Abstract
This retrospective cohort study aimed to compare coronavirus disease 2019 (COVID-19)-related clinical outcomes between patients with and without gout. Electronic health record-based data from two centers (Seoul National University Hospital [SNUH] and Boramae Medical Center [BMC]), from January 2021 to April 2022, were mapped to a common data model. Patients with and without gout were matched using a large-scale propensity-score algorithm based on population-level estimation methods. At the SNUH, the risk for COVID-19 diagnosis was not significantly different between patients with and without gout (hazard ratio [HR], 1.07; 95% confidence interval [CI], 0.59–1.84). Within 30 days after COVID-19 diagnosis, no significant difference was observed in terms of hospitalization (HR, 0.57; 95% CI, 0.03–3.90), severe outcomes (HR, 2.90; 95% CI, 0.54–13.71), or mortality (HR, 1.35; 95% CI, 0.06–16.24). Similar results were obtained from the BMC database, suggesting that gout does not increase the risk for COVID-19 diagnosis or severe outcomes.
Graphical Abstract
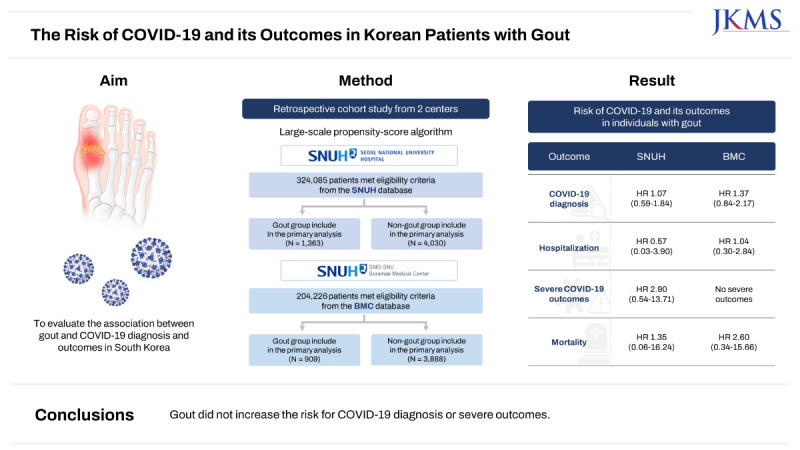
Patients with autoimmune inflammatory rheumatic diseases are at higher risk for coronavirus disease 2019 (COVID-19) diagnosis, hospitalization and worse outcomes compared with the general population.1 Gout is the most common inflammatory arthritis among adults worldwide.2 However, the association between gout and the susceptibility to and severity of COVID-19 remains unclear.345 This uncertainty arises from two key factors contributing to the risk for COVID-19 in patients with gout: the indirect impact of metabolic comorbidities, and the direct effect of gout itself, which often leads to a hyperinflammatory state.6 Moreover, unlike other autoimmune inflammatory conditions, gout has not been thoroughly investigated in the context of COVID-19. Therefore, this study aimed to evaluate the association between gout and the diagnosis and outcomes of COVID-19 in South Korea, using the Observational Health Data Sciences and Informatics’ Common Data Model (OHDSI-CDM) data.
This multicenter, retrospective, observational cohort study used data collected from two electronic health record (EHR)-based databases in two centers in South Korea, Boramae Medical Center (BMC) and Seoul National University Hospital (SNUH), from January 2021 to April 2022. Both EHR-based databases were standardized to the Observational Medical Outcomes Partnership-CDM, version 5.4, covering the years from 2004 to 2022 at SNUH and from 2010 to 2022 at BMC. The study design was established through collaboration between clinicians and data scientists, using the cohort definition feature of the CDM ATLAS platform. Data analysis was conducted using the CohortMethod R package. This package was executed separately for each database, and predefined statistical results without patient-level information were shared.
Adult patients aged 18 years or older were identified into two groups: those with a history of gout (the gout group) and those without a history of gout (the non-gout group) throughout the study period. The diagnosis of gout was ascertained using diagnosis codes obtained through concept ID codes within the CDM database. Detailed concept ID codes are included in Supplementary Table 1 for reference. The index date was defined as the first visit to each center during the study period. Individuals enrolled in the database for less than one year before the index date were excluded. In addition, patients with a history of COVID-19 at any time before the index date were also excluded. Clinical outcomes of interest included COVID-19 diagnosis, defined as either positive COVID-19 laboratory test results or specific COVID-19 diagnosis codes, as well as hospitalization, severe outcomes (defined as the use of mechanical ventilation, tracheostomy or extracorporeal membrane oxygenation), and death within 30 days of COVID-19 diagnosis. These outcomes were also identified using concept ID codes in the CDM database (Supplementary Table 1). To estimate propensity scores (PS), regularized logistic regression models that incorporate more than 20,000 baseline variables between individuals with and without gout were used.7 The variables in each database included demographics, diagnostic codes, medication records, procedures, device exposure, measurements, and visit count data. The study population was matched using 1:5 PS matching as the primary analysis to assess the association between gout and COVID-19. Cox proportional hazard regression models were used to calculate hazard ratios (HRs) with corresponding 95% confidence intervals (CIs). Kaplan-Meier curves for COVID-19 diagnosis were compared between the two groups using log-rank tests. The risk window commenced 1 day after the index date. Patients were censored when they were no longer included in the database. As part of the sensitivity analysis, three additional PS adjustments were performed: 1:1 PS matching, and PS stratification using PS quintiles and deciles. All statistical analyses were performed using R version 4.2.2 (R Foundation for Statistical Computing, Vienna, Austria). A P value of < 0.05 was considered to be statistically significant.
A total of 528,311 patients across the two facility databases were included in the analysis: SNUH, gout (n = 2,515) and non-gout (n = 321,570); BMC, gout (n = 1,053) and non-gout (n = 203,173) (Fig. 1). After 1:5 PS matching, the absolute standardized differences for all baseline patient characteristics between the gout and non-gout groups were < 0.1 within each database. Baseline characteristics of the study population before and after PS matching for each database are summarized in Table 1 and Supplementary Table 2. The median follow-up duration was 119 days for the gout group and 113 days for the non-gout group in the SNUH, and 588 days for the gout group and 562 days for the non-gout group in the BMC.
In the BMC database, before PS matching, there were 23 COVID-19 diagnosis events in the gout group and 3,049 in the non-gout group. The Kaplan-Meier curves for COVID-19 diagnosis after PS matching at the BMC are presented in Fig. 2. The risk for COVID-19 diagnosis was not significantly different between individuals with and without gout at both the SNUH (HR, 1.07; 95% CI, 0.59–1.84) and BMC (HR, 1.37; 95% CI, 0.84–2.17). Furthermore, even after various PS adjustments, there was no significant difference in the risk for COVID-19 diagnosis between individuals with and without gout in either database (Supplementary Table 3 and 4).
There was no significant difference in the risk for hospitalization within 30 days following COVID-19 diagnosis between individuals with and without gout, as indicated by an HR of 0.57 (95% CI, 0.03–3.90) at the SNUH and 1.04 (95% CI, 0.30–2.84) at the BMC. The results of sensitivity analysis for hospitalization with different PS adjustments were consistent with those of the primary analysis (Supplementary Table 3 and 4). At the SNUH, the gout group exhibited a tendency toward a higher risk for severe outcomes (HR, 2.90; 95% CI, 0.54–13.71) and mortality (HR, 1.35; 95% CI, 0.06–16.24) within 30 days following COVID-19 diagnosis, although these differences did not reach statistical significance. However, when using PS stratification with 5 or 10 strata, individuals with gout exhibited significantly higher risks for severe COVID-19 outcomes compared to those without gout (5 strata: HR, 4.66, 95% CI, 1.36–12.27; 10 strata: HR, 4.22, 95% CI, 1.17–12.21). In the BMC database, there were no severe outcomes reported within 30 days of COVID-19 diagnosis among the gout group. Individuals with gout did not have a significantly different risk of mortality (HR, 2.60; 95% CI, 0.34–15.66) compared to those without gout.
In this retrospective cohort study, patients with gout did not exhibit a significantly different risk for COVID-19 than those without gout. Furthermore, there were no significant differences in the risk for hospitalization, severe clinical outcomes, or mortality due to COVID-19 between individuals with and without gout. These findings were consistent across different databases and sensitivity analyses using various PS adjustments.
The association between gout and COVID-19 has been inconsistent, primarily because individuals with gout often have multiple comorbidities or are predisposed to various other medical conditions, including hypertension, myocardial infarction, stroke, obesity, hyperlipidemia, type 2 diabetes mellitus and chronic kidney disease.458 In a recent study that used Mendelian randomization analysis to establish unconfounded associations, gout was genetically linked to a 4.6% higher risk for critically ill COVID-19.9 There was a trend towards an increased risk of COVID-19 diagnosis and hospitalized COVID-19 in patients with gout, but these associations did not reach statistical significance.9 In this study, we used a large-scale PS model with > 20,000 baseline characteristics of individuals with and without gout to ensure balanced characteristics between the two groups. Consistent with a previous Mendelian randomization study, individuals with gout did not exhibit an increased risk for COVID-19 diagnosis or hospitalization due to COVID-19.
Interestingly, individuals with gout had a significantly increased risk for severe COVID-19 outcomes compared to those without gout in populations stratified into 5 or 10 strata using PS. These findings suggest that gout itself may be associated with severe COVID-19 outcomes rather than increased susceptibility to COVID-19. Hyperuricemia can induce epigenetic reprogramming in immune cells, rendering them more responsive to inflammatory stimuli and reinforcing a heightened state of chronic inflammation in gout.61011 Hence, in addition to genetic factors and the influence of metabolic comorbidities, immune cells primed by hyperuricemia in patients with gout may contribute to an exaggerated inflammatory response to infection with severe acute respiratory syndrome coronavirus 2 (SARS-CoV-2), potentially leading to severe COVID-19 outcomes.6 The disparity in results between PS stratification and PS matching may be due to the extremely low occurrence of these outcomes, and in PS matching, the smaller control group sample sizes might contribute to this difference.
Strengths of our study include the inclusion of Korean cohorts, whereas previous studies have primarily focused on Caucasian populations. This is important because COVID-19 outcomes vary depending on the geography and ethnicity of the affected population.1213 A previous study in the USA found that the overall odds of death from COVID-19 for non-Whites were 22% higher compared to Whites. When stratified by SARS-CoV-2 variants, non-Whites had 21%, 48%, and 19% higher odds of death than Whites during the alpha, delta, and omicron periods, respectively. Additionally, the BMC, a hospital specializing in COVID-19 treatment in Seoul, South Korea, has treated a substantial number of COVID-19 patients, resulting in a wealth of COVID-19 data. This study, however, also has several limitations. First, the risk of death from COVID-19 was associated with SARS-CoV-2 strains, and the proportion of individuals fully vaccinated.13 The strains of SARS-CoV-2 can be classified based on the following time periods: alpha (January 2021 to May 2021), delta (May 2021 to November 2021), and omicron (December 2021 to April 2022). However, specific data regarding COVID-19 vaccination is managed and provided by the government in South Korea, rather than being within the purview of hospitals. As a result, we encountered challenges in obtaining this information directly from the EHR-based database. Interestingly, male sex was significantly associated with higher COVID-19 vaccine acceptance rates in the general population, with 90% of the patients included in this study being male.14 On the other hand, vaccination rates are suboptimal among patients with inflammatory arthritis.15 Therefore, further research is necessary to analyze data in conjunction with the national database on COVID-19 vaccination. Moreover, healthcare professionals need to adopt a strategic approach to restore trust in vaccines within this patient population. Secondly, COVID-19 diagnoses were solely based on diagnosis codes or COVID-19 test results obtained from each center, leading to the inclusion of only those patients diagnosed at SNUH or BMC. Since a substantial number of COVID-19 diagnoses occurred outside these hospitals, the study exhibited low COVID-19 incidence rates, resulting in a limited occurrence of adverse outcomes and COVID-19-related deaths. Due to the restricted number of outcome occurrences, it may not have been feasible to observe a significant difference between the two groups. Third, the non-gout group may have included patients diagnosed with gout at other hospitals. Nevertheless, this was likely to have had only a minimal impact on the results given that both databases yielded similar findings.
In conclusion, individuals with gout did not exhibit an increased risk for COVID-19 or experience worse COVID-19 outcomes than those without gout. Nevertheless, further research investigating whether gout is associated with an elevated risk of severe COVID-19 is warranted.
This study was approved by the Institutional Review Board (IRB) of the BMC (IRB No. 07-2022-31). Requirements for informed written consent were waived due to the anonymized nature of the databases. The study was conducted in accordance with the principles of the Declaration of Helsinki.
Notes
Funding: This research was supported by a grant of Patient-Centered Clinical Research Coordinating Center (PACEN) funded by the Ministry of Health & Welfare, Republic of Korea (grant number: HC21C0010), as well as a grant from the Medical Data-Driven Hospital Support Project through the Korea Health Information Service (KHIS), also funded by the Ministry of Health & Welfare, Republic of Korea.
References
1. Shin YH, Shin JI, Moon SY, Jin HY, Kim SY, Yang JM, et al. Autoimmune inflammatory rheumatic diseases and COVID-19 outcomes in South Korea: a nationwide cohort study. Lancet Rheumatol. 2021; 3(10):e698–e706. PMID: 34179832.
2. Roddy E, Doherty M. Epidemiology of gout. Arthritis Res Ther. 2010; 12(6):223. PMID: 21205285.
3. Topless RK, Gaffo A, Stamp LK, Robinson PC, Dalbeth N, Merriman TR. Gout and the risk of COVID-19 diagnosis and death in the UK Biobank: a population-based study. Lancet Rheumatol. 2022; 4(4):e274–e281. PMID: 35128470.
4. Xie D, Choi HK, Dalbeth N, Wallace ZS, Sparks JA, Lu N, et al. Gout and excess risk of severe SARS-CoV-2 infection among vaccinated individuals: a general population study. Arthritis Rheumatol. 2023; 75(1):122–132. PMID: 36082457.
5. Jatuworapruk K, Montgomery A, Gianfrancesco M, Conway R, Durcan L, Graef ER, et al. Characteristics and outcomes of people with gout hospitalized due to COVID-19: data from the COVID-19 Global Rheumatology Alliance Physician-reported registry. ACR Open Rheumatol. 2022; 4(11):948–953. PMID: 36000538.
6. Tai V, Robinson PC, Dalbeth N. Gout and the COVID-19 pandemic. Curr Opin Rheumatol. 2022; 34(2):111–117. PMID: 34907115.
7. Tian Y, Schuemie MJ, Suchard MA. Evaluating large-scale propensity score performance through real-world and synthetic data experiments. Int J Epidemiol. 2018; 47(6):2005–2014. PMID: 29939268.
8. Choi HK, McCormick N, Yokose C. Excess comorbidities in gout: the causal paradigm and pleiotropic approaches to care. Nat Rev Rheumatol. 2022; 18(2):97–111. PMID: 34921301.
9. Peng H, Wu X, Xiong S, Li C, Zhong R, He J, et al. Gout and susceptibility and severity of COVID-19: a bidirectional Mendelian randomization analysis. J Infect. 2022; 85(3):e59–e61. PMID: 35724757.
10. Crișan TO, Cleophas MC, Oosting M, Lemmers H, Toenhake-Dijkstra H, Netea MG, et al. Soluble uric acid primes TLR-induced proinflammatory cytokine production by human primary cells via inhibition of IL-1Ra. Ann Rheum Dis. 2016; 75(4):755–762. PMID: 25649144.
11. Tanaka T, Milaneschi Y, Zhang Y, Becker KG, Zukley L, Ferrucci L. A double blind placebo controlled randomized trial of the effect of acute uric acid changes on inflammatory markers in humans: a pilot study. PLoS One. 2017; 12(8):e0181100. PMID: 28786993.
12. Conway R, Nikiphorou E, Demetriou CA, Low C, Leamy K, Ryan JG, et al. Temporal trends in COVID-19 outcomes in people with rheumatic diseases in Ireland: data from the COVID-19 Global Rheumatology Alliance registry. Rheumatology (Oxford). 2022; 61(SI2):SI151–SI156. PMID: 35258593.
13. Tabatabai M, Juarez PD, Matthews-Juarez P, Wilus DM, Ramesh A, Alcendor DJ, et al. An analysis of COVID-19 mortality during the dominancy of alpha, delta, and omicron in the USA. J Prim Care Community Health. 2023; 14:21501319231170164. PMID: 37083205.
14. Yasmin F, Najeeb H, Moeed A, Naeem U, Asghar MS, Chughtai NU, et al. COVID-19 vaccine hesitancy in the United States: a systematic review. Front Public Health. 2021; 9:770985. PMID: 34888288.
15. Moraliyska R, Georgiev T, Bogdanova-Petrova S, Shivacheva T. Adoption rates of recommended vaccines and influencing factors among patients with inflammatory arthritis: a patient survey. Rheumatol Int. Forthcoming. 2023; DOI: 10.1007/s00296-023-05476-2.
SUPPLEMENTARY MATERIALS
Supplementary Table 2
Baseline characteristics of the study population in the Seoul National University Hospital
Supplementary Table 3
Sensitivity analysis for the risk of COVID-19 diagnosis, hospitalization, severe COVID-19 outcomes and mortality in the Seoul National University Hospital database
Supplementary Table 4
Sensitivity analysis for the risk of COVID-19 diagnosis, hospitalization and mortality in the Boramae Medical Center database
Fig. 1
Flowchart of the study population. (A) SNUH database; (B) BMC database.
SNUH = Seoul National University Hospital, BMC = Boramae Medical Center.
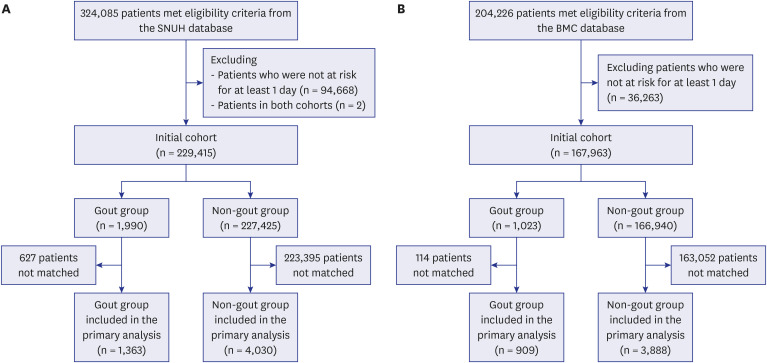
Fig. 2
Kaplan-Meier plots for the risk of coronavirus disease 2019 diagnosis in a 1:5 propensity score-matched cohort of individuals with gout and those without in the Boramae Medical Center database.
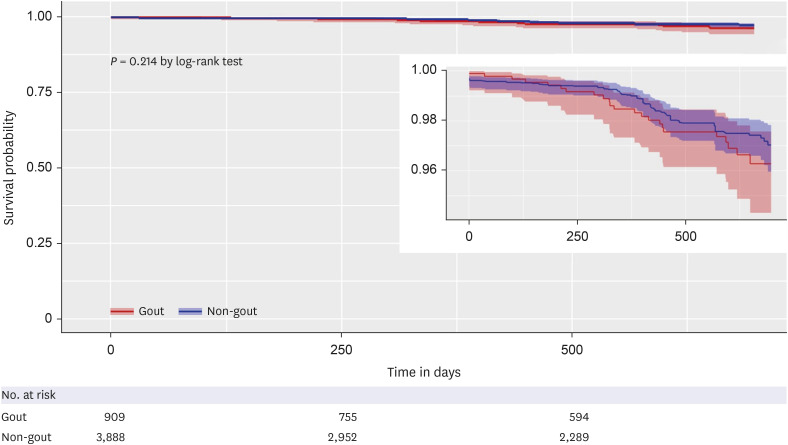
Table 1
Baseline characteristics of the study population in the Boramae Medical Center
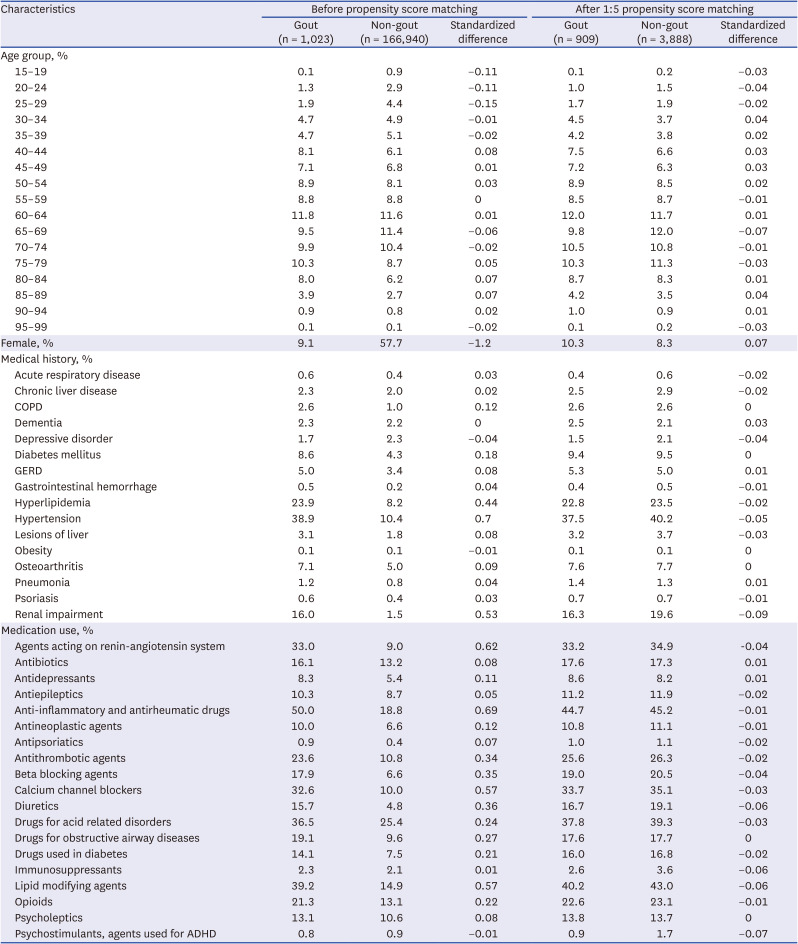