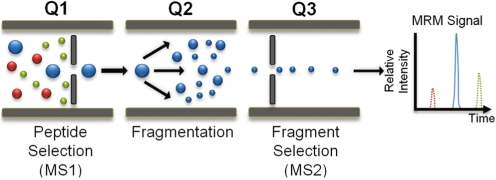