Abstract
Objective
To compare a high acceleration three-dimensional (3D) T1-weighted gradient-recalled-echo (GRE) sequence using the combined compressed sensing (CS)-sensitivity encoding (SENSE) method with a conventional 3D GRE sequence using SENSE, with respect to image quality and detectability of solid focal liver lesions (FLLs) in the hepatobiliary phase (HBP) of gadoxetic acid-enhanced liver MRI.
Materials and Methods
A total of 217 patients with gadoxetic acid-enhanced liver MRI at 3T (54 in the preliminary study and 163 in the main study) were retrospectively included. In the main study, HBP imaging was done twice using the standard mDixon-3D-GRE technique with SENSE (acceleration factor [AF]: 2.8, standard mDixon-GRE) and the high acceleration mDixon-3D GRE technique using the combined CS-SENSE technique (CS-SENSE mDixon-GRE). Two abdominal radiologists assessed the two MRI data sets for image quality in consensus. Three other abdominal radiologists independently assessed the diagnostic performance of each data set and its ability to detect solid FLLs in 117 patients with 193 solid nodules and compared them using jackknife alternative free-response receiver operating characteristics (JAFROC).
Results
There was no significant difference in the overall image quality. CS-SENSE mDixon-GRE showed higher image noise, but lesser motion artifact levels compared with the standard mDixon-GRE (all p < 0.05). In terms of lesion detection, reader-averaged figures-of-merit estimated with JAFROC was 0.918 for standard mDixon-GRE, and 0.953 for CS-SENSE mDixon-GRE (p = 0.142). The non-inferiority of CS-SENSE mDixon-GRE over standard mDixon-GRE was confirmed (difference: 0.064 [−0.012, 0.081]).
With recent technological developments in MRI including faster scanning acquisitions and new MRI contrast agents (1234), liver MRI is now widely utilized as a primary diagnostic test for the evaluation of malignant liver tumors (5678). However, liver MRI has its inherent problems of low speed, when compared to multidetector CT, resulting in image blurring with decreased spatiotemporal resolution and motion-related artifacts (91011). Various research efforts have been directed towards finding ways to reduce the acquisition time of MR images, including partial k-space sampling (12), parallel imaging (PI) such as sensitivity encoding (SENSE) (1314), and compressed sensing (CS) (101516). Indeed, PI techniques using the inherent spatial sensitivity of the phased-array coils, like the generalized autocalibrating partially parallel acquisition (GRAPPA), SENSE, and controlled aliasing in parallel imaging results in higher acceleration (CAIPIRINHA), have successfully demonstrated improved image quality while maintaining or even shortening acquisition times, making their way into the mainstream of clinical imaging (91718). However, scan time reduction using these techniques has some limitations including phase errors, noise, and imperfection in the coils, especially when PI acceleration factors (AF) > 3 are applied. Therefore, although PI with AF > 3–4 is feasible for recent scanners, three-dimensional (3D) T1-weighted (T1W) images may show unacceptable image degradation (13192021).
In this regard, CS may hold some answers. CS is a relatively new concept in MRI acquisition derived from a mathematical idea, from the approximation and information theories (222324). As maximum amplitudes and slew-rate of gradients are limited in MR acquisition due to the possibility of peripheral nerve stimulation (24), reduction of the acquisition time should be achieved by curtailing redundancies in MRI data (25). The CS technique focuses on the sparsity of images in a certain domain and attempts to avoid under-sampling artifacts using incoherent sampling with nonlinear reconstruction (222426). Moreover, CS-MRI offers a synergistic enhancement to PI with built-in noise reduction. Combining the CS and PI techniques has been shown to provide better-reconstructed images than PI and partial-Fourier techniques, enabling highly accelerated imaging (9152027). However, despite the recently rising interest in CS-MRI among researchers and clinicians for rapid body MRI, CS-MRI is still in its infancy, and there have only been a few reports focused on accelerated 3D T1W imaging, mainly on perfusion imaging using non-cartesian trajectories or pediatric imaging (92628).
Therefore, the purpose of this study was to determine if the CS-SENSE mDixon-3D gradient-recalled-echo (GRE) technique can offer adequate image quality and detectability of solid focal liver lesions (FLLs) in the hepatobiliary phase (HBP) of gadoxetic acid-enhanced liver MRI, when compared with the standard mDixon-3D GRE technique. The HBP sequence was selected not only due to its importance in liver imaging but also because relatively high, homogeneous and prolonged enhancement of liver parenchyma can be achieved during the phase (29303132).
The Institutional Review Board of our hospital approved this retrospective study and the requirement for informed consent was waived. Using a computerized search for MRI images obtained between June 2016 and March 2017 at our institution, 217 consecutive patients who underwent gadoxetic acid-enhanced liver MRI including HBP mDixon-3D GRE using a 3T scanner (Ingenia CX, Philips Healthcare, Best, the Netherlands) were included. Among them, during the initial parameter-optimization period of CS 3D GRE sequence, 54 consecutive patients underwent four different HBP protocols. After that, according to the results of preliminary optimization study, the following 163 consecutive patients underwent two HBP protocols including the HBP mDixon-3D GRE images using the standard two-dimensional (2D) SENSE technique or CS technique (Fig. 1). Primary indications for liver MRI were either for characterization of FLLs detected from ultrasonography or CT, or for the detection of FLLs when suspecting HCC or liver metastases. In total, 54 patients with a mean age ± SD of 60.9 ± 10.8 years (35 men [mean age, 62.6 ± 9.0 years; age range, 46–78] and 19 women [57.7 ± 13.3 years; 35–81]) were included in the preliminary study and 163 patients with a mean age ± SD of 61.8 ± 11.2 years (113 men [69.9 ± 11.3 years; 25–87] and 50 women [63.8 ± 11.1 years; 25–82]) were included in the main study.
In all patients, MRI was performed using a 3T scanner (Ingenia CX, Philips Healthcare) equipped with multi-transmit RF excitation technology (3334) and a 16-element anterior coil accompanied by a built-in posterior coil. Routine liver MR sequences are described in the Supplementary Materials (in the online-only Data Supplement).
For parameter optimization, 54 patients underwent four different HBP protocols including two HBP mDixon-3D GRE scans using the CS-SENSE and two HBP mDixon-3D GRE scans with the 2D SENSE technique. Detailed MR parameters used in the preliminary study and the results are provided in the Supplementary Materials and Supplementary Tables 1 and 2 (in the online-only Data Supplement).
According to the results from preliminary study, for the main study, HBP images were also obtained in the axial plane at 20 minutes after beginning the contrast medium injection using two kinds of T1W image sets: 1) standard mDixon-3D GRE with an AF of 2.8; and 2) CS-mDixon-3D GRE with a total AF of 4.5 (32). Image reconstruction was performed with integrated CS and SENSE reconstruction, which employed both coil sensitivities and sparsity for image acceleration (15). Receive sensitivity correction was implicitly applied in the CS-SENSE algorithm to improve the signal homogeneity of MR images. Detailed MR parameters for T1-GRE scans are provided in Table 1.
Standard mDixon-3D GRE T1W imaging (standard mDixon-GRE) was carried out using the dS-SENSE technique with an AF of 2.8 (2 in the phase-encoding direction and 1.4 in the slice encoding direction). CS-mDixon 3D GRE T1W imaging (CS-SENSE mDixon-GRE) was performed using a total AF of 4.5 (2 in the phase-encoding direction, 1.4 in the slice encoding direction, and 1.6 extrareduction factor accomplished with the integrated CS-SENSE algorithm). K-space sampling was performed in an irregular pattern (variable density Poisson disk). For CS-SENSE reconstruction, a regularization factor corresponding to a denoising level of 20% was used. The regularization factor has an impact on the balance between reliance to the measured data and that to the prior knowledge on the use of wavelets. Therefore, higher the regularization factor, greater the strength of wavelet regularization (higher the denoising value, lesser the noise; albeit more blurring) (24). Total time of reconstruction of the CS-SENSE mDixon-GRE image set was approximately 30 seconds.
The image quality of each HBP dataset from 217 patients (54 for preliminary study and 163 for formal review) was evaluated by two abdominal radiologists (with 6 and 23 years of experience, respectively, in the interpretation of abdominal MRI) blinded to the MR technique independently, and then in consensus. For each patient, heavily T2-weighted images were given to provide basic anatomical information. Image quality assessment of the two image sets included: blurring of the margins and fine details of anatomic structures, noise and artifacts, and overall image quality. Image blurring was assessed based on organ conspicuity, including liver edge sharpness and pancreatic margin sharpness, hepatic vessel conspicuity, and bile duct conspicuity. For the evaluation of noise and artifacts, overall image noise, the presence of in-plane aliasing artifacts, motion artifacts, fat suppression deficiency, and overall artifact level were graded. The scoring scales were set by referring to previous literature (Table 2) (3235363738). Finally, overall image quality was evaluated on a five-point scale (Table 2).
For a more concentrated review, only solid nodules were evaluated, and patients with more than 7 nodules were excluded (n = 46). Determination of the presence of true nodules was made by one radiologist (a 3rd-year radiology resident) after reviewing clinical-laboratory information, radiologic examinations including other MR sequences and CT, if available, and follow-up examinations. Finally, 117 out of 163 patients were evaluated: 91 with solid nodules and 26 without solid nodules. In total, 193 nodules were under review: 186 hypo-intense nodules and 7 hyperintensity nodules. The characteristics of the nodules are provided in the Supplementary Materials (in the online-only Data Supplement).
In total, 234 sets of HBP axial scans, either the standard mDixon-GRE or CS-SENSE mDixon-GRE data sets, were randomly distributed with matched heavily T2-weighted images to exclude cysts or hemangiomas. Three independent readers (with 5, 10, and 8 years of experience, respectively) evaluated the lesion conspicuity of each suspected nodule on a five-point scale and recorded the location and size for lesion localization. A picture archiving and communication system workstation (Infinitt, Seoul, Korea) was used.
The image quality of standard mDixon and CS-SENSE mDixon-GRE datasets from the consensus reading and the image quality for lesion detection evaluated by each reader were compared using the Wilcoxon signed-rank test. Levels of interobserver agreement were evaluated using intraclass correlation coefficients (ICCs) with a two-way model: 0.76–1.0, excellent agreement; 0.40–0.75, fair to good agreement; < 0.40, poor agreement (39). Statistical analyses were performed using MedCalc software version 15.8 (MedCalc, Mariakerke, Belgium).
For solid FFL detectability analysis, the primary endpoint of the study was set as the comparison between the ability to detect nodules from standard and CS-SENSE mDixon-GRE data sets. The non-inferiority of the CS-SENSE mDixon-GRE technique over the standard mDixon-GRE technique was established if the lower limit for the 95% confidence interval (CI) for the difference was greater than −0.1, based on previous studies on lesion detection (4041). Figure-of-merit (FOM), i.e., the probability of the rating of the highest-rated lesion with correct localization exceeding that of the highest-rated non-localized mark in a normal case was evaluated and compared with the random case, the fixed reader method of Jackknife alternative free-response receiver operating characteristic (JAFROC) analysis (40) using JAFROC version 4.2.1. For all tests, values of p < 0.05 were considered to indicate statistical significance.
There was no overall difference in image quality between the two HBP scans (p = 0.663). Among all the 163 patients, 4.3% (7/163) were scored as unreadable or poor in overall image quality in both the standard and CS-SENSE mDixon-GRE techniques. However, CS-SENSE mDixon-GRE showed higher image noise (standard mDixon-GRE vs. CS-SENSE mDixon-GRE; 3.01 ± 0.50 vs. 2.53 ± 0.65, p < 0.0001) and aliasing artifacts (2.75 ± 0.44 vs. 2.42 ± 0.52, p < 0.0001) when compared with standard mDixon-GRE. However, CS-SENSE mDixon-GRE showed less motion artifacts (3.69 ± 0.75 vs. 3.85 ± 0.66, p = 0.005) (Table 3, Figs. 2,3,4). The standard mDixon-GRE showed decreased overall artifact level (3.74 ± 0.62 vs. 3.65 ± 0.70, p = 0.045). Two readers showed fair to excellent interobserver agreement on all parameters, of which the ICCs ranged between 0.514–0.805 (Table 3).
Table 4 demonstrates the estimated values of nodule-based and patient-based performances of the three readers in detecting solid FFLs using the JAFROC analysis. All three readers demonstrated a fair nodule-based detection rate (i.e., detected true nodules/true nodules) using both the standard mDixon-GRE images, 71.0% (137/193), 77.2% (149/193), and 89.6% (173/193), and the CS-SENSE mDixon-GRE images, 67.9% (131/193), 81.9% (158/193), and 90.2% (174/193), for reader 1, 2, and 3, respectively. With respect to patient-based estimate, all readers showed high sensitivities and specificities in both scans, all higher than 0.8 (Table 4).
In JAFROC analysis, the reader-averaged FOMs were calculated as 0.918 and 0.953 for standard mDixon and CS-SENSE mDixon-GRE image sets, respectively (Table 5). The 95% CI for the difference in the FOM between the two image sets was −0.012 to 0.081. JAFROC analysis indicated that the difference between the two image data sets was not significant for the detection of solid FLLs at the 5% level (p = 0.142). Finally, the 95% CI for the difference between two FOMs ranged higher than −0.1, which showed non-inferiority of CS-SENSE mDixon-GRE technique in solid FFL detection. Representative cases are presented in Figures 2,3,4.
Our study results showed that nine-second breath-hold HBP using CS-SENSE mDixon-GRE provided image quality comparable to that of the standard mDixon-GRE image. Moreover, CS-SENSE mDixon-GRE technique showed non-inferior diagnostic performance when compared with the standard mDixon-GRE method in the detection of solid FLLs. Our study results are in agreement with a previous study on CS-SENSE in phantom and brain imaging (15). Our study also reconfirmed the results of a study by Zhang et al. (42), which showed the feasibility of combined PI-compressed sensing method in pediatric clinical setting. The time resolution we applied on CS-SENSE mDixon-GRE sequence was 1.6 times faster than our standard mDixon-GRE sequence, and 2–2.4 times faster than usual breath-holding time of up to 18–22 seconds given in literature. The confirmed non-inferiority of the CS-SENSE mDixon-GRE sequence over standard mDixon-GRE sequence for detection of solid FLLs may have clinical value in increasing the tolerance of patients to liver MRI, which currently requires multiple, relatively long breath-holding sessions of up to 18–22 seconds.
In addition, we also found that the CS-SENSE mDixon-GRE and standard mDixon-GRE image sets showed differences in image noise and motion artifacts. CS-SENSE mDixon-GRE showed higher image noise but demonstrated lower motion artifacts than the standard mDixon-GRE. Indeed, it can be expected that the CS-SENSE mDixon-GRE image set with higher acceleration may exhibit a higher level of image noise than the standard mDixon-GRE image set due to the undersampling of the data (910). Nonetheless, although previous studies dealing with the CS technique reported that the CS technique created a blurring of fine details in brain and spine MRI (4344), in our study, CS-SENSE mDixon-GRE image showed at least similar results with respect to image blurring (organ margin conspicuity and intrahepatic structures conspicuity). This discrepancy may be attributed to the integrated CS-SENSE algorithm as well as optimization of the regulation parameter for CS-SENSE mDixon-GRE. Previous studies on CS (4344) demonstrated that the artifacts became prominent and disruptive to diagnosis at AF > 2, but we used a reduction factor of 1.6 using CS. The decreased motion artifacts and non-inferior solid FFL detectability of CS-SENSE mDixon-GRE compared with standard mDixon-GRE could be attributed to the shorter acquisition time (Standard vs. CS-SENSE mDixon-GRE, 15 seconds vs. 9 seconds) and random sampling pattern without phase information. Reduced motion artifacts might especially benefit patients with limited respiratory function (Fig. 2). In contrast, the CS-SENSE mDixon-GRE image set showed higher aliasing artifacts, when compared with the standard mDixon-GRE image set, which could be related to the more frequent reconstruction errors from both steps of CS and SENSE (15). However, the disadvantages of higher image noise and aliasing artifacts of the CS-SENSE mDixon-GRE method were balanced out by the occurrence of less motion artifacts when compared with the standard method, and this resulted in equivalent overall image quality between the two techniques.
The results of sparse reconstruction including that for CS techniques depend highly on the choice of the regularization parameters, the sampling pattern of MR data, and the degree of undersampling (11). The choice of the regularization parameters is very important to maintain image quality with the use of CS techniques. An excessively high regularization parameter value can lead to excessive removal of low-value coefficients in the sparse domain, resulting in image blurring or loss of small image features, while an excessively low value can lead to incomplete removal of incoherent artifacts (10). In our study, we used a total AF of 4.5 for CS-SENSE mDixon-GRE images, which is a relatively high acceleration of acquisition speed. In addition, we also used irregular sampling pattern (pseudorandom), which resulted in higher central sampling and lower peripheral data sampling. Furthermore, regularization corresponding to a denoising level of 20% was selected to improve denoising without significant loss in detail. By integrating CS and SENSE reconstruction, which employs both coil sensitivities and sparsity for image acceleration, additional acceleration of 1.6 times could be achieved in both Ky and Kz directions. The selection of additional AF of 1.6 was based on the results of previous studies showing that large noise amplification could occur when AF > 2, when SENSE (Ky or Kz) or CS is used (1520). As solid FFL detectability showed non-inferiority in our study, we believed that CS AF smaller than 2 was chosen for good-quality reconstruction (1624). This may be possible especially for those patients with respiratory difficulties who will most benefit from higher acceleration using the CS technique. Currently, the available 2D PI techniques such as 2D-SENSE or 2D CAIPIRINHA also could reduce the overall acquisition time of 3D T1W GRE imaging for dynamic and HBP examinations from longer than 20 seconds to 13 seconds, using an AF of 4 or 5, with less motion artifacts and improved patient compliance (324546). In our study, however, scans with SENSE technique with AF of 4.5 exhibited high noise and overall artifact level resulting in suboptimal image quality, when compared to standard SENSE.
There are some limitations in our study. First, there might be an inevitable selection bias due to the retrospective study design, but we enrolled consecutive patients to avoid it. Second, the study cohort was a heterogeneous patient population. However, we believe that this may provide a potential benefit in assessing the feasibility of the CS-SENSE mDixon-GRE technique in various clinical circumstances. Third, we fixed the AFs of CS-SENSE mDixon-GRE technique at 4.5. Further studies with different AFs of CS-SENSE are thus warranted. Moreover, comparison with other accelerated methods without using CS will be valuable. Fourth, many solid FLLs were not pathologically confirmed, however, this study was mainly designed for detection rather than a precise diagnosis. Fifth, quantitative measurement of image quality such as signal-to-noise ratio (SNR) was not performed, as the measurement of SNR using a conventional region-of-interest method may not appropriately represent the true SNR due to the nonlinear nature of CS reconstruction (10). Finally, to perform a head-to-head comparison between two sequences in the same individuals, we acquired both image sets during HBP after single administration of gadoxetic acid. Given that during HBP imaging, relatively high, homogeneous and prolonged enhancement of liver parenchyma can be achieved (29303132), the HBP could be considered appropriate to compare the two T1W sequences. However, as dynamic imaging is crucial in making a diagnosis of FLLs, further studies using dynamic images obtained by the CS-SENSE mDixon-GRE methods are warranted.
In conclusion, the CS-SENSE mDixon-GRE sequence provided comparable overall image quality and non-inferior solid FFL detectability when compared with the standard mDixon-GRE sequence in HBP images, with reduced acquisition time.
Notes
Conflicts of Interest: Two of our authors (E.K. and M.D.) were employees of Philips Healthcare. They provided technical advice for the implementation and optimization of the CS-mDixon-3D GRE sequence for HBP imaging of gadoxetic acid-enhanced MRI, but had no direct or indirect conflicts of interest. The other authors not associated with Philips Healthcare (J.G.N., J.M.L., S.M.L., H.J.K., E.S.L, B.Y.H., and J.H.Y.) maintained full control of the data and information submitted for publication at all times.
References
1. Bangiyev L, Raz E, Block TK, Hagiwara M, Wu X, Yu E, et al. Evaluation of the orbit using contrast-enhanced radial 3D fat-suppressed T-1 weighted gradient echo (radial-VIBE) sequence. Br J Radiol. 2015; 88:20140863. PMID: 26194589.
2. Breuer FA, Blaimer M, Heidemann RM, Mueller MF, Griswold MA, Jakob PM. Controlled aliasing in parallel imaging results in higher acceleration (CAIPIRINHA) for multi-slice imaging. Magn Reson Med. 2005; 53:684–691. PMID: 15723404.


3. Breuer FA, Blaimer M, Mueller MF, Seiberlich N, Heidemann RM, Griswold MA, et al. Controlled aliasing in volumetric parallel imaging (2D CAIPIRINHA). Magn Reson Med. 2006; 55:549–556. PMID: 16408271.


4. Runge VM, Richter JK, Heverhagen JT. Speed in clinical magnetic resonance. Invest Radiol. 2017; 52:1–17. PMID: 27828787.


5. Bruix J, Sherman M. American Association for the Study of Liver Disease. Management of hepatocellular carcinoma: an update. Hepatology. 2011; 53:1020–1022. PMID: 21374666.


6. European Association for the Study of the Liver, European Organisation for Research and Treatment of Cancer. EASL-EORTC clinical practice guidelines: management of hepatocellular carcinoma. J Hepatol. 2012; 56:908–943. PMID: 22424438.
7. Liver imaging reporting and data system (LI-RADS). Acr.org Web site. Published July 2018. Accessed August 29, 2018. https://www.acr.org/Clinical-Resources/Reporting-and-Data-Systems/LI-RADS.
8. Yoon JH, Park JW, Lee JM. Noninvasive diagnosis of hepatocellular carcinoma: elaboration on Korean Liver Cancer Study Group-National Cancer Center Korea practice guidelines compared with other guidelines and remaining issues. Korean J Radiol. 2016; 17:7–24. PMID: 26798212.


9. Jaspan ON, Fleysher R, Lipton ML. Compressed sensing MRI: a review of the clinical literature. Br J Radiol. 2015; 88:20150487. PMID: 26402216.


10. Feng L, Benkert T, Block KT, Sodickson DK, Otazo R, Chandarana H. Compressed sensing for body MRI. J Magn Reson Imaging. 2017; 45:966–987. PMID: 27981664.


11. Yang AC, Kretzler M, Sudarski S, Gulani V, Seiberlich N. Sparse reconstruction techniques in magnetic resonance imaging: methods, applications, and challenges to clinical adoption. Invest Radiol. 2016; 51:349–364. PMID: 27003227.
12. McGibney G, Smith MR, Nichols ST, Crawley A. Quantitative-evaluation of several partial Fourier reconstruction algorithms used in MRI. Magn Reson Med. 1993; 30:51–59. PMID: 8371675.
13. Pruessmann KP, Weiger M, Scheidegger MB, Boesiger P. SENSE: sensitivity encoding for fast MRI. Magn Reson Med. 1999; 42:952–962. PMID: 10542355.


14. Griswold MA, Jakob PM, Heidemann RM, Nittka M, Jellus V, Wang JM, et al. Generalized autocalibrating partially parallel acquisitions (GRAPPA). Magn Reson Med. 2002; 47:1202–1210. PMID: 12111967.


15. Liang D, Liu B, Wang J, Ying L. Accelerating SENSE using compressed sensing. Magn Reson Med. 2009; 62:1574–1584. PMID: 19785017.


16. Lustig M, Donoho D, Pauly JM. Sparse MRI: the application of compressed sensing for rapid MR imaging. Magn Reson Med. 2007; 58:1182–1195. PMID: 17969013.


17. Glockner JF, Hu HH, Stanley DW, Angelos L, King K. Parallel MR imaging: a user's guide. Radiographics. 2005; 25:1279–1297. PMID: 16160112.


18. Wright KL, Harrell MW, Jesberger JA, Landeras L, Nakamoto DA, Thomas S, et al. Clinical evaluation of CAIPIRINHA: comparison against a GRAPPA standard. J Magn Reson Imaging. 2014; 39:189–194. PMID: 24123420.


19. Sodickson DK, Manning WJ. Simultaneous acquisition of spatial harmonics (SMASH): fast imaging with radiofrequency coil arrays. Magn Reson Med. 1997; 38:591–603. PMID: 9324327.


20. Liu F, Duan Y, Peterson BS, Kangarlu A. Compressed sensing MRI combined with SENSE in partial k-space. Phys Med Biol. 2012; 57:N391–N403. PMID: 23073235.
21. Vasanawala SS, Alley MT, Hargreaves BA, Barth RA, Pauly JM, Lustig M. Improved pediatric MR imaging with compressed sensing. Radiology. 2010; 256:607–616. PMID: 20529991.


22. Candès EJ, Romberg J, Tao T. Robust uncertainty principles: exact signal reconstruction from highly incomplete frequency information. IEEE Trans Inf Theory. 2006; 52:489–509.
24. Lustig M, Donoho DL, Santos JM, Pauly JM. Compressed sensing MRI. IEEE Signal Process Mag. 2008; 25:72–82.


25. Hollingsworth KG. Reducing acquisition time in clinical MRI by data undersampling and compressed sensing reconstruction. Phys Med Biol. 2015; 60:R297–R322. PMID: 26448064.


26. Chandarana H, Feng L, Block TK, Rosenkrantz AB, Lim RP, Babb JS, et al. Free-breathing contrast-enhanced multiphase MRI of the liver using a combination of compressed sensing, parallel imaging, and golden-angle radial sampling. Invest Radiol. 2013; 48:10–16. PMID: 23192165.


27. Runge VM. Current technological advances in magnetic resonance with critical impact for clinical diagnosis and therapy. Invest Radiol. 2013; 48:869–877. PMID: 24126386.


28. Chandarana H, Feng L, Ream J, Wang A, Babb JS, Block KT, et al. Respiratory motion-resolved compressed sensing reconstruction of free-breathing radial acquisition for dynamic liver MRI. Invest Radiol. 2015; 50:749–756. PMID: 26146869.
29. Lee YJ, Lee JM, Lee JS, Lee HY, Park BH, Kim YH, et al. Hepatocellular carcinoma: diagnostic performance of multidetector CT and MR imaging-a systematic review and meta-analysis. Radiology. 2015; 275:97–109. PMID: 25559230.


30. Niekel MC, Bipat S, Stoker J. Diagnostic imaging of colorectal liver metastases with CT, MR imaging, FDG PET, and/or FDG PET/CT: a meta-analysis of prospective studies including patients who have not previously undergone treatment. Radiology. 2010; 257:674–684. PMID: 20829538.


31. Guo J, Seo Y, Ren S, Hong S, Lee D, Kim S, et al. Diagnostic performance of contrast-enhanced multidetector computed tomography and gadoxetic acid disodium-enhanced magnetic resonance imaging in detecting hepatocellular carcinoma: direct comparison and a meta-analysis. Abdom Radiol (NY). 2016; 41:1960–1197. PMID: 27318936.


32. Yoon JH, Lee JM, Yu MH, Kim EJ, Han JK, Choi BI. High-resolution T1-weighted gradient echo imaging for liver MRI using parallel imaging at high-acceleration factors. Abdom Imaging. 2014; 39:711–721. PMID: 24557640.


33. Takayama Y, Nishie A, Asayama Y, Ishigami K, Kakihara D, Nakayama T, et al. Image quality of Gd-EOB-DTPA-enhanced magnetic resonance imaging of the liver using dual-source parallel radiofrequency transmission technology: comparison with the post-processing correction method for B1 inhomogeneity-induced signal loss. Eur J Radiol. 2012; 81:3035–3040. PMID: 22578836.


34. Rahbar H, Partridge SC, DeMartini WB, Gutierrez RL, Parsian S, Lehman CD. Improved B1 homogeneity of 3 tesla breast MRI using dual-source parallel radiofrequency excitation. J Magn Reson Imaging. 2012; 35:1222–1226. PMID: 22282269.


35. Lum DP, Busse RF, Francois CJ, Brau AC, Beatty PJ, Huff J, et al. Increased volume of coverage for abdominal contrast-enhanced MR angiography with two-dimensional autocalibrating parallel imaging: initial experience at 3.0 tesla. J Magn Reson Imaging. 2009; 30:1093–1110. PMID: 19856443.


36. Mostardi PM, Glockner JF, Young PM, Riederer SJ. Contrast-enhanced MR angiography of the abdomen with highly accelerated acquisition techniques. Radiology. 2011; 261:587–597. PMID: 21900616.


37. Nael K, Ruehm SG, Michaely HJ, Pope W, Laub G, Finn JP, et al. High spatial-resolution CE-MRA of the carotid circulation with parallel imaging: comparison of image quality between 2 different acceleration factors at 3.0 tesla. Invest Radiol. 2006; 41:391–339. PMID: 16523022.
38. Morakkabati-Spitz N, Gieseke J, Kuhl C, Lutterbey G, von Falkenhausen M, Träber F, et al. MRI of the pelvis at 3T: very high spatial resolution with sensitivity encoding and flip-angle sweep technique in clinically acceptable scan time. Eur Radiol. 2006; 16:634–641. PMID: 16228214.


39. McClellan TR, Motosugi U, Middleton MS, Allen BC, Jaffe TA, Miller CM, et al. Intravenous gadoxetate disodium administration reduces breath-holding capacity in the hepatic arterial phase: a multi-center randomized placebo-controlled trial. Radiology. 2016; 282:361–368. PMID: 27509544.


40. Fletcher JG, Yu L, Li Z, Manduca A, Blezek DJ, Hough DM, et al. Observer performance in the detection and classification of malignant hepatic nodules and masses with CT image-space denoising and iterative reconstruction. Radiology. 2015; 276:465–478. PMID: 26020436.


41. Lee SJ, Park SH, Kim AY, Yang SK, Yun SC, Lee SS, et al. A prospective comparison of standard-dose CT enterography and 50% reduced-dose CT enterography with and without noise reduction for evaluating Crohn disease. AJR Am J Roentgenol. 2011; 197:50–57. PMID: 21701010.


42. Zhang T, Chowdhury S, Lustig M, Barth RA, Alley MT, Grafendorfer T, et al. Clinical performance of contrast enhanced abdominal pediatric MRI with fast combined parallel imaging compressed sensing reconstruction. J Magn Reson Imaging. 2014; 40:13–25. PMID: 24127123.


43. Sharma SD, Fong CL, Tzung BS, Law M, Nayak KS. Clinical quality assessment of accelerated magnetic resonance neuroimaging using compressed sensing. Invest Radiol. 2013; 48:638–645. PMID: 23538890.
44. Worters PW, Sung K, Stevens KJ, Koch KM, Hargreaves BA. Compressed-sensing multispectral imaging of the postoperative spine. J Magn Reson Imaging. 2013; 37:243–248. PMID: 22791572.


45. Park YS, Lee CH, Kim IS, Kiefer B, Woo ST, Kim KA, et al. Usefulness of controlled aliasing in parallel imaging results in higher acceleration in gadoxetic acid-enhanced liver magnetic resonance imaging to clarify the hepatic arterial phase. Invest Radiol. 2014; 49:183–188. PMID: 24276676.


46. Zhang T, Cheng JY, Potnick AG, Barth RA, Alley MT, Uecker M, et al. Fast pediatric 3D free-breathing abdominal dynamic contrast enhanced MRI with high spatiotemporal resolution. J Magn Reson Imaging. 2015; 41:460–473. PMID: 24375859.


Supplementary Materials
The online-only Data Supplement is available with this article at https://doi.org/10.3348/kjr.2018.0310.
Fig. 1
Flowchart of inclusion and exclusion criteria of our main study population.
CS = compressed sensing, GRE = gradient-recalled-echo, HBP = hepatobiliary phase, SENSE = sensitivity coding, 3D = three-dimensional
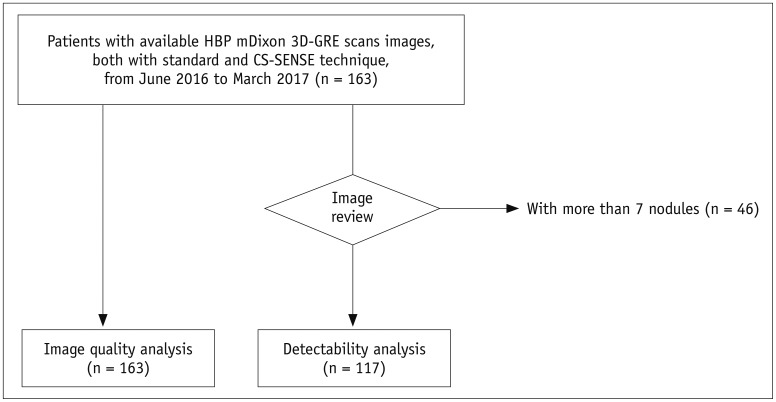
Fig. 2
59-year-old male patient who had undergone liver segmentectomy due to HCC underwent gadoxetic acid-enhanced MRI for surveillance of recurrence of HCC.
HBP standard mDixon-GRE image (A) shows severe motion artifacts (score 2) resulting in image blurring, and similar level of motion artifacts is also seen on other phases of standard mDixon-GRE images (C). Motion artifact level was significantly decreased to score of 4 on HBP CS-SENSE mDixon-GRE image (B) although image noise is more prominent than on standard mDixon-GRE image. HCC = hepatocellular carcinoma
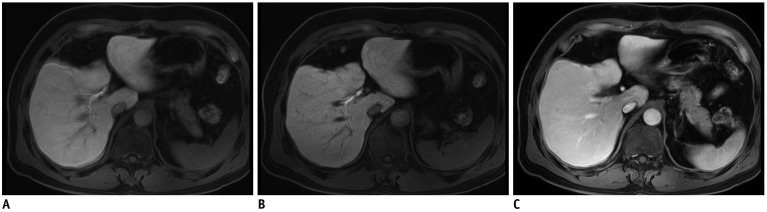
Fig. 3
75-year-old female patient with multiple lesions of HCC.
Standard mDixon-GRE image obtained during HBP (A) shows severe motion artifacts (score 2). Contrarily, motion artifacts are significantly decreased to score of 4 on HBP CS-SENSE mDixon-GRE image (B). Note that CS-SENSE mDixon-GRE image demonstrates better lesion conspicuity as well as depiction of intrahepatic vessels and intrahepatic bile ducts. Similar level of motion artifacts was also demonstrated on portal venous phase image with standard mDixon-GRE images (C), representing limited breath-holding capacity of patient.
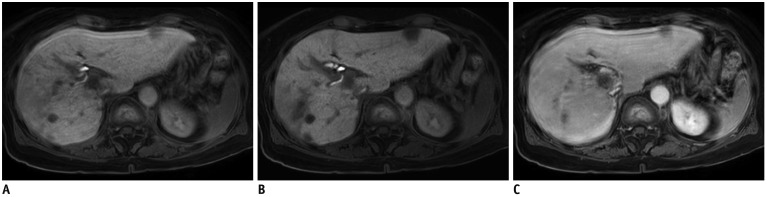
Fig. 4
65-year-old male patient with multiple HCCs.
Standard mDixon-GRE image (A) and CS-SENSE mDixon-GRE image (B) obtained during HBP showed similar level of motion artifact (both score 3). However, aliasing artifact was more prominent in CS-SENSE mDixon-GRE image (B, arrowheads).
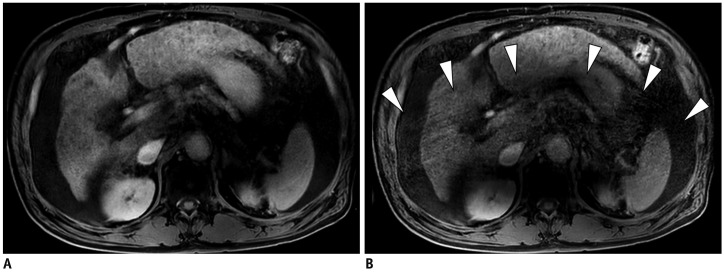
Table 1
MR Parameters of Two T1-Weighted mDixon-GRE Sequences of Hepatobiliary Phase Imaging of Gadoxetic Acid-Enhanced Liver MRI
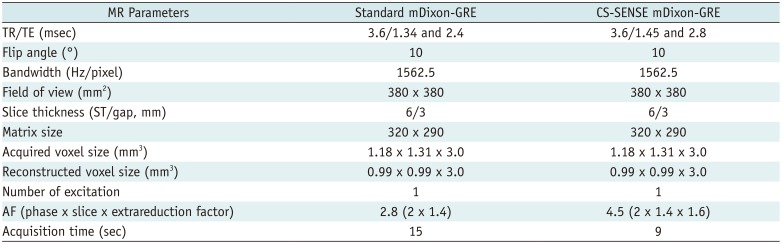
Table 2
Scoring Scale for Various Parameters in Assessment of Image Quality for Each Hepatobiliary MR Scan
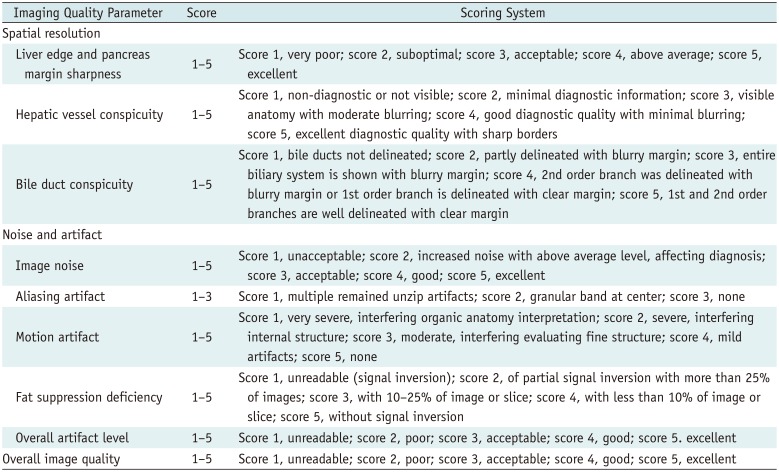
Table 3
Qualitative Analysis Results for Image Quality based on Consensus Reading of Two Independent Reviewers
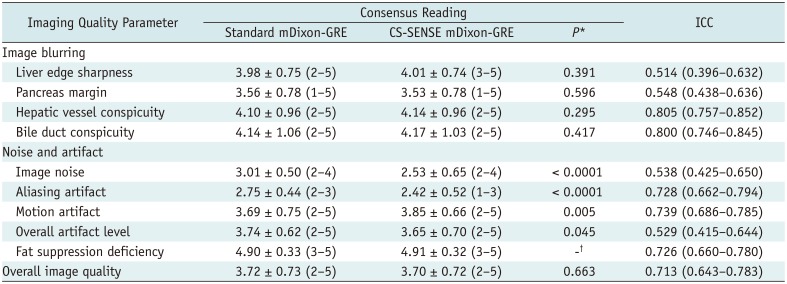
Table 4
Nodule- and Patient-Based Estimates in Detection of Solid Focal Liver Lesions
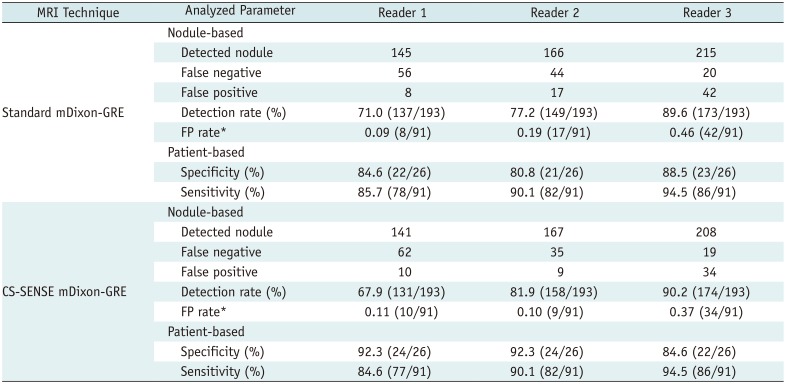