Abstract
Objective
To identify the optimal factors in diffusion tensor imaging for predicting corticospinal tract (CST) injury caused by brain tumors.
Materials and Methods
This prospective study included 33 patients with motor weakness and 64 patients with normal motor function. The movement of the CST, minimum distance between the CST and the tumor, and relative fractional anisotropy (rFA) of the CST on diffusion tensor imaging, were compared between patients with motor weakness and normal function. Logistic regression analysis was used to obtain the optimal factor predicting motor weakness.
Results
In patients with motor weakness, the displacement (8.44 ± 6.64 mm) of the CST (p = 0.009), minimum distance (3.98 ± 7.49 mm) between the CST and tumor (p < 0.001), and rFA (0.83 ± 0.11) of the CST (p < 0.001) were significantly different from those of the normal group (4.64 ± 6.65 mm, 14.87 ± 12.04 mm, and 0.98 ± 0.05, respectively) (p = 0.009, p < 0.001, and p < 0.001). The frequencies of patients with the CST passing through the tumor (6%, p = 0.002), CST close to the tumor (23%, p < 0.001), CST close to a malignant tumor (high grade glioma, metastasis, or lymphoma) (19%, p < 0.001), and CST passing through infiltrating edema (19%, p < 0.001) in the motor weakness group, were significantly different from those of the patients with normal motor function (0, 8, 1, and 10%, respectively). Logistic regression analysis showed that decreased rFA and CST close to a malignant tumor were effective variables related to motor weakness.
For patients with brain tumors which permit surgical resection, the intact fiber pathway always predicts a good prognosis and should be protected during surgery (1). The preoperative exploration and estimation of the intactness of fiber pathways is of great value in optimizing the surgical plan and protecting the important fibers. Currently, diffusion tensor imaging (DTI) is the only available tool that displays the pathway of neural fibers in vivo. Thus, it is critical to evaluate the involvement of white matter by reconstruction of the fiber pathway and fractional anisotropy (FA) changes. Previous studies have found that the displacement, deformation, infiltration, disruption, and disorganization of the fibers due to tumors are well recognized by the destruction of fiber tracts or decreased FA values from DTI tractography (234).
As corticospinal tract (CST) is the most important pathway responsible for intact motor functions, it has been widely explored using DTI. In a study of brain tumors with abnormal CST (including deviated, deformed, and interrupted tract), DTI showed high sensitivity in predicting the functional injury of the peritumoral white matter in the brain stem, although with low specificity (5). This effect causes a dilemma for neurosurgeons, and might result in overestimating the destruction of neural fibers (6). Similar results were also obtained in stroke patients by using a morphological evaluation (7). Compared with evaluation of morphological changes, evaluating neural fiber injury by using quantificational DTI metrics might be more reliable.
Several studies demonstrated that the FA value decreased in patients with abnormal motor function (8910). In stroke patients, Puig et al. (11) used a cutoff value of 0.925 for relative FA (rFA) to predict motor deficits, and obtained high sensitivity and specificity of 95.2% and 94.9%, respectively. However, as a non-specific parameter, it is unclear whether FA can accurately predict the structural damage to the CST in brain tumor. Few studies have assessed the value of FA in predicting the CST damage (91213). Thus, it is of great clinical significance to estimate the value of FA in predicting the destruction of the CST, and explore the optimal factors to help improve diagnostic accuracy. We hypothesized that FA combined with other DTI alterations, could accurately predict CST injury in patients with brain tumors. The purpose of this study was to identify the optimal factor of predicting CST injury caused by brain tumors.
This study was approved by the Institution Review Board. All patients provided written informed consent. Patients with suspected brain tumors who had undergone preoperative DTI, were prospectively enrolled in this study. Patients were excluded if: 1) they had received hormone therapy or radiotherapy before the MRI scan; 2) they had a large tumor involving the bilateral CST; 3) the motor cortex was invaded by a tumor, edema, or compression; or 4) they had infratentorial tumors. Finally, a total of 97 patients (58 males and 39 females; mean age, 51 years; age range, 14–78 years) with intracranial tumors confirmed by histopathology, were included in the study. Of these patients, 31 were low-grade gliomas (World Health Organization [WHO] grade I–II), 30 were high grade gliomas (WHO grade III–IV), 14 were metastatic tumors, 20 were meningiomas, and 2 were lymphomas. There were 54 tumors located in the frontal region, 17 in the temporal region, 9 in the basal ganglia region, 5 in the temporo-occipital region, and the remaining were located in the occipital, parietal, frontotemporal, temporoparietal, and frontoparietal regions. The muscle strength of the upper and lower limbs was evaluated with a clinical examination (level 0–5) (14). Reduced muscle strength was present in 33 patients, and the remaining 64 patients showed normal strength. For subjects with inconsistency in the upper and lower limb strength, the weaker limbs were used for the analysis.
MRI scanning was accomplished using Signa GE 3T HDxt (GE Healthcare, Milwaukee, WI, USA) with an eight-channel head coil. Before DTI acquisition, conventional T1-weighted imaging (T1WI), T2-weighted imaging (T2WI), fluid attenuated inversion recovery, and contrast enhanced T1WI were performed. DTI parameters were as follows: repetition time and echo time of 10000 ms and 87 ms, respectively; 30 directions; b values of 0/1000 s/mm2; matrix of 128 × 128 interpolated to an image matrix of 256 × 256; and slice thickness of 4 mm with no gaps. A total of 30 consecutive slices were acquired in 6 minutes. The original data were exported to DTIStudio (Johns Hopkins University; https://www.mristudio.org/). Images were observed in the “Original View” and remarkable motion artifacts were removed. An affine warp model in Automated Image Registration was used to correct image distortions due to eddy currents, and misregistration due to head motion. After correction of the data, the three eigenvalues, λ1, λ2, and λ3, were calculated. The FA maps were also calculated.
Fiber tracing was carried out after calculating the tensor. The criterion to stop the tracking was set to a turning angle greater than 60 degrees, and the FA threshold was set to 0.15. According to the expected CST pathway, the tracer for a region of interest (ROI) in the anterior pons, posterior internal capsule and pre- and post-central gyrus was determined. The logical operator “and” was used to obtain fiber bundles passing through the three ROIs, while the operator “not” was used to remove fiber bundles outside of the ROIs. The CST displacement of the affected side was measured according to the location of the contralateral CST at the level of the maximum tumor size (Fig. 1). The minimum distance between the affected CST and the tumor was also measured. The boundaries of the tumors were evaluated by a specialist (having more than 20 years of diagnostic experience) using all MR images, including contrast-enhanced images. The average FA of the whole CST was evaluated using the “tract statistics” function in DTIStudio. The rFA was calculated as the ratio between the FA of the affected side and the FA of the contralateral side. To evaluate the reproducibility and repeatability of the rFA measurement, the rFA was re-measured and re-calculated more than 3 months later by the same author. A medical student, who was also trained to trace the CST and was blinded to the previous results, measured and calculated the rFA. The relationship between the CST and the location of the tumor or edema was recorded. It included: the CST passing through the tumor, CST close to the tumor, CST close to a malignant tumor (including high-grade glioma, metastasis and lymphoma), CST passing through an infiltrating edema (areas of high signal intensity around a high grade glioma and lymphoma observable in T2WI), and CST passing through a pure vasogenic edema (areas of high signal intensity around the metastasis and meningioma). The expression “close to” was defined as true when the minimum distance between the CST and the tumor was zero.
Using a t test, the displacement, minimum distance, and rFA of the CST of patients with motor weakness were compared to patients with normal muscle strength. Receiver operating characteristic (ROC) curve was used to assess their classification efficiency. The optimal cutoff value was chosen to maximize the Youden index. Chi-square test was used to compare the frequency number of the CST location between patients with normal and decreased muscle strength. Logistic regression analysis (conditional forward) was used to determine the factors contributing to motor function. A predictive equation was generated with effective factors, and used to predict the motor weakness with a cutoff of 0.5. Intra-class correlation coefficients were calculated to assess intra- and inter-observer reproducibility for rFA. Statistical analyses were performed with SPSS software (version 22, IBM, Armonk, NY, USA), with two-sided p < 0.05 indicating a significant difference.
In 33 patients with motor weakness, the degree of motor weakness was level 0 in 1, level 2 in 2, level 3 in 10, and level 4 in 20 patients. In patients with motor weakness, the displacement (8.44 ± 6.64 mm) of the CST was significantly higher than in patients with normal motor function (4.64 ± 6.65 mm) (p = 0.009) (Table 1). Minimum distance (3.98 ± 7.49 mm) and rFA (0.83 ± 0.11) were also lower in patients with motor weakness than in patients with normal motor function (14.87 ± 12.04 mm and 0.98 ± 0.05, respectively) (p < 0.001). ROC curve analysis revealed that the areas under the curve were 0.92 for rFA, 0.81 for minimum distance, and 0.69 for displacement (Fig. 2).
Using the optimum cutoff value of 0.95 for rFA, the sensitivity and specificity to predict motor weakness were 84.8% and 87.5%, respectively. In patients with motor weakness, rFA with a level 2–3 (0.77 ± 0.09, n = 13) was significantly lower than rFA with a level 4 (0.87 ± 0.11, n = 20) in normal patients (p < 0.001). The chi-square test showed that the frequency of patients with the CST passing through the tumor in the motor weakness group (6%) was significantly different from that of patients with normal motor function (0) (p = 0.002) (Fig. 3). Similarly, the frequency of CST in the motor weakness group close to the tumor (23%, p < 0.001), close to a malignant tumor (19%, p < 0.001) (Figs. 4,5), and passing through infiltrating edema (19%) (Fig. 5), were also significantly different from that of patients with normal motor function (8, 1, and 10%, respectively) (Table 2). Logistic regression analysis showed that the factors rFA and CST close to a malignant tumor were effective contributors affecting the muscle strength, while the remaining factors were not included in the regression function. With a classification cutoff of 0.5, a total of 94.8% cases could be correctly classified by the predictive equation:
The area under the ROC curve generated with predicted probabilities of the equation was 0.96. There was a good correlation between repeated measurements of rFA. The correlation coefficient for the two measurements from the same author was 0.94 (p < 0.001), whereas the correlation between the measurements of two different authors was 0.90 (p < 0.001).
Our results show that the displacement of the CST, minimum distance between the affected CST and the tumor, as well as rFA and the relative location of the CST with respect to tumors, impacted the motor function of patients with brain tumors. Among them, rFA and CST close to malignant tumors were the major contributors. In patients with decreased muscle strength, rFA was significantly lower than that of the normal muscle strength group, which is consistent with previous studies (8,9,10). In this group, rFA was an important marker for CST injury in brain tumors. With an optimum cutoff value of 0.95 for rFA, the sensitivity and specificity were 84.8% and 87.5%, respectively. In stroke patients, the sensitivity and specificity were 95.2% and 94.9%, to predict motor deficit with a cutoff value of 0.925 for rFA (11). The lower predicting accuracy in the current study may be associated with more complex pathological changes of white matter in patients with tumors (15). In areas of marked peritumoral edema, although the FA decreased, the integrity of the CST bundle was retained (1617). Therefore, significantly decreased FA also observed in some patients with normal muscle strength. A previous study showed that the function of the white matter tracts in the area of edema, which showed interruption or branching, can be normal (16).
Upon combining with information regarding the closeness of the CST to a malignant tumor, there was an improvement in the predicting accuracy of rFA. The invasive growth of malignant tumors are capable of damaging the surrounding tissues. In our results, motor weakness occurred in 18 out of 19 patients with the CST close to a malignant tumor. Therefore, the “closeness to a malignant tumor” of the CST may supplement the rFA, and thereby improve the diagnostic accuracy. Preoperative determination of tumor histopathology may be difficult. Nevertheless, these malignant tumors generally show significant enhancement, thus being easily distinguished from a low grade glioma and meningioma.
The morphological changes of white matter include compression and displacement (18192021). For patients with motor weakness, the displacement of the CST is significantly higher. However, this was not a major factor affecting motor function in the logistic regression. Studies have shown that in cases of simple compression, the structure of neural fibers can still retain their integrity (2223). Long-term continuous compression may lead to a decrease in the number of neural fibers or demyelination, causing a change in the FA (1324). In a study of meningioma patients, motor weakness was found to be related to lower FA resulting from increased radial diffusivity (RD) of the CST (13). RD is the diffusivity perpendicular to the neural fibers, reflecting the integrity of the myelin sheath. This suggests that functional abnormality caused by white matter compression may be a result of the long-term effects of neural fiber degeneration.
The minimum distance between the CST and the tumor was significantly different between the two groups. This could be explained by the infiltrating growth of some brain tumors, especially malignant gliomas (25). The pathological condition, involving the CST passing through the tumor or infiltrating edema, was associated with motor weakness, except for pure vasogenic edema. Pure vasogenic edema around a meningioma and metastasis has a smaller effect on brain tissue, leaving the neural fibers undamaged (26). However, none of these factors were included in the regression function due to low specificity.
Challenges could be present in quantitatively evaluating white matter tracts. An important reason is the lack of an objective and consistent method to trace specific white matter tracts (4). However, the anatomical structure of the CST is easier to distinguish, and is relatively straightforward compared to other white matter tracts. Additionally, FA varies across individuals and different ages. Fortunately, FA was found to have good bilateral symmetry (2728). In the current study, we used the relative FA compared to the opposite side, to minimize the effects of age and individual variation. Compared with the ROI-based method, measuring average FA of the whole CST showed less inter-observer variability with increased repeatability, especially in cases of distorted white matter (8). The high inter- and intra-observer correlation confirmed the reliability of our method.
This study has some limitations. First, the fiber-tracking method we used does not display complete CST bundles. When a voxel contains cross-fibers, namely more than two running directions of the fiber bundle, fiber tractography can only be obtained according to the synthetic direction connection (16). Therefore, at the centrum semiovale, the CST and the longitudinal beam cross-section of the fibers cannot be displayed (1629). Second, due to peritumoral edema and infiltration, the lowest FA threshold (0.15) was selected to represent the structural state of the white matter (30). However, there is still no consensus on the threshold. In addition, this study is not based on the number of fibers and the white matter bundle volume in DTI as the analysis index; it mainly takes into account the variation of these two quantitative evaluation indices (28). Finally, the injection of gadolinium may have some influence on the FA value. However, the changes after contrast enhancement were mainly in the enhanced tumor region, while lesser changes occurred in the regions of edema and normal white matter (3132).
In conclusion, in patients with brain tumors, many factors including decreased FA, higher CST displacement, and lower distance between the CST and the tumor (in comparison with patients with normal muscle strength), the CST passing through the tumor, closeness to the tumor, closeness to a malignant tumor, and CST passing through infiltrating edema, contribute to the motor weakness. However, decreased FA combined with closeness of fiber tracts to a malignant tumor, is the optimal factor predicting CST injury in patients with brain tumors.
References
1. Dimou S, Battisti RA, Hermens DF, Lagopoulos J. A systematic review of functional magnetic resonance imaging and diffusion tensor imaging modalities used in presurgical planning of brain tumour resection. Neurosurg Rev. 2013; 36:205–214. discussion 214. PMID: 23187966.


2. Yu CS, Li KC, Xuan Y, Ji XM, Qin W. Diffusion tensor tractography in patients with cerebral tumors: a helpful technique for neurosurgical planning and postoperative assessment. Eur J Radiol. 2005; 56:197–204. PMID: 15916876.


3. Itagiba VGA, Borges R, da Cruz LCH Jr, Furtado AD, Domingues RC, Gasparetto EL. Use of diffusion tensor magnetic resonance imaging in the assessment of patterns of white matter involvement in patients with brain tumors: is it useful in the differential diagnosis? Radiol Bras. 2010; 43:362–368.
4. Laundre BJ, Jellison BJ, Badie B, Alexander AL, Field AS. Diffusion tensor imaging of the corticospinal tract before and after mass resection as correlated with clinical motor findings: preliminary data. AJNR Am J Neuroradiol. 2005; 26:791–796. PMID: 15814922.
5. Kovanlikaya I, Firat Z, Kovanlikaya A, Uluğ AM, Cihangiroglu MM, John M, et al. Assessment of the corticospinal tract alterations before and after resection of brainstem lesions using diffusion tensor imaging (DTI) and tractography at 3T. Eur J Radiol. 2011; 77:383–391. PMID: 19767164.


6. Min ZG, Rana N, Niu C, Ji HM, Zhang M. Does diffusion tensor tractography of the corticospinal tract correctly reflect motor function? Med Princ Pract. 2014; 23:174–176. PMID: 23949557.


7. Kwon YH, Son SM, Lee J, Bai DS, Jang SH. Combined study of transcranial magnetic stimulation and diffusion tensor tractography for prediction of motor outcome in patients with corona radiata infarct. J Rehabil Med. 2011; 43:430–434. PMID: 21403983.


8. Kim CH, Chung CK, Kim JS, Jahng TA, Lee JH, Song IC. Use of diffusion tensor imaging to evaluate weakness. J Neurosurg. 2007; 106:111–118.


9. Morita N, Wang S, Kadakia P, Chawla S, Poptani H, Melhem ER. Diffusion tensor imaging of the corticospinal tract in patients with brain neoplasms. Magn Reson Med Sci. 2011; 10:239–243. PMID: 22214908.


10. Stadlbauer A, Nimsky C, Gruber S, Moser E, Hammen T, Engelhorn T, et al. Changes in fiber integrity, diffusivity, and metabolism of the pyramidal tract adjacent to gliomas: a quantitative diffusion tensor fiber tracking and MR spectroscopic imaging study. AJNR Am J Neuroradiol. 2007; 28:462–469. PMID: 17353313.
11. Puig J, Pedraza S, Blasco G, Daunis-I-Estadella J, Prats A, Prados F, et al. Wallerian degeneration in the corticospinal tract evaluated by diffusion tensor imaging correlates with motor deficit 30 days after middle cerebral artery ischemic stroke. AJNR Am J Neuroradiol. 2010; 31:1324–1330. PMID: 20299434.


12. De Belder FE, Oot AR, Van Hecke W, Venstermans C, Menovsky T, Van Marck V, et al. Diffusion tensor imaging provides an insight into the microstructure of meningiomas, high-grade gliomas, and peritumoral edema. J Comput Assist Tomogr. 2012; 36:577–582. PMID: 22992609.


13. Kim MS, Chung CK, Jung HW, Park CK, Kim CH, Kim JS. Preoperative weakness and demyelination of the corticospinal tract in meningioma patients: changes in diffusion parameters using diffusion tensor imaging. J Korean Neurosurg Soc. 2014; 55:267–272. PMID: 25132933.
14. Cuthbert SC, Goodheart GJ Jr. On the reliability and validity of manual muscle testing: a literature review. Chiropr Osteopat. 2007; 15:4. PMID: 17341308.


15. Kim M, Kim HS. Emerging techniques in brain tumor imaging: what radiologists need to know. Korean J Radiol. 2016; 17:598–619. PMID: 27587949.


16. Berman JI, Berger MS, Mukherjee P, Henry RG. Diffusiontensor imaging-guided tracking of fibers of the pyramidal tract combined with intraoperative cortical stimulation mapping in patients with gliomas. J Neurosurg. 2004; 101:66–72. PMID: 15255253.


17. Hendler T, Pianka P, Sigal M, Kafri M, Ben-Bashat D, Constantini S, et al. Delineating gray and white matter involvement in brain lesions: three-dimensional alignment of functional magnetic resonance and diffusion-tensor imaging. J Neurosurg. 2003; 99:1018–1027. PMID: 14705730.
18. Mori S, Frederiksen K, van Zijl PC, Stieltjes B, Kraut MA, Solaiyappan M, et al. Brain white matter anatomy of tumor patients evaluated with diffusion tensor imaging. Ann Neurol. 2002; 51:377–380. PMID: 11891834.


19. Clark CA, Barrick TR, Murphy MM, Bell BA. White matter fiber tracking in patients with space-occupying lesions of the brain: a new technique for neurosurgical planning? Neuroimage. 2003; 20:1601–1608. PMID: 14642471.


20. Yamada K, Kizu O, Mori S, Ito H, Nakamura H, Yuen S, et al. Brain fiber tracking with clinically feasible diffusion-tensor MR imaging: initial experience. Radiology. 2003; 227:295–301. PMID: 12668749.


21. Bagadia A, Purandare H, Misra BK, Gupta S. Application of magnetic resonance tractography in the perioperative planning of patients with eloquent region intra-axial brain lesions. J Clin Neurosci. 2011; 18:633–639. PMID: 21371893.


22. Mathias J, Koessler L, Brissart H, Foscolo S, Schmitt E, Bracard S, et al. Giant cystic widening of Virchow-Robin spaces: an anatomofunctional study. AJNR Am J Neuroradiol. 2007; 28:1523–1525. PMID: 17846204.


23. Young RJ, Lee V, Peck KK, Sierra T, Zhang Z, Jacks LM, et al. Diffusion tensor imaging and tractography of the corticospinal tract in the presence of enlarged Virchow-Robin spaces. J Neuroimaging. 2014; 24:79–82. PMID: 22082139.


24. Ng WH, Cheong DL, Khu KJ, Venkatesh G, Ng YK, Lim CC. Diffusion tensor tractography: corticospinal tract fiber reduction is associated with temporary hemiparesis in benign extracerebral lesions. Neurosurgery. 2008; 63:452–458. discussion 458-459. PMID: 18812956.
25. Yamahara T, Numa Y, Oishi T, Kawaguchi T, Seno T, Asai A, et al. Morphological and flow cytometric analysis of cell infiltration in glioblastoma: a comparison of autopsy brain and neuroimaging. Brain Tumor Pathol. 2010; 27:81–87. PMID: 21046309.


26. Goebell E, Paustenbach S, Vaeterlein O, Ding XQ, Heese O, Fiehler J, et al. Low-grade and anaplastic gliomas: differences in architecture evaluated with diffusion-tensor MR imaging. Radiology. 2006; 239:217–222. PMID: 16484348.


27. Schlüter M, Stieltjes B, Hahn HK, Rexilius J, Konrad-verse O, Peitgen HO. Detection of tumour infiltration in axonal fibre bundles using diffusion tensor imaging. Int J Med Robot. 2005; 1:80–86.


28. Reich DS, Smith SA, Jones CK, Zackowski KM, van Zijl PC, Calabresi PA, et al. Quantitative characterization of the corticospinal tract at 3T. AJNR Am J Neuroradiol. 2006; 27:2168–2178. PMID: 17110689.
29. Yamada K, Kizu O, Kubota T, Ito H, Matsushima S, Oouchi H, et al. The pyramidal tract has a predictable course through the centrum semiovale: a diffusion-tensor based tractography study. J Magn Reson Imaging. 2007; 26:519–524. PMID: 17729353.


30. Stadlbauer A, Nimsky C, Buslei R, Salomonowitz E, Hammen T, Buchfelder M, et al. Diffusion tensor imaging and optimized fiber tracking in glioma patients: histopathologic evaluation of tumor-invaded white matter structures. Neuroimage. 2007; 34:949–956. PMID: 17166744.


31. Bae MS, Jahng GH, Ryu CW, Kim EJ, Choi WS, Yang DM. Effect of intravenous gadolinium-DTPA on diffusion tensor MR imaging for the evaluation of brain tumors. Neuroradiology. 2009; 51:793–802. PMID: 19636547.


32. Zolal A, Sameš M, Burian M, Nováková M, Malucelli A, Hejčl A, et al. The effect of a gadolinium-based contrast agent on diffusion tensor imaging. Eur J Radiol. 2012; 81:1877–1882. PMID: 21664087.


Fig. 1
Measuring displacement of affected CST.
Displacement of affected CST was determined according to distance (m) between symmetrical position of contralateral CST and actual CST position. Vertical distance between contralateral CST and its symmetrical position to center line is same (a). CST = corticospinal tract
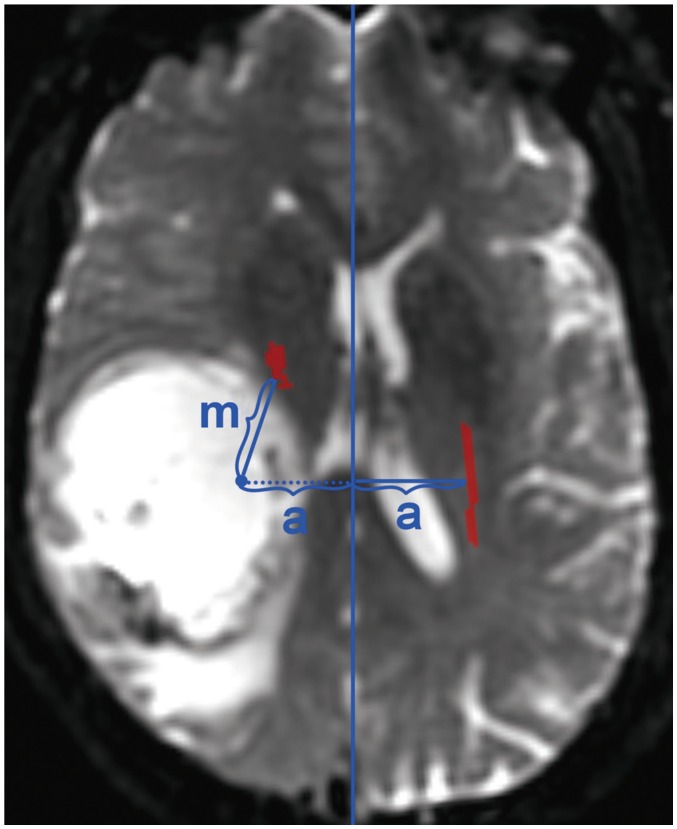
Fig. 2
ROC curves for predicting motor weakness.
ROC curves of rFA, minimum distance, displacement and predicted probabilities from logistic regression model. Areas under curves were 0.92, 0.81, 0.69, and 0.96, respectively. rFA = relative fractional anisotropy, ROC = receiver operating characteristic
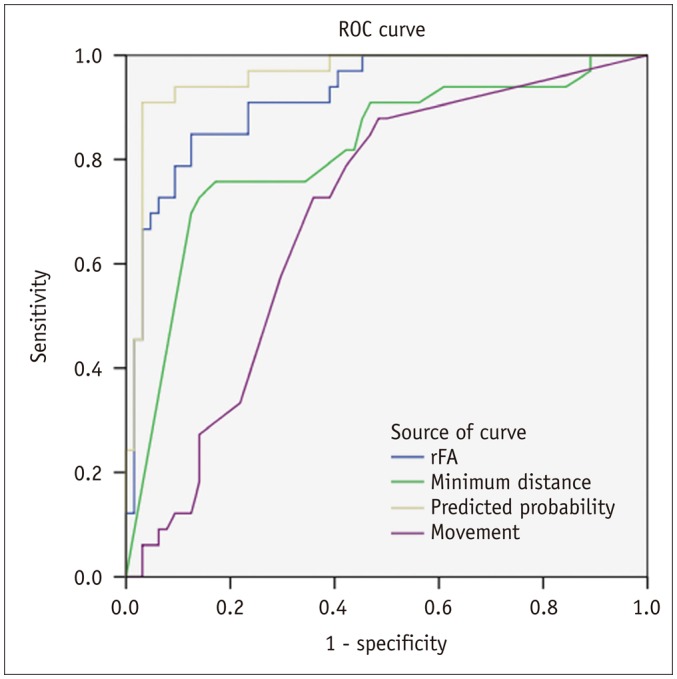
Fig. 3
Predictable value of CST passing through tumor.
A, B. Affected CST (red) passes through low-grade glioma (WHO grade II) in left basal ganglia region. C. Some fiber tracts are interrupted. rFA of affected side is 0.54. Logistic regression result was positive. Right upper and lower extremity muscle strength were both level 4. WHO = World Health Organization
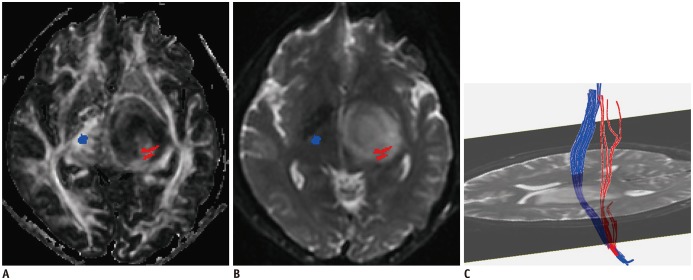
Fig. 4
Predictable value of CST close to malignant tumor.
Affected CST (red) close to metastatic tumor is mildly displaced, and FA (A) is slightly decreased (rFA = 0.98) compared to contralateral CST (blue). Fibers passing through peritumoral edema (B) show integration (C). Logistic regression result was positive. Right upper and lower extremity muscle strength were both level 3. FA = fractional anisotropy
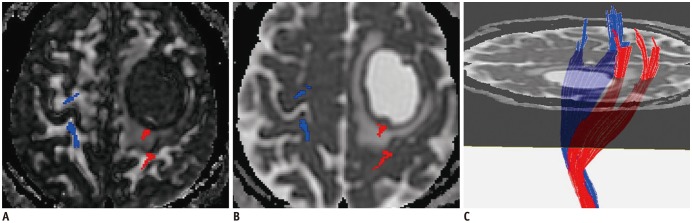
Fig. 5
Predictable value of decreased rFA.
Affected CST (red) close to anaplastic astrocytoma (WHO grade III) is slightly displaced posteriorly, and FA (A) is decreased (rFA = 0.79) compared to contralateral CST (blue). Fibers passing through peritumoral edema (B) show integration (C). Logistic regression result was positive. Right upper and lower extremity muscle strength were both level 3.
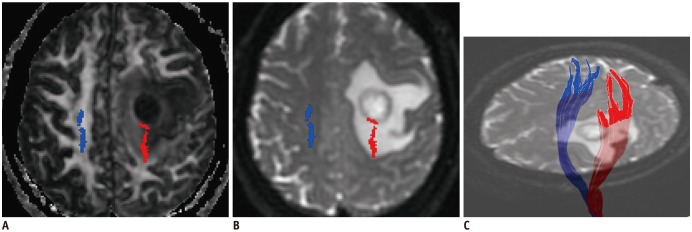
Table 1
Displacement, Minimum Distance and rFA of Corticospinal Tract between Normal and MW Group

NMW (n = 64) | MW (n = 33) | P | |
---|---|---|---|
Displacement (mm) | 4.64 ± 6.65 | 8.44 ± 6.64 | 0.009 |
Minimum distance (mm) | 14.87 ± 12.04 | 3.98 ± 7.49 | < 0.001 |
rFA | 0.98 ± 0.05 | 0.83 ± 0.11 | < 0.001 |
Table 2
Frequency of Number of Corticospinal Tract Locations between Normal and MW Group
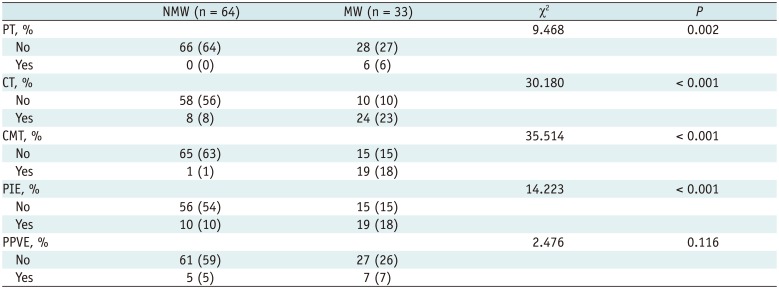